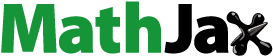
ABSTRACT
The American Statistical Association’s Symposium on Statistical Inference (SSI) included a session on how editorial practices should change in a universe no longer dominated by null hypothesis significance testing (NHST). The underlying assumptions were first, that NHST is problematic; and second, that editorial practices really should change. The present article is based on my talk in this session, and on these assumptions. Consistent with the spirit of the SSI, my focus is not on what reviewers and editors should not do (e.g., NHST) but rather on what they should do, with an emphasis on changes that are not obvious. The recommended changes include a wider consideration of the nature of the contribution than submitted manuscripts usually receive; a greater tolerance of ambiguity; more of an emphasis on the thinking and execution of the study, with a decreased emphasis on the findings; replacing NHST with the a priori procedure; and a call for reviewers and editors to recognize that there are many cases where the basic assumptions of inferential statistical procedures simply are not met, and that inferential statistics (even the a priori procedure) may consequently be inappropriate.
It is no secret that editorial practices can and should be improved. Some potential improvements are obvious. These include rendering data accessible for others to perform their own analyses, increased transparency, disclosure of conflicts of interests, and others. It is difficult to imagine that many researchers would disagree with these. However, there are other changes that, while not necessarily surprising, would less obviously elicit universal agreement, and might even elicit some disagreement. My aim is to introduce these less obvious changes and make the case that they nevertheless are worth discussing and possibly accepting.
1 More Consideration of the Nature of the Contribution
At first glance, this seems (a) obvious and (b) what editors and reviewers are already doing. Of course, editors and reviewers consider the nature of the contribution! But perhaps there can be more consideration with respect to the following issues.
The first issue is that there are many ways of contributing, including new theory, new application, integration, unification, new data, and others. Most journals tend to feature only two or three kinds of contributions, but editors and reviewers might be more open to contributions that are less traditional for the journal. Consider, for example, a contribution by Albert Einstein (related by Einstein Citation1961). Einstein presented a formula that describes the shrinkage of objects in their direction of motion, depending on their relative velocity.1 It is entertaining to imagine a hypothetical scenario where Einstein attempts to publish the formula in a journal whose editorial practices are those prevalent in contemporary academic journals. As Einstein admitted, there is a major potential problem with the formula, which is that Lorenz published the formula long before Einstein thought of it. As journals are looking for novel contributions, Einstein’s formula should be rejected, and would be rejected. Such are contemporary editorial practices.
But wait! There is a subtlety to consider. In addition to suggesting the formula and admitting that Lorenz got there first, Einstein also pointed out a major difference in the context of the formula, for himself and Lorenz. For Lorenz, the formula was a special hypothesis designed to save the data. In contrast, for Einstein, the formula was a derivation from a larger theory, relativity theory. Therefore, the formula was unifying in the Einstein context but was not unifying in the Lorenz context. From a unification perspective, the formula was an important contribution after all because it provided a demonstration of the ability of relativity theory to unify physics. This hardly can be considered trivial, and yet the formula would be difficult to publish under contemporary editorial practices (Trafimow and Rice Citation2009). Of course, not all reviewers would fail to recognize such contributions, but it would be a positive development if more reviewers and editors were open to subtle, but nevertheless important, contributions, such as those exemplified by the Einstein and Lorenz episode.
Another contribution issue pertains to whether the submission potentially benefits the journal, the area, the field, or all of science. To take an example from my own area of social psychology, and the journal I edit, Basic and Applied Social Psychology (BASP), consider the following items:
The submission contributes to BASP.
The submission contributes to the attitude area.
The submission contributes to the general field of social psychology.
The submission contributes to all of science.
Hopefully, the bullet-listed items go well together, and a submission would contribute to the journal, the area, the field, and perhaps even to all of science.
And yet, the bullet-listed items might not go together well. For example, as an editor, I have seen reviewers recommend the rejection of manuscripts based on perceptions of too much overlap with previous literature. From a journal-centric point of view, it seems sensible to recommend rejection to avoid using up valuable journal space on a manuscript that fails to contribute much to the journal because its components are not novel. But what if, despite the lack of novelty in the components, the manuscript nevertheless potentially contributes to the area, possibly by integrating the components in a way that suggests new implications? Or better yet, as in the Einstein example, there might be a unifying principle. In such cases, what seems best for the journal (from a narrow perspective) and what is best for science are not necessarily in complete accord. I urge reviewers and editors to place more weight on what is best for science, even if it comes at a seeming cost to what is best for the journal.
As a personal example of practicing what I am preaching, consider recent BASP editorials (Trafimow Citation2014; Trafimow and Marks Citation2015, Citation2016). These editorials have little to do with social psychology—the substantive topic of BASP—but have a lot to do with methodological and statistical practices. From a journal-centric perspective, I arguably should not have published the editorials,2 but a broader perspective suggests the opposite conclusion. In summary, there are many ways of contributing, and different levels and scopes of contributions; and reviewers and editors could make recommendations and decisions based on wider, more sophisticated, and more philosophically informed evaluation processes.
2 Tolerate Some Ambiguity
Having read countless reviews, little kills a submitted manuscript more assuredly than ambiguous findings. Reviewers see the ambiguities quickly, emphasize them in their reviews, and perforce recommend rejection. In turn, based on the negative reviewer recommendations, the editor inevitably decides to reject the manuscript. And the rejection makes sense. Why commit valuable journal pages to an article with ambiguous findings when the pages could be committed to an article that contains unambiguous findings? Nevertheless, I urge greater toleration of ambiguity.
Suppose that an author submits a manuscript, using a typical sample size, and the findings are either statistically significant or not. There are several issues with this scenario, to be addressed later, but one matter is particularly relevant to ambiguity tolerance. To see it, imagine that we had access to Laplace’s Demon, who knows everything, including whether the sample effect size is close to the population effect size. Let us further imagine that the Demon guarantees that the nonsignificant sample effect size is close to the population effect size; that is, the population effect size is small but greater than zero in the direction hypothesized by the researcher. Assuming all is well with respect to theory and experimental design, should the finding be published?
The question is a difficult one. On the positive side, the small population effect size is in the predicted direction, and consequently may support the theory (but see Trafimow Citation2017 for a potential discrepancy). On the negative side, the fact that the effect size is small renders the support unimpressive, as it is easier to predict or account for small than large effect sizes, ceteris paribus (all else being equal). With only a small effect size to account for, the reviewers and editor likely can come up with alternative explanations. A way out might be to simply ask the Demon if the theory is true, but the Demon refuses to impart more information. The upshot is that it really is not clear what to believe about the theory, and all we can be sure of is a small population effect size in the “right” direction. Despite the Demon’s valuable help in guaranteeing the population effect size, and even acknowledging the positive reason for publishing the manuscript, the case for acceptance is thus far weak.
But there is another consideration. The blunt fact of the matter is that most population effect sizes in research really are small, though it is not difficult to think of experiments that would result in large effect sizes. For a large effect size thought experiment, suppose participants in the experimental condition were instructed to write an X in the upper right-hand corner of their questionnaires and participants in the control condition were not. Doubtless, the population effect size would be gigantic (and the sample effect size too). The problem, of course, is that finding that participants can comply with a simple instruction would be of trivial importance at both the theoretical and applied levels. Because researchers wish to test interesting ideas, and interesting ideas, by their nature, engender some subtlety, the population effect sizes likely will be small. In addition, even well-tested dependent measures are not perfectly reliable and valid, and even well-tested manipulations likely do not take for every participant, thereby lowering the effect size still further from what it otherwise would be. Thus, although large effect sizes might be superior to small effect sizes, ceteris paribus; this ceteris paribus condition rarely applies. To really hammer home the general lack of applicability of the ceteris paribus condition, consider what some believe is the most important experiment in the history of science—the work by Michelson and Morley (Citation1887)—that failed to detect the existence of the hypothesized luminiferous ether that was thought to provide the medium by which light waves could propagate, and reach Earth from the stars. Today, scientists agree that there is no luminiferous ether, and so the population effect size is 0. But the population effect size of 0 does not detract from the extremely high value of the research. Small—or even zero—effect sizes can matter.3
The bottom line, then, is that some small effect sizes are very important. One should assess the theory, auxiliary assumptions connecting non-observational terms in the theory to observational terms in empirical hypotheses, quality of the experimental design, and implications for applications, as important components of one’s evaluation of a manuscript. Because most experimental predictions (e.g., in psychology, medicine, marketing, and so on) are directional rather than being point predictions and given the foregoing assertion that many interesting ideas involve small rather than large population effect sizes, it is an inevitability that there will be ambiguity. This is a fact of life in science, and reviewers, and editors would do well to admit it and tolerate the ambiguity that comes with it. When the sample effect size is small, reviewers and editors should nevertheless accept it, regardless of p-values, if it is of basic or applied importance and the sample size is large enough to engender confidence in its accuracy. Moreover, the present scenario dramatically underestimates the degree of ambiguity in normal science, where researchers do not have access to Laplace’s Demon. When addressing the normal science scenario in the next section, we will see yet more reason to tolerate ambiguity in the results, support for an increased focus on theoretical and design issues, and a decreased focus on how the results come out.
3 Emphasize Thinking and Execution, Not Results
Reviewers and editors often attend, insufficiently, to the fact that a p-value is a sample statistic.4 It is not difficult to imagine a scenario where the same experiment is performed repeatedly to obtain a distribution of p-values for that experiment. Of course, in normal science, the experiment is performed only once. But the value of imagining a p-value distribution is that it immediately becomes clear that the obtained p-value is only one of the many p-values that could have been obtained.
In the previous section, we imagined a small population effect size, and a small sample effect size that accurately estimates the small population effect size. But in real research, the sample effect size might be large, small, or even in the wrong direction. Unfortunately, science has been dominated by null hypothesis significance testing (NHST), that uses a threshold (usually 0.05) for statistical significance. If the sample effect size is sufficiently large to render a p-value coming in under 0.05, the manuscript is deemed publishable (if other aspects pass muster); otherwise, it is not. Because there is a distribution of sample effect sizes, and a distribution of associated p-values, it should be obvious that getting lucky (picking a large sample effect size and low p-value from the distributions of sample effect sizes and p-values) greatly aids in publishing one’s work. But as was made salient by a recent discussion in BASP (Hyman Citation2017; Kline Citation2017; Locascio Citation2017a, Citation2017b; Marks Citation2017), it should be clear that publishing predominantly the “lucky” findings results in a literature of published effect sizes that exceeds the effect sizes that would be obtained if all findings were published. Empirical support for such effect size overestimation was obtained by the Open Science Collaboration (Citation2015), who found that the average effect size in the original cohort of published articles was 0.403 whereas it was only 0.197 in the replication cohort. To avoid scientific literatures littered with overly optimistic sample effect sizes, Locascio suggested that publication decisions should be based on the worth of the theory and the execution of the experiment, rather than on the findings. Whether or not one is willing to go as far as Locascio wishes to go is a matter for serious discussion, but it is at least possible to move in that direction, which would be good for science.5
An additional way to address this problem is to emphasize replications. If a finding is lucky, it likely would not hold up when submitted to replication attempts, especially not multiple replication attempts. In contrast, greater trust can be placed in findings that do replicate. Some caveats are as follows. First, findings can be unlucky as well as lucky, and so even a correct finding might not always replicate when subjected to replication attempts. Second, it is possible for lucky events to happen again, which is a reason for multiple replication attempts rather than single replication attempts. Third, there may be outside reasons (e.g., lack of money, inaccessible participant populations, and so on) why replications are not feasible with respect to particular findings. Despite the caveats, replications are desirable when practical, though what is meant by replicability can be a complicated topic (see Trafimow Citation2018 for a discussion).
4 Replace NHST with A Priori Thinking
Invoking Laplace’s Demon again, suppose that the Demon warned us that our sample statistics would be completely unrepresentative of their corresponding population parameters. In that case, scientists likely would no longer be interested in sample statistics. Put another way, absent the Demon, the reason scientists are interested in sample statistics is because they believe that the sample statistics they intend to obtain will provide reasonable estimates of corresponding population parameters. In fact, researchers often are taught to collect the largest feasible sample size because it is well-known that, under the usual assumptions of random and independent sampling, the larger the sample, the more the sample resembles the population. The larger the sample, the more confident the researcher can be that the sample statistics to be obtained will be close to their corresponding population parameters. But if the goal is to be confident that the sample statistics will be close to their corresponding population parameters, it makes sense to ask: “How close do we want to be?” and “How confident do we want to be that we are that close?” Given specifications of closeness and confidence, it is possible to calculate the necessary sample size. It is worthwhile to pause for a moment to emphasize that the goal is not to obtain sufficient participants to obtain a p-value of a particular size, or a confidence interval of a particular width; but rather to obtain sample statistics that the researcher can be confident are close to their corresponding population parameters. A consequence of this subtle, but nevertheless important change from typical statistical thinking, is that the expected effect size plays no part in the calculations (Trafimow Citation2017; Trafimow and MacDonald (Citation2017)), as will become clear with the subsequent example.
Consider the extremely simple example where there is only one group, each participant is randomly and independently sampled from a normally distributed population, and the researcher is interested in the sample mean as an estimate of the population mean. Trafimow (Citation2017) provided an accessible proof of EquationEquation (1)(1)
(1) , where f is the fraction of a standard deviation defined as “close,” ZC
is the z-score that corresponds to the probability the researcher wants to have of being close (i.e., confidence), and n is the necessary sample size to meet the specifications for closeness and confidence:
(1)
(1)
To see the implications, suppose that the researcher wishes to have a 95% probability that the sample mean to be obtained will be within 0.2 standard deviations of the population mean. The z-score that corresponds to a 95% probability is 1.96, and so the result is as follows: Rounding to the nearest whole number, the researcher needs to collect 96 participants to have a 95% probability of obtaining a sample mean within 0.2 standard deviations of the corresponding population parameter. Note that the researcher does not need to know or guess the population mean, standard deviation, nor effect size to make the calculation. Also, if the sample size is not under the experimenter’s control, and hence is given, algebraic manipulation of EquationEquation (1)
(1)
(1) can be made to render the probability of obtaining a sample mean, given the sample size, within the desired distance of the population mean.
Thus, using the a priori procedure, the researcher makes specifications of closeness and confidence before collecting data, finds the necessary sample size, collects that sample size or a larger one, calculates the sample statistics of interest, and then comes the easy part. The researcher simply believes that the sample statistics accurately estimate the corresponding population parameters. The reason such belief is justified is that the researcher sets up the conditions, a priori, for such belief. Using a priori equations, of which EquationEquation (1)(1)
(1) is a simple example, costs practically nothing but can provide a useful way to decide on sample sizes. Or from the viewpoint of a reviewer or editor who is faced with already collected data, these equations can be used to estimate, in a posteriori fashion, how likely the sample statistics presented are to be good estimates of the corresponding population parameters at the desired level of precision.
Lest the a priori procedure be confused with traditional power analysis, two obvious differences are worth relating. First, the goal of traditional power analysis is to find the sample size needed so the researcher can have a good chance of obtaining a statistically significant finding. Regarding NHST, it is interesting to ponder the overwhelming tendency of speakers at the SSI (2017) to eschew it. But if we are to reject NHST, then power analysis designed to facilitate NHST does not make much sense either. In contrast, the goal of the a priori procedure is to find the sample size needed to obtain sample estimates of population parameters at specified levels of precision and confidence. Second, the effect size—or expected effect size—plays a crucial role for power analysis computations whereas it plays no role whatsoever for a priori procedure computations. Philosophically, this is because the goal is to obtain sample statistics that accurately estimate population parameters and not to obtain sufficiently low p-values to reject hypotheses. Mathematically, this is because the standard deviation cancels out in the derivation of a priori equations (see Trafimow Citation2017 for an accessible proof). Given the effect size lesson exemplified by the Michelson and Morley (Citation1887) experiment, it is a plus that the a priori procedure is uninfluenced by the effect size or expected effect size.
A final point with respect to the a priori procedure pertains to the replication crisis that has become such a concern as of late. Interestingly, this procedure suggests the possibility of computing the probability of replication, also in an a priori way, though under an assumption of ideal replication conditions. Imagine an ideal replication that is the same as the original experiment, with the sole exception of randomness. In that case, the probability that both replications will have sample statistics within the specified distances of the corresponding population parameters, at the sample size used, is simply the square of the probability that this will be so for one of the experiments (Trafimow Citation2018). A caveat, however, is that the probability of replication, when computed in this way, should be considered an ideal probability. In real science, the replication will not be exact (Hubbard Citation2016), and so the a priori probability of replication should be considered an upper bound (Trafimow Citation2018). But this is nevertheless useful. When confronted with a manuscript, reviewers and editors can run their own calculations using a priori equations. If even the ideal probability of replication is low (and it often is low at typical sample sizes), the reviewers and editors can be assured that the real probability of replication is even lower than that. And if probability of replication is important for the journal, a low ideal probability of replication might become a strong criterion for rejection. Going the other way, if the ideal probability of replication is impressive, there is at least some reason for optimism, and reviewers and editors can use it as a starting point for their subjective judgments of how likely the findings would be to replicate in the real scientific universe.
There is one last point to be made pertaining to the a priori procedure. EquationEquation (1)(1)
(1) provides the simplest possible case, but the simplicity of the example does not imply that the procedure will not work for more complex cases. On the contrary, Trafimow and MacDonald (Citation2017) expanded the procedure to work for however many groups the experimenter wishes to use. In addition, Trafimow, Wang, and Wang (Citation2018) have expanded the a priori procedure to apply to skewed distributions and to locations as well as means. Equations not yet published also have been invented concerned with estimating differences between means or locations as opposed to the means or locations themselves, and for estimating standard deviations, scales, and shape parameters.6 Work is in progress that pertains to the estimation of correlation coefficients, proportions, and others.7 Consequently, we expect that soon, researchers will be able to make a priori calculations for practically anything they find to be of interest, under a variety of possible assumptions. And, of course, reviewers and editors also will be able to make these calculations to aid in their manuscript evaluations.
5 The Assumptions of Random and Independent Sampling Might Be Wrong
Berk and Freedman (2003) argued that it is the rare study that uses sampling that is completely random and where each participant sampled is independent of the other participants sampled. Without making assertions about exactly how often these assumptions are importantly violated, there can be little doubt that the violation prevalence is considerable. In that case, no inferential statistical procedures, including even the a priori procedure discussed in the foregoing section, are completely valid. When no inferential statistical procedures are valid, reviewers and editors may be doing a disservice to science by either allowing, or even insisting on, researchers performing them. There are times when science is better served by reviewers and editors simply admitting that the assumptions of random and independent sampling are inapplicable. In those cases, an option is for authors to report only descriptive statistics, and reviewers and editors should be open to that.8 The notion that inferential statistical procedures may sometimes, and even often, be inappropriate, may be tough medicine for reviewers and editors to swallow. But the medicine nevertheless is therapeutic. Another option is to use methods for addressing violations of independence or random sampling (e.g., Liu and Singh Citation1995), while keeping in mind that these have their own assumptions and drawbacks.
That inferential statistical assumptions are practically never perfectly accurate is well-known, but perhaps the imperfections are addressed by the famous quotation by Box and Draper (Citation1987): “Essentially, all models are wrong, but some are useful” (p. 424). But at least with respect to p-values, this quotation does not work as well as some might hope. To see why, consider that if one admits that the model, including all assumptions, is never exactly right, then that means that the model is always wrong, though it may be close to right and may even be close enough to right to be useful. Well, then, if the model is known wrong as Box and Draper admit, why compute a p-value to obtain evidence against a known wrong model? The model is wrong no matter what p-value is obtained! Nor does the p-value give a valid indication of how close the model is to being right nor to how useful it is. Then, too, the p-value does not validly indicate the amount of evidence against the model being close to right, only against the model being exactly right.
The issue of not being exactly right is not necessarily as problematic with respect to alternative inferential procedures. For example, consider again the a priori procedure. Although the model again likely is not exactly right, remember that the goal is not to test a (known wrong) model but rather to obtain a sample size that engenders confidence that the sample statistics to be obtained are precise estimates of corresponding population parameters. Well, then, suppose that the model is close to being right, though it remains wrong. The slight wrongness of the model implies that a priori calculations will result in the researcher collecting a sample size that either is slightly insufficient to meet objectives or slightly in excess. In the latter case, little harm is done except that the researcher undergoes a bit more effort than is needed and enjoys the compensation of extra precision. In the former case, the researcher will have slightly too much confidence in the precision of the sample statistics. These problems need not be fatal, whereas for p-values, they are fatal as the known wrongness of the model really does render p-values pointless at best, and harmful at worst.
6 Conclusion
Like many people who work, academic researchers are interested in their careers. Because promotions in academia depend largely on publications, academic researchers are strongly motivated to publish. Thus, journal editors have much power. If journal editors insist on practices that are good for science, authors will comply, and science will benefit accordingly. In contrast, a failure to insist on practices that are good for science, and even an insistence on practices that are bad for science (such as NHST), not surprisingly work to the detriment of science. In some cases, it is obvious what constitute good or bad scientific practices, and in those cases, editors likely will insist on good ones. I know of no journal editors who would encourage scientific practices that they believe to be detrimental to science. But what is good or bad for science is not always obvious. The five recommendations that provided the present focus are some of them, though there are many more. In addition, however, consistent with the focus of the SSI session on journal editing, there is a general need to greatly expand the discussion of editorial issues. As academia becomes increasingly ruled by a publish-or-perish ethos; and journal editors consequently continue to gain in collective influence, though not necessarily in wisdom; it is increasingly vital to improve the evaluation procedures of reviewers and editors. Hopefully, the five present recommendations provide a useful continuation of the focus of the SSI session on editorial practices in a post p < 0.05 scientific universe.
Notes
1 , where L is the observed length, LO
is the proper length, v is the relative velocity between observer and moving object, and c is the speed of light.
2 In fact, the editorials worked out well for BASP, though I had no way to know this beforehand.
3 It is interesting that Carver (1933) reanalyzed the Michelson and Morley (Citation1887) data using the null hypothesis significance testing procedure. Carver obtained a statistically significant effect! Had Michelson and Morley performed a significance test, as journal editors would insist on today, they would have concluded that they had supported the existence of the luminiferous ether. The negative consequences for science, had this happened, are incalculable. For example, the equation in Footnote 1 is an outgrowth of Michelson and Morley’s disconfirmation of the luminiferous ether.
4 Although there are many criticisms of how researchers use p-values to perform null hypothesis significance tests, I call the reader’s attention to two recent books (Hubbard Citation2016; Ziliak and McCloskey Citation2016). Both are noteworthy because they are extremely readable, place the discussion in larger conceptual backgrounds than is typical, and provide rich contexts.
5 One way to deal with optimistic effect sizes statistically is to use regression equations to estimate the extent to which the effect size could be expected to shrink upon a replication attempt. A potential drawback, however, is that the researcher might not know the values to instantiate into the equations.
6 I take this opportunity to thank Tonghui Wang, Cong Wang, and Hunter Myüz for their high-quality help, without which these advances would not have been made.
7 I again thank Tonghui Wang, Cong Wang, and Hunter Myüz for their invaluable aid.
8 In general, whether inferential statistical procedures are valid or not, a strong case could be made for expanded descriptive statistics, possibly accompanied by improved visual displays. These points have been elaborated by Valentine, Aloe, and Lau (Citation2015).
References
- Berk, R. A., and Freedman, D. A. (2003), “Statistical Assumptions as Empirical Commitments.” in Law, Punishment, and Social Control: Essays in Honor of Sheldon Messinger, eds. T. G. Blomberg and S. Cohen (2nd ed), New York: Aldine de Gruyter, pp. 235–254.
- Box, G. E. P., and Draper, N. R. (1987), Empirical Model-Building and Response Surfaces, New York: Wiley.
- Carver, R. P. (1993), “The Case Against Statistical Signi?cance Testing, Revisited,” Journal of Experimental Education, 61, 287–292. DOI: 10.1080/00220973.1993.10806591.
- Einstein, A. (1961), Relativity: The Special and the General Theory, Robert W. Lawson, Trans., New York: Crown Publishers.
- Hubbard, R. (2016), Corrupt Research: The Case for Reconceptualizing Empirical Management and Social Science, Los Angeles, CA: Sage Publications.
- Hyman, M. (2017), “Can ‘Results Blind Manuscript Evaluation’ Assuage ‘Publication Bias’?” Basic and Applied Social Psychology, 39(5), 247–251. DOI: 10.1080/01973533.2017.1350581.
- Kline, R. (2017). “Comment on Locascio, Results Blind Science Publishing,” Basic and Applied Social Psychology, 39(5), 256–257. DOI: 10.1080/01973533.2017.1355308.
- Locascio, J. (2017a). “Results Blind Publishing,” Basic and Applied Social Psychology, 39(5), 239–246. DOI: 10.1080/01973533.2017.1336093.
- Locascio, J. (2017b), “Rejoinder to Responses to ‘Results Blind Publishing,”’ Basic and Applied Social Psychology, 9(5), 258–261. DOI: 10.1080/01973533.2017.1356305.
- Liu, R. Y., and Singh, K. (1995), “Using i.i.d, Bootstrap Inference for General non-i.i.d, Models,” Journal of Statistical Planning and Inference, 43, 67–75. DOI: 10.1016/0378-3758(94)00008-J.
- Marks, M. J. (2017), “Commentary on Locascio 2017,” Basic and Applied Social Psychology, 39(5), 252–253. DOI: 10.1080/01973533.2017.1350580.
- Michelson, A. A., and Morley, E. W. (1887), “On the Relative Motion of Earth and Luminiferous Ether,” American Journal of Science, Third Series, 34, 233–245, available at http://history.aip.org/exhibits/gap/PDF/michelson.pdf
- Open Science Collaboration (2015), “Estimating the Reproducibility of Psychological Science,” Science, 349, aac4716.
- Trafimow, D. (2014), “Editorial,” Basic and Applied Social Psychology, 36, 1–2. DOI: 10.1080/01973533.2014.865505.
- Trafimow, D. (2017), “Using the Coefficient of Confidence to Make the Philosophical Switch from a Posteriori to a priori Inferential Statistics,” Educational and Psychological Measurement, 77(5), 831–854. DOI: 10.1177/0013164416667977.
- Trafimow, D. (2018), “An a priori Solution to the Replication Crisis,” Philosophical Psychology, 31, 1188–1214. DOI: 10.1080/09515089.2018.1490707.
- Trafimow, D., and Marks, M. (2015), “Editorial,” Basic and Applied Social Psychology, 37, 1–2. DOI: 10.1080/01973533.2015.1012991.
- Trafimow, D., and Marks, M. (2016), “Editorial,” Basic and Applied Social Psychology, 38, 1–2. DOI: 10.1080/01973533.2016.1141030.
- Trafimow, D., and MacDonald, J. A. (2017), “Performing Inferential Statistics Prior to Data Collection,” Educational and Psychological Measurement, 77(2), 204–219. DOI: 10.1177/0013164416659745.
- Trafimow, D., and Rice, S. (2009), “What If Social Scientists Had Reviewed Great Scientific Works of the Past?” Perspectives in Psychological Science, 4, 65–78. DOI: 10.1111/j.1745-6924.2009.01107.x.
- Trafimow, D., Wang, T., and Wang, C. (2018), “From a Sampling Precision Perspective, Skewness is a Friend and Not an Enemy!” Educational and Psychological Measurement, 79, 129–150. DOI: 10.1177/0013164418764801.
- Valentine, J. C., Aloe, A. M., and Lau, T. S. (2015), “Life After NHST: How to Describe Your Data Without ‘p-ing’ Everywhere,” Basic and Applied Social Psychology, 37, 260–273. DOI: 10.1080/01973533.2015.1060240.
- Ziliak, S. T., and McCloskey, D. N. (2016), The Cult of Statistical Significance: How the Standard Error Costs us Jobs, Justice, and Lives, Ann Arbor, MI: The University of Michigan Press.