ABSTRACT
The inference of particle size distributions from differential mobility analyzer (DMA) data requires knowledge of the charge distribution on the particles being measured. The charge distribution produced by a bipolar aerosol charger depends on the properties of the ions produced in the charger, and on the kinetics of charge transfer from molecular ions or ion clusters to the particles. A single parameterization of a theoretically predicted charge distribution is employed in most DMA analyses regardless of the atmospheric conditions being probed. Deviations of the actual charge distribution from that assumed in the data analysis will bias the estimated particle size distribution. We examine these potential biases by modeling measurements and data inversion using charge distributions calculated for a range of atmospheric conditions. Moreover, simulations were performed using the ion-to-particle flux coefficients predicted for a range of properties of both the particles and ions. To probe the biases over the full range of particle sizes, the measurements were simulated through an atmospheric new particle formation event. The differences between the actual charge distribution and that according to the commonly used parametrization resulted in biases as large as a factor of 5 for nucleation-mode particles, and up to 80% for larger particles. Incorrect estimates of the relative permittivity of the particles or not accounting for the temperature and pressure effects for measurements at 10 km altitude produced biases in excess of 50%; three-fold biases result from erroneous estimates of the ion mobility distribution. We further report on the effects of the relative permittivity of the ions, the relative concentrations of negative and positive ions, and truncation of the number of charge states considered in the inversion.
Copyright © 2017 American Association for Aerosol Research
EDITOR:
1. Introduction
The differential mobility analyzer (DMA; Knutson and Whitby Citation1975) is the primary instrument used to measure size distributions of submicron aerosol particles. It separates particles from a small aerosol flow according to the velocities with which they migrate across a larger, clean, particle-free sheath flow under the influence of an electric field. The migration velocity of a particle depends on its electrical mobility; only those particles that carry electric charge are transmitted and counted. The probability that a particle carries one or more charges varies widely over the 1 nm to 1 µm size domain now covered by DMAs. That probability must be known to infer the particle size distribution from the numbers of charged particles that are transmitted through the mobility classifier. To ensure a stable and known charge distribution, most DMA measurements of particle size distributions are made using a so-called bipolar diffusion charger in which the aerosol is exposed to an ion cloud produced by radioactive decay, soft X-rays, or other methods. This device is also called an aerosol neutralizer because the mean charge state acquired is approximately neutral. The ions then undergo charge-transfer reactions with the aerosol particles, some of which increase the number of charges, while others neutralize previously attached charges. Ideally, a steady-state charge distribution is established, providing a well-defined charge state that can be used in concert with well-established models of the performance of the DMA (Stolzenburg Citation1988; Zhang and Flagan Citation1996; Flagan Citation1999; Hagwood et al. Citation1999) to infer the particle size distribution from counts of the small fraction of the particles that are charged.
Most studies of the charge distribution derive from the pioneering work of Fuchs (Citation1963) who developed a heuristic model to describe the flux of gas ions to the surfaces of neutral and charged particles ranging from ones small compared to the mean-free-path, λi, of the ions (free molecular regime) to ones much larger than λi (continuum regime). In this way, he derived attachment coefficients throughout the transition regime. Those attachment coefficients can be used to predict the charge distribution under different scenarios. The most common scenario explored is the steady-state bipolar charge distribution produced by exposure of the aerosol to an ion cloud generated by energetic particles from radioactive decay. This pioneering science laid the groundwork for the use of mobility-based methods for particle size distribution measurements. By bringing the aerosol particles to a known charge distribution, number concentrations obtained as a function of electrical mobility in DMA analysis can be inverted to deduce the particle size distribution. New sources of gas ions have been developed to replace the radioisotope sources of the traditional neutralizer, thereby reducing regulatory barriers to transporting instruments and using them in a number of environments. The alternative methods for ion production include soft X-ray sources (Shimada et al. Citation2002; Jiang et al. Citation2007; Yun et al. Citation2009), dual-corona discharge (Qi and Kulkarni Citation2013), surface-discharge microplasma (Kwon et al. Citation2006), and carbon fiber ionizer (Han et al. Citation2009). Shimada et al. (Citation2002) demonstrated attainment of a steady-state bipolar charge distribution by the combination of direct charging by soft X-rays and diffusion charging, establishing the viability of the soft X-ray source for aerosol neutralization.
Fuchs (Citation1963) used his attachment coefficients to derive the steady-state bipolar charge distribution that results when, as in the neutralizer, the aerosol is exposed to a large excess of gas ions. At small sizes, most particles in this steady-state distribution carry at most one elementary unit of charge, e, but the probability that a particle will carry multiple charges increases rapidly with increasing size above threshold of about 20 nm. Hoppel and Frick (Citation1986) corrected some subtle errors in the original model and undertook extensive computer simulations to predict the steady-state bipolar charge distribution under atmospheric conditions. Recognizing the need for an accessible model for use in inferring particle size distributions from DMA measurements, Wiedensohler (Citation1988) fitted the results of the Hoppel and Frick (HF) simulations to a readily evaluated empirical expression, establishing the bipolar charge distribution that has been used in the interpretation of particle size distributions in most DMA-based measurements made to date. The Fuchs/Hoppel-Frick/Wiedensohler (FHFW) charge distribution has thus played such a central role in enabling DMA measurements to provide a comprehensive view of the dynamics of small aerosol particles that it is incorporated into the ISO 15900 standard.
Though the theory of bipolar aerosol charging is well established and extensively used, questions about the accuracy of the predicted charge distribution remain. Soon after calculating the steady-state bipolar charge distribution, Hoppel and Frick (Citation1990) observed that small electric fields within, or downstream of, the aerosol neutralizer can dramatically bias the charge distribution. More recently, the ion-to-particle flux coefficients have received renewed attention with Ouyang et al. (Citation2012) probing the influence of attractive singular contact potentials on the collision process, which increases the relative velocity between an ion and an oppositely charged particle, and may lead to higher ion-to-particle flux coefficient in the transition regime, Gopalakrishnan et al. (Citation2013b) deriving equations to account for non-spherical geometries of the particles, and López-Yglesias and Flagan revising the FHFW model (Citation2013a). Further, a method to estimate the charge distribution without the need to calculate the flux coefficients was developed (Gopalakrishnan et al. Citation2013a) and found to be in good agreement with the FHFW model at room temperature and atmospheric pressure (Gopalakrishnan et al. Citation2015). Finally, recent studies suggest that achieving the steady-state bipolar charge distribution in the charger, which is a critical assumption in most mobility-based particle size distribution measurements, could depend on the concentrations of measured particles (de la Verpilliere et al. Citation2015; Tigges et al. Citation2015) and on the aerosol flowrate through the charger (He and Dhaniyala Citation2014), adding to the uncertainty.
The main limitation of the FHFW model is that the influence of the electrostatic potential on the thermal speeds of the ions is neglected (Filippov Citation1993; Gopalakrishnan and Hogan Citation2012; Ouyang et al. Citation2012). The resulting underestimation of the ion speeds under the attractive potential between an ion and an oppositely charged particle result in a corresponding overestimation of the flux coefficient in the transition regime (Gopalakrishnan et al. Citation2015). Even with this limitation, however, the bipolar charge distribution predictions of the FHFW model were in good agreement with measurements (Gopalakrishnan et al. Citation2015).
In a recent reexamination of the FHFW model, López-Yglesias and Flagan (Citation2013a,Citationb) identified some errors, as well as some numerical approximations that arose due to the computing resources available at the time of HF simulations. The effects were minor through the mid-range of sizes where most measurements have traditionally been made, but for particles in the low nanometer regime (<20 nm diameter), and for particles larger than a few hundred nanometers, the errors can become significant. That study refined the general Fuchs/HF model of the charging kinetics, and examined the effects of the refinements, albeit with some simplifying assumptions. Notably, the positive and negative gas ions were assumed to have the same mobilities as those used in the original HF simulations to facilitate direct comparison of the two versions of the model. Results from that study suggest that biases could result when the bipolar charge distribution predicted for sea level charging of conductive particles is applied to different kinds of aerosols, or to measurements at different altitudes. That study did not, however, provide sufficient calculations to draw any quantitative conclusions about the uncertainties in the particle size distribution that may result from the combined effects of imperfect knowledge of ion and particle properties, and model limitations.
The present study employs the refined FHFW model of aerosol particle charging to explore how ion and particle properties, and atmospheric conditions may influence the interpretation of DMA data, and to quantify potential biases in the measured particle size distribution caused by differences between the charge distribution acquired in the charger and that assumed for data analysis by inversion. More specifically, we will separately study parameters that define the steady-state bipolar charge distribution and assess the potential bias in the particle size distribution caused by each of these parameters. The probed parameters include relative permittivity of the particles, mobility distribution, mass and relative permittivity of the charger ions, and the relative concentrations of positive and negative ions. As measurements are conducted at a range of altitudes, including airborne measurements (Clarke et al. Citation1998; Mirme et al. Citation2010; Coggon et al. Citation2014) and measurements at high altitude field stations such as Jungfraujoch, Switzerland (3580 m asl; Weingartner et al. Citation1999), we also explore the bias that results from failing to account for the pressure and temperature variation with altitude. Finally, we will quantify the bias due to insufficient number of particle charge states considered in the inversion. While we discuss DMA-based particle size distribution measurements, the conclusions are not specific to the DMA, and apply to measurements made with other mobility classifiers.
2. Methods
Unless otherwise noted, we use the following notation throughout this article: diameter refers to mobility equivalent diameter, flux coefficient refers to ion-to-particle flux coefficient, charge distribution refers to steady-state bipolar charge distribution, concentration refers to number concentration, and particle size distribution refers to distribution of particle number concentration with respect to particle size.
The following procedure was used to quantify the magnitude of bias in the measured particle size distribution due to differences between the charge distribution acquired in the charger, fcha, and that assumed for data analysis by inversion, finv: (Equation1[1] ) We used known particle size distributions from aerosol dynamics simulations as a synthetic sample aerosol. These particle size distributions will be referred to as simulated distributions. (2) The simulated distributions were converted into “signals” emulating the working principle of a DMA, including an assumption on fcha. This pseudo-instrument approach acknowledges that high-resolution DMA measurements are now possible over the entire size range, albeit not with a single instrument. (Equation3
[3] ) Particle size distributions were inferred from the synthetic signals using the same inversion algorithm that has been applied to the raw signals from an actual instrument. The inversion algorithm included an assumption on finv, and these particle size distributions are referred to as inverted distributions. (Equation4
[4] ) The simulated and inverted distributions were compared to each other, with any differences between the two distributions originating from the difference between fcha and finv, provided that the inversion characterizes other features of the pseudo-instrument correctly. This procedure represents measurement conditions in which the instrument is characterized perfectly, except for the charge distribution acquired in the charger, which allowed us to quantify the bias in the particle size distribution caused by fcha ≠ finv. Some features of the pseudo-instrument were simpler than corresponding features of an actual instrument, which does not affect the conclusions of this study as long as the inversion algorithm characterizes those features correctly. A similar procedure could be used to study other features of the inversion algorithm, but the focus of this study is on the charge distribution acquired in the aerosol charger.
In the remainder of this section, we will present the steps of the analysis in more detail. We will also present the assumptions made when calculating the ion-to-particle flux coefficients, which were needed for calculating the charge distributions.
2.1. Calculation of the charge distributions
The charge distribution, f, of an aerosol is defined as the collection of fractional populations, fk, of all charge states considered, i.e., f = {f-y, f-y+1,…, f-2, f-1, f0, f1, f2,…, fy-1, fy}, where y = kmax, which is the maximum number of charges a particle is assumed to acquire. fk(dp), denotes the fraction of particles with a diameter, dp, that have the charge state k, i.e., fk(dp) = nk(dp)/nT(dp), where nk and nT are the concentration of particles having charge state k and the total particle concentration, respectively.
The charge distribution in bipolar steady-state can be estimated using a Brownian dynamics method (Gopalakrishnan et al. Citation2013a) or deduced from the ion-to-particle flux coefficients by solving a system of population balance equations (Hussin et al. Citation1983; Hoppel and Frick Citation1986), with the latter method used in this study. To that end, we calculated the flux coefficients using the refined FHFW model as described by López-Yglesias and Flagan (Citation2013a) with two notable differences: First, while the initial work with this model assumed that all ions of one polarity have the same mobility, Zi, we follow the approach of Lee et al. (Citation2005) to describe the ions using a discrete mobility distribution, ni±(Zi); both ambient (Ehn et al. Citation2010) and laboratory (Steiner et al. Citation2014; Maißer et al. Citation2015) measurements have revealed that a range of ions are generally present, as has previously been assumed in the Brownian dynamics studies of the charge distribution (Gopalakrishnan et al. Citation2013a, Citation2015; Maißer et al. Citation2015). Second, we account for the polarization of air molecules in the relation between ion mass and mobility.
To describe the charger ions, we measured the ion mobility distribution of the ions produced from HEPA-filtered laboratory air passing through a TSI 3077 bipolar 85Kr aerosol charger using a Radial Opposed Migration Ion and Aerosol Classifier (ROMIAC; Mui et al. Citation2013, Citation2017), and a TSI 3068 electrometer. For comparison, we also used an ion mobility distribution based on that measured by Steiner and Reischl (Citation2012). These distributions will be described as the measured and the SR distributions, respectively; a more detailed description of these distributions can be found in the online supplemental information (SI, Appendix A1).
The flux coefficients were calculated for particle diameters ranging from 1 nm to 10 μm, using 90 size sections uniformly spaced on a logarithmic scale; flux coefficients were calculated for particle charge states up to ± 30 charges. The following input parameters were varied when calculating the flux coefficients: temperature (T) and pressure (p), relative permittivity of the particles (χp), ion mobility (Zi), relative permittivity of the ions (χi), and ion mass (mi).
The flux coefficients were calculated for 35, logarithmically spaced values of the ion mobility in the range 0.5–2.5 cm2 V−1 s−1. The range was chosen such that it covers the values observed in both the measured distribution and the SR distribution. The corresponding ion masses were calculated using the Stokes–Millikan law in the form[1] where mg is the mass of a carrier gas molecule, qi is the charge of the ion (one elementary charge), μ is the viscosity of the carrier gas, di,mass = (6mi/πρi)1/3 is the mass diameter of the ion, and Kn is the Knudsen number defined as 2λ/(di,mass+dg), where λ is the mean free path of the carrier gas. The effective diameter of a gas molecule, dg, was assumed to be 0.3 nm (Ku and de la Mora Citation2009), while the density of the ion, ρi, was used as a free parameter. The empirical coefficients, 1.257, 0.4, and 1.1, in the slip correction factor were according to Davies (Citation1945). The first term on the right-hand side of Equation (Equation1
[1] ) accounts for the finite mass of the ion, as suggested by Tammet (Citation1995). The polarization correction factor, L, is given as (Larriba and Hogan Citation2013):
[2a]
[2b] where ξ is the momentum scattering coefficient, ϕ = Upol((di,mass+dg)/2)/kBT with kB being the Boltzmann constant, and the polarization energy at distance r is given by
[3] where a is the polarizability of gas molecules (∼1.7 Å3 in air), χ0 is the vacuum permittivity, and χg is the relative permittivity of air. The empirical coefficients in Equation (Equation1
[1] ) correspond to ξ = 1.36, which does not hold exactly for all types of ions (Ouyang et al. Citation2013; Larriba-Andaluz et al. Citation2015). While a recent study by Maißer et al. (Citation2015) suggests that a single mobility–mass relationship is unlikely to work for the range of ions present in aerosol chargers, Equations Equation(1)–(3) have been shown to be in good agreement with experimental results (Larriba and Hogan Citation2013; Ouyang et al. Citation2013). To remove the small discontinuity at ϕ = 1 in Equations (Equation2a
[2a] ) and (Equation2b
[2b] ), we defined L as
[4] which results to better performance of iterative algorithms at ϕ ≈ 1, but has negligible effect on the actual values of L.
We chose T = 298 K and p = 96,757 Pa to represent laboratory conditions in Pasadena, CA, where the ion mobilities were measured. When other values of T and p were used when calculating the flux coefficient, the ion mobility in those conditions was calculated according to Equation (Equation1[1] ) assuming that the ion mass stays constant.
The input parameters used in the calculations are summarized in . The values of T and p correspond to conditions in our laboratory (Scenarios 1–7 and 10–13), conditions at 3 km altitude (Scenario 8), and conditions at 10 km altitude (Scenario 9). The altitudes of 3 and 10 km were chosen to approximately correspond to the maximum flight altitude of an unpressurized aircraft and a common flight altitude of a commercial passenger jet, respectively. Lacking data to guide a simulation under those conditions, we employ the same aerosol scenarios as in the laboratory conditions. The particle was assumed to be conductive (χp = ∞), polystyrene (χp = 2.6), a commonly used material for instrument calibration, or NaCl (χp = 6), the main component of sea-salt aerosol. The chosen values of the relative permittivity of the ion correspond to conductive material (χi = ∞), air at room temperature (χi = 1.00059), or NaCl (χi = 6). The values of ρi were chosen to cover a wide range of reasonable values, including the bulk densities of major chemical compounds in ions as identified by Ehn et al. (Citation2010).
Table 1. Simulation scenarios used when calculating the charge distributions. For Scenarios 1–7 and 12–13, the flux coefficients were calculated for 35 values of ion mobility, Zi, that covered the range 0.5–2.5 cm2 V−1 s−1. For Scenarios 8 and 9, the ranges were 0.65–3.1 cm2 V−1 s−1 and 1.7–7.0 cm2 V−1 s−1, respectively. For Scenarios 10 and 11, only the mean and median, respectively, of negative and positive ion mobilities were used. For each scenario, the calculations were performed using the measured ion mobility distribution and the SR distribution, with the ion mass and mobility values corresponding to the latter given in parenthesis.
We also probed the influence of using effective ion properties for Scenario 1, as in Hoppel and Frick (Citation1986), by using f calculated using only the mean, Zi,ave, or median, Zi,med, mobility of the ion mobility distribution, and setting the ratio of total concentration of positive and negative ions, ni,T+/ni,T−, to 1.1 or 1.2, instead of 1, which was used otherwise. ni,T+/ni,T− = 1 is often assumed for bipolar chargers based on the assumption that the processes governing the ion concentrations are the ion production by energetic particles, and removal due to ion–ion recombination, both of which affect the negative and positive concentrations in exactly the same way. Differences of ion mobilities can, however, introduce a polarity bias as particles are lost to surfaces of the charger or downstream plumbing. The values of 1.1 and 1.2 were arbitrarily chosen, but are of reasonable magnitude based on previous studies (Hoppel and Frick Citation1990; Tigges et al. Citation2015). In the case of varying the value of ni,T+/ni,T−, the shapes of the mobility distributions of negative and positive ions were kept constant. It should be noted that the value of ni,T+/ni,T− affects the charge distribution, but not the flux coefficients.
2.2. Aerosol dynamic simulations
Recent observations of nucleation events in a wide range of atmospheric conditions have created much interest in measurements of ultrafine aerosol particles that could not be quantified at the time the FHFW charge distribution model was developed (Kulmala et al. Citation2004; Kulmala and Kerminen Citation2008), and stimulated many advances in aerosol instrumentation, including two-stage condensation particle counters (Iida et al. Citation2009) and mobility analyzers suitable for sub-10 nm particle measurements (Rosser and de la Mora Citation2005; Brunelli et al. Citation2009; Mui et al. Citation2013, Citation2017). Thus, atmospheric nucleation events in clean and polluted environments were selected for this study of potential biases in mobility-based particle size distribution measurements.
Since knowledge of the charge distribution is incomplete, we probed potential biases using the aerosol evolution simulations during a new particle formation event, thereby eliminating measurement biases from the baseline data. The new particle formation events were simulated using the Ion-UHMA model (University of Helsinki Multicomponent Aerosol Model for neutral and charged particles; Leppä et al. Citation2009) and covered a particle diameter range from 1.6 nm to 1.0 μm using 240 logarithmically spaced size sections. The parameters used as input in the model were the formation rate of 1.6 nm particles, concentrations of condensing vapors, initial particle size distribution, and the increase in the height of the boundary layer, which was modeled by diluting particle concentrations as if being mixed with particle free air. The values for these parameters were arbitrarily chosen to produce new particle formation events with characteristics similar to those observed in the field measurements at Hyytiälä, Finland, (Mäkelä et al. Citation1997), at Mukteshwar, India (Neitola et al. Citation2011; Hyvärinen et al. Citation2011), and New Delhi, India (Mönkkönen et al. Citation2005). These locations were chosen to represent clean background conditions (Hyytiälä), polluted background conditions (Mukteshwar), and polluted urban conditions (New Delhi).
2.3. Conversion of simulated data into a synthetic signal: Pseudo-instrument
The new particle formation event simulations provided the time evolution of the particle size distribution. Using models of instrument performance, called transfer functions, these particle size distributions were translated into a signal emulating actual instruments. This conversion is referred to as pseudo-instrument (). We first calculated the particle mobilities, Zp, corresponding to the particle diameters in the simulation grid according to Equation (Equation1[1] ) assuming singly charged particles with a density of 1500 kg m−3. We then made a grid of 100 diameters, dS, covering size range from 1.0 nm to 1.0 μm using logarithmic steps, to represent the channels of the pseudo-instrument, and calculated the mobilities, ZS, corresponding to those channels. The signal, Sm, for a channel m was then calculated according to
[5] where Zp,l is the electrical mobility of a singly charged particle corresponding to section l in the mobility grid of the simulation, f±j(Zp,l) is the fraction of negative or positive particles with mobility Zp,l and charge state j, nT(Zp,l) is the concentration of particles with mobility Zp,l, and w is the number of sections in the mobility grid. The transfer function of the pseudo-instrument, Ωm, describing the probability that a particle with electrical mobility jZp,l is detected in channel m (corresponding to electrical mobility ZS,m), was defined as
[6]
Figure 1. Processes involved in a typical particle size distribution measurement (left), and in the present simulation approach (middle); schematic illustrations of these steps (right). Note that particles with the same size but different charge state fall into different mobility sections in the signal grid, since Zp(q) = qZp(q = 1) (two topmost panels on the right). Furthermore, negative and positive particles provide separate signals, with neutral particles not detected.
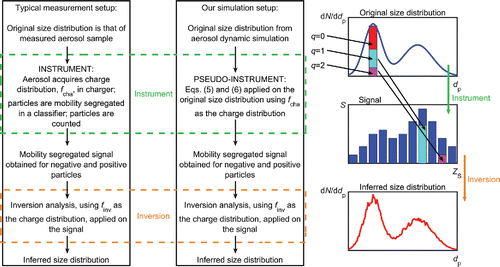
In other words, for the simplicity of the presentation, the transfer function was assumed to be a triangle on a logarithmic mobility axis, i.e., the transfer function of a channel with nominal mobility of ZS,m has the maximum value of 1 at the mobility jZp,l = ZS,m and the value decreases as a function of log(jZp,l) on both sides of the maximum reaching 0 at jZp,l = ZS,m-1 and at jZp,l = ZS,m+1. Consecutive channels overlap in a way that the sum of the transfer functions is unity over the mobility range, i.e., all particles are sampled with identical efficiency. Ωm is very similar to the ideal transfer function presented by Knutson and Whitby (Citation1975), except for the function being triangular on a logarithmic mobility axis instead of linear one. The exact shape of the chosen transfer function does not affect the conclusions of this study, however, provided that the transfer function is reasonably similar to those used to characterize actual instruments and, especially, that the same transfer function is used both in the pseudo-instrument and in the inversion algorithm. Justifications for the chosen transfer function and the number of mobility channels used in the pseudo-instrument are provided in the SI (Appendix A4). The detection efficiency of the pseudo-instrument was assumed to be unity and wall losses were assumed to be negligible. Neither of these approximations is valid for actual instruments over the entire particle diameter range considered in this study, but if the detection efficiency and wall losses are known and used correctly in the inversion, they will not introduce additional biases. Exploring those biases is not in the scope of this study, which examines only the biases associated with the charge distribution. It should be noted that when using Equations (Equation5[5] ) and (Equation6
[6] ), the signal consists of concentrations as a function of electrical mobility with the multiply charged particles contributing to the signal corresponding to a higher mobility than that of the singly charged particles of the same size (see “Instrument” in ).
The charge distributions used in Equation (Equation5[5] ) for the different simulation Scenarios are those listed in , representing the charge distributions acquired in the charger, fcha. With the procedure described above, the pseudo-instrument emulates an instrumentation setup employing a bipolar aerosol charger followed by an idealized classifier, such as DMA, and a detector, such as CPC; complexities of diffusional broadening of DMA transfer functions, losses, and sigmoidal counting efficiency curves for the detector are not relevant to our present purpose, and are not considered.
The signals were calculated from the data of Hyytiälä simulation (Section 2.2) using the charge distributions corresponding to all Scenarios listed in . Additionally, the signals were calculated using the data from the Mukteshwar and New Delhi simulation with charge distribution corresponding to either Scenario 1 or 9. Motivation for our choice of charge distributions is provided in Section 3.2.3. Regardless of the charge distribution and simulation data used, the signals were calculated for both negative and positive polarities.
2.4. Inferring the particle size distributions from the synthetic signals
Particle size distributions were calculated from the synthetic signals described in Section 2.3 using an inversion algorithm (“Inversion” in ). The inversion algorithm uses an interior-point gradient method for solving totally nonnegative least-square problems (Merritt and Zhang Citation2005). In the inversion, the detection efficiency of the detector and the transfer function of the classifier were assumed to be the same as in the pseudo-instrument described in Section 2.3.
We examine the effects of different assumptions regarding the charge distribution, by employing distributions, finv, that were derived based upon different sets of ion and particle parameters than those used to obtain the “true” charge distribution in the pseudo-instrument, fcha. Because the pseudo-instrument is otherwise perfectly characterized in the inversion algorithm, the differences between the simulated and the corresponding inverted distributions can be attributed to the difference in the charge distributions, fcha and finv. The present simulations therefore reveal the uncertainty imposed by imperfect knowledge of the actual charge distribution.
For practical reasons, the maximum number of particle charges considered in the inversion, qmax, needs to be limited. In order to study a possible bias in the inferred particle size distribution caused by too small of a value of qmax, we used values of 3, 6, and 9 in the inversion, even though the value used when calculating the signal was 30. These limited values of qmax were only applied to inversion using the charge distribution corresponding to Scenario 1; qmax = 15 was used in the inversion otherwise.
The uncertainties in the DMA-based particle size distribution measurements are not expected to result from the numerical inaccuracies in the inversion algorithm per se (Wiedensohler et al. Citation2012). If the assumptions the inversion algorithm is based on do not characterize the instrument properly, however, biases will arise.
3. Results
3.1. Aerosol dynamic simulations, signal, and inversion analysis
The time evolution of the particle size distributions in the Hyytiälä, Mukteshwar, and New Delhi simulations is shown in . Each of these three simulations captures a new particle formation event with the nucleation mode particles growing into Aitken regime. The total particle concentrations and the relative concentrations in different size ranges differed significantly among these scenarios. While the simulated particle diameter range was 1.6–1000 nm, the results are shown for 2–1000 nm; signals from particles smaller than 2 nm in diameter represent a convolution of charger ions and aerosol particles (see the SI, Appendix A1).
Figure 2. Top row: Time evolution of the particle size distribution in Hyytiälä (left), Mukteshwar (middle), and New Delhi (right) simulations. The color denotes the particle size distribution, dN/dlogdp, in cm−3. Bottom left: Time evolution of the particle size distribution inferred from the signal of the Hyytiälä simulation. Bottom middle: The ratio, R(dp,t), of the inverted (lower left panel) and simulated (upper left panel) distributions. The subscript “sim” and “inv” refer to the simulated and inverted distributions, respectively. Bottom right: The time averaged ratio of the inverted and simulated distributions, R*,−, and its running average, R*,−ave, according to Equations (Equation8[8] ) and (Equation9
[9] ), respectively. The data in the lower panels are shown for Hyytiälä simulation with fcha = finv and when negative particles were counted; the results for positive polarity were very similar.
![Figure 2. Top row: Time evolution of the particle size distribution in Hyytiälä (left), Mukteshwar (middle), and New Delhi (right) simulations. The color denotes the particle size distribution, dN/dlogdp, in cm−3. Bottom left: Time evolution of the particle size distribution inferred from the signal of the Hyytiälä simulation. Bottom middle: The ratio, R(dp,t), of the inverted (lower left panel) and simulated (upper left panel) distributions. The subscript “sim” and “inv” refer to the simulated and inverted distributions, respectively. Bottom right: The time averaged ratio of the inverted and simulated distributions, R*,−, and its running average, R*,−ave, according to Equations (Equation8[8] ) and (Equation9[9] ), respectively. The data in the lower panels are shown for Hyytiälä simulation with fcha = finv and when negative particles were counted; the results for positive polarity were very similar.](/cms/asset/0870d92f-13f6-40f3-80d2-574baa18e7d8/uast_a_1341039_f0002_oc.gif)
To assess the reliability of our theoretical approach (), we used the same charge distribution both when computing the synthetic signal with the pseudo-instrument and when inferring the size distribution with the inversion analysis, i.e., fcha = finv. In this case, there was no bias caused by the difference in the charge distributions used in different phases of the analysis; any difference between the simulated and inverted distributions resulted from numerical inaccuracies. Both the simulated distribution and corresponding inverted distribution for the Hyytiälä simulation are given in . The ratio of the two, R(dp,t), is also shown. The ratio of the inverted and simulated distribution, R(dp,t), as a function of time, t, and diameter is[7] where (dN/dlogdp)sim = nsim and (dN/dlogdp)inv = ninv refer to simulated and inverted distributions, respectively. The value of R(dp,t) for any particular particle size varied little with time over the periods during which the concentration of particles in that size was significant. This feature allowed us to remove the time dependence in R(dp,t) by calculating the time-averaged ratios of inverted to simulated distribution, R*(dp), using the simulated distribution as the weighing factor, and summing over v particle size distributions in the time series, i.e.,
[8] When fcha = finv, the value of R*(dp) fluctuated on both sides of unity with the vast majority of values being within 10% of unity (). This fluctuation was a numerical issue caused by switching from one grid to another along the analysis (): the particles in diameter grid corresponding to simulation are converted to a signal with a mobility grid, and the signal is then converted to a particle size distribution corresponding to another diameter grid. To better visualize the results, we calculated the running five-point average of the R*(dp), R*ave(dp), as
[9] where u is the number of diameters, dp,l, in the diameter grid of the inverted distribution. For the smoothed data with fcha = finv, R*ave(dp) deviated from unity by less than 2%, except at the very ends of the diameter range. Thus, any larger deviation of R*ave(dp) was likely caused by the difference between fcha and finv.
3.2. Bias in the inferred particle size distribution due to fcha ≠ finv
As was seen when fcha = finv, R(dp,t) did not show any systematic variation with time even when fcha ≠ finv (data not shown), so we employ R*ave(dp) to explore the biases resulting from employing erroneous charge distribution models in the data inversion, i.e., when the charge distribution used in the inversion differs from the actual charge distribution on the aerosol that enters the instrument (i.e., the “true” distribution; ). We begin with a case that represents a typical situation in measurements: the charger generates a certain fcha on the sampled aerosol, but values found in the literature are used for finv. Common data analysis procedures have applied the FHFW charge distribution to measurements made at a range of temperatures and altitudes, implicitly assuming that the charge distribution determined for sea level ambient conditions is applicable to disparate environmental conditions. To examine the biases that may result from this mismatch, we examine the differences in particle size distribution estimations that result when finv is determined under different environmental conditions than fcha. For charge distribution estimations, both fcha and finv were calculated using either the measured or SR ion mobility distributions. The environmental conditions examined, and the resulting values of R*ave are given in for different particle diameter ranges. The results shown focus on the observed biases in the inferred particle size distribution, but the differences in the flux coefficients resulting to those biases are provided in the SI (Appendix A2).
Table 2. Biases observed in the particle size distributions inferred from measurements in which the charge distribution used in the pseudo-instrument differed from that in the inversion analysis, i.e., fcha ≠ finv. The ranges of biases are given separately for negative and positive particles, R*,−ave and R*,+ave, respectively, and for particles smaller than or larger than 10 nm in diameter. The values in parenthesis were obtained using the SR distribution instead of the measured distribution. A value of R*,±ave that differs from unity reveals underestimation or overestimation of the particle concentration. The first row provides the baseline-case, i.e., fcha = finv (according to Scenario 1). In the second row, fcha was that from Scenario 1, but FHFW charge distribution was employed in the inversion. In the third row, fcha was calculated using the measured distribution, while finv was calculated using SR distribution, with both being according to Scenario 1. In other rows, the parameter that was changed when calculating fcha and finv is given in the first column, with the values used when calculating fcha and finv given in the second and third column, respectively, the other inputs were the same.
3.2.1. Bias in the inferred particle size distribution due to using literature values for finv
Let us assume that the conditions of Scenario 1 represent the conditions in the charger, in which case the fcha is the charge distribution calculated for those conditions. For typical measurements, however, the finv is estimated using a parameterization that was derived by Wiedensohler (Citation1988) from the theoretical results of Hoppel and Frick (Citation1986). These two charge distributions differ significantly (see the SI, Appendix A2). Before we examine the effects on full particle size distribution measurements, consider a monodisperse aerosol for which particles acquire no more than one charge. In this case, the charge distributions acquired in the charger and used in the inversion are, fcha = {f-1,cha, f0,cha, f1,cha}, and finv = {f-1,inv, f0,inv, f1,inv}, respectively, and we can write Equation (Equation5[5] ) as
[10] where S± is the signal when negative or positive particles were counted, kmax = 1, nsim is the number concentration in the simulation, and we assume that there exists a channel with nominal mobility equaling the mobility of the monodisperse distribution, i.e., Ω = 1. With those assumptions, the number concentration after the inversion becomes
[11]
Using Equations (Equation10[10] ) and (Equation11
[11] ), we can write the ratio of concentrations in the simulation and after the inversion as
[12] from which we see that if the fractional population used in the inversion, f±1,inv, is higher than the one acquired in the charger, f±1,cha, then the particle size distribution is underestimated and vice versa. In the case of a polydisperse aerosol, especially one that includes large, multiply charged particles, the situation is more complicated, but the same logic still holds.
As expected based on Equation (Equation12[12] ), and noting that fcha was considerably lower than literature estimates (see the SI, Appendix A2), the concentrations of particles smaller than 15 nm were significantly underestimated when the measured ion mobility distribution was used (). Depending on whether negative or positive particles were counted, the concentrations of the smallest particles could be underestimated by a factor of 5, though the bias factor decreases with increasing particle size, reaching a level of ∼1.5 at dp = 15 nm. For particles with dp > 15 nm, the biases for both polarities vary between underestimation by a factor of 1.5 for the largest positively charged particles and overestimation by a factor of 1.2 for negatively charged particles. The magnitude of the bias did not vary significantly among the aerosol scenarios for Hyytiälä, Mukteshwar, or New Delhi.
Figure 3. Left panel: The bias observed in the inferred particle size distribution when fcha was calculated using input values based on our measurements (Scenario 1 in ) and finv was according to FHFW charge distribution. The line style denotes the simulation, and signal polarity is labeled. Right panel: The same as the left panel, except that the SR distribution, instead of the measured distribution, was used when calculating fcha.
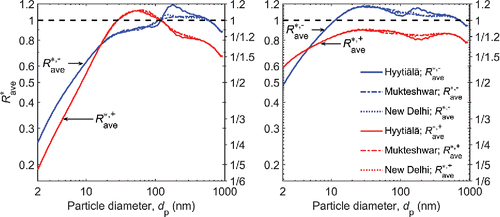
The biases observed when SR distribution was used for fcha were similar to those when the measured distribution was used, with the following differences: the concentration of the smallest particles was underestimated by a factor of 2 and the bias for particles with dp > 15 nm was at most 30%. Further, if negative (positive) particles were counted, the concentration was overestimated (underestimated) for the range of 15 nm < dp < 700 nm.
It should be noted that the bias in the particle size distribution resulting from the Wiedensohler parameterization reflects the difference between the parameterization and the charge distribution that corresponds to the measured and SR distributions in the ambient conditions of our measurements. As temperature, pressure, and properties of ions and particles change in different measurement environments, the fcha also changes, as does the bias.
3.2.2. Bias in the inferred particle size distribution due to ion mobility distribution
The mean mobilities of charger ions found in the literature vary from 0.97 cm2 V−1 s−1 (Lee et al. Citation2005) to 2.09 cm2 V−1 s−1 (Steiner and Reischl Citation2012) with the mobilities of the positive ions being systematically lower than those of the negative ones observed for the same measurement setup (see Tigges et al. Citation2015, for a review of literature values). Compared to the literature values, the mean mobilities of the measured distribution in this study, Zi,ave+ = 1.12 cm2 V−1 s−1 and Zi,ave− = 1.53 cm2 V−1 s−1, are closer to the low mobilities, while the mean mobilities of SR distribution, Zi,ave+ = 1.66 cm2 V−1 s−1 and Zi,ave− = 2.14 cm2 V−1 s−1, are found at the other extreme. When the measured distribution was used for fcha and the SR distribution for finv, the concentrations of 2 nm particles were underestimated by a factor of 3 when the positive particles were counted (). The bias was observed to decrease with increasing particle size, and a bias of less than 25% was observed for sizes >15 nm, with overestimation observed in the size range of 21 nm < dp < 230 nm. If negative particles were counted, the concentrations of 2 nm particles were underestimated by a factor of 1.9 with the bias again decreasing with increasing particle size, and a bias <10% was observed for particles >110 nm in diameter. The magnitude of the bias did not vary significantly among the aerosol scenarios, i.e., Hyytiälä, Mukteshwar, or New Delhi.
Figure 4. The bias observed in the inferred particle size distribution when fcha was calculated using the measured ion mobility distribution and finv was calculated using the SR distribution, with other inputs being the same. The line style denotes the simulation, and signal polarity is labeled.
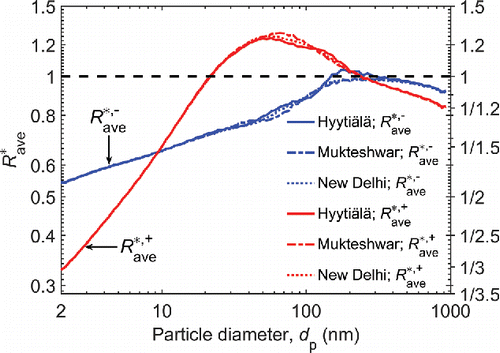
In Sections 3.2.3–3.3, either the measured or SR distribution was used when calculating both the fcha and the finv, with the results presented separately for both distributions.
3.2.3. Bias in the inferred particle size distribution due to temperature and pressure effects
Most size distribution measurements are conducted at the ground level, but airborne measurements are increasingly used in climate change research (Clarke et al. Citation1998; Mirme et al. Citation2010; Coggon et al. Citation2014), as are measurements at alpine sites that allow long-term probing of the free troposphere (Weingartner et al. Citation1999). While T and p are typically measured in conjunction with particle size distribution measurements, they are typically not, however, taken into account when estimating finv. To examine the biases that may result when the ion distribution obtained at one altitude are used to interpret mobility distribution obtained at a different altitude, we calculated the signal using the charge distribution corresponding to conditions at 3 and 10 km altitude, but used the charge distribution corresponding to T and p at laboratory conditions in the inversion, as has been done in most studies involving measurements at higher altitudes to date. Lacking data to guide a simulation under these conditions, we employ the same aerosol scenarios. At 3 km altitude, when the measured distribution was used and negative (positive) particles were counted, the observed particle size distribution bias factor was as large as 1.32 (1.22), with underestimation being more frequent when dp < 300 nm (170 nm) and overestimation more frequent in sizes >300 nm (170 nm) (). When the SR distribution was used, the biases were qualitatively similar, but the magnitudes were slightly smaller, with the maximum bias factor being 1.17 (1.11) when negative (positive) particles were counted. At 10 km altitude, the bias was even larger than at 3 km altitude. The highest overestimation was about two-fold at dp = 2.0 nm for both the measured and SR distribution when positive particles were counted. When negative particles were counted, the concentration at dp = 2.0 nm was overestimated by a factor of 1.89 for the SR distribution, but underestimated by a factor of 1.46 for the measured distribution. This was the only occasion in which the observed bias due to the parameter(s) being probed, T and p in this case, was considerable, but qualitatively different for the measured and SR distributions. Except for the dp < 30 nm when negative particles were counted, the biases with the measured and SR distributions were qualitatively similar, though the biases were in general somewhat smaller in the case of SR distribution.
Figure 5. Upper panels: The bias observed in the inferred particle size distribution when fcha corresponded to T and p representing conditions in laboratory (T = 298.15 K and p = 96,757 Pa; “Lab.”), at 3 km altitude (T = 269 K and p = 70,120 Pa; “3 km”), or at 10 km altitude (T = 223 K and p = 26,500 Pa; “10 km”), and finv corresponded to T and p in laboratory. The line style denotes the conditions, with markers denoting different simulations, shown only in the “10 km” case. Results corresponding to negative (positive) particle measurements are shown on the left (right). The lines depicting the “10 km” cases are practically coincident for dp < 20 nm and dp > 500 nm. Lower panels: The same as the upper panels, except that the SR distribution, instead of the measured distribution, was used when calculating fcha.
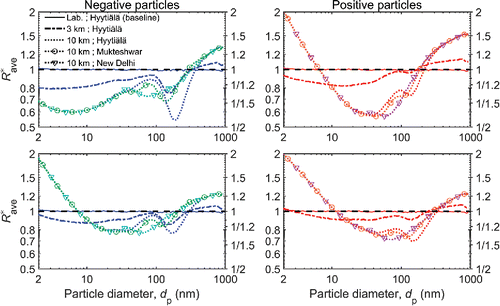
The bias in the particle size distributions of both the Mukteshwar and New Delhi simulations were similar to those for Hyytiälä (). For dp < 30 nm, the biases differed little between the simulation scenarios, as expected from Equation (Equation12[12] ) because the vast majority of the particles in that size range carried at most one charge. Moreover, the biases for dp > 570 nm differed little because multiply charged larger particles did not contribute significantly to the signal for those sizes, which was, at least partly, due to lack of >1 μm particles considered in the simulation scenarios. Over the 30 nm < dp < 570 nm size range, the biases were qualitatively similar and within a factor of 1.3 from each other, except in the case of the measured distribution and negative particles being counted, when the bias in the Hyytiälä simulation was by a factor of ∼1.5 smaller than that of the other two simulations. This was the largest difference in the biases between the three simulation scenarios observed in this study. We will therefore focus our discussion in the remainder of this section on the Hyytiälä simulation.
3.2.4. Bias in the inferred particle size distribution due to material properties of charger ions and measured particles
Since the masses of the charger ions were not measured, we estimated them according to Equation (Equation1[1] ) by making an assumption on the effective density of the ions, ρi. The real value of ρi probably varied between ion species, but a single value was used for the whole ion distribution for the sake of simplicity. This approximation does not affect the conclusions of this study. Based on the values reported in literature (Ehn et al. Citation2011), we used ρi = 1500 kg m−3 when calculating finv. The resulting ion masses ranged from 220 Da to 1800 Da, corresponding to mobility range of the measured ions, 0.6–1.6 cm2 V−1 s−1, or from 55 Da to 840 Da, corresponding to mobility range of the SR distribution, 0.9–2.5 cm2 V−1 s−1. When the ion masses used to calculate fcha differed significantly from this value, a considerable bias in the inferred particle size distribution was observed (). If the ion masses were smaller (larger) when calculating fcha than when calculating finv, the ninv was overestimated (underestimated), i.e., R*ave(dp) > 1 (R*ave(dp) < 1). The bias was the largest for the smallest sizes because a low ion mass increases the charging probability. The biases were slightly smaller on average for the SR distribution than for the measured distribution.
Figure 6. Left panel: The bias observed in the inferred particle size distribution when the ion masses ranged from 130 to 1000 Da (ρi = 800 kg m−3), from 220 to 1800 Da (ρi = 1500 kg m−3), or from 300 to 2700 Da (ρi = 2200 kg m−3) when calculating fcha, but were from 220 to 1800 Da when calculating finv, as indicated in the legend. Right panel: The same as the left panel, except that the SR distribution, instead of the measured distribution, was used when calculating fcha. The corresponding ion masses are given in the legend.
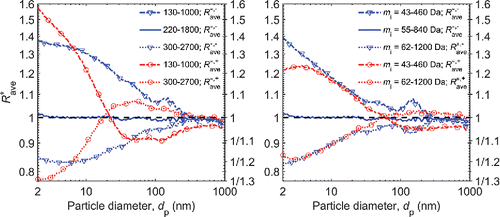
In most particle size distribution measurements, the chemical composition of the measured particles, and, therefore, their relative permittivities, χp, are unknown. Notable exceptions occur in the use of a known aerosol for calibration purposes, and, of course, when the chemical composition is measured in conjunction with the particle size distribution. The Hoppel and Frick (Citation1986) simulations on which most DMA data inversions to date have been based were based on conductive particles. Assuming particles to be conductive when calculating finv leads to underestimation of the particle concentrations, especially for sizes <200 nm in diameter, when the permittivity used when calculating fcha was considerably lower (). Regardless of whether negative or positive particles were counted and whether the measured or SR distribution was used, when fcha was calculated for χp = 2.6, corresponding to the common material of calibration aerosol particles, polystyrene, and conductive particles, χp = ∞, were assumed when calculating finv, particle concentration estimates were 40%–50% low at nanometer sizes. Assuming NaCl particles, χp = 6, instead of polystyrene when calculating fcha decreased the bias to about 20%. Thus, conductive particles represent a reasonable approximation, even for compounds with relatively small relative permittivity.
Figure 7. Left panel: The bias observed in the inferred particle size distribution when particles were assumed to be conductive, χp = ∞, or dielectric with χp = 6 or χp = 2.6 when calculating fcha, but they were assumed to be conductive when calculating finv, as indicated in the legend. Right panel: The same as the left panel, except that the SR distribution, instead of the measured distribution, was used when calculating fcha.
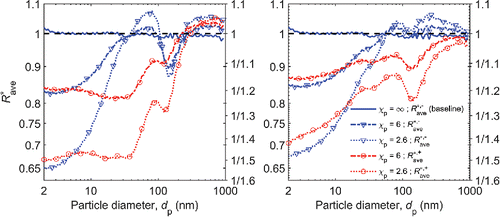
Minor biases, typically <5%, in the particle size distribution were observed due to incorrect assumption on the relative permittivity of the ions or describing the whole ion mobility distribution using only the mean or median mobility when calculating the charge distribution in the inversion, or due to truncation of the number of charge states considered in the inversion. Biases were found to be smaller when the mean mobility was used instead of the median, and considering at least six charge states was sufficient to remove any considerable bias. It should be noted that a higher number of charge states could be needed in other situations, especially if particles larger than 1000 nm in diameter were included (López-Yglesias and Flagan Citation2013b). More details about this part of the study can be found in the SI (Appendix A3).
3.2.5. Bias in the inferred particle size distribution due to relative concentrations of positive and negative ions
It is often assumed that the loss of ions in a bipolar charger is dominated by the ion–ion recombination, which results in symmetric concentrations of negative and positive ions. While we did not observe any considerable difference in the negative and positive ion concentrations, the higher mobility of negative ions could result in a smaller concentration of negative than positive ions due to losses occurring between the charger and the electrometer. Furthermore, the aerosol charge distribution could change between the charger and the classifying unit due to attachment of the charger ions in the stream following the charger (Hoppel and Frick Citation1990; Tigges et al. Citation2015). Differences in the negative and positive ion concentrations would be expected if ion loss by attachment to particles was substantial. In that case, however, a greater concern would be whether the charge distribution reaches steady-state or not (de la Verpilliere et al. Citation2015; Tigges et al. Citation2015), but that is beyond the scope of this study.
To explore the role of uncertainties in the charger ion imbalance on the inferred particle size distributions, we examined scenarios in which ni,T+/ni,T− = 1.0, 1.1, and 1.2 when calculating fcha, but applied the common assumption of ni,T+/ni,T− = 1.0 when calculating finv. Underestimating the value of ni,T+/ni,T− when calculating finv resulted in underestimating the particle concentrations, i.e., R* < 1 when probing negative particle signal, and R* > 1 for positive particles (). This can be demonstrated for singly charged particles, by writing the fractional population as (Hussin et al. Citation1983; Gagné et al. Citation2012)[13] where βq± is the flux coefficient of positive or negative ion with a particle with q number of charges. For singly charged, monodisperse particles, by denoting the value of ni,T+/ni,T− when calculating f±1,cha and f±1,inv as rcha and rinv, respectively, Equations (Equation12
[12] ) and (Equation13
[13] ) yield
[14]
Figure 8. Left panel: The bias observed in the inferred particle size distribution when ni,T+/ni,T− was 1.0, 1.1, or 1.2 when calculating fcha, but ni,T+/ni,T− = 1.0 was used when calculating finv. The line style denotes the ni,T+/ni,T− when calculating fcha, and the marker and line color denote the signal polarity. Right panel: The same as the left panel, except that the SR distribution, instead of the measured distribution, was used when calculating fcha.
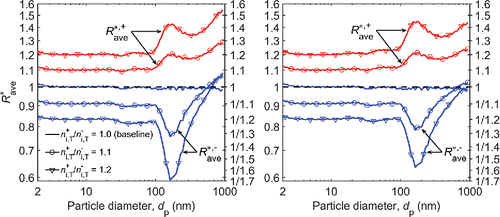
Equation (Equation14[14] ) accurately represented the bias in the particle size distribution due to different ion concentrations for dp < 25 nm, i.e., when the majority of particles were singly charged (). For sizes >25 nm, multiply charged particles affected the bias, especially when dp > 100 nm. The observed bias was not sensitive to whether the measured distribution or the SR distribution was used.
3.3. Bias in the total particle concentration
In Section 3.2, the bias in the particle size distribution due to various parameters affecting the aerosol charge distribution was presented. This section covers how those biases translate to bias in the total particle concentration. Unlike the biases in the particle size distribution, the biases in the total particle concentrations were time dependent. In all three simulation scenarios, i.e., Hyytiälä, Mukteshwar, and New Delhi, the most important process changing the particle size distribution was the new particle formation and growth event (). Consequently, in some cases, a considerable time variation was observed in the total particle concentration bias (). When time variation in the particle concentration bias was observed, one of the extreme (high or low) was typically seen during the new particle formation event. For that reason, the values given in denote both the range of bias and the value of bias during the moment of highest particle concentration, which occurred during the new particle formation in all three simulation scenarios.
Table 3. The biases, ninv/nsim, observed in the total particle concentrations that result from using different charge distributions in the pseudo-instrument than in the inversion analysis, i.e., fcha ≠ finv. The biases are given as a range from minimum to maximum bias during the simulation followed by the bias observed at the instance of the highest particle concentration. The values in parenthesis were obtained using the SR distribution in place of the measured ion mobility distribution presented in this study. The biases are given separately for particle size ranges of 2–1000 nm and 10–800 nm, and also for particle size distributions based on counting negative and positive particles.
The observed biases in the total particle concentration exceeded 50% in the following three cases: (Equation1[1] ) the fcha was calculated for the laboratory conditions, but finv was according to Wiedensohler (Citation1988); (2) the measured distribution was used for fcha, but the SR distribution was used for finv; (Equation3
[3] ) the fcha was calculated for conditions at 10 km altitude, but finv was calculated for laboratory conditions (). The highest bias, underestimation by a factor of ∼3, was observed for the first case listed above, but only if the measured distribution was used and only for the Hyytiälä and New Delhi simulations; the total particle concentration was less affected by the new particle formation in the Mukteshwar simulation. In the first case listed above, if the SR distribution was used instead of the measured one, the highest observed bias was underestimation by a factor of 1.5, again only for the Hyytiälä and New Delhi simulations. Further, biases above a factor of 1.2 were observed if, when calculating finv, the ion masses were overestimated, the ni,T+/ni,T− = 1.2 was not accounted for, or polystyrene particles were assumed to be conductive.
3.4. Charge distribution uncertainty as an explanation to bias observed in the DMA-based measurements of total particle concentrations
Recently, Wiedensohler et al. (Citation2012) conducted multiple DMA intercomparison workshops with one of the aims being to determine the present uncertainties of particle mobility spectrometers, such as scanning mobility particle sizer (SMPS) and differential mobility particle sizer (DMPS). In that study, the FHFW charge distribution (Wiedensohler Citation1988) was applied when inverting the signal from each instrument. As the instruments were sampling the same aerosol, it is reasonable to assume that the ion species in the charger of each of the instruments were similar, unless the ion production was influenced by the prior use of the instrument (Steiner and Reischl Citation2012; Maißer et al. Citation2015). Differences in the charge distributions acquired in the instruments could still arise from the different charger types (Jiang et al. Citation2014; He and Dhaniyala Citation2014). Such differences could either lead to different ratios of negative to positive ion concentrations, or cause the aerosol to reach different non-steady-state bipolar charge distributions. The latter is not addressed in this study. The former effect would lead to consistent biases between instruments for dp < 25 nm (); instead, the observed biases exhibited a strong diameter dependence. In short, the differences between the particle size distributions obtained with different instruments were probably not caused by variation in the steady-state bipolar charge distribution, but the failure to achieve steady-state remains a possibility.
As shown in Section 3.3, biases in the inferred particle size distributions result in corresponding biases in the total particle concentrations, depending on the particle size distribution itself. In the study by Wiedensohler et al. (Citation2012), the total particle concentrations obtained by integrating the particle size distributions over the diameter range from 10 nm to 800 nm were observed to be smaller than those measured using a reference condensation particle counter (CPC). For short periods of time, underestimation in excess of 20% was observed when comparing the reference particle mobility spectrometer against the reference CPC; it was argued that this could be due to presence of considerable number of particles with dp ∼10 nm, only a fraction of which was counted by the particle mobility spectrometer. The results presented above suggest that these biases might, instead, result from differences between the assumed FHFW charge distribution and that acquired in the charger. The present results suggest that the bias was not likely to be caused by non-unity ratio of positive to negative ions; it is also unlikely that the T and p in those measurement conditions would have played a major role.
The FHFW charge distribution was derived for the charging of conductive particles by negative and positive ions with mobilities (masses) of 1.60 cm2 V−1 s−1 (130 Da) and 1.35 cm2 V−1 s−1 (148 Da), respectively. If the actual charger ions had been less mobile, considerably more massive, or if the measured particles were composed of a material with very low relative permittivity, underestimations of the observed magnitude would be expected (). The simplifying assumptions made by Hoppel and Frick (Citation1986) when calculating the flux coefficients could also contribute to the underestimation (López-Yglesias and Flagan Citation2013a). Thus, while the presence of particles near the lower detection threshold of the instruments could produce the observed difference between the particle concentrations obtained by integrating the particle size distribution and the CPC measurements, errors in the charge distribution assumed in the inversion are more likely at the root of this discrepancy than is the sensitivity of the DMA.
4. Discussion and conclusions
Because recent reexamination of the theory of aerosol particle charging revealed differences between the actual charging probability and that assumed in most DMA-based particle size distribution analysis, we examined the effect that such differences may have on how well particle size distributions determined from mobility-based measurements reproduce actual particle size distributions. To make the simulations relevant to ambient measurements, an experimentally measured ion mobility distribution emanating from a bipolar aerosol charger was applied to aerosols from common scenarios that span a wide range of sizes encountered in atmospheric measurements, namely, atmospheric nucleation events. However, ion properties have been shown to depend upon the charger used and, perhaps, even on the history of the experimental system due to trace species that may volatilize from walls of the apparatus. The influence of ion and particle properties on the particle size distribution inferred from DMA data was examined. We further examined the influence of temperature and pressure on the resulting charge distribution, and on the interpretation of DMA data. An idealized instrument was employed to span the size range of the aerosol particles to prevent bias in the inferred particle size distribution resulting from sources other than the charge distribution. Not surprisingly, when the charge distribution on the sampled aerosol particles differs from that assumed in the data inversion, biases result. We have quantified such biases for a range of scenarios.
The mean mobility of the measured charger ions was at the low end of values reported in the literature. When the charge distribution assumed in the inversion was based on an ion mobility distribution measured by Steiner and Reischl (Citation2012), which had a considerably higher mean mobility, the concentration of 2 nm particles was underestimated by up to a factor of 3. This bias decreased rapidly with increasing particle size, but over- and underestimation in the range of 1.2 to 1.5 was still observed over the range of 10 nm < dp < 100 nm.
Most DMA-based particle size distribution measurements to date have been interpreted using the charge distribution derived by Hoppel and Frick (Citation1986) using simulations based upon the theory of Fuchs (Citation1963), and reduced to a practical form in the parameterization by Wiedensohler (Citation1988). The nature of the gas ions responsible for particle charging was not known at the time of those pioneering works, so it is not surprising that biases occur, as measurements extend to particle sizes and measurement environments that could not be probed with instruments available at the time. Over the mid-range of particle sizes probed in most previous measurements, 20 nm < dp < 1000 nm, the FHFW charge distribution introduces relatively little bias in the measured particle size distribution. However, at the large and small particle extremes, the biases can become substantial; when FHFW charge distribution was assumed in the inversion, the concentrations of particles below 10 nm were underestimated by a factor of 1.6 to 5, when the charger ions were characterized by the mobility distribution presented in this study, or by a factor of up to 2, when the charger ion mobilities were according to Steiner and Reischl (Citation2012). This bias decreased rapidly with increasing size. Above 10 nm, the bias was less than 30% for most particle sizes, with underestimating (overestimating) the concentrations for negative (positive) charge measurements.
When particle size distribution measurements are performed at mountain-top sites, or at higher altitudes in airborne measurements, pressure and temperature effects lead to large deviations. Particle concentrations estimated from simulated measurements at 10 km altitude, were overestimated by a factor as large as 2 in the nucleation mode aerosol, i.e., particles smaller than a few nanometers in size. Again, the biases depend strongly on diameter, underestimating the concentrations for some sizes, and overestimating for others; at 100 nm, the inferred particle concentrations were 54% to 77% of the sampled particle concentration, depending on the ion mobility distribution. As expected, the bias was smaller at lower altitude (3 km), and generally resulted in underestimation. A bias as large as 27% was observed for dp < 100 nm.
Material properties also influence the data interpretation. The original Hoppel–Frick (Citation1986) charge distributions were calculated for conductive particles. For particles with a very low relative permittivity (dielectric particles), predicted concentrations could be underestimated if the charge distribution used in the inversion was assumed to be that for conductive particles. For polystyrene particles, χp = 2.6, concentration estimates were as much as 54% low for dp < 10 nm, with the bias decreasing with increasing particle size. For NaCl particles, χp = 6, the bias was reduced to half of that found for polystyrene particles. The ion relative permittivity, however, had negligible effect.
Though we measured the ion mobility distribution, the charge distribution calculations required an estimate of the mass of ions (for convenience, represented in terms of an effective bulk density of the ions). By estimating ρi = 1500 kg m−3, an over (under) estimation of up to a factor of 1.6 (1.3) was observed in the particle size distribution, if the actual ρi had been 800 kg m−3 (2200 kg m−3). The bias decreased with increasing particle size with negligible bias observed when dp > 300 nm.
The concentrations of negative and positive ions in the charger are often assumed to be the same, but a charge imbalance could result from differences in the loss rates of negative and positive ions due to ion–aerosol attachment (de la Verpilliere et al. Citation2015; Tigges et al. Citation2015) or as the aerosol passes through the transition between the charger and the detector (Hoppel and Frick Citation1990). Assuming charge balance (ni,T+/ni,T− = 1) in the inversion instead of a reasonable imbalance of ni,T+/ni,T− = 1.1 or 1.2 in the charger led to approximately 10% or 20%, respectively, under- or overestimation of the concentration of sub 20 nm particles, depending on signal polarity. For the small particles that acquired at most one charge, the bias in the inferred particle size distribution varied linearly with the magnitude of the error in the charge imbalance ratio. For larger particles that may acquire multiple charges, the bias varied strongly with diameter, reaching levels up to 70%. It should be noted that the process resulting in charge imbalance could also result to the aerosol not reaching a steady-state charge distribution, which could be an even more concerning issue (de la Verpilliere et al. Citation2015; Tigges et al. Citation2015), but beyond the scope of this study.
The simulations used to estimate the charge distribution in this study were computationally intensive. We have explored a broad range of the parameter space that may be encountered in mobility-based particle size distribution measurements, but, clearly, much more needs to be done for other measurement scenarios not examined here. To lessen the computational burden, we examined the effects of simplifying such simulations by using a single value of ion mobility, instead of whole mobility distribution. Using the mean ion mobility was found to result in a smaller bias than using the median ion mobility, with the maximum bias factors being 1.06 and 1.18, respectively.
Simulations were performed in which the maximum number of charges considered in the inversion was as low as 3; this simplified the inversion analysis considerably, but increased the bias in particle size distribution estimates for larger particles. Our simulations reveal that consideration of six charges eliminated the bias for submicron particles; more charges would be needed if supermicron particles were considered.
Three new particle formation event scenarios were examined in this study. The observed biases in the inferred particle size distributions were insensitive to the shape of the actual particle size distribution under consideration: in the most pronounced example within these simulations, the difference in the bias factors corresponding to these three scenarios only approached 30% for particles with dp > 300 nm, though a value as high as 50% observed over a very narrow size range. In general, the differences were much smaller than these extremes.
While the biases in the particle size distributions stayed constant in time, a considerable time variation was observed in the bias in the total particle concentration, especially during the new particle formation event. The largest bias, an underestimation by a factor of 3, was observed when the charge distribution acquired in the charger was calculated using parameters from our measurements, but the FHFW charge distribution was assumed in the inversion. Biases above a factor of 1.5 also occurred if the ion mobility distribution assumed in the inversion was substantially different from the one seen in the charger, or the conditions at 10 km altitude were not taken into account.
In a previous study, the particle concentration obtained by integrating the particle size distribution sometimes underestimated the total particle concentration when compared to a CPC measurement (Wiedensohler et al. Citation2012). The biases due to the charge distribution assumed in the inversion explained these underestimations. For the 10 to 800 nm particle size range, discrepancies up to 20% between total particle concentrations based on particle mobility spectrometer and CPC measurements could be attributed to uncertainty in the ion mass, relative permittivity of the particles, or concentration ratio of negative and positive ions. Considerably higher discrepancies could be explained by not properly accounting for the ion mobilities or T and p effects when calculating the charge distribution assumed in the inversion.
The weak link in differential mobility analysis of particle size distributions is the charge distribution, not the size/mobility resolution of the DMA. As convenient as it may be, there is not likely to be a one-size-fits-all charge distribution. Combined with recent studies that show strong variation in the charge distribution from one charger to another (Jiang et al. Citation2014; Kallinger and Szymanski Citation2015), the present study makes it clear that better understanding of the charge distribution is required to make mobility-based particle size distribution measurements truly quantitative. Using a different charge distribution in the inversion than that present on the aerosol particles introduces biases in the inferred particle size distribution. Moreover, measurements made in different environments may encounter different charge distributions. Steiner and Reischl (Citation2012) showed that the molecules that form ions in different chargers differ from one charger to another; they attributed this difference to contaminants accumulated during prior use of the device. Thus, even measurement history may be a factor. Measurements made at high altitude are particularly problematic.
While the biases can be substantial, the present results make it possible to constrain their magnitudes over the entire range for which DMAs are used to characterize atmospheric and other aerosols. By our current estimates, the most extreme variation arises when the FHFW parameterization (Wiedensohler Citation1988) is applied to particles as small as 1 nm, where deviations may approach an order of magnitude.
Unfortunately, at these very small sizes the current methods for determining the charge distribution also have the highest uncertainties. The main issue with the FHFW model rises from neglecting the influence of the strongly attractive potential on the speed and direction of movement of an ion in the vicinity of an oppositely charged particle. Furthermore, the FHFW model assumes that both the ion and the particle are spherical, which might not be a good approximation for some solid particles regardless of the particle size, but this approximation certainly becomes suspect when the particle size approaches molecular sizes. In the case of attractive potential, the FHFW model accounts for the possibility of one collision with a neutral gas molecule occurring inside the limiting sphere, i.e., three-body trapping, but if additional collisions occur, the flux coefficient may be underestimated. The Brownian dynamics (BD) model, on the other hand, accounts for the strongly attractive potential by construct, and can also account for non-spherical particle geometries (Gopalakrishnan et al. Citation2013a). Limiting approximations in the BD model are that the ions are modeled as point masses, with the ion mass assumed to be much larger than that of gas molecules, but substantially smaller than the particle mass. The accuracy of these approximations decreases with decreasing particle size and ion mass. Finally, both of these models assume that each ion-to-particle collision results in a charge transfer, which might not be a good approximation for sufficiently small particles depending on the chemical compositions of the ion and the particle. For both of these methods, however, the main limitation in using them to estimate the charge distribution for given measurement conditions is imperfect knowledge of ion and particle characteristics that are needed as input.
We have based these estimates on our current best estimate of the ion-to-particle flux coefficients, taking into account ion and particle properties as well as atmospheric conditions. Further work is required to fully characterize both the ions and the aerosol properties over the range of conditions at which these techniques are applied. Regardless of what is the true charge distribution, using the wrong one in inverting the data can lead to substantial biases. While we have only examined bipolar charge distributions, the same sources of uncertainty apply to techniques using unipolar charge distributions. Because of the fundamental importance of the charge distribution to mobility-based analysis of aerosols, a future article will provide parameterizations of attachment coefficients and steady-state bipolar charge distributions as a function of ion and particle properties, as well as atmospheric parameters, especially temperature and pressure. Further work and, likely, new experimental methods or instruments will be needed to fully constrain this source of uncertainty in measurements of fine and ultrafine aerosol particles.
UAST_1341039_Supplementary_File.zip
Download Zip (2.7 MB)Funding
This work has been supported by the Magnus Ehrnrooth Foundation, the Jane and Aatos Erkko Foundation, the Emil Aaltonen Foundation, and by NSF grants CBET-1236909 and AGS-1602086.
References
- Brunelli, N. A., Flagan, R. C., and Giapis, K. P. (2009). Radial Differential Mobility Analyzer for One Nanometer Particle Classification. Aerosol Sci. Technol., 43(1):53–59. doi: 10.1080/02786820802464302.
- Clarke, A. D., Varner, J. L., Eisele, F., Mauldin, R. L., Tanner, D., and Litchy, M. (1998). Particle Production in the Remote Marine Atmosphere: Cloud Outflow and Subsidence During ACE 1. J. Geophys. Res., 103:16397–16409.
- Coggon, M. M., Sorooshian, A., Wang, Z., Craven, J. S., Metcalf, A. R., Lin, J. J., Nenes, A., Jonsson, H. H., Flagan, R. C., and Seinfeld, J. H. (2014). Observations of Continental Biogenic Impacts on Marine Aerosol and Clouds Off the Coast of California. J. Geophys. Res., 119:6724–6748. doi: 10.1002/2013JD021228.
- Davies, C. N. (1945). Definitive Equations for the Fluid Resistance of Spheres. P. Phys. Soc., 57:259–270.
- de La Verpilliere, J. L., Swanson, J. J., and Boies, A. M. (2015). Unsteady Bipolar Diffusion Charging in Aerosol Neutralisers: A Non-Dimensional Approach to Predict Charge Distribution Equilibrium Behaviour. J. Aerosol Sci., 86:55–68.
- Ehn, M., Junninen, H., Petäjä, T., Kurtén, T., Kerminen, V.-M., Schobesberger, S., Manninen, H. E., Ortega, I. K., Vehkamäki, H., Kulmala, M., and Worsnop, D. R. (2010). Composition and Temporal Behavior of Ambient Ions in the Boreal Forest. Atmos. Chem. Phys., 10:8513–8530. doi: 10.5194/acp-10-8513-2010.
- Ehn, M., Junninen, H., Schobesberger, S., Manninen, H. E., Franchin, A., Sipilä, M., Petäjä, T., Kerminen, V.-M., Tammet, H., Mirme, A., Mirme, S., Hõrrak, U., Kulmala, M., and Worsnop, D. R. (2011). An Instrumental Comparison of Mobility and Mass Measurements of Atmospheric Small Ions. Aerosol Sci. Technol., 45(4):522–532.
- Filippov, A. V. (1993). Charging of Aerosol in the Transition Regime. J. Aerosol Sci., 42:423–436.
- Flagan, R. C. (1999). On Differential Mobility Analyzer Resolution. Aerosol Sci. Technol., 30(6):556–570.
- Fuchs, N. A. (1963). On the Stationary Charge Distribution on Aerosol Particles in a Bipolar Ionic Environment. Geofis. Pura Appl., 56:185–192.
- Gagné, S., Leppä, J., Petäjä, T., McGrath, M. J., Vana, M., Kerminen, V.-M., Laakso, L., and Kulmala, M. (2012). Aerosol Charging State at an Urban Site: New Analytical Approach and Implications for Ion-Induced Nucleation. Atmos. Chem. Phys., 12:4647–4666. doi: 10.5194/acp-12-4647-2012.
- Gopalakrishnan, R., and Hogan, C. J. (2012). Coulomb-Influenced Collision in Aerosols and Dusty Plasmas. Phys. Rev. E, 85:026410.
- Gopalakrishnan, R., McMurry, P. H., and Hogan, C. J. (2015). Bipolar Charging of Nanoparticles: A Review and Development of Approaches for Non-spherical Particles. Aerosol Sci. Technol., 49:1181–1194.
- Gopalakrishnan, R., Meredith, M. J., Larriba-Andaluz, C., and Hogan, C. J. (2013a). Brownian Dynamics Determination of the Bipolar Steady State Charge Distribution on Spheres and Non-spheres in the Transition Regime. J. Aerosol Sci., 63:126–145.
- Gopalakrishnan, R., Thajudeen, T., Ouyang, H., and Hogan, C. J. (2013b). The Unipolar Diffusion Charging of Arbitrary Shaped Aerosol Particles. J. Aerosol Sci., 64:60–80.
- Hagwood, C., Sivathanu, Y., and Mulholland, G. (1999). The DMA Transfer Function with Brownian Motion, A Trajectory/Monte Carlo Approach. Aerosol Sci. Technol., 30:40–61.
- Han, B., Hudda, N., Ning, Z., Kim, H.-J., Kim, Y.-J., and Sioutas, C. (2009). A Novel Bipolar Charger for Submicron Aerosol Particles using Carbon Fiber Ionizers. J. Aerosol Sci., 40(4):285–294.
- He, M., and Dhaniyala, S. (2014). Experimental Characterization of Flowrate-Dependent Bipolar Diffusion Charging Efficiencies of Sub-50 nm Particles. J. Aerosol Sci., 76:175–187.
- Hoppel, W. A., and Frick, G. M. (1986). Ion-Aerosol Attachment Coefficients and the Steady-State Charge Distribution on Aerosols in a Bipolar Ion Environment. Aerosol Sci. Technol., 5:1–21.
- Hoppel, W. A., and Frick, G. M. (1990). The Nonequilibrium Character of the Aerosol Charge Distributions Produced by Neutralizes. Aerosol Sci. Technol., 12:471–496.
- Hussin, A., Scheibel, H. G., Becker, K. H., and Porstendörfer, J. (1983). Bipolar Diffusion Charging of Aerosol Particles–1: Experimental Results within the Diameter Range 4–30 nm. J. Aerosol Sci., 14(5):671–677.
- Hyvärinen, A.-P., Raatikainen, T., Komppula, M., Mielonen, T., Sundström, A.-M., Brus, D., Panwar, T. S., Hooda, R. K., Sharma, V. P., de Leeuw, G., and Lihavainen, H. (2011). Effect of the Summer Monsoon on Aerosols at two Measurement Stations in Northern India – Part 2: Physical and Optical Properties. Atmos. Chem. Phys., 11:8283–8294. doi: 10.5194/acp-11-8283-2011.
- Iida, K., Stolzenburg, M. R., and McMurry, P. H. (2009). Effect of Working Fluid on Sub-2 nm Particle Detection with a Laminar Flow Ultrafine Condensation Particle Counter. Aerosol Sci. Technol., 43:81–96. doi: 10.1080/02786820802488194.
- Jiang, J., Hogan, C. J., Chen, D.-R., and Biswas, P. (2007). Aerosol Charging and Capture in the Nanoparticle Size Range (6–15 nm) by Direct Photoionization and Diffusion Mechanisms. J. Appl. Phys., 102:034904.
- Jiang, J., Kim, C., Wang, X., Stolzenburg, M. R., Kaufman, S. L., Qi, C., Sem, G. J., Sakurai, H., Hama, N., and McMurry, P. H. (2014). Aerosol Charge Fractions Downstream of Six Bipolar Chargers: Effects of Ion Source, Source Activity, and Flowrate. Aerosol Sci. Technol., 48(12):1207–1216. doi: 10.1080/02786826.2014.976333.
- Kallinger, P., and Szymanski, W. (2015). Experimental Determination of the Steady-State Charging Probabilities and Particle Size Conservation in Non-radioactive and Radioactive Bipolar Aerosol Chargers in the Size Range of 5–40 nm. J. Nanopart. Res., 17:171. doi: 10.1007/s11051-015-2981-x.
- Knutson, E. O., and Whitby, K. T. (1975). Aerosol Classification by Electric Mobility: Apparatus, Theory and Applications. J. Aerosol Sci., 6:443–451.
- Ku, B. K., and de la Mora, J. F. (2009). Relation between Electrical Mobility, Mass, and Size for Nanodrops 1–6.5 nm in Diameter in Air. Aerosol Sci. Technol., 43(3):241–249. doi: 10.1080/02786820802590510.
- Kulmala, M., and Kerminen, V.-M. (2008), On the Formation and Growth of Atmospheric Nanoparticles. Atmos. Res., 90:132–150.
- Kulmala, M., Vehkamäki, H., Petäjä, T., Dal Maso, M., Lauri, A., Kerminen, V.-M., Birmili, W., and McMurry, P. H. (2004). Formation and Growth Rates of Ultrafine Atmospheric Particles: A Review of Observations. J. Aerosol Sci., 35:143–176.
- Kwon, S. B., Sakurai, H., Seto, T., and Kim, Y. J. (2006). Charge Neutralization of Submicron Aerosols using Surface-Discharge Microplasma. J. Aerosol Sci., 37:483–499.
- Larriba, C., and Hogan, C. J. (2013). Ion Mobilities in Diatomic Gases: Measurement vs. Prediction with Non-Specular Scattering Models. J. Phys. Chem. A 117:3887–3901.
- Larriba-Andaluz, C., Fernández-García, J., Ewing, M. A., Hogan, C. J., and Clemmer, D. E. (2015). Gas Molecule Scattering & Ion Mobility Measurements for Organic Macro-Ions in He Versus N2 Environments. Phys. Chem. Chem. Phys., 17:15019–15029.
- Lee, H. M., Kim, C. S., Shimada, M., and Okuyama, K. (2005). Effects of Mobility Changes and Distribution of Bipolar Ions on Aerosol Nanoparticle Diffusion Charging. J. Chem. Eng. Jpn., 38:486–496.
- Leppä, J., Kerminen, V.-M., Laakso, L., Korhonen, H., Lehtinen, K. E. J., Gagné, S., Manninen, H. E., Nieminen, T., and Kulmala, M. (2009). Ion-UHMA: A Model for Simulating the Dynamics of Neutral and Charged Aerosol Particles. Boreal Env. Res., 14:559–575.
- López-Yglesias, X., and Flagan, R. (2013a). Ion-Aerosol Flux Coefficients and the Steady State Charge Distribution of Aerosols in a Bipolar Ion Environment. Aerosol Sci. Technol., 47(6):688–704.
- López-Yglesias, X., and Flagan, R. (2013b). Population Balances of Micron-sized Aerosol in a Bipolar Ion Environment. Aerosol Sci. Technol., 47(6):681–687.
- Maißer, A., Thomas, J. M., Larriba-Andaluz, C., He, S., and Hogan, C. J. (2015). The Mass-Mobility Distributions of Ions Produced by a Po-210 Source in Air. J. Aerosol Sci., 90:36–50.
- Mäkelä, J. M., Aalto, P., Jokinen, V., Pohja, T., Nissinen, A., Palmroth, S., Markkanen, T., Seitsonen, K., Lihavainen, H., and Kulmala, M. (1997). Observations of Ultrafine Aerosol Particle Formation and Growth in Boreal Forest. Geophys. Res. Lett., 24(10):1219–1222. doi: 10.1029/97GL00920.
- Merritt, M., and Zhang, Y. (2005). Interior-Point Gradient Method for Large-Scale Totally Nonnegative Least Squares Problems. J. Optimiz. Theory App., 126(1):191–202. doi: 10.1007/s10957-005-2668-z.
- Mirme, S., Mirme, A., Minikin, A., Petzold, A., Hõrrak, U., Kerminen, V.-M., and Kulmala, M. (2010). Atmospheric Sub-3 nm Particles at High Altitudes. Atmos. Chem. Phys., 10:437–451. doi: 10.5194/acp-10-437-2010.
- Mönkkönen, P., Koponen, I. K., Lehtinen, K. E. J., Hämeri, K., Uma, R., and Kulmala, M. (2005). Measurements in a Highly Polluted Asian Mega City: Observations of Aerosol Number Size Distribution, Modal Parameters and Nucleation Events. Atmos. Chem. Phys., 5:57–66. doi: 10.5194/acp-5-57-2005.
- Mui, W., Thomas, D. A., Downard, A. J., Beauchamp, J. L., Seinfeld, J. H., and Flagan, R. C. (2013). Ion Mobility-Mass Spectrometry with a Radial Opposed Migration Ion and Aerosol Classifier (ROMIAC). Anal. Chem., 85(13):6319–6326. doi: 10.1021/ac400580u.
- Mui, W., Mai, H., Downard, A. J., Seinfeld, J. H., and Flagan, R. C. (2017). Design, Simulation, and Characterization of a Radial Opposed Migration Ion and Aerosol Classifier (ROMIAC). Aerosol Sci. Technol., 51(7):801–823. doi: 10.1080/02786826.2017.1315046.
- Neitola, K., Asmi, E., Komppula, M., Hyvärinen, A.-P., Raatikainen, T., Panwar, T. S., Sharma, V. P., and Lihavainen, H. (2011). New Particle Formation Infrequently Observed in Himalayan Foothills – Why? Atmos. Chem. Phys., 11:8447–8458. doi: 10.5194/acp-11-8447-2011.
- Ouyang, H., Gopalakrishnan, R., and Hogan, C. J. (2012). Nanoparticle Collisions in the Gas Phase in the Presence of Singular Contact Potentials. J. Chem. Phys., 137(6):064316.
- Ouyang, H., Larriba-Andaluz, C., Oberreit, D. R., and Hogan, C. J. (2013). The Collision Cross Sections of Iodide Salt Cluster Ions in Air via Differential Mobility Analysis-Mass Spectrometry. J. Am. Soc. Mass Spectrom., 24:1833–1847. doi: 10.1007/s13361-013-0724-8.
- Qi, C., and Kulkarni, P. (2013). Miniature Dual-Corona Ionizer for Bipolar Charging of Aerosol. Aerosol Sci. Technol., 47(1):81–92. doi: 10.1080/02786826.2012.728301.
- Rosser, S., and de la Mora, J. F. (2005). Vienna-Type DMA of High Resolution and High Flow Rate. Aerosol Sci. Technol., 39(12):1191–1200. doi: 10.1080/02786820500444820.
- Shimada, M., Han, B., Okuyama, K., and Otani, Y. (2002). Bipolar Charging of Aerosol Nanoparticles by a Soft X-ray Photoionizer. J. Chem. Eng. Jpn., 35(8):786–793.
- Steiner, G., Jokinen, T., Junninen, H., Sipilä, M., Petäjä, T., Worsnop, D. R., Reischl, G. P., and Kulmala, M. (2014). High-Resolution Mobility and Mass Spectrometry of Negative Ions Produced in a 241Am Aerosol Charger. Aerosol Sci. Technol., 48(3):261–270. doi: 10.1080/02786826.2013.870327.
- Steiner, G., and Reischl, G. P. (2012). The Effect of Carrier Gas Contaminants on the Charging Probability of Aerosols under Bipolar Charging Conditions. J. Aerosol Sci., 54:21–31.
- Stolzenburg, M. R. (1988). An Ultrafine Aerosol Size Distribution Measuring System. University of Minnesota, Minneapolis.
- Tammet, H. (1995). Size and Mobility of Nanometer Particles, Clusters, and Ions. J. Aerosol Sci., 26:459–475.
- Tigges, L., Jain, A., and Schmid, H.-J. (2015). On the Bipolar Charge Distribution used for Mobility Particle Sizing: Theoretical Considerations. J. Aerosol Sci., 88:119–134.
- Weingartner, E., Nyeki, S., and Baltensperger, U. (1999). Seasonal and Diurnal Variation of Aerosol Size Distributions (10 < D < 750 nm) at a High-Alpine Site (Jungfraujoch 3580 m asl). J. Geophys. Res., 104(D21):26809–26820.
- Wiedensohler, A. (1988). An Approximation of the Bipolar Charge Distribution for Particles in the Submicron Size Range. J. Aerosol Sci., 19:387–389.
- Wiedensohler, A., Birmili, W., Nowak, A., Sonntag, A., Weinhold, K., Merkel, M., Wehner, B., Tuch, T., Pfeifer, S., Fiebig, M., Fjäraa, A. M., Asmi, E., Sellegri, K., Depuy, R., Venzac, H., Villani, P., Laj, P., Aalto, P., Ogren, J. A., Swietlicki, E., Williams, P., Roldin, P., Quincey, P., Hüglin, C., Fierz-Schmidhauser, R., Gysel, M., Weingartner, E., Riccobono, F., Santos, S., Grüning, C., Faloon, K., Beddows, D., Harrison, R., Monahan, C., Jennings, S. G., O'Dowd, C. D., Marinoni, A., Horn, H.-G., Keck, L., Jiang, J., Scheckman, J., McMurry, P. H., Deng, Z., Zhao, C. S., Moerman, M., Henzing, B., de Leeuw, G., Löschau, G., and Bastian, S. (2012). Mobility Particle Size Spectrometers: Harmonization of Technical Standards and Data Structure to Facilitate High Quality Long-Term Observations of Atmospheric Particle Number Size Distributions. Atmos. Meas. Tech., 5:657–685. doi: 10.5194/amt-5-657-2012.
- Yun, K. M., Lee, S. Y., Iskandar, F., Okuyama, K., and Tajima, N. (2009). Effect of X-Ray Energy and Ionization Time on the Charging Performance and Nanoparticle Formation of a Soft X-Ray Photoionization Charger. Adv. Powder Technol., 20(6):529–536.
- Zhang, S.-H., and Flagan, R. C. (1996). Resolution of the Radial Differential Mobility Analyzer for Ultrafine Particles. J. Aerosol Sci., 27:1179–1200.