Abstract
In this article we draw on the power of geographic information systems (GIS) to examine the progression of HIV/AIDS in Africa for the period from 1986 to 2003. We use GIS for two purposes: (1) to transform and interpolate a set of annual point-based HIV/AIDS rates into area data; and (2) to extract or “mine” annual HIV/AIDS prevalence rates from the interpolated area (country) level maps. Once the rates are extracted from the GIS analysis we then model and forecast them into the near future (i.e., 2004–2010) by using the UNAIDS Estimation and Projection Package (EPP) software. The article offers an alternative grounded in geospatial analysis for yielding estimates of HIV/AIDS rates in Africa. Using incomplete sets of data for the period from 1986 to 2003, mean HIV/AIDS rates are generated from spatially interpolated data and future trends are estimated. The results indicate that the HIV/AIDS epidemic for many countries in Africa has reached the saturation or maturity level as evidenced by the typical S-shaped curves in the trends over time. As a matter of fact, some countries have begun to experience a sustained decline in the rates (e.g., Uganda, Burundi, Rwanda, and Zimbabwe).
En este artículo utilizamos el poder de los sistemas de información geográfica (SIG) para examinar el avance del VIH/SIDA en África en el periodo de 1986 a 2003. El SIG fue utilizado con dos propósitos: (1) transformar e interpolar en datos del área un conjunto de tasas anuales de VIH/SIDA basado en puntos; y (2) extraer o “minar” de los mapas de nivel del área interpolada (páıs) las tasas anuales de predominancia de VIH/SIDA. Una vez extraídas las tasas del análisis GIS, las modelamos y pronosticamos para el futuro cercano (esto es, 2004-2010) usando el programa Paquete de Cálculo y Proyección (Estimation and Projection Package) de UNAIDS. En el artículo se ofrece una alternativa basada en el análisis geoespacial para obtener cálculosaproximados de las tasas de VIH/SIDA en África. Mediante el uso de conjuntos incompletes de datos del período comprendido entre 1986 y 2003, se generan tasas medias de VIH/SIDA a partir de datos espacialmente interpolados, y se calculan aproximadamente las tendencias futuras. Los resultados indican que en muchos pá ses de África la epidemia de VIH/SIDA ha alcanzado el nivel de saturación o madurez, como lo demuestran las curvas típicas en forma de S de las tendencias a través del tiempo. De hecho, algunos páıses han comenzado a experimentar una disminución sostenida de las tasas (por ejemplo, Uganda, Burundi, Ruanda y Zimbabue).
AIDS as a relatively new disease has caused unspeakable distress to millions of people throughout the world, in sub-Saharan Africa more than anywhere else. More than twenty-five years have elapsed since the onset of this epidemic in Africa. Recent figures from UNAIDS (2005) are overwhelming. Easily the world's worst current pandemic, HIV/AIDS continues to decimate humanity, with sub-Saharan Africa being the worst hit. UNAIDS estimates for sub-Saharan Africa, which has just over 10 percent of the world's population, indicate that the continent had more than 60 percent of all the people living with HIV/AIDS, estimated at between 23.4 million and 28.4 million in 2004 (UNAIDS 2004). Similarly the nine countries of the southern Africa region constitute only 2 percent of the world population, yet globally 30 percent of people living with HIV/AIDS are concentrated in these countries (10.5-12.6 million).
CitationBrunn (1992), in an editorial of the Annals of the Association of American Geographers, put out a clarion call for geographers to focus their efforts on important pressing issues, among them HIV/AIDS, an area that had a big gap in geographic literature. At around the same time, a few geographers interested in the geography of health care and disease began to join in the research to shed light on the diffusion processes of HIV/AIDS in time and space. Smallman-Raynor, Cliff, and Haggett (1992) produced an impressive International Atlas of AIDS. In this atlas there were several comprehensively written chapters on the diffusion of HIV/AIDS in sub-Saharan Africa. In these chapters, scholars advanced and attempted to test three important hypotheses to explain the diffusion process of this disease: the truck town hypothesis, in which major roads were the principal corridors of virus spread; the international and local migrant labor hypothesis; and the civil war hypothesis.
In the late 1980s, North American geographers had already begun to produce a number of influential articles and books. CitationWood (1988) offered a valuable summary of major trends and implications of the AIDS epidemic on a global basis and CitationGould (1989) examined the geographic dimensions of the AIDS epidemic within the continental United States. CitationGould (1989) argued that to understand an epidemic, the geographic perspective is crucial because the “where” of an epidemic is as important as the “when.” Perhaps the most controversial and commented on article was that by CitationShannon and Pyle (1989) titled “The Origin and Diffusion of AIDS: A View from Medical Geography.” The article made the controversial claim that HIV/AIDS originated from somewhere in central Africa and diffused to the rest of the world via Haiti to North America and directly to Europe. In spite of the criticism of their initial article (see CitationNewman 1990; CitationWatts, Okello, and Watts 1990), Shannon, Pyle, and Bashshur (1991) soon released a book titled The Geography of AIDS: Origins and Course of an Epidemic, in which they expanded on their earlier article in the Annals of the Association of American Geographers. However, this time around they admitted the limitations of their work. Gould also expanded his 1989 article into a book titled The Slow Plague: A Geography of the AIDS Epidemic (CitationGould 1993). In this book he examined the dynamics of HIV/AIDS at the global level, highlighting the geography and devastating consequences this disease was having on humanity.
Since then quite a few geographers have taken up the mantle to examine the progression and trajectories of this disease in recent times. Of importance in this work has been an examination of the factors that result in people's vulnerability to the disease using the political economy or structuralist theoretical perspective (see, for example, CitationOppong 1998; CitationYoung and Ansell 2003; Kalipeni et al. 2004). Other geographers have joined the modeling group to examine the diffusion of the disease in geographic space and time using recent advances in techniques and geographic tools, thereby adding a much needed geographic perspective to the plethora of literature coming from the biomedical and epidemiological sciences about this disease. The works of Löytönen (1991, 1994), CitationGould and Wallace (1994), CitationLöytönen and Arbona (1996), CitationLöytönen and Maasilta (1997), CitationCochrane (2000), and CitationLam and Liu (1996) stand out in this regard. CitationLöytönen (1991) and CitationLöytönen and Arbona (1996), for example, have used S-shaped growth curves to model and forecast the progression of HIV/AIDS in Finland and Puerto Rico, respectively. We see the analysis in this article as contributing significantly to the growing modeling and forecasting literature as far as this disease is concerned with reference to sub-Saharan Africa.
Although UNAIDS and other agencies have developed sophisticated methods to estimate the progress of the epidemic, attempting to estimate and forecast its future trends has proven to be problematic due to lack of pertinent data. However, sentinel surveillance data for selected HIV testing sites throughout the African continent have been collected, although haphazardly, to provide estimates of prevalence rates (see, for example, the U.S. Census Bureau 2003). As the U.S. Census Bureau (2003) notes, information on the AIDS pandemic and on HIV seroprevalence (infection) in population groups in developing countries is only available in scattered small-scale surveys. HIV prevalence data are often insufficient to allow for reliable forecasts. Hence UNAIDS, the World Health Organization (WHO), and other agencies rely on the sentinel surveillance data collected mostly from pregnant women attending prenatal clinics both to gauge HIV trends and prevalence levels over time (WHO and UNAIDS 2003).
This is where the discipline of geography can contribute significantly in generating missing data using geographic information systems (GIS). GIS and remote sensing from earth-observing satellites are sophisticated and powerful technologies for geospatial analysis (GSA) that are finding applications far beyond those originally intended (WHO 2005). Over the past two decades the development of computer-based GIS has enabled researchers in geography and other related disciplines to manage and analyze a plethora of spatially referenced data to evaluate spatial processes and patterns and to display the results of such analyses (see CitationGolub, Gorr, and Gould 1993; CitationLiverman et al. 1998). GIS and remote sensing have become vital tools in public health analysis (e.g., CitationGatrell and Löytönen 1998; CitationCromley and McLafferty 2002).
The aim of this article is to offer an alternative method that utilizes sentinel data from HIV/AIDS testing centers to derive interpolated rates over space and time and show spatial-temporal trends using the powerful tool of GIScience and to use the interpolated rates to predict future HIV prevalence. Using the Spatial Analyst module in ArcGIS 9.1 we generate rates for each single year from 1986 to 2003 (ESRI 2005). These rates are then entered into the UNAIDS Estimation and Projection Package (EPP) to smooth the GIS-generated HIV/AIDS prevalence rates by fitting curves and then projecting the rates from 2004 to 2010. Using the smoothed rates, the number of people living with HIV for the years 2004 through 2010 is then automatically estimated by EPP. In short, this article should be read as a contribution to the methodologies currently being developed to predict the future trends of the epidemic as an important step toward understanding likely HIV/AIDS implications in the future, and for planning and implementing relevant interventions in a timely fashion in the specific context of incomplete and irregular data.
Geospatial Data Analysis: Inverse Distance Weighted Algorithm versus Kriging
Spatial analysis with GIS was used to generate continuous surface data from point data of HIV infection rates for the entire African continent. The data were provided by Centra Technology, Inc. (http://www.centrava.com/centratechnology.cfm).Footnote 1 Centra Technology obtained the original data set from the U.S. Census Bureau HIV Surveillance Database (see U.S. Census Bureau 2003). The U.S. Census Bureau compiled the data set from those studies appearing in the medical and scientific literature, presented at international conferences, data reported to UNAIDS by governments and also those appearing in the press. Furthermore the data in the data set contained both variants of HIV rates: HIV-1 largely concentrated in eastern, central, and southern Africa and HIV-2 found in parts of West Africa. Thus the analysis in this study is based on prevalence rates without distinction as to whether they relate to HIV-1 or HIV-2. Given the multiplicity of sources that were used in building this data set by the U.S. Census Bureau, the quality of the data is varied and needs to be used with caution. In many cases the surveillance data were restricted to pregnant women at prenatal clinics whose service area was not geographically defined. Thus if 500 pregnant women visiting a clinic in a given year were tested for HIV and 150 of them tested positive for the virus, the rate for all adults attending that clinic would be recorded as 30 percent. Centra Technology geocoded the location of the HIV surveillance centers by adding the geographic latitude and longitude coordinates. For the period from 1985 to 2003, the Africa-wide annual HIV rates included data from 1,442 sentinel sites. shows the distribution of the surveillance points. Although the number of centers generally increased over time, reporting on a year-by-year basis was erratic and irregular, hence the variation in number of points from year to year.
The geocoded tabular data of annual HIV/AIDS rates were then imported into Arc-GIS9.1 GIS software as mapped points (). We then created map layers for each year consisting of only the points that had data values for that year. Care was taken to ensure that points without data were not converted to spurious zero prevalence values, which happens when a dBase table is imported into ArcGIS as X, Y coordinates. Years that had less than forty continent-wide data points were removed. Hence, the final data set covered eighteen years of data (1986–2003) varying from 49 to 568 data points each year.
The Spatial Analyst module of ArcGIS was used to spatially interpolate the continent-wide HIV/AIDS infection data points for each of the eighteen years within the boundaries of the African continent using two spatial interpolation techniques: the inverse distance weighted (IDW) algorithm and the kriging function. Spatial interpolation belongs to a family of spatial statistical procedures whose main function is to predict the spatial occurrence of data values in places that have no data from locations that have data values (see, for example, CitationHaining, Griffith, and Bennett 1983; CitationGetis 1991; CitationGetis and Ord 1992; CitationMitasova and Mitas 1993; CitationMitasova et al. 1995).
Interpolation methods are based on Waldo Tobler's first law of geography: “everything is related to everything else, but near things are more related than distant things” (CitationTobler 1970, 236). Objects that are close to one another are more related than those that are farther apart. The methods fall into two groups: deterministic and geostatistical. Deterministic techniques use the degree of similarity (e.g., IDW) or the level of smoothing (e.g., radial basis functions) to create a predicted surface from known measured point locations. Geostatistical methods (e.g., kriging) use probabilistic statistical models of the measured points. Deterministic interpolation techniques are called global if they compute predictions using the entire data set or local when they use measured points within specific neighborhoods to capture assumed local variation. They are considered exact if the resulting surface passes through the data value (i.e., minimum and maximum values of the generated surface occur only at sample points) and inexact if they do not pass through measured data values.
Inverse Distance Weighted Algorithm
IDW is an exact interpolator that assumes that each measured point in a data set has local influence on unmeasured locations, and the influence diminishes with distance. In predicting a value for an unmeasured location, IDW assigns more weight to measured values located closest to the prediction location than those farther away. IDW uses cross-validation to select an optimal power value (p) that gives the lowest root mean square prediction error (RMSPE) when the measured point is compared to the predicted value for that location. Each measured location is assigned a weight proportional to the inverse distance raised to the power value p. The power value, neighborhood selection strategy, and whether the neighborhood is local or not determine the surface calculated. After several iterations, we settled for a local neighborhood selected with a variable distance of twelve measured points around the prediction point, a p value of 2, and an output spatial resolution of 1 km for the IDW interpolation.
Kriging
Kriging is similar to IDW in that it also weights measured values using mathematical models based on distance decay to predict values at unmeasured locations. In addition, kriging uses the overall spatial configuration of the measured points. Kriging uses probabilistic statistical techniques and the concept of autocorrelation to quantify the spatial arrangement of the sample points around the prediction point based on a mathematical function called a semivariogram. Consequently, the weights used in kriging are more sophisticated than IDW weights. However, because of the probabilistic statistics used in predictions, kriging performs better for large samples. For small samples, and when locations of available measured values change from year to year as was the case in this study, interyear variability increases and the reliability of kriging is reduced. We used the standard ordinary kriging method, which assumes there is no trend in data values, the spherical semivariogram model, and a variable neighborhood of twelve measured data points nearest each prediction point. CitationLam (1983), CitationDavid (1977), and CitationIsaaks and Srivastava (1989) offer a detailed review and discussion of spatial interpolation methods.
Trend Surface Maps from IDW and Kriging Techniques
Any interpolation method comes with errors with situation-specific significance. Accordingly, the interpolation literature suggests that the relative value of IDW and kriging for any set of circumstances has to be empirically determined. Because the interpolated values were used as a basis for estimation and projections of national HIV/AIDS rates, we tested both IDW and kriging. The expected superiority of the kriging method over IDW was undermined by small sample sizes and the variability of number and location of measured points from year to year, which hiked interyear variability in prevalence. Although IDW surfaces can be sensitive to clustering and presence of outliers, IDW performed robustly under such conditions. Both methods also offer great visualization tools for the spatial and temporal trends of HIV/AIDS rates. As shown later, we also compared the outputs with simple averages of actual point data and projections by UNAIDS.
Using the IDW and kriging interpolation techniques as already described and a spatial mask to limit the interpolation within the African continent and Madagascar, we produced a continuous surface map for each year, with each 1-km pixel containing an estimate of the HIV rate. A sample of the resulting surface maps is shown in . shows maps of HIV prevalence rates for selected years using first the IDW method and then the kriging technique. The maps reveal spatial and temporal trends in HIV prevalence for sub-Saharan Africa over time.
Figure 2 Interpolated smooth surface maps of HIV/AIDS prevalence rates for 1986, 1990, 1994, 1999, and 2003 for IDW and kriging. IDW = inverse distance weighted.
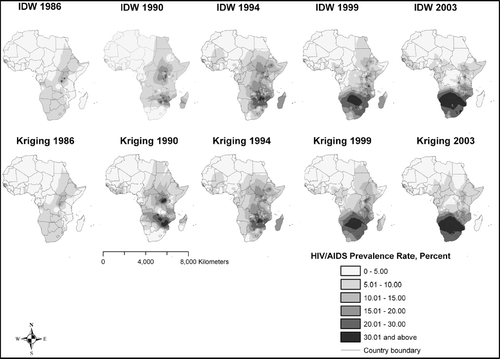
The next step involved spatially extracting the interpolated IDW and kriging HIV rates as a single mean HIV rate for each country in sub-Saharan Africa using a political boundary map of African countries and the zonal statistics function in ArcGIS Spatial Analyst. For this purpose, we assigned a unique numeric ID (one to fifty-six) to each African country, and converted the country map to raster format with 1 km spatial resolution. For each interpolation method, two versions of the zonal statistics procedure were run to generate (1) a continent-wide map showing extracted mean HIV/AIDS rates for each country (see ), and (2) a table in dBase format containing summary statistics for each country. The mean country rates for each of the eighteen years were extracted from the resulting tables and entered into a new table for all sub-Saharan African countries. However, because there were only few data points available for African countries north of the Sahara and island states (see ), only continental sub-Saharan countries and Madagascar were included in further analysis ().
Figure 3 HIV/AIDS prevalence rates (percent) by country for 1986, 1990, 1994, 1999, and 2003 for IDW and kriging. IDW = inverse distance weighted.
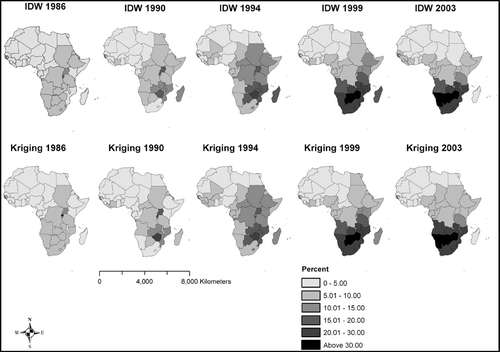
Table 1 Average HIV/AIDS prevalence rates derived from the IDW interpolation method and smoothed using EPP software, 1986–2010
Note that in we present the EPP smoothed rates extracted from the IDW method only. The reason for this is that after conducting correlation analysis between time (year) and HIV rates, the IDW technique consistently gave higher and more significant correlation coefficients for better predictive power. Although IDW had significant correlation coefficients for twenty-seven countries, assuming a linear relationship for purposes of comparison, only twenty-one countries turned with significant correlation coefficients on the kriging method. Equipped with the knowledge that the IDW technique was under these circumstances superior in its estimates, we decided to use the results of IDW in further analysis. We also conducted a paired correlation test for selected years between UNAIDS, kriging, IDW, and simple means. It is clear in that our results for the year 2003 are highly correlated to the figures provided by UNAIDS in 2003. It is also clear from that the results of IDW and kriging were more highly correlated than those between IDW and simple means or kriging. Estimates from kriging largely corroborated those from IDW, although simple averages from the original data set were less reliable because of missing data and small sample size.
Table 2 Comparison of UNAIDS figures with IDW, kriging, and simple means for selected years using Kolmogorov–Smirnov Z test
Although correlation analysis gave us an indication that the results of kriging and IDW were virtually identical and there was high correlation between the results of kriging and IDW versus the rates provided by UNAIDS and simple means, we were cognizant that correlation analysis might not be appropriate in our selection of IDW for further analysis, as all it does is compare how two variables track each other, without considering whether the values are correct or not. In other words, if one graphs rates provided by UNAIDS and one of the estimated rates from IDW or kriging, what we would like to see is a 1:1 line. There can be a high correlation but a 1:2 or 2:1 (or other ratio) line. We therefore used an additional test, the Kolmogorov–Smirnoff Z (K–S Z) test, which compares both how the variables track each other and whether or not the two estimates have similar values (see ). This test corroborated the results of correlation analysis. In other words, as seen from K–S Z results for the selected years, the differences in the estimates from UNAIDS versus kriging and IDW were statistically insignificant. Furthermore the K–S Z test for the IDW versus kriging indicated that the results of IDW and kriging were virtually identical, hence our selection to proceed with the results of IDW for further analysis.
provides a comparative analysis of the results of IDW and kriging versus those provided by UNAIDS for HIV prevalence rates for the year 2003, the latest year for which UNAIDS had estimates (see UNAIDS 2004). As illustrated in , the results of our analysis were comparable, if not superior, to those provided by UNAIDS. Given that the UNAIDS rates are considered to be the gold standard, we used the t test, a more robust and conservative method, to compare our results to UNAIDS as follows: IDW–UNAIDS rate, kriging–UNAIDS rate, and IDW–kriging rate. The t tests for equality of means between IDW–UNAIDS, kriging–UNAIDS, and IDW–kriging indicated that the means for these three pairs were not statistically different from each other in all three cases: IDW–UNAIDS (t = 0.501, p = 0.618), kriging–UNAIDS (t = 0.436, p = 0.664), and IDW–kriging (t = 0.063, p = 0.950). This gave us confidence to conclude that our estimates are robust to continue with further analysis. Furthermore, the rates estimated by UNAIDS are incomplete. Some countries had no estimates for 2003 because there were no reliable data for that year. In such cases IDW and kriging proved to be superior because they provided us HIV/AIDS prevalence estimates for each country, including countries that had data gaps, based on the concepts of spatial autocorrelation. Simple country-level averages from the original data were also unsatisfactory because of data gaps for nearly half of the countries (see for the year 2003 and see the column for N in ).
Table 3 Comparison of HIV/AIDS rates for 2003 from IDW, kriging, UNAIDS, and simple averages
HIV Surveillance Data Quality Issues
The method we used is an explicitly geographic approach to mine a rather insufficient point-based data set using the concept of spatial autocorrelation to generate HIV/AIDS estimates and compute forecasts. However, as is common in data collection and analysis, there are issues and challenges associated with the quality of available data, the best methods to process and analyze the data, and reliability of research findings. With the gravity of the HIV/AIDS pandemic, national and international agencies and policymakers are looking for actionable information that can provide answers to the who, how many, where, when, and how questions of the HIV/AIDS pandemic. A common problem is that analysts almost always operate with imperfect data, a situation exacerbated in the case of the HIV/AIDS pandemic in Africa by the relative novelty of the disease and lack of resources to screen for the disease and track its progress. As such, “there is no simple and reliable way to assess HIV incidence in Sub-Saharan Africa” (UNAIDS 2004, 21). Nevertheless, the sentinel data of HIV prevalence among pregnant clinic-going women used in this study represent the best proxy available at a continental level. According to UNAIDS, thirty-nine of the forty-three countries in sub-Saharan Africa and 118 countries worldwide implement HIV surveillance in antenatal clinics (WHO and UNAIDS 2003). Yet such a continent-wide data set undoubtedly has limitations. It is a composite of data collected at the national level, but without standard population sampling methods, standardized HIV testing procedures, and reporting requirements needed to produce comparable data.
First is the issue of representativeness of the sampled subpopulation: how well pregnant women who attend antenatal clinics represent the entire adult population. Several publications have looked at this limitation, and the need for additional data that are more representative of the entire population, such as demographic surveys (e.g., CitationFylkesness et al. 1998; CitationGlynn et al. 2001; CitationGregson et al. 2002; WHO and UNAIDS 2003). Among the major criticisms leveled against sentinel surveillance data obtained from pregnant women is the exclusion of men, nonpregnant women, and younger age groups from such a data set. As such, these data might not accurately represent the population, including inhabitants in rural areas where prenatal clinics are scarce and younger or older non-childbearing-age groups (CitationGarbus 2003).
This certainly points out the need for large and representative population samples in testing for HIV. Ironically, just such a representative sample survey, the Demographic and Health Surveys conducted in a substantial number of African countries during the 1990s and early 2000s, did not collect information on HIV prevalence or incidence in most of the countries surveyed. This demonstrates challenges on the sensitivity of sexual matters and HIV/AIDS. The surveys concentrated on collecting information on knowledge and attitudes concerning HIV, particularly the use of condoms. It was only in a handful of countries that information on HIV prevalence was collected, namely Burkina Faso for the year 2003, Cameroon for the year 2004, Ghana for the year 2003, Kenya for the year 2003, Mali for the year 2001, Tanzania for the year 2003–2004, and Zambia for the year 2001–2002 (see Orcmacro 2005). As such the data from these surveys, although useful, are incomplete to inform policy in many African countries. The data set that we used is probably the richest available.
As for the interpolated HIV prevalence rates, because the IDW algorithm uses both magnitude and geographic location of data points for interpolation, the varying yearly spatial distribution affects the extrapolated rates. However, the averaging process involved tended to dampen this effect, and the relative magnitudes and trends of averaged rates across countries tended to be maintained. In addition, the use of the UNAIDS EPP software to smooth the data gave an added advantage to the IDW and kriging estimates. Nevertheless, these projections need to be used with caution given the data limitations highlighted earlier, which are likely to influence the projection's reliability.
Furthermore, our findings suggest that advantages of using GSA to generate HIV rates for future predictions outweigh not only associated costs, but also the benefits from standard aspatial methods, such as simple averaging of small-N samples by country. First, GSA allows us to predict HIV rates in locations for which there were no data (see CitationAnselin 2000; CitationBennett, Haining, and Griffith 1984). For Africa the reliability of current HIV projections derived purely from aspatial methods, let alone the ability to generate national estimates at all, is limited by the small number, uneven spread, and erratic reporting from surveillance sentinel points. Gaps in the Simple Averages column in clearly demonstrate the inadequacy and unreliability of sentinel-based HIV data in Africa and the subsequent difficulty in drawing statistical conclusions from such (raw) data. Second, the HIV rates and projections obtained from GSA were robust. We compared national averages derived from IDW and kriging with those from simple averages of original point data and UNAIDS rates for the year 2003 (see ). One-way analysis of variance (ANOVA) for IDW, kriging, and UNAIDS rates showed that there were no significant differences among them at the 0.05 level (p = 0.6). When applied to the EPP software to generate short-term future projections of the number of people living with HIV (), our GSA-based projections were, with explainable exceptions (e.g., exceptions highlighted in ), comparable to the best available estimates provided by UNAIDS using more sophisticated models. Third, in addition to providing an alternative spatially explicit method that generates robust, first-order projections of numbers of people infected with HIV/AIDS under conditions of chronic poor quality data, GSA provided powerful spatial “views” of the progression of the pandemic over space and time on a continental scale.
Table 4 Projected population living with HIV using the EPP software package, 2004–2010
Finally, spatial interpolation (IDW and kriging) has the added potential advantage of generating HIV rates and projection summaries at scales different from those at which the data were collected. This opens up opportunities for refining the first-order projections obtained in this study or for conducting additional analyses at such other scales as the country, region, or district. Such refinement is made possible by integrating additional data variables (and new analytical methods such as spatial statistical regression modeling) that are more readily available at such finer scales, including migration patterns, transportation networks, and economic data, and behavioral factors.
Spatial-Temporal Trends in HIV Prevalence for the Past Two Decades
One major contribution of GIS and GSA to HIV/AIDS surveillance is the addition of a spatial component and revelation of spatial trends over time in innovative ways that are visually powerful for both analysts and policymakers. The well-known saying “a picture is worth a thousand words” is also applicable to maps and images. GSA can reveal spatial and temporal trends masked by national statistics. and show interpolated HIV prevalence rates and the spatial and temporal progression of HIV/AIDS across sub-Saharan Africa for the years 1986, 1990, 1994, 1999, and 2003 for both IDW and kriging techniques. shows the trend surface maps and gives the average HIV/AIDS rates extracted at the national level from the trend surface maps. These maps show that southern Africa has consistently had HIV prevalence rates in excess of 5 percent since 1986, and parts of north and west Africa have generally had the lowest prevalence, below 5 percent. However, over time the epicenter of HIV/AIDS (10–20 percent prevalence) located in the Great Lakes region of East Africa (Burundi, Rwanda, Tanzania, and Uganda) and an isolated pocket in west Africa (Guinea-Bissau) in 1986, had by 1990 expanded in both prevalence level and spatial extent within the Great Lakes region and developed a second nucleus in the southern Africa region (Zimbabwe, Zambia, Mozambique, and Malawi). By 1994 a more intense (15 to over 30 percent) southern African epicenter had expanded to include Botswana and parts of South Africa, whereas the Great Lakes nucleus had broken down into isolated pockets and a new nucleus had developed over Ethiopia/Eritrea. The years 1999 and 2003 saw an even more intense southern African expansion to include Namibia, South Africa, Swaziland, and Lesotho, with isolated, less intense pockets in the east Africa region (Kenya, Uganda, Tanzania, and Ethiopia) and Cameroon and Côte d'Ivoire in western Africa. The spatial and temporal trends so vividly brought out by the continuous interpolated maps largely conform with UNAIDS estimates of an escalating epidemic in southern Africa, and with signs of stabilization or decline in the east African region. At present southern Africa remains the worst affected subregion in the world, with HIV prevalence rates in excess of 25 percent, a sharp rise from around 5 percent in 1986 (UNAIDS 2004).
Curve Fitting and Forecasting the HIV Positive Population from 2004 to 2010
As noted earlier, the preceding GSA is grounded in a long tradition of works by geographers in diffusion studies. Such work can be traced back to the empirical studies of CitationHägerstrand (1968) on how innovations diffuse in time and space. One important contribution in the Hägerstrand models of space–time diffusion was the realization that the resistance of a population to adopting an innovation or being infected with a disease usually follows an S-shaped curve (CitationHaggett 2001, 490). In the case of epidemics, they often follow an S-shaped curve or bell-shaped curve, with rates rising rapidly early in the epidemic and then slowing down, reaching a plateau, and even declining to a point where they are completely extinguished. The S-shaped curve can be approximated by a logistic distribution given by the following simple equation (see CitationHaggett 2001, 490):
As Haggett (2001) noted, the constant b has a critical effect on the form of the S-shaped curve. Low values describe smooth curves, whereas higher b values describe rates of infection that have a slow initial build-up, explode rapidly in a middle period, and enter a final period of slow or stable growth (see CitationGould 1969). Guided by such early work in diffusion modeling by geographers, particularly the S-shaped logistic curves, scientists are now building mathematically sophisticated dynamic models to back-calculate and forecast into the near future the trajectories of various diseases including HIV/AIDS. However, the basis of these models remains the parameters highlighted in the more widely applicable logistic distribution presented earlier.
One such dynamic but simple-to-use model is the UNAIDS EPP prepared by the UNAIDS Reference Group on Estimates, Models and Projections. The development of this projection package is a direct response to uncertainties in forecasting future trends of the AIDS pandemic. The EPP was developed to assist in the estimation and projection of prevalence rates, incidence rates, and population size living with HIV in countries where HIV is firmly established in the general population such as in sub-Saharan Africa (see CitationGhys et al. 2004). The EPP is a simple epidemiological model that produces the basic S-shaped or bell-shaped curves found in most epidemics. In this package, the user fits a model (S-or bell-shaped) to the available HIV prevalence data over time. The EPP software automatically finds a mathematically reasonable fit to the data. Once a user enters data into the program's spreadsheet, EPP searches for the best values for four key parameters (described later) through an iteration process that starts with arbitrary estimates for the four parameters and returns an HIV prevalence curve that best fits the surveillance data. The best fit is determined by minimizing the sum of the squared errors (the differences between the model curve and the surveillance estimates in each year). In most cases it is a very close fit, requiring no adjustment. A user can choose the year to project to.
Occasionally, the fit is not so close to the observed data. In such cases, the EPP user can adjust the fit of the data (i.e., the exact shape of the S-or bell-shaped curve) if the automatic fit is not acceptable. An interactive process allows the user to change the values of four model parameters similar to those of the logistic distribution described earlier. The four parameters are the start year of the epidemic, growth rate, prevalence peak of the epidemic, and the shape of the epidemic after the peak. The actual model parameters are t o , the initial year of the epidemic; f o , the initial proportion of the population that is in the at-risk category; r, the force of infection that governs the rate at which people in the susceptible population become infected; and f or phi, a parameter that affects the distribution of new entrants to the not-at-risk and at-risk categories (see CitationGhys et al. 2004). The EPP is based on a set of clearly thought out assumptions such as survival to age fifteen, the prevailing birth rate, fertility reduction caused by HIV, perinatal transmission rate, and the distribution or progression from infection to AIDS death. These values are pre-fixed for each specific country and are derived directly by the program from country-level population estimates and projections from the United Nations Population Division that come with the program. The EPP software and user manuals are available for free download at http://www.unaids.org/en/HIV_data/Epidemiology/episoftware.asp. Details on exact equations, assumptions, and explanations of the EPP software are available at the same Web site (UNAIDS Reference Group on Estimates, Modelling and Projections 2002; CitationGhys et al. 2004).
We used this software package to fit epidemic curves to the HIV prevalence rates generated from IDW and kriging analysis and make predictions for the years 2004 to 2010. In short, the fitted and projected curves produced by this simple-to-use software package provided a picture of virtually the entire course of the epidemic from the start in 1980 to 2010. EPP was used not only to forecast but also to smooth the annual fluctuations inherent in GIS-generated HIV/AIDS rates. The results of curve fitting using EPP are shown in , , and . The EPP-smoothed HIV/AIDS rates derived from GIS analysis are provided in .
Figure 4 Curve fitting for HIV/AIDS prevalence rates for selected countries in east and central Africa.
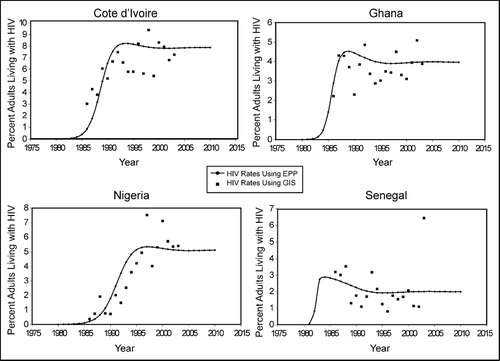
The curves in , , and clearly illustrate that the various regions in Africa have experienced HIV/AIDS epidemics that are quite different in character and are specific to each region. clearly shows that countries in the central and eastern African regions were indeed the first to feel the brunt of this epidemic. The epidemic peaked in Kenya and Central African Republic between 1985 and 1990 and in Tanzania and Cameroon between 1990 and 1995. Furthermore, the rates at which countries in the two regions peaked varied between 12 and 16 percent in Tanzania and Kenya in east Africa to between 8 and 10 percent in Cameroon and Central African Republic in central Africa. The data in these figures confirm the increasingly accepted observation that there are many different HIV/AIDS epidemics, not just one. The curves also show projections of the HIV/AIDS rates to 2010 to indicate the future situation, assuming past trends continue.
shows sample epidemic curves for four countries in western Africa, namely Côte d'Ivoire, Ghana, Nigeria, and Senegal. The curves clearly show that the rates in western Africa have remained at low levels with the exception of Côte d'Ivoire, which peaked in 1990 at about 8 percent prevalence. For other countries such as Ghana, Nigeria, and Senegal, the rates peaked in the early 1990s at 2 percent for Senegal, 4 percent for Ghana, and 5 percent for Nigeria. HIV prevalence has remained stable, or in the case of Senegal declined slightly, to between 1 and 2 percent since the early 1990s. Even Côte d'Ivoire has stabilized at between 7 and 8 percent prevalence.
gives a sample of curves from southern Africa for Botswana, Namibia, Swaziland, and South Africa. The curves indicate that the disease essentially reached this region in the early 1990s, rising rapidly to reach levels of over 20 percent for many countries in this region, including the four shown here. However, in the early 2000s the disease began to peak and level off and projections into the near future indicate the continuation of this leveling and, in some cases (e.g., Zimbabwe), significant declines have recently started. This is good news for southern African countries, but the bad news is that the leveling off is taking place at high prevalence rates ranging from 20 to 30 percent.
The projected population living with HIV for sub-Saharan African countries generated from logistic forecast models is given in . The last column in gives the value of the log likelihood (LL), a measure of fit for the model for each country. Ordinarily, the appropriate measure for this type of model is the chi-square goodness-of-fit test, which is computed by multiplying –2 times the likelihood ratio (see CitationBrown et al. 2006). However, instead of giving the likelihood ratio, the EPP software we used only provided the LL as the best measure of how well the curve fits the data.
EPP2005 uses a maximum likelihood approach to fit a smooth curve to the data (see UNAIDS 2007). EPP 2005 fits the data by iteratively changing the parameters and calculating the LL, readjusting the parameters and recalculating the LL, and continuing with this process until it finds the maximum value of the likelihood. This corresponds to the smallest possible value of LL. Thus, the LL is a measure of how good the fit is and the objective is to minimize it, or make it as small as possible. EPP automatically fits and finds what it believes to be the set of parameters making LL as small as possible. It is this LL that we report in the last column of . The LL gives us an idea of the fit of the models as they vary in time and space.
, , and show that the fit appears to be much better for the epidemics in the southern African nations than east or west African nations in terms of the logistic curve. However, a closer examination of the LL values in indicates otherwise. The LL values are smallest in west Africa, followed by east Africa and then much larger than expected in southern Africa. In other words the models in west Africa fit the data quite well, whereas in southern Africa there are more fluctuations in the data in spite of the illusion in the graphs that southern Africa had a much better fit. This has to do with the nature of the epidemic in the various regions. In western Africa the epidemic has remained at low levels resulting in small fluctuations in the data. In southern Africa, the disease spread rapidly and increased within a short period from 0 to rates of well over 30 percent, resulting in larger fluctuations in the data from year to year, hence much higher LL values. Nevertheless, the LL values are bunched within a reasonable range from 47.20 for Ghana to a high of 99.19 for Madagascar. These values indicate that the generated models fit the data reasonably well. CitationIliffe (2006) offers a detailed discussion of the reasons behind the differing nature and trajectories of the epidemic as experienced in western Africa, central Africa, eastern Africa, and southern Africa. According to Iliffe the reasons for the different spatial-temporal trajectories include the political, cultural, social, and economic contexts, particularly the colonial economy based on labor migration, which allowed the disease to spread rapidly with such an explosive force in southern Africa in comparison to the other regions of the continent.
The projected numbers of people living with HIV given in were obtained directly from the EPP using the GIS-generated HIV/AIDS rates. A comparison of the projected populations in with the UNAIDS total figure for sub-Saharan Africa for the year 2005 yields a very close result. Our projected total population living with HIV in the year 2005 is 27.7 million people. Although this total is slightly above the upper bound of the UNAIDS estimate, it is nonetheless quite close. The UNAIDS range for 2005 is given as 21.6 million to 27.4 million people (UNAIDS 2005). This is also true for the year 2004. UNAIDS reports that there were about 25.4 million people in sub-Saharan Africa living with HIV/AIDS in 2004, and gives a range of 23.4 million to 28.4 million. The projections in this study based on GIS-generated prevalence rates yield an estimate of 27.2 million adults for that year, which falls squarely within the UNAIDS range. The comparable results between our GIS-based projections and those of UNAIDS add confidence to the forecasts in . The forecasts suggest that by 2010 the number of people living with HIV will increase to 30.2 million people for sub-Saharan Africa if the trends of the last two decades continue. Some countries will be affected more than others. However, the HIV/AIDS pandemic is dynamic. For instance, effects of preventive and treatment measures, even regress in response measures in some countries, and other unknown factors might not be adequately captured by the EPP model. For this reason we do not feel confident enough to make projections beyond the immediate future (i.e., up to the year 2010).
Discussion of Results, Policy, and Research Implications
The use of GIS to spatially extrapolate and fill gaps created by insufficient data has allowed otherwise complex spatial-temporal trends in HIV/AIDS prevalence and projections to be made with relative ease. GIS spatial interpolation revealed spatial patterns of HIV prevalence in sub-Saharan Africa and how the disease (and its epicenter) has progressed over the past two decades ( and ). GIS analysis of sentinel surveillance data from antenatal clinics allowed for the generation of HIV/AIDS rates that were then entered into a more sophisticated forecasting software package (EPP) to model and forecast the progression of the disease for sub-Saharan African countries (, , and and ). With these models, major subregional trends have been detected, largely confirming reports by UNAIDS (e.g., UNAIDS 2004, 2005), but adding a visual and regional perspective. GIS revealed spatial patterns including HIV hot spots or epicenters and temporal trends at a regional level that are usually masked by aspatial statistics of HIV prevalence at national or other scales. The models permitted short-term projections of HIV prevalence rates into the near future assuming that current trends will continue, and first-order estimates of the number of people that will be living with HIV up to the year 2010 ().
Epidemiological studies suggest that disease prevalence is subject to autocorrelation as shown by a tendency for clustering (e.g., CitationGolub, Gorr, and Gould 1993; CitationRuiz et al. 2004; CitationKrieger 2003; CitationSabel and Löytönen 2004; CitationTedesco 2004). This suggests that spatial interpolation, which assumes autocorrelation, is a suitable approach for understanding the spatial nature of the HIV/AIDS pandemic in Africa. For the majority of cases, spatial interpolation is a robust technique with the primary values of filling spatial and temporal (e.g., years when sentinel data are unavailable for one reason or another) gaps in data and vividly bringing out spatial-temporal trends in HIV prevalence. Admittedly, spatial interpolation has a smoothing effect that tends to underestimate islands of high values in regions of generally lower values (e.g., Cameroon and Côte d'Ivoire) and to overestimate islands of low values in regions of higher rates (e.g., Angola). This is particularly evident, as island biogeography teaches us, in cases where major physical barriers undermine the utility of spatial autocorrelation and interpolation. HIV estimates for small island nations are likely to be overdetermined by mainland rates, thus the exclusion of such states in this analysis. However, even for large island states such as Madagascar (included in this study), the utility of analyses based on autocorrelation seems to break down across the physical barrier of oceans. The scantiness of data, for instance the fact that in 1997 and 2003 Madagascar reported HIV rates from only two testing centers, compounds effects of physical barriers. HIV rates for Madagascar during this time averaged around 1.2 percent. However, despite masking out oceans in map outputs, interpolation with IDW generated higher average rates of around 10 percent because of the influence of points from more infected continental southeastern Africa. In addition interpolation still needs a reasonable number of points that are evenly distributed spatially to provide reliable data. However, because of data problems that afflict Africa as discussed earlier, GSA using interpolation offers a unique opportunity to provide HIV data much needed for policymaking, advocacy work, program planning, and evaluation, from surveillance data. To a certain extent, spatial autocorrelation allows the filling in of such spatial and temporal data gaps, and provides information that is reasonable, and certainly better than no information.
HIV levels, trends, and projections made in this study can help to inform and galvanize international and national policies to combat the deadly disease. Persistently high HIV prevalence rates in excess of 30 percent recorded in countries like Botswana, Lesotho, Namibia, and Swaziland underscore the gravity of the problem. Yet the projected stabilization in some southern African countries such as Botswana, Namibia, South Africa, Malawi, Zambia, Zimbabwe, and others in recent years, and a decline in HIV prevalence in the Great Lakes countries of Uganda and Burundi offer hope that this disease can be defeated. GSA is likely to play a more important role as various economic, social, and demographic variables are incorporated into HIV surveillance and projections to further refine HIV estimates and provide more reliable information to policymakers on various scales.
Conclusion
As the AIDS epidemic in Africa continues to rage, there is a need to develop sound techniques to project the trajectory of the disease into the near future to inform policy. This study is testament to the usefulness of GIS techniques and spatial analysis in forecasting the HIV/AIDS epidemic in both space and time. The article offers an alternative grounded in geographic analysis for yielding estimates of HIV/AIDS rates in Africa. Using incomplete sets of data covering the period between 1986 and 2003, mean HIV/AIDS rates are generated spatially, and temporal trends estimated using the UNAIDS EPP. Keeping in mind that the prevalence rates were largely estimated from pregnant women, the EPP is then used to generate short-term forecasts of the total number of adults (age fifteen years and older) living with HIV for each country from 2004 to 2010. Interested parties, including national authorities, can scale such analyses as this study down to a finer scale, and refine them with additional data, including demographics, transportation networks, and behavioral factors that influence the spread and transmission of HIV/AIDS in space and time at national and subnational scales.
In conclusion, the results of the analysis in this article and their broader implications offer great hope for the continent. Although we graphically illustrate the complex nature of this disease and the manner in which it rapidly spread throughout the continent, the growth curves and forecasts indicate that HIV prevalence rates have now peaked in almost all African countries, some at very low rates and others at very high rates. The sophisticated GIS and EPP analyses provide ample evidence that in some countries the epidemic has leveled, in others it is actually on the decline (e.g., Uganda), and in still others where government commitment was strong immediately after the epidemic was recognized the prevalence rates have been kept at very low levels (e.g., Senegal). Thus, in spite of the tragic consequences for the peoples of Africa of this insidious epidemic, it is gratifying to note that local, national, and international efforts to combat this epidemic are finally yielding the intended results as exemplified by the peaking and leveling off of prevalence rates. We therefore wish to end this article on a positive note by highlighting the growing international pressures to provide life-saving antiretroviral medications, the ongoing research on vaccines by international pharmaceutical corporations, the continuing grassroots political activism, and the emergence of compassionate organizations such as The AIDS Support Organization (TASO) in Uganda and Treatment AIDS Campaign (TAC) in South Africa, all of which have contributed significantly to the peaking and leveling off of the disease. The broader implication of these encouraging results is that policymakers and program implementers at the local, national, and international levels should continue their vigorous and aggressive efforts at combating and eradicating this scourge; there is no room for complacency.
EZEKIEL KALIPENI is an Associate Professor in the Department of Geography at the University of Illinois at Urbana-Champaign, 607 South Mathews Avenue, Urbana, IL 61801. E-mail: [email protected]. His research interests focus on the geographies of health, population, and the environment with specific reference to Africa.
LEO ZULU is an Assistant Professor in the Department of Geography at Michigan State University, 103 Geography Building, East Lansing, MI 48824. E-mail: [email protected]. His research interests include political ecology, GIS, remote sensing, and geospatial analytical techniques in general.
Notes
aMeans kriging and IDW values are out of UNAIDS range.
1The data were provided in Excel spreadsheet format by Centra Technology Offices, 4121 Wilson Blvd., Suite 800, Ballston, Arlington, VA.
Literature Cited
- Anselin , L. 2000 . The alchemy of statistics, or creating data where no data exist . Annals of the Association of American Geographers , 90 ( 3 ) : 587 – 92 .
- Bennett , R. J. , Haining , R. P. and Griffith , D. A. 1984 . The problem of missing data on spatial surface . Annals of the Association of American Geographers , 74 ( 1 ) : 138 – 56 .
- Brown , T. , Grassly , N. C. , Garnett , G. and Stanecki , K. 2006 . Improving projections at the country level: The UNAIDS Estimation and Projection Package 2005 . Sexually Transmitted Infections , 82 : iii34 – iii40 . (Supplement III)
- Brunn , S. D. 1992 . Are we missing our “forests” and our “trees”? It's time for a census . Annals of the Association of American Geographers , 82 ( 1 ) : 1 – 2 .
- Cochrane , M. 2000 . The politics of AIDS surveillance . The Professional Geographer , 52 ( 2 ) : 205 – 18 .
- Cromley , E. and McLafferty , S. 2002 . GIS and public health , New York : Guilford .
- David , M. 1977 . Geostatistical ore reserve estimation , Amsterdam : Elsevier .
- ESRI . 2005 . Arc GIS: What is ArcGIS? , Redlands, CA : Environmental Systems Research Institute .
- Fylkesness , K. , Ndhlovu , Z. , Kasumba , K. , Mubanga , M. R. and Sichone , M. 1998 . Studying dynamics of the HIV epidemic: Population-based data compared with sentinel surveillance in Zambia . AIDS , 12 : 1227 – 34 .
- Garbus , L. 2003 . HIV/AIDS in Malawi: Country AIDS policy analysis project , San Francisco : AIDS Policy Research Center, University of California . http://hivinsite.ucsf.edu/InSite?page=cr-ari(last accessed 22 January 2007)
- Gatrell , A. C. and Löytönen , M. 1998 . GIS and health , London : Taylor & Francis .
- Getis , A. 1991 . Spatial interaction and spatial autocorrelation: A cross-product approach . Environment and Planning A , 23 : 1269 – 77 .
- Getis , A. and Ord , J. K. 1992 . The analysis of spatial autocorrelation by use of distance statistics . Geographical Analysis , 24 : 189 – 206 .
- Ghys , P. D. , Brown , T. , Grassly , N. C. , Garnett , G. , Tanecki , S. K. A. , Stover , J. and Walker , N. 2004 . The UNAIDS estimation and projection package: A software package to estimate and project national HIV epidemics . Sexually Transmitted Infections , 80 ( 1 ) : i5 – i9 .
- Glynn , J. R. , Buve , A. , Carael , M. , Musonda , R. M. , Kahindo , M. , Macauley , I. , Tembo , F. and Zekeng , L. 2001 . Factors influencing the difference in HIV prevalence between antenatal clinic and general population in sub-Saharan Africa . AIDS , 15 ( 13 ) : 1717 – 25 .
- Golub , A. , Gorr , W. L. and Gould , P. R. 1993 . Spatial diffusion of the HIV/AIDS epidemic: Modeling implications and case study of AIDS incidence in Ohio . Geographical Analysis , 25 ( 2 ) : 85 – 100 .
- Gould , P. 1969 . Spatial diffusion , Washington, DC : Commission on College Geography, Association of American Geographers .
- Gould , P. 1989 . Geographic dimensions of the AIDS epidemic . The Professional Geographer , 41 ( 1 ) : 71 – 78 .
- Gould , P. 1993 . The slow plague: A geography of the AIDS pandemic , Cambridge, MA : Blackwell .
- Gould , P. and Wallace , R. 1994 . Spatial structures and scientific paradoxes in the AIDS pandemic . Geografiska Annaler , 76B ( 2 ) : 105 – 16 .
- Gregson , S. , Terceira , N. , Kakowa , M. , Mason , P. R. , Anderson , R. A. , Chandiwana , S. K. and Carael , M. 2002 . Study of bias in antenatal clinic HIV-1 surveillance data in a high contraceptive prevalence population in sub-Saharan Africa . AIDS , 16 ( 4 ) : 643 – 52 .
- Hägerstrand , T. 1968 . Innovation diffusion as a spatial process , Chicago : University of Chicago Press .
- Haggett , P. 2001 . Geography: A global synthesis , Harlow, U.K. : Pearson Education .
- Haining , R. , Griffith , D. and Bennett , R. 1983 . Simulating two-dimensional autocorrelated surfaces . Geographical Analysis , 15 : 245 – 55 .
- Iliffe , J. 2006 . The African AIDS epidemic: A history , Athens : Ohio University Press .
- Isaaks , E. H. and Srivastava , R. M. 1989 . An introduction to applied geostatistics , New York : Oxford University Press .
- Kalipeni , E. , Craddock , S. , Oppong , J. R. and Ghosh , J. , eds. 2004 . HIV/AIDS in Africa: Beyond epidemiology , Oxford, U.K. : Blackwell .
- Krieger , N. 2003 . Place, space, and health: GIS and epidemiology . Epidemiology , 14 : 384 – 85 .
- Lam , N. S. 1983 . Spatial interpolation methods: A review . American Cartographer , 10 ( 2 ) : 129 – 49 .
- Lam , N. S. and Liu , K. 1996 . Use of space-filling curves in generating a national rural sampling frame for HIV/AIDS research . The Professional Geographer , 48 ( 3 ) : 321 – 32 .
- Liverman , D. , Moran , E. F. , Rindfuss , R. R. and Stern , P. C. 1998 . People and pixels: Linking remote sensing and social science , Washington, DC : National Academy Press .
- Löytönen , M. 1991 . The spatial diffusion of human immunodeficiency virus type 1 in Finland . Annals of the Association of American Geographers , 81 ( 1 ) : 127 – 51 .
- Löytönen , M. 1994 . Growth models and the HIV epidemic in Finland . Social Science and Medicine , 38 ( 1 ) : 179 – 85 .
- Löytönen , M. and Arbona , S. I. 1996 . Forecasting the AIDS epidemic in Puerto Rico . Social Science and Medicine , 42 ( 7 ) : 997 – 1011 .
- Löytönen , M. and Maasilta , P. 1997 . Forecasting the HIV epidemic in Finland by using functional small area units . GeoJournal , 41 ( 3 ) : 215 – 23 .
- Mitasova , H. and Mitas , L. 1993 . Interpolation by regularized spline with tension: I. Theory and implementation . Mathematical Geology , 25 : 641 – 55 .
- Mitasova , H. , Mitas , L. , Brown , W. M. , Gerdes , D. P. , Kosinovsky , I. and Baker , T. 1995 . Modelling spatially and temporally distributed phenomena: New methods and tools for GRASS GIS . International Journal of Geographical Information Systems , 9 ( 4 ) : 433 – 46 .
- Newman , J. L. 1990 . On the transmission of AIDS in Africa . Annals of the Association of American Geographers , 80 ( 2 ) : 300 – 301 .
- Oppong , J. R. 1998 . A vulnerability interpretation of the geography of HIV/AIDS in Ghana, 1986–1995 . The Professional Geographer , 50 ( 4 ) : 437 – 48 .
- Orcmacro . 2005 . Data tables: HIV/AIDS survey indicators database http://www.measuredhs.com/hivdata/data/(last accessed 27 January 2006)
- Ruiz , M. O. , Tedesco , C. , McTighe1 , J. T. , Austin , C. and Kitron , U. 2004 . Environmental and social determinants of human risk during a West Nile virus outbreak in the greater Chicago area, 2002 . International Journal of Health Geographics , 3 ( 8 ) http://www.ij-healthgeographics.com/content/pdf/1476-072X-3-8.pdf(last accessed 16 June 2005)
- Sabel , C. E. and Löytönen , M. 2004 . “ Clustering of disease ” . In GIS in public health practice , Edited by: Maheswaran , R. and Craglia , M. 51 – 70 . Boca Raton, FL : CRC Press .
- Shannon , G. W. and Pyle , G. F. 1989 . The origin and diffusion of AIDS: A view from medical geography . Annals of the Association of American Geographers , 79 ( 1 ) : 1 – 24 .
- Shannon , G. W. , Pyle , G. F. and Bashshur , R. 1991 . The geography of AIDS: Origins and course of an epidemic , New York : Guilford .
- Smallman-Raynor , M. , Cliff , A. and Haggett , P. 1992 . International atlas of AIDS , London : Blackwell .
- Tedesco , C. E. 2004 . Understanding an outbreak: A multidimensional spatial analysis of the Chicago 2002 West Nile virus outbreak , MA thesis Department of Geography, University of Illinois at Urbana-Champaign .
- Tobler , W. 1970 . A computer movie simulating urban growth in the Detroit area . Economic Geography , 46 ( 2 ) : 234 – 40 .
- UNAIDS . 2004 . Report on the global HIV/AIDS epidemic, July 2004 , Geneva, , Switzerland : UNAIDS . http://www.unaids.org/bangkok2004/report.html(last accessed 13 December 2005)
- UNAIDS . 2005 . AIDS epidemic update: December 2005 , Geneva, , Switzerland : UNAIDS . http://www.unaids.org/epi/2005/doc/EPIupdate2005_pdf_en/epi-update2005_en.pdf(last accessed 2 February 2005)
- UNAIDS . 2007 . Estimating national adult prevalence of HIV-1 in generalized epidemics—Manual , Geneva, , Switzerland : UNAIDS and WHO . http://data.unaids.org/pub/Manual/2007/epp_genepi_2007_en.pdf(last accessed 18 June 2007)
- UNAIDS Reference Group on Estimates, Modelling and Projections . 2002 . Improved methods and assumptions for estimation of the HIV/AIDS epidemic and its impact: Recommendations of the UNAIDS Reference Group on Estimates, Modelling and Projections . AIDS , 16 : W1 – W14 .
- U.S. Census Bureau . 2003 . HIV surveillance database , Washington, DC : International Programs Center, Population Division, U.S. Census Bureau . http://www.census.gov/ipc/www/hivaidsd.html(last accessed 14 June 2005)
- Watts , S. J. , Okello , R. and Watts , S. 1990 . Medical geography and AIDS . Annals of the Association of American Geographers , 80 ( 2 ) : 301 – 4 .
- Wood , W. B. 1988 . AIDS north and south: Diffusion patterns of a global epidemic and a research agenda for geographers . The Professional Geographer , 40 ( 3 ) : 266 – 79 .
- World Health Organization . 2005 . WHO GIS and public health mapping http://www.who.int/csr/mapping/gisandphm/en/index.html(last accessed 13 June 2005)
- World Health Organization and UNAIDS . 2003 . Reconciling antenatal clinic-based surveillance and population-based survey estimates of HIV prevalence in sub-Saharan Africa http://www.unaids.org/(last accessed 3 July 2005)
- Young , L. and Ansell , N. 2003 . Fluid households, complex families: The impacts of children's migration as a response to HIV/AIDS in southern Africa . The Professional Geographer , 55 ( 4 ) : 464 – 76 .