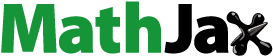
ABSTRACT
Researchers commonly use p-values to answer the question: How strongly does the evidence favor the alternative hypothesis relative to the null hypothesis? p-Values themselves do not directly answer this question and are often misinterpreted in ways that lead to overstating the evidence against the null hypothesis. Even in the “post p < 0.05 era,” however, it is quite possible that p-values will continue to be widely reported and used to assess the strength of evidence (if for no other reason than the widespread availability and use of statistical software that routinely produces p-values and thereby implicitly advocates for their use). If so, the potential for misinterpretation will persist. In this article, we recommend three practices that would help researchers more accurately interpret p-values. Each of the three recommended practices involves interpreting p-values in light of their corresponding “Bayes factor bound,” which is the largest odds in favor of the alternative hypothesis relative to the null hypothesis that is consistent with the observed data. The Bayes factor bound generally indicates that a given p-value provides weaker evidence against the null hypothesis than typically assumed. We therefore believe that our recommendations can guard against some of the most harmful p-value misinterpretations. In research communities that are deeply attached to reliance on “p < 0.05,” our recommendations will serve as initial steps away from this attachment. We emphasize that our recommendations are intended merely as initial, temporary steps and that many further steps will need to be taken to reach the ultimate destination: a holistic interpretation of statistical evidence that fully conforms to the principles laid out in the ASA statement on statistical significance and p-values.
1 Introduction
1.1 The Recommendations
Even as we begin to enter the “post p < 0.05 era,” we anticipate that p-values will continue to be widely reported. In statistical practice, perhaps the single biggest problem with p-values is that they are often misinterpreted in ways that lead to overstating the evidence against the null hypothesis. In this article, we recommend three practices, in increasing level of sophistication, that would help safeguard against such misinterpretation.
Recommendation 0.1: If using the current language of “statistical significance” for a novel discovery, replace the 0.05 threshold with 0.005. Refer to discoveries with a p-value between 0.05 and 0.005 as “suggestive,” rather than “significant.”
Recommendation 0.2: When reporting a p-value, p, in a test of the null hypothesis H0 versus an alternative H1, also report that the data-based odds of H1 being true to H0 being true are at most , where
is the natural logarithm and e is its constant base.
Recommendation 0.3: Determine and report your prior odds of H1 to H0 (i.e., the odds of the hypotheses being true prior to seeing the data), and derive and report the final (posterior) odds of H1 to H0, which are the prior odds multiplied by the data-based odds. Alternatively, report that the final (posterior) odds are at most the prior odds multiplied by .
Note that we label these recommendations 0.1, 0.2, and 0.3 to emphasize that each is a small step away from current practice and that, even taken all together, they do not bring us to the ultimate destination of the ideal “post p < 0.05 era.” In later sections, we discuss and motivate each of these recommendations, although reversing the order of the first two, for easier flow.
1.2 Context
After the influential ASA statement on statistical significance and p-values (Wasserstein and Lazar Citation2016) and the call for papers to air different perspectives on the “post p < 0.05 era,” we considered the possibility that science might not directly move to this new era that many would like to see. After all, major changes regarding significance testing have been repeatedly advocated for roughly 80 years, and it is not clear that this new initiative will succeed where others have failed.
In this context, our goal is to suggest minimal changes that would require little effort for the scientific community to implement. Motivating this goal are our hope that easy (but impactful) changes might be adopted and our worry that more complicated changes could be resisted simply because they are perceived to be too difficult for routine implementation.
For instance, we agree with the ASA statement (Wasserstein and Lazar Citation2016) that “Scientific conclusions and business or policy decisions should not be based only on whether a p-value passes a specific threshold.” Such dichotomization is often harmful because it obscures the uncertainty that is intrinsic to any statistical evidence (e.g., Amrhein and Greenland Citation2018; McShane and Gelman Citation2017). Moreover, use of a fixed level discourages consideration of crucial elements of the problem, such as the magnitude of the effect, costs of false positives and false negatives (or more generally the loss function in a decision), prior information, etc. (Lakens et al. 2018; Bayarri et al. Citation2016). Indeed, in our own statistical practice, we strive to fully use all such information. But we need to recognize the possibility that many (most?) investigators will simply find giving up on “statistical significance” too difficult and will end up staying with the current system.
We believe that our recommendations, if adopted, will move the scientific community toward the ideal “post p < 0.05 era”: if p < 0.05 no longer provides a license for treating a conclusion as “true,” then seriously thinking about other elements of the problem in the statistical analysis (e.g., transparency of statistical analysis and reporting) will become more appealing. We thus view the recommendations merely as initial, temporary steps on the way to the final destination, but steps that make significant progress immediately. Once our recommendations are adopted, we will advocate for further steps that move us yet closer to the ideal, holistic approach to assessing statistical evidence summarized in the ASA statement (Wasserstein and Lazar Citation2016).
Finally, note that our recommendations only address the “significance” part of the problem. As it is put in the ASA statement (Wasserstein and Lazar Citation2016): “A p-value, or statistical significance, does not measure the size of an effect or the importance of a result.” Assessing the magnitude of the effect (i.e., “practical significance”) is typically at least as important. We have our preferred methods for making such assessments, but we do not discuss them here because our focus in this article is on the use of p-values. Nonetheless, we repeat that it is essential to assess practical importance.
2 The Strength of Evidence Question (SOEQ)
Our recommendations are intended to help researchers, journals, and other parties more accurately answer the question they commonly use p-values to answer: How strongly does the evidence from the data favor the alternative hypothesis relative to the null hypothesis? We call this question the Strength of Evidence Question (SOEQ). For example, in an experiment, researchers want to know how strong the evidence from the data is that some treatment has an effect, as opposed to no effect.
Of the many misinterpretations of p-values (for some examples, see Goodman Citation2008; Greenland et al. Citation2016), some are attempts to use the p-value to answer the SOEQ. For example, one possible misunderstanding is that the p-value is the probability that the test statistic would have its observed value under the null hypothesis as opposed to the alternative hypothesis. Thus, a p-value of 0.05 is interpreted as meaning that the observed data only had a 5% chance of occurring if the null hypothesis were true but a 95% chance of occurring if the alternative hypothesis were true. Under this misinterpretation, p = 0.05 corresponds to odds of 19:1 in favor of the alternative hypothesis relative to the null hypothesis. This misinterpretation generates a perception that a p-value of 0.05 provides much greater evidence against the null hypothesis than it actually does. (As we explain below, p = 0.05 actually corresponds to odds of at most 2.44:1 in favor of the alternative hypothesis relative to the null hypothesis.)
The correct definition of the p-value is the probability, under the null hypothesis, of observing a test statistic as extreme or more extreme than its observed value. Thus, the p-value cannot provide a direct answer to the SOEQ for two reasons. First, the p-value is evaluated only under the null hypothesis and, hence, cannot say anything directly about the comparison between the null and alternative hypotheses. (For some purposes, this property may be touted as a strength of the p-value, but in terms of providing an answer to the SOEQ, it is not a strength.) Second, it is the probability that the test statistic is as extreme or more extreme than its observed value, whereas the SOEQ asks about the likelihood of the data that was obtained, not the likelihood of more extreme data that might have been obtained. In the above example of a misinterpretation, both of these reasons pertain. In our experience, each of these two reasons roughly equally affects the difficulty with the interpretation of p-values.
To a Bayesian, there is a straightforward, direct answer to the SOEQ. This answer is the Bayes factor, defined as
(The average in the numerator is taken with respect to an assumed prior distribution of parameter values under the alternative hypothesis. This statement of the definition assumes that the null hypothesis is simple, with the parameter equal to a specific value such as zero; otherwise, the denominator also needs to be an average.) The Bayes factor is the odds of observing the data under the alternative hypothesis to observing it under the null hypothesis. Traditionally, the Bayes factor has been considered unsatisfactory to frequentists because the “average likelihood” in the numerator of BF needs to be computed using some assumed prior distribution for the parameter values under the alternative hypothesis. However, we recently showed, in Bayarri et al. (Citation2016), that BF has a fully frequentist justification for many common situations involving testing a null hypothesis of zero effect versus an alternative hypothesis of nonzero effect. Thus, Bayesians and frequentists can unite in promoting BF as the correct tool for answering the SOEQ.
Compared with the p-value, however, the Bayes factor has two practical disadvantages. First, depending on the assumed prior distribution for the alternative hypothesis, the computation of the numerator of BF may not be as straightforward as running a prepackaged command. Second, specification of the prior distribution (or range of prior distributions) might lead to disagreements, or simply be viewed as too complex. Because of these disadvantages—together with the fact that Bayes factors are simply less familiar than p-values to most researchers—Bayes factors are unlikely to be adopted quickly for widespread use in answering the SOEQ. In the foreseeable future, it seems likely that researchers will continue misusing p-values for that purpose.
3 Converting p-Values Into Bayes Factors
To extract useful information about the SOEQ from a p-value, we would ideally translate a p-value into a Bayes factor. Unfortunately, there is no unique mapping between p-values and Bayes factors because, unlike calculating the p-value, calculating the Bayes factor requires specifying an alternative hypothesis (more specifically, a prior distribution for the parameter values under the alternative hypothesis).
Fortunately, several methods (Edwards, Lindman, and Savage Citation1963; Vovk Citation1993; Johnson Citation2013; Sellke, Bayarri, and Berger Citation2001) have been developed for using the p-value to calculate an upper bound on BF, called the Bayes factor bound, which we denote by BFB. The methods for calculating BFB differ from each other in terms of what they assume about the class of alternative hypotheses, but in many relevant applied settings, they generate similar values of BFB (Benjamin et al. 2018). The Bayes factor bound represents the strongest case for the alternative hypothesis relative to the null hypothesis: the highest possible BF consistent with the observed p-value. Because the Bayes factor is justifiable to both Bayesians and frequentists, the Bayes factor bound is, as well.
Held and Ott (Citation2018) provide practical advice about which Bayes factor bound to use depending on the context. For concreteness, we focus here on the simplest formula for calculating a Bayes factor bound
(1) The bound was originally derived by Vovk (Citation1993), and Sellke, Bayarri, and Berger (Citation2001) showed that it holds under quite general conditions. This value of BFB can be straightforwardly calculated as a simple function of the p-value, using only the natural logarithm and its base, the constant e. Calculating BFB does not require specifying an alternative hypothesis because it is an upper bound across a large class of reasonable alternative hypotheses.
The following table shows the value of BFB for a wide range of p-values. For those who are more comfortable with posterior probabilities than odds, the table also gives the corresponding upper bound on the posterior probability of H1. When the prior probabilities of H0 and H1 are equal, this upper bound on the posterior probability of H1 is given by .
Table
These calculations illustrate how p-values often point to much weaker evidence against the null hypothesis than researchers typically assume. Indeed, results that just reach conventional levels of significance do not actually provide very strong evidence against the null hypothesis. For example, a p-value of 0.05 corresponds to a Bayes factor of at most 2.44:1. That is, the data imply odds in favor of the alternative hypothesis relative to the null hypothesis of at most 2.44 to 1. So if the null and alternative hypotheses were originally equally likely, there remains, at least, a 29% chance that the null hypothesis is true. A p-value of 0.01—often considered “highly significant”—corresponds to at most 8.13 to 1 odds, hardly overwhelmingly convincing odds. In this case, upon starting from equally likely null and alternative hypotheses, there remains at least an 11% chance that the null hypothesis is true.
4 Justification of the Recommendations
The three recommended practices all involve interpreting p-values in light of their corresponding BFB. Doing so provides some safeguard against overestimating the strength of evidence from the p-value.
4.1 Recommendation 0.2
Recommendation 0.2 codifies the idea of converting a p-value into interpretable odds. To be concrete, in place of the current practice of reporting “p = 0.05,” we advocate reporting “p = 0.05, BFB = 2.44.” Since BFB indicates the strongest potentially justifiable inference from the data, reporting it would alert researchers when seemingly strong evidence is actually not very compelling. Its use would therefore help prevent researchers from being misled into concluding too much from the p-value of a finding.
Relative to the crude Recommendation 0.1, Recommendation 0.2 has the virtue of moving beyond a bright-line threshold and interpreting the p-value, correctly, as a continuous variable. Thus, under Recommendation 0.2 (and the follow-on Recommendation 0.3), the language of “significant” and “suggestive” can be dispensed with altogether.
Interestingly, although BFB is only an upper bound on the Bayes factor, we report evidence in Bayarri et al. (Citation2016) that, when calculated from real data from a range of scientific fields, BFB is often not that far from the BF implied by a scientifically reasonable alternative hypothesis.
4.2 Recommendation 0.1
Recommendation 0.1 is a crude follow-up to the idea of interpreting a p-value in terms of its corresponding BFB. The recommendation is that if—despite its inappropriateness—a researcher, journal, or other party insists on using a threshold for “statistical significance” for assessing the credibility of a novel discovery, using 0.005 as that threshold is better than using 0.05. The argument against a 0.05 threshold is clear from the table above: a p-value of 0.05 corresponds to odds of at most 2.44 to 1 against the null hypothesis, which is fairly weak evidence. If researchers treat findings with p-values just below 0.05 as having been established, then many “established” findings will turn out to be false positives and will fail to replicate. This is a purely statistical fact; it will be true even if the original study had no other problems (such as poor study design or multiple hypothesis testing) that could lead to false positives. We believe that this statistical fact has contributed to the alarmingly high levels of nonreplicability that have been documented in several research communities (e.g., Open Science Collaboration Citation2015; Camerer et al. Citation2016; Johnson et al. Citation2017). It should be emphasized that we are discussing evidence for novel discoveries here; odds of 2.44 to 1 may be adequate for other purposes, such as replicating a previous finding.
While any threshold is necessarily arbitrary, the table above shows that a p-value of 0.005 can correspond to much stronger evidence, with odds of up to 13.9 to 1 against the null hypothesis. We suspect that for most researchers, the term “statistical significance” connotes odds of this order of magnitude. Thus, the redefinition to a threshold of 0.005 would align the term’s actual meaning with how it is interpreted. Relabeling findings with p-values between 0.05 and 0.005 as “suggestive” would similarly communicate more accurately the answer to the SOEQ implied by a p-value in that range. The recommendation to redefine statistical significance to the 0.005 threshold has been made several times previously (e.g., Greenwald et al. Citation1996; Johnson Citation2013), and we recently joined many other researchers in endorsing it (Benjamin et al. 2018).
We leave it up to the authors and readers of papers to judge whether a finding is a “novel discovery,” just as such judgment is left up to the authors and readers of papers today. Researchers have an incentive to claim novelty if they can do so credibly, since novelty is (appropriately) rewarded by the scientific community. Recommendation 0.1, however, would imply that such claims must be accompanied by a stricter threshold for describing findings as “significant.”
In Benjamin et al. (2018), we responded to four potential objections to Recommendation 0.1. Here, we briefly summarize one of these and our response to it. The objection is that changing the significance threshold from 0.05 to 0.005 would cause an unacceptable increase in the rate of false negatives. Our response is 2-fold. First, failing to reject the null hypothesis does not mean accepting the null hypothesis. Indeed, relabeling p-values between 0.05 and 0.005 as “suggestive” provides a middle ground between acceptance and rejection. Second, the false negative rate will not increase if sample sizes are increased so that statistical power is held constant. For a wide range of common statistical tests, holding statistical power constant would require an increase in sample sizes of roughly 70%. Such an increase is not trivial, but it is achievable in many domains of research, and we believe that the gains in credibility of results are worth the cost.
We caution, however, that even a p-value of 0.005 does not ensure that the evidence against the null hypothesis is strong. The corresponding values of BFB are upper bounds, and the true strength of evidence will sometimes be much weaker. (This would occur if the observed evidence is not only highly inconsistent with the null hypothesis but also highly inconsistent with reasonable alternative hypotheses.) Nonetheless, Recommendation 0.1 would guard against weak evidence being mistaken for strong evidence.
Although we have put forth Recommendation 0.1, we strongly discourage its long-term and widespread use. We fully agree with the ASA statement on statistical significance and p-values (Wasserstein and Lazar Citation2016) that “Scientific conclusions and business or policy decisions should not be based only on whether a p-value passes a specific threshold.” Recommendation 0.1 should be adopted only temporarily and only in those research fields in which access to statistical expertise is insufficient to implement Recommendations 0.2 or 0.3 (or approaches beyond Recommendation 0.3).
4.3 Recommendation 0.3
While researchers often ask themselves the SOEQ, the question they really want to answer is a Bayesian one: How likely is the alternative hypothesis relative to the null hypothesis? We call this the More Likely Hypothesis Question (MLHQ). In a typical experiment, this question is: how likely is it that there is truly an effect of the treatment, as opposed to no effect? This is the crucial question for understanding what we should conclude from the results of a research study. For instance, does p = 0.01 really mean the same thing in a study of a new medical treatment, a test of whether a particular gene is related to a particular disease, and an investigation of extrasensory perception? Clearly, the answer to the MLHQ should depend on the prior odds of the alternative hypothesis relative to the null hypothesis. While it might be reasonable to assign prior odds of 1 to 1 for the medical treatment, prior odds for genetic studies are often chosen to be 1 to 100,000 (Wellcome Trust Case Control Consortium, Citation2007), and prior odds for extrasensory perception might be extremely small for most scientists.
To a Bayesian, the MLHQ can be answered straightforwardly—without any need for a p-value—by multiplying the Bayes factor by the prior odds, yielding what we have called the “post-experimental odds” (Bayarri et al. Citation2016). To be concrete about Recommendation 0.3 in cases where the Bayes factor has been calculated, analyses should include a statement such as “the Bayes factor of 4:1, when combined with our prior odds of 1:2, implies post-experimental odds of in favor of the alternative hypothesis.”
Recommendation 0.3 also accommodates cases where, instead of the Bayes factor, only a p-value is available. Then, according to our Recommendation 0.3, analyses should include a statement such as “the p-value of 0.05 corresponds to the upper bound on the Bayes factor of 2.44 which, combined with our prior odds of 1:2, implies post-experimental odds of at most in favor of the alternative hypothesis.”
The prior odds can be informed by researchers’ beliefs, scientific consensus, and evidence from related research questions. See, for example, Dias, Morton, and Quigley (Citation2018) for a review of methods for eliciting subjective prior odds, Dreber et al. (Citation2015) for an estimate of prior odds based on researchers’ beliefs elicited using prediction markets, and Johnson (2013) for an estimate of prior odds based on evidence from replication studies. If there is a range of prior odds, then the corresponding range of post-experimental odds should be calculated and presented. The prior odds should be justified by the researchers, and this justification should be critically evaluated by a paper’s referees and readers. To ensure that the prior odds are chosen before the data are seen, it should be expected that the prior odds and their justification be pre-registered.
In Recommendation 0.1, the threshold of 0.005 was stated to apply to “novel discoveries.” The intent of this language was to highlight “novel” situations where the prior odds of a discovery are no higher that 1 to 1. Then the post-experimental odds of a discovery can be no higher than the corresponding BFB and, hence, no larger than 13.9 to 1 for a p-value equal to this threshold of 0.005. In some scenarios—such as replicated studies or Phase III clinical trials (where there is considerable prior evidence available from Phase I and Phase II trials)—the prior odds in favor of the alternative hypothesis can be considerably larger than 1 to 1. In general, in such situations, it is strongly preferable to combine the information from all studies on a topic (e.g., via meta-analysis) and assess the evidence as a whole.
We put Recommendation 0.3 last because we understand that being fully transparent about prior beliefs is not part of the current scientific culture. Nonetheless, we believe it should become part of the culture in the “post p < 0.05 era” because the prior odds can matter greatly in evaluating the odds of an alternative hypothesis relative to the null hypothesis (see, e.g., Nuzzo Citation2014). Note that, if Recommendations 0.2 and 0.3 are adopted, there is no need to worry about whether the discovery is “novel” or not; the issue of its prior probability will be addressed directly.
5 Summary
All three of our recommendations involve interpreting a p-value in terms of its corresponding Bayes factor bound BFB. BFB has both Bayesian and frequentist justification, and it is as simple to calculate as the p-value. Moreover, it has a straightforward interpretation as an answer to the SOEQ, which we believe is the question that researchers often aim to answer when they report p-values. While we would encourage researchers to adopt all our recommendations, we have purposely given a hierarchy of recommendations according to simplicity, to pre-empt the claim that it is too difficult to change current practice.
In addition, we admit to having an ulterior motive in promoting use of the Bayes factor bound. If our recommendations are adopted, they may have the useful side effect of making researchers more comfortable with Bayesian tools and ideas, in particular the Bayes factor and prior odds. We hope that this greater familiarity and comfort could help facilitate interest in a much wider range of useful statistical tools that go beyond p-values.
Indeed, regardless of which recommendation is initially adopted in a particular field, we strongly urge continued and rapid progress toward adopting each higher numbered recommendation and then beyond, toward a holistic interpretation of statistical evidence that fully conforms to the principles laid out in the ASA statement on statistical significance and p-values (Wasserstein and Lazar Citation2016).
5 Acknowledgments
The authors thank the referees, associate editor, and especially the editor, Allen Schirm, for extensive and helpful comments that considerably improved the article.
Additional information
Funding
References
- Amrhein, V., and Greenland, S. (2018), “Remove, Rather Than Redefine, Statistical Significance,” Nature Human Behaviour, 2, 4. DOI: 10.1038/s41562-017-0224-0.
- Bayarri, M. J., Benjamin, D., Berger, J., and Sellke, T. (2016), “Rejection Odds and Rejection Ratios: A Proposal for Statistical Practice in Testing Hypotheses,” Journal of Mathematical Psychology, 72, 90–103. DOI: 10.1016/j.jmp.2015.12.007.
- Benjamin, D., Berger, J., Johannesson, M., Nosek, B. A., Wagenmakers, E.-J., Berk, R., Bollen, K. A., Brembs, B., Brown, L., Camerer, C., Cesarini, D., Chambers, C. D., Clyde, M., Cook, T. D., De Boeck, P., Dienes, Z., Dreber, A., Easwaran, K., Eferson, C., Fehr, E., Fidler, F., Field, A. P., Forster, M., George, E. I., Gonzalez, R., Goodman, S., Green, E., Green, D. P., Greenwald, A., Hadfeld, J. D., Hedges, L. V., Held, L., Ho, T. H., Hoijtink, H., Hruschka, D. J., Imai, K., Imbens, G., Ioannidis, J. P. A., Jeon, M., Jones, J. H., Kirchler, M., Laibson, D., List, J., Little, R., Lupia, A, Machery, E., Maxwell, S. E., McCarthy, M., Moore, D., Morgan, S. L., Munafó, M., Nakagawa, S., Nyhan, B., Parker, T. H., Pericchi, L., Perugini, M., Rouder, J., Rousseau, J., Savalei, V., Schönbrodt, F. D., Sellke, T., Sinclair, B., Tingley, D., Van Zandt, T., Vazire, S., Watts, D. J., Winship, C., Wolpert, R. L., Xie, Y., Young, C., Zinman, J., and Johnson, V. E. (2018), “Redefine Statistical Significance,” Nature Human Behaviour, 2, 6–10. DOI: 10.1038/s41562-017-0189-z.
- Camerer, C. F., Dreber, A., Forsell, E., Ho, T.-H., Huber, J., Johannesson, M., Kirchler, M., Almenberg, J., Altmejd, A., Chan, T., Heikensten, E., Holzmeister, F., Imai, T., Isaksson, S., Nave, G., Pfeiffer, T., Razen, M., and Wu, H. (2016), “Evaluating Replicability of Laboratory Experiments in Economics,” Science, 351, 1433–1436. DOI: 10.1126/science.aaf0918.
- Dias, L. C., Morton, A., and Quigley, J. (2018), Elicitation: The Science and Art of Structuring Judgement, Cham: Springer.
- Dreber, A., Pfeiffer, T., Almenberg, J., Isaksson, S., Wilson, B., Chen, Y., Nosek, B. A., and Johannesson, M. (2015), “Using Prediction Markets to Estimate the Reproducibility of Scientific Research,” Proceedings of the National Academy of Sciences, 112, 15343–15347. DOI: 10.1073/pnas.1516179112.
- Edwards, W., Lindman, H., and Savage, L. (1963), “Bayesian Statistical Inference for Psychological Research,” Psychological Review, 70, 193–242. DOI: 10.1037/h0044139.
- Goodman, S. (2008), “A Dirty Dozen: Twelve P-Value Misconceptions,” Seminars in Hematology, 45, 135–140. DOI: 10.1053/j.seminhematol.2008.04.003.
- Greenland, S., Senn, S., Rothman, K., Carlin, J., Poole, C., Goodman, S., and Altman, D. (2016), “Statistical Tests, P Values, Confidence Intervals, and Power: A Guide to Misinterpretations,” European Journal of Epidemiology, 31, 337–350. DOI: 10.1007/s10654-016-0149-3.
- Greenwald, A. G., Gonzales, R., Harris, R. J., and Guthrie, D. (1996), “Effect Sizes and p Values: What Should Be Reported and What Should Be Replicated?,” Psychophysiology, 33, 175–183.
- Held, L., and Ott, M. (2018), “On P-Values and Bayes Factors,” Annual Review of Statistics and Its Application, 5, 393–419. DOI: 10.1146/annurev-statistics-031017-100307.
- Johnson, V. (2013), “Revised Standards for Statistical Evidence,” Proceedings of the National Academy of Sciences, 110, 19313–19317. DOI: 10.1073/pnas.1313476110.
- Johnson, V., Payne, R., Wang, T., Mandal, S., and Asher, A. (2017), “On the Reproducibility of Psychological Science,” Journal of the American Statistical Association, 112, 1–10. DOI: 10.1080/01621459.2016.1240079.
- Lakens, D., Adolfi, F. G., Albers, C. J., Anvari, F., Apps, M. A. J., Argamon, S. E., Baguley, T., Becker, R. B., Benning, S. D., Bradford, D. E., Buchanan, E. M., Caldwell, A. R., van Calster, B., Carlsson, R., Chin Chen, S., Chung, B., Colling, L. J., Collins, G. S., Crook, Z., Cross, E. S., Daniels, S., Danielsson, H., DeBruine, L., Dunleavy, D. J., Earp, B. D., Feist, M. I., Ferrell, J. D., Field, J. G., Fox, N. W., Friesen, A., Gomes, C., Gonzalez-Marquez, M., Grange, J. A., Grieve, A. P., Guggenberger, R., Grist, J., van Harmelen, A.-L., Hasselman, F., Hochard, K. D., Hoffarth, M. R., Holmes, N. P., Ingre, M., Isager, P. M., Isotalus, H. K., Johansson, C., Juszczyk, K., Kenny, D. A., Khalil, A. A., Konat, B., Lao, J., Larsen, E. G., Lodder, G. M. A., Lukavský, J., Madan, C. R., Manheim, D., Martin, S. R., Martin, A. E., Mayo, D. G., McCarthy, R. J., McConway, K., McFarland, C., Nio, A. Q. X., Nilsonne, G., Lino de Oliveira, C., Orban de Xivry, J.-J., Parsons, S., Pfuhl, G., Quinn, K. A., Sakon, J. J., Saribay, S. A., Schneider, I. K., Selvaraju, M., Sjoerds, Z., Smith, S. G., Smit, T., Spies, J. R., Sreekumar, V., Steltenpohl, C. N., Stenhouse, N., Świ@@@@atkowski, W., Vadillo, M. A., Van Assen, M. A. L. M., Williams, M. N., Williams, S. E., Williams, D. R., Yarkoni, T., Ziano, I., and Zwaan, R. A. (2018), “Justify Your Alpha,” Nature Human Behaviour, 2, 168–171. DOI: 10.1038/s41562-018-0311-x.
- McShane, B. B. and Gelman, A. (2017), “Abandon Statistical Significance,” Nature, 551(7682), 582.
- Nuzzo, R. (2014), “Scientific Method: Statistical Errors,” Nature News, 506, 150. DOI: 10.1038/506150a.
- Open Science Collaboration (2015), “Estimating the Reproducibility of Psychological Science,” Science, 349, aac4716.
- Sellke, T., Bayarri, M. J., and Berger, J. O. (2001), “Calibration of p Values for Testing Precise Null Hypotheses,” The American Statistician, 55, 62–71. DOI: 10.1198/000313001300339950.
- Vovk, V. G. (1993), “A Logic of Probability, With Application to the Foundations of Statistics,” Journal of the Royal Statistical Society, Series B, 55, 317–351. DOI: 10.1111/j.2517-6161.1993.tb01904.x.
- Wasserstein, R., and Lazar, N (2016), “The ASA’s Statement on p-Values: Context, Process, and Purpose,” The American Statistician, 70, 129–133. DOI: 10.1080/00031305.2016.1154108.
- Wellcome Trust Case Control Consortium (2007), “Genome-Wide Association Study of 14,000 Cases of Seven Common Diseases and 3,000 Shared Controls,” Nature, 447, 661–678.