Abstract
We propose a new method for collision-free planning using Conditional Generative Adversarial Networks (cGANs) to transform between the robot's joint space and a latent space that captures only collision-free areas of the joint space, conditioned by an obstacle map. Generating multiple plausible trajectories is convenient in applications such as the manipulation of a robot arm by enabling the selection of trajectories that avoids collision with the robot or surrounding environment. In the proposed method, various trajectories that avoid obstacles can be generated by connecting the start and goal state with arbitrary line segments in this generated latent space. Our method provides this collision-free latent space, after which any planner, using any optimization conditions, can be used to generate the most suitable paths on the fly. We successfully verified this method with a simulated and actual UR5e 6-DoF robotic arm. We confirmed that different trajectories could be generated depending on optimization conditions.
GRAPHICAL ABSTRACT
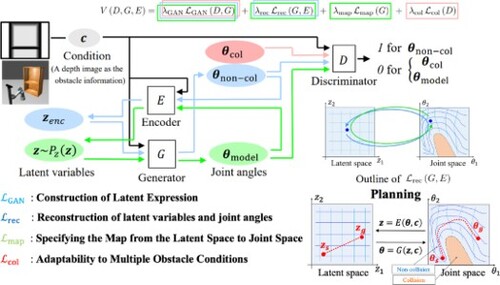
Acknowledgements
The authors would like to thank Avinash Ummadisingu for the proof check. H. Mori would like to thank all colleagues in the ETIS lab at the Cergy-Pontoise Univ., especially Prof. Mathias Quoy, Prof. Philippe Gaussier, and Assoc. Prof. Alexandre Pitti for discussion about a preliminary result of the basic idea of this article when he came up with the basic idea at the lab in 2016.
Disclosure statement
No potential conflict of interest was reported by the author(s).
Correction Statement
This article has been republished with minor changes. These changes do not impact the academic content of the article.
Notes
1 The link of the article for pre-experiment is the following: https://arxiv.org/abs/2202.07203.
Additional information
Notes on contributors
Tomoki Ando
Tomoki Ando received BS and MS degrees from Waseda University in 2020 and 2022, respectively. He was a technical staff in the National Institute of Advanced Industrial Science and Technology (AIST) from 2019–2022. He has been working for Sony Semiconductor Solutions Corporation since 2022. His research interest is deep learning for robotics.
Hiroto Iino
Hiroto Iino received a BS degree from Waseda University, Tokyo, Japan, in 2022. He is currently pursuing an MS degree at Waseda University. He is also a technical staff in the National Institute of Advanced Industrial Science and Technology (AIST). His current research interests include machine learning for robotics. He is a Student Member of The Japan Society of Mechanical Engineers.
Hiroki Mori
Hiroki Mori received BS and MS degrees from Toyohashi University of Technology and received a Ph.D. degree from the University of Tokyo in 2004, 2006, and 2011, respectively. He was a researcher of the JST ERATO Asada Synergistic Intelligence Project (2009–2011), an assistant professor at Osaka University (2011–2016), a researcher at the University of Cergy-Pontoise (2016–2017), a lecturer at Waseda University (2017), an associate professor in Waseda University (2017–2022). He is a visiting associate professor for Future Robotics Organization, Waseda University, and a visiting researcher of Advanced Industrial Science and Technology (AIST). He is also a co-founder/director of Tokuiten Inc. He received the Best paper award from SII2022 and the Best paper award in Cognitive Robotics from ICRA 2021. His interests are robotics, deep learning, neuroscience, emergence and development of artificial/natural intelligence.
Ryota Torishima
Ryota Torishima received BS and MS degrees from Waseda University in 2019 and 2021, respectively. He was a technical staff in the National Institute of Advanced Industrial Science and Technology (AIST) from 2018–2021. He has been working for SoftBank Corp. since 2021. His research interest is deep learning for robotics.
Kuniyuki Takahashi
Kuniyuki Takahashi received BS, MS, and Ph.D. degrees from Waseda University, in 2011, 2013, and 2017, respectively. He has been working for Preferred Networks, Inc. since 2017. His research interest is machine learning for robotics. He received FA Foundation Paper Award in 2019, Advanced Robotics Best Paper Award in 2019, the ICRA2019 Best Paper Award finalist, and the ICRA2018 Best Paper Award on HRI. He is a member of IEEE.
Shoichiro Yamaguchi
Shoichiro Yamaguchi received BS and MS degrees from Kyoto University, in 2016 and 2018, respectively. He has been working for Preferred Networks, Inc. since 2018. His research interest is machine learning.
Daisuke Okanohara
Daisuke Okanohara received BS, MS, and Ph.D. degrees from the University of Tokyo in 2001, 2007, and 2010, respectively. He is a Representative Director & Chief Executive Researcher of Preferred Networks, Inc. He is also the Chief Executive Officer of Preferred Computational Chemistry. His interests are deep learning for applications.
Tetsuya Ogata
Tetsuya Ogata received the B.S., M.S., and D.E. degrees in mechanical engineering from Waseda University, Tokyo, Japan, in 1993, 1995, and 2000, respectively. He was a Research Associate with Waseda University from 1999 to 2001. From 2001 to 2003, he was a Research Scientist with the RIKEN Brain Science Institute, Saitama, Japan. From 2003 to 2012, he was an Associate Professor at the Graduate School of Informatics, Kyoto University, Kyoto, Japan. Since 2012, he has been a Professor at the Faculty of Science and Engineering, at Waseda University. Since 2017, he is a Joint-appointed Fellow with the Artificial Intelligence Research Center, National Institute of Advanced Industrial Science and Technology, Tokyo. He is a director of the Institute of AI and Robotics, at Waseda University since 2020.