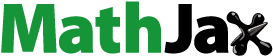
ABSTRACT
Human resource management was of great significance for enterprises to formulate development strategies and improve competitiveness. With the rapid development of new technologies such as knowledge economy and big data, the traditional human resource performance appraisal model had certain limitations and was not objective, which had been difficult to adapt to the increasingly highly integrated development needs of modern enterprises. Therefore, this paper used big data analysis and new technical means to put forward the study of human resource performance evaluation model based on Bayesian network, aiming to provide some theoretical reference for modern enterprise human resource management. First of all, it summarized the related concepts of performance appraisal and its important position in enterprise management, analyzed the characteristics of human resources performance appraisal indicators and their mutual relations, and expounded the common methods and characteristics needed to build a performance appraisal system. Secondly, it summarized the relevant theory of Bayesian network and its advantages in solving complex random problems, and proposed a performance appraisal model based on Bayesian network for the needs of enterprise human resources management. Finally, the balanced scorecard method was used to build a performance appraisal system, and the performance appraisal model proposed in this paper was tested by experiments, and compared with the traditional method. The results showed that the performance appraisal model proposed in this paper had significant advantages and could be better applied to the performance appraisal management of enterprise human resources. The research of this paper can not only provide theoretical guidance for further research on enterprise human resource allocation and management, but also provide reference for performance appraisal in other fields.
Introduction
With the continual expansion of the economy, science, and technology, the survival and development of businesses rely heavily on human resources. Human resources have steadily become the most competitive and creative resource among all resources, thanks to the fast expansion of the knowledge economy. It may not only provide competitive benefits to businesses, but it can also influence their growth plan. Human resources, unlike other common material resources, are alive and have distinct features (Shen Citation2020). As a result, organizations often place a premium on human resource management. Human resource management is analogous to production, operation, and finance management in company management. The evaluation of human resource performance significantly influences the production and operation of businesses. It can not only help businesses understand their workers’ working conditions and contributions in real-time, but it can also help businesses allocate human resources scientifically, establish development plans, and increase their competitiveness. Human resource performance assessment management is primarily concerned with evaluating workers’ work tasks and performance regularly by the enterprise’s growth goals to comprehend the fulfillment of employees’ work objectives in a timely way (Luo, Xing, and Ren Citation2022 L. L. Zhou, Mao, and Li Citation2020). The performance assessment may effectively adapt the organization’s production and operation management plan, fully use the work potential and enthusiasm of the personnel, and provide the enterprise with the power to reach the ultimate objective. With the advent of the big data era, contemporary organizations’ human resource performance evaluation style has increasingly shifted from conventional static management to dynamic and systematic management.
Traditional enterprises generally manage the performance distribution of employees according to the division of department functions and responsibilities (L. T. Zhou, Wu, and Chen Citation2022). Given businesses’ various natures and growth aims, establishing a focused human resource performance assessment system has become critical for contemporary businesses to devise development plans and increase management levels and competitiveness. Although it can reflect the primary responsibility of employees in the department, it does not consider the overall interests of employees in the enterprise, making it difficult for employee performance appraisal results to provide a scientific basis for enterprise adjustment and development strategy. And Since the old performance assessment model prioritizes departmental interests and lacks integrity, it isn’t easy to fulfill the objectives of contemporary company management and growth (Wang, Yuan, and Elhoseny Citation2019). The goal of performance assessment is to enhance the overall advantages of the organization and to reflect the personal performance of workers. Only by using current and scientific techniques of human resource performance evaluation can the relevance of performance appraisal findings be recognized and organizations’ ongoing growth supported. As a result, this article offers the research of a human resource performance assessment model based on a Bayesian network employing contemporary big data analysis and technology methods to provide a theoretical reference for modern corporate human resource management.
Related Works
In the 1970s, some scholars put forward the concept of human resources and performance management. With the continuous development of economy and society, human resource management has played an important role in enterprises, and performance evaluation has become an important part of human resource management (Goes and Oliveira Citation2020). In terms of performance appraisal and management, some early scholars have made more achievements through practice and formed a series of related theories. For example, some people have put forward incentive theories including cognitive evaluation method, objective management method and fair management method. In recent years, some scholars have improved the performance management methods on the basis of the existing incentive theory, and put forward some new methods to build the performance appraisal system. For example, the balanced scorecard method, the full perspective evaluation method and the key indicator evaluation method (Lidinska and Jablonsky Citation2018; Zhao et al. Citation2021). Among them, the balanced scorecard method mainly divides the performance evaluation into different dimensions according to the main observation points affecting performance, and then subdivides into several different evaluation indicators under each dimension. The key index evaluation method is mainly to explore the main factors that affect the performance of employees, take them as the standard of performance evaluation, and evaluate employees.
As an important part of enterprise management, human resources management mainly uses modern scientific means to effectively allocate and organize personnel, give full play to the initiative and enthusiasm of employees, and provide guarantee for the realization of enterprise development goals. The task of human resources management is to coordinate the relationship between employees, organize employees to complete their jobs in an orderly manner, combine the development goals of the enterprise with personal interests, fully mobilize the working ability of employees, and provide strong backing for the realization of the enterprise goals. With the development of modern science and technology and information technology, the personnel management involved in enterprises is becoming more and more complex. The traditional human resource management mode based on manual mode can not meet the needs of modern personnel management. In order to improve the level of scientific decision-making of enterprises, some people began to use big data technology and information technology to carry out human resources management (Hu and Zhao Citation2020; Zhao, Ding, and Wang Citation2020). For example, by developing various human resource management information systems and effectively applying them to enterprise personnel management, it can not only improve the management efficiency with the full help of computer technology, but also accurately analyze the data in human resource management, thus providing a basis for formulating a scientific human resource performance evaluation system.
Employees are not only the producers and managers of enterprises, but also the fundamental force that determines the survival and development of enterprises. Therefore, human resource performance management is of great significance to the development of employees and enterprises. The research shows that the potential of employees can be tapped through performance evaluation, and classified management can be carried out according to their own strengths. The results of performance appraisal can not only promote employees to do their jobs well, but also help to make full use of human resources. Therefore, some scholars have carried out a lot of research on the construction of performance appraisal system. With the rapid development of big data and computer technology, enterprises are gradually changing to informatization in production management. For example, enterprise resource planning (ERP) has been widely used in many enterprises and played an irreplaceable role (Sohail et al. Citation2021). However, some domestic enterprises are still limited to the traditional mode in human resource management, and less modern scientific and technological means are applied to employee performance appraisal. At present, the production management of most enterprises has been transformed to a high degree of informatization. Therefore, as an important part of human resource management, performance appraisal needs to be integrated into the informatization integrated management platform as soon as possible. Because the traditional performance appraisal model lacks certain objectivity and scientificity, it is not only an urgent problem for enterprises to solve under the new situation to build an effective performance appraisal system by using new technologies or methods, but also provides powerful guidance for enterprises to formulate development strategies.
Human resource management (Zhao et al. Citation2021) plays a crucial role in helping enterprises formulate development strategies and improve their competitiveness. However, with the rapid development of new technologies such as the knowledge economy and big data, traditional human resource performance appraisal models have limitations and may not be objective enough to meet the highly integrated development needs of modern enterprises. Therefore, this paper proposes a new approach to human resource performance evaluation based on Bayesian network analysis and new technical means. The goal is to provide theoretical guidance for modern enterprise human resource management.
The paper first provides an overview of performance appraisal and its significance in enterprise management. It then analyzes the characteristics of human resources performance appraisal indicators and their interrelationships, as well as the common methods and characteristics needed to build a performance appraisal system. Next, the paper explores the relevant theory of Bayesian network and its advantages in solving complex random problems. Based on this theory, the paper proposes a performance appraisal model for the needs of enterprise human resources management.
To test the effectiveness of the proposed model, the paper uses the balanced scorecard method to build a performance appraisal system and conducts experiments to compare the proposed model with traditional methods. The results demonstrate that the proposed model has significant advantages in terms of objectivity and adaptability, and can be better applied to the performance appraisal management of enterprise human resources.
Overall, the research in this paper highlights the importance of using new technologies such as big data analysis and Bayesian network to improve the objectivity and adaptability of traditional performance appraisal models. The paper also contributes to the advancement of human resource management in modern enterprises by providing theoretical guidance for further research on enterprise human resource allocation and management.
Performance Appraisal and Related Theoretical Basis
Performance Appraisal Concept
In enterprise management, performance evaluation can provide a reliable basis for human resources management and decision-making for the human resources department of the enterprise, and performance evaluation system is an important mechanism for personnel management and benefit tracking control of the enterprise. A perfect performance management system can ensure the normal operation and management activities of the enterprise, otherwise it cannot guarantee the realization of the production management tasks and objectives of the enterprise (Li and Pahlevanzadeh Citation2022). As shown in , it reflects the role of performance appraisal in enterprise human resource management and the relationship between performance appraisal and other functions.
The so-called performance refers to the completion of a predetermined task or the achievement of an expected goal, and the production of a certain function or efficiency. Performance is the labor or behavior that people pay to complete the output. For example, enterprise performance is the performance and value of employees. Enterprise human resource performance appraisal is based on individual performance management, and performance is the performance of individual behavior and results. Since behavior is the actual work that individuals put into to complete tasks, and is the result of intellectual and physical efforts, it is necessary to consider both input and output in the performance evaluation of individuals (Goes and Oliveira Citation2020).
Performance appraisal is also called performance appraisal. It mainly adopts certain appraisal standards and methods to comprehensively evaluate the employees of the enterprise, so as to understand the status of employees, and formulate corresponding rectification measures according to the appraisal results, so as to effectively promote the development of the enterprise and the realization of its objectives. Performance appraisal not only reflects the work status and evaluation results of enterprise personnel, but also can timely feed back the work performance of employees to employees and their departments. Through performance appraisal, we will constantly promote the work enthusiasm of enterprise employees and their relevance to enterprise organizations. In the process of performance appraisal, it is necessary to follow the basic principles of paying attention to personal performance, scientific and objective principles, timeliness and continuity to ensure the fairness, impartiality and seriousness of the evaluation results (Nasar et al. Citation2021).
Performance Appraisal Indicators
In order to scientifically and objectively evaluate the performance of enterprise employees, it is necessary to build a complete and operable performance evaluation system. As the performance appraisal is closely related to the development objectives of enterprises, when building the performance appraisal system, we should not only start from the perspective of enterprise development, but also combine the characteristics of enterprises. Otherwise, the established assessment system can not truly realize the objective evaluation of the employees of the enterprise, thus affecting the enthusiasm of the employees and the efficiency of the enterprise. In addition, the construction of the performance appraisal system also needs to follow some basic principles, such as the principle of objective, measurable, integrity, consistency and cost-effectiveness (Alsaleh et al. Citation2020).
In the performance appraisal of enterprise human resources, although the actual situations such as the departments, positions and work nature of different employees may be different, and the appraisal indicators are different, the construction principles of the performance appraisal system and the design of relevant indicators are basically similar (Chiang and Lin Citation2020). As an important part of the performance appraisal system, the appraisal index is the main content and standard for evaluating the performance of employees, and is an important reference for measuring the performance appraisal system. Since the evaluation index is the wind vane to guide the employees’ work attitude and behavior, the index setting has important strategic significance for the enterprise performance appraisal system. Performance appraisal is mainly divided into qualitative and quantitative parts. The corresponding evaluation indicators also include qualitative indicators and quantitative indicators. Among them, qualitative indicators are mainly used to assess the work process of employees, while quantitative indicators are mainly used to assess the work performance of employees. It can be seen that when building the enterprise performance appraisal system, the design of relevant evaluation indicators should not only select the corresponding qualitative and quantitative indicators according to the development and actual situation of the enterprise, but also follow the requirements of objectivity and applicability (Luo, Xing, and Ren Citation2022).
In the constructed performance appraisal system, in addition to each evaluation index, it is also necessary to determine the corresponding index weight. For this reason, common weight determination methods such as expert scoring method, analytic hierarchy process and subjective evaluation method can be adopted. In order to give the weight value of each evaluation index scientifically and objectively, the analytic hierarchy process (AHP) with less workload and more scientific can be adopted (Nasar et al. Citation2021). This method first needs to get the relative weights of each indicator according to expert evaluation, then use statistical methods to analyze the evaluation results and establish the weight matrix of each indicator. Finally, the weight matrix is analyzed to determine the weight value of each indicator. The analytic hierarchy process not only makes use of the expert evaluation results, but also through scientific calculation and analysis, so the results obtained by this method are relatively objective (Atienza, Bielza, and Larranaga Citation2022). The relative weight matrix of each index obtained by the analytic hierarchy process is as follows:
Each relative weight value in the judgment matrix is usually the judgment result given by experts according to specific evaluation indicators. For each index, the element in the above judgment matrix represents the relative weight value of the index
with respect to
. Generally, the relative weight value of each indicator can be divided into several levels, and each level represents the relative importance of different indicators.
Since the weight value of
relative to
is exactly the reciprocal of
, there is the following expression for any judgment matrix:
Since the elements in the judgment matrix are only the relative weight values of each indicator, in order to obtain the weight values of each indicator in the performance appraisal system, it is necessary to further perform relevant processing on the judgment matrix.
First, the judgment matrix is processed by the normalization method. The specific calculation formula is as follows:
Next, each element in the normalized judgment matrix is added by row, as follows:
Finally, the relative weight of each indicator can be calculated according to the above results. The specific calculation formula is as follows:
Common Methods of Performance Appraisal
In order to ensure that the enterprise performance evaluation can achieve the expected objectives, it is a prerequisite to adopt appropriate methods to evaluate the performance. In recent years, the methods of performance appraisal at home and abroad mainly include objective management method, key performance indicator (KPI) method, balanced scorecard and full perspective assessment method (Atienza, Larranaga, and Bielza Citation2022). Although various enterprises adopt different methods in performance appraisal, the purpose is to use the results of performance appraisal to stimulate the enthusiasm of employees and achieve more benefits for the enterprise.
Management by objectives is a performance appraisal method used by relevant managers and employees to formulate work objectives according to the enterprise development objectives, and then regularly check the completion of objectives. Setting goals can not only clarify the work direction of employees, but also compare the actual work results with the goals, so as to adjust the implementation of various work tasks in time. During the implementation of the management by objectives method, it is necessary to subdivide the overall objectives of enterprise development and set specific objectives for managers at different levels and front-line employees. Then, carry out regular evaluation on the preset task objectives of personnel at all levels to check the work completion status of relevant departments and personnel, and take this as the basis for rewarding and punishing employees (Lidinska and Jablonsky Citation2018). Due to the adjustment of enterprise development objectives, the objectives of managers and employees at different levels should also be dynamically adjusted when adopting the objective management method for performance appraisal. Therefore, this method can be better applied in most enterprises. Considering the timeliness and integrity of the enterprise’s development objectives, there may be continuous adjustment of the objectives when applying the objective management method to the performance appraisal, thus affecting the stability and sustainability of the performance appraisal of the entire enterprise.
Different from the traditional performance appraisal method based on financial indicators, the balanced scorecard mainly evaluates the performance of enterprise employees from four aspects: enterprise finance, customer market, internal management and technological innovation (Beliveau and Gustafson Citation2021). The specific appraisal structure is shown in . The balanced scorecard not only takes into account the short-term and long-term objectives of enterprise development, but also can achieve the balance between various indicators.
During the implementation of the balanced scorecard, it is necessary to determine the performance appraisal objectives of employees from four different dimensions according to the enterprise development plan. When setting various appraisal indicators, we should not only consider the relationship between different indicators, but also establish the relationship between employee performance and appraisal results. At the same time, the unreasonable assessment indicators shall be dynamically adjusted according to the regular assessment results. The balanced scorecard can not only avoid the disadvantages brought by the traditional assessment methods, but also encourage the employees to closely combine personal performance and enterprise interests. Because the balanced scorecard needs to consider many factors such as enterprise development strategy, finance and personnel when it is implemented, it is difficult to apply it to performance appraisal.
The key performance indicator assessment method is mainly based on the analysis of human resource performance characteristics, mining important indicators that affect performance, and thus building a performance assessment model. This method takes the key indicators as the evaluation standard, and establishes the causal relationship between employee performance and key indicators. On the basis of determining the enterprise development goals, the key indicators of each department are established through goal decomposition, and then the department indicators are decomposed one by one into the key indicators required for employee assessment. During the performance appraisal of employees, it is necessary to determine the weight and scoring requirements of each indicator, and then compare the task completion of employees with the requirements of key indicators, and finally get the performance appraisal results of each employee. The performance appraisal system constructed by this method needs to be based on the set key indicators, and the definition of key indicators is based on whether it can have a significant impact on the completion of enterprise objectives (Wang, Yuan, and Elhoseny Citation2019). Therefore, this method has certain limitations in application.
The full perspective assessment method mainly assesses the performance of employees from the perspective of different subjects, and obtains the assessment results of employees through comprehensive evaluation (Scanagatta et al. Citation2018). As shown in , it is a schematic diagram of the working process of the full perspective assessment method. In the comprehensive perspective assessment method, first of all, the assessment team must be clear. Its members must understand the assessed objects and set different scoring weights for the members. Secondly, each team member objectively evaluates the employees according to the assessment requirements. Finally, according to the evaluation results given by all the assessment members, the employees are comprehensively assessed. This method can avoid the unfairness of traditional assessment methods, and at the same time, the examinees can comprehensively check their work completion according to the evaluation results of each team member. In addition, because the method requires more personnel to participate in the performance appraisal, the workload is large in the specific implementation of the appraisal and there are large subjective factors in the appraisal results, it is difficult to promote the method in practical application.
The proposed method for human resource performance evaluation based on Bayesian network analysis and the balanced scorecard method can be extended to cover a wider scientific area by applying it to other fields of study. For example, the method could be used to evaluate the performance of employees in other areas of business, such as marketing, finance, or operations. The key advantage of the proposed method is its ability to identify biases in the performance appraisal process and develop more effective strategies for managing human resources. This same approach could be applied to other areas of study to identify biases and develop more effective strategies for managing resources and improving performance. However, it is important to note that while the proposed method can be extended to other fields, it may require some adaptation to fit the specific needs and characteristics of each field. This could involve modifying the performance appraisal criteria, adjusting the Bayesian network analysis, or using different techniques altogether. Overall, we believe that the proposed method has the potential to be applied to a wide range of scientific areas beyond human resource management. By incorporating advanced statistical analysis techniques and new technologies, we can develop a more nuanced understanding of performance appraisal and how it can be improved to meet the needs of modern organizations. While adaptation may be required to fit the needs of different fields, we encourage researchers to explore the potential applications of this method in their own areas of study.
Human Resource Performance Appraisal Model Based on Bayesian Network
In this paper, we propose a new approach to human resource performance evaluation based on advanced statistical analysis techniques and new technologies. The proposed method is based on the use of Bayesian network analysis and the balanced scorecard method to build a performance appraisal system. The key contribution of this paper is to demonstrate how advanced statistical analysis techniques can be used to improve the objectivity and adaptability of traditional performance appraisal models in the context of human resource management. We show how the proposed method can identify biases in the appraisal process and provide insights into how to adjust the process to make it more objective and effective. By using advanced statistical analysis techniques, we can develop a deeper understanding of the performance appraisal process and how it can be improved. We can identify patterns in the data set that may not be apparent using traditional methods, and we can develop more effective strategies for managing human resources in modern enterprises. Overall, the research in this paper represents an important step forward in the field of human resource management. By incorporating advanced statistical analysis techniques and new technologies, we can develop a more nuanced understanding of the performance appraisal process and how it can be improved to meet the needs of modern enterprises. We hope that this paper will serve as a valuable resource for researchers and practitioners in the field of human resource management.
Overview of Bayesian Networks (BN)
As a common method of applying probability and statistics theory to the analysis of complex problems, Bayesian network is mainly a tool for analyzing complex data and reasoning uncertain factors (Canonne et al. Citation2020). In recent years, it has been widely used in the field of artificial intelligence. Bayesian networks are mainly divided into static and dynamic types. Static Bayesian network mainly uses directed acyclic graph to describe the causal relationship between various random variables. It is mainly composed of network structure and parameters.
From the perspective of network structure, in the directed acyclic graph of Bayesian network, nodes are used to represent each random variable in the Bayesian model, and the directed edges represent the causal relationship between each variable. The network parameters are mainly described by the conditional probability table, and each variable in the Bayesian network has a probability distribution. It may be assumed that the parent node of a node is represented by
, and the root node conforms to the edge distribution, but the relationship between the root node and its parent node conforms to the conditional probability distribution
.
The directed acyclic graph used in Bayesian network can not only describe the interdependence between different random variables, but also reflect the joint probability distribution law between different variables. If the random variables in the Bayesian network are , the joint probability distribution between these variables can be calculated as follows [16]:
The dynamic Bayesian network (DBN) mainly relates the static Bayesian network with time, that is, it extends the static Bayesian network in time. Dynamic Bayesian network is a random network model which can process real-time data based on probability distribution. By adding time elements to the static Bayesian network, not only the random variable data in the Bayesian network can be updated in real time, but also the network structure and parameters are constantly changing. Therefore, dynamic Bayesian network can be better used to deal with real-time data and hierarchical knowledge expression.
If a dynamic Bayesian network is represented by , where
represents a basic static Bayesian network, assuming that its initial conditional probability distribution is
,
shows a Bayesian network including adjacent time periods, and given the conditional distribution of each random variable in the adjacent time periods, the calculation formula is as follows (Gao, Yang, and Guo Citation2019):
Where represents the node
at a certain time
, and the parent node of
is represented by
. The parameters of all nodes in the first time period of the dynamic Bayesian network can be omitted, and the conditional probability distribution of each node in the second time period is
. Node
and its parent node can be in the same time period or in the previous time period of node
. Generally, the directed edges in dynamic Bayesian networks change with time, in which the directed edges in the same time period mainly play a transient role, while the directed edges in the adjacent time periods mainly play a time-varying role (Canonne et al. Citation2020; Scanagatta et al. Citation2018).
As shown in , it reflects the formation process of the dynamic Bayesian network model.
Bayesian network can be used to deal with and analyze uncertain and complex problems. For the problem of random events with probabilistic characteristics, we can use the graph theory and probability theory in Bayesian networks to explore the causal relationship between random events. For example, the structure diagram in Bayesian networks can intuitively reflect the interaction relationship between random variables. For the random events with uncertain characteristics, Bayesian network can not only make full use of the existing prior knowledge and massive data to effectively avoid the influence of various subjective factors on the network construction process, but also can use expert knowledge and combine the existing real data to construct the network. In addition, for the processing of incomplete data sets, Bayesian networks can solve the problems of incomplete data sets by means of structure learning and parameter learning.
As an effective reasoning tool of expert system for uncertain random events, Bayesian network can explore the internal laws hidden in massive data, and has been well applied in artificial intelligence and data mining (Gao, Yang, and Guo Citation2019; Scutari Citation2020). In the process of building the relationship network of random events, we need to analyze and process the data through Bayesian network learning method. Among them, structural learning is mainly to build the structural relationship of the network model and determine the conditional probability parameters of the network model. Parameter learning is to explore the distribution law of conditional probability parameters on the basis of the existing network structure.
In constructing the structure of Bayesian network, it can be constructed by expert knowledge or on the basis of data analysis and processing. Because the network structure constructed according to expert knowledge is greatly affected by human subjective factors and may lead to inaccurate structure, the Bayesian network structure is usually constructed by referring to existing expert knowledge and adopting data analysis and processing methods to ensure the effectiveness of the network structure. Through the structure learning of Bayesian network, data can be effectively analyzed, and the network structure between random variables can be established, so as to explore the qualitative and quantitative relationships between various variables. Generally, the structure learning of Bayesian networks can adopt the learning method based on data analysis or the learning method based on score search.
Construction of Human Resources Performance Appraisal Model
Using the above dynamic Bayesian network, we can build a performance evaluation model of enterprise employees, and use this model to realize the dynamic evaluation and management of enterprise employees’ performance. According to the relevant theories of performance appraisal, in combination with the development objectives of the enterprise and the nature of employees’ work, it is necessary to select certain appraisal indicators before building the appraisal system, and then use the dynamic Bayesian network theory to build the appraisal model. Due to the large number of employees and the complex nature of work, in order to objectively determine the work performance of employees, Bayesian network theory can be used in combination with actual relevant data to assess the performance of employees at different levels, and to scientifically evaluate and predict the performance of employees.
Generally, the performance appraisal of enterprise human resources is mainly carried out from the aspects of employee performance, responsibility, ability, customer feedback, etc. Therefore, the common balanced scorecard method can be used to establish the employee’s performance appraisal model, and the performance appraisal content can be divided into four different dimensions: finance, customer, attendance and quality. At the same time, each dimension is further subdivided into several specific appraisal indicators.
As shown in , it is the schematic diagram of the personnel performance appraisal model constructed by the balanced scorecard method.
Figure 5. Schematic diagram of the personnel performance appraisal model constructed by the balanced scorecard method.
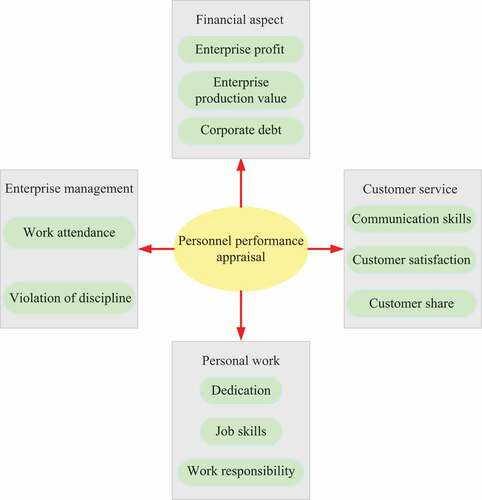
According to the personnel performance appraisal method, the output results of the whole model can be calculated as follows:
Where represents the output value of the personnel performance appraisal model,
shows the number of dimensions of the model,
indicates the
-th dimension, and
is the weight of the
-th dimension.
denotes the
-th indicator under the
-th dimension.
is the
-th indicator weight under the
-th dimension.
denotes a processing function for normalizing the index value of
. Formula (10) indicates that the weight sum of each dimension is 1, while formula (11) denotes that the weight sum of all indicators under each dimension is 1.
The performance appraisal model mainly describes various influencing factors of employee performance, and these factors are independent of each other. According to the Bayesian network construction method, we can further construct the causal relationship diagram between various factors affecting employee performance. As shown in , the causal relationship between different influencing factors is described.
Considering that there may be some repeated causal relationships among the influencing factors of employee performance, the causal relationship diagram between the above factors can be optimized (Cengiz, Dunder, and Senel Citation2018). The specific optimization process is as follows: if there are two different paths between nodes in the network relationship diagram, the indirect path between the two nodes is retained and the direct path is deleted. As shown in , the process of optimizing the factor relationship diagram between each node is described.
Therefore, we can reprocess the employee performance influencing factor relationship graph according to the optimization method of the above node relationship graph to obtain the employee performance evaluation model based on Bayesian network, as shown in .
Experiment and Analysis
Experimental Design and Data Processing Method
In order to test the effectiveness of the human resource performance appraisal model based on Bayesian network, this paper takes the employee performance appraisal of an enterprise as an example and constructs the employee performance appraisal system by using the balanced scorecard method. According to the set performance evaluation system, the employee performance is evaluated by the comprehensive perspective evaluation method. The evaluation process mainly includes the mutual evaluation between the employees of the enterprise, the evaluation of the competent leaders on the employees, and the evaluation of the personnel management department on the employees. It is assumed that the evaluation score range is 1 to 5, where 5 represents the highest and 1 represents the lowest. After weighting the evaluation scores of employees by different departments or personnel, the final evaluation results of each employee can be obtained.
It may be assumed that represents the evaluation result of the
-th employee for the
-th employee.
denotes the proportion of the score value of the
-th employee to the performance of the
-th employee. Thus, the performance evaluation value of the
-th employee can be obtained, and the calculation formula is as follows:
Where is the evaluation score of the
-th employee on the
-th index.
represents the number of personnel participating in performance appraisal,
shows the number of indicators for appraisal,
is the weight of the
-th indicator in the appraisal system under the corresponding dimension, and
indicates the weight of the dimension containing the
-th indicator in the whole appraisal system.
Results and Analysis
As shown in , it is the employee performance appraisal system constructed by the balanced scorecard method. It mainly includes four different dimensions, such as finance, personal work, enterprise management and customer service, which affect employee performance. Under each dimension, different evaluation indicators affecting employee performance are given. In addition, considering the importance of different dimensions and indicators in the performance appraisal system, the corresponding weights are given.
Table 1. Employee performance appraisal system based on balanced scorecard.
Using the employee performance appraisal scoring method, the performance appraisal results of all employees can be obtained according to the above appraisal system. As shown in , it is the performance appraisal results of some employees.
Table 2. Performance appraisal results of some employees randomly sampled.
In addition, we also compare the performance appraisal model proposed in this paper with the traditional performance appraisal method. As shown in , it reflects the comparison of the results of employee performance assessment by different methods.
In order to show the feasibility and superiority of the performance appraisal method proposed in this paper, the satisfaction analysis of the performance appraisal results obtained by different methods was carried out in the experiment. As shown in , the analysis and comparison of satisfaction after performance evaluation by different methods are given. From the comparison results reflected in the figure, it can be seen that the satisfaction obtained by using the performance evaluation method proposed in this paper is generally better than the traditional method.
Figure 10. Comparison of employee performance appraisal satisfaction rate obtained by different methods.
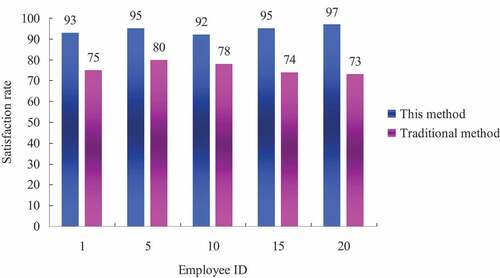
It is important to note that this paper assumes a basic understanding of statistical analysis techniques and human resource management. While the paper does not go into great detail about the specific dataset used in the study, it does provide a general overview of the performance appraisal process and how it can be improved using advanced statistical analysis techniques. The proposed method is based on the use of Bayesian network analysis and the balanced scorecard method to build a performance appraisal system. While the specifics of the dataset used in the study are not provided, the results of the experiments demonstrate the effectiveness of the proposed method in improving the objectivity and adaptability of traditional performance appraisal models. Overall, the research in this paper emphasizes the importance of using advanced statistical analysis techniques and new technologies to improve the objectivity and adaptability of traditional performance appraisal models in the context of human resource management. The paper provides practical guidance for improving the performance appraisal process in modern enterprises.
Conclusion
With the rapid development of new technologies such as economic society, computer and big data, the problems of traditional human resource performance management can not meet the actual needs of modern enterprise development. This paper used big data analysis and new technology methods to put forward the research of human resource performance evaluation model based on Bayesian network. By analyzing the relevant concepts of performance appraisal and its important role in enterprise management, this paper explained the human resources appraisal indicators and their impact on employee performance, and analyzed the common application methods in building the performance appraisal system. The proposed method is based on the use of Bayesian network analysis and the balanced scorecard method to build a performance appraisal system. The experiments conducted in the study provide evidence of the effectiveness of the proposed method in improving the objectivity and adaptability of traditional performance appraisal models. Then, the paper expounded the relevant theory of Bayesian network and its application advantages in solving random problems, and proposed a human resource performance evaluation model based on Bayesian network. In this paper, the balanced scorecard method was used to build a performance appraisal system, and the performance appraisal model proposed in this paper was tested by an example. The results of the experiments demonstrate the ability of the proposed method to identify and address typical patterns in the data set. For example, the method can help to identify biases in the appraisal process and provide insights into how to adjust the process to make it more objective and effective. Overall, the research in this paper emphasizes the importance of using advanced statistical analysis techniques and new technologies to improve the performance appraisal process in the context of human resource management.
One of the main advantages of the proposed method is its ability to improve the objectivity and adaptability of traditional performance appraisal models. The use of advanced statistical analysis techniques allows us to identify biases in the appraisal process and develop more effective strategies for managing human resources in modern enterprises. Furthermore, the balanced scorecard method provides a comprehensive framework for evaluating employee performance that takes into account a range of factors, including financial performance, customer satisfaction, and employee development.
However, there are also some potential drawbacks to the proposed method. One potential issue is the complexity of the Bayesian network analysis, which may require specialized knowledge and expertise to implement effectively. Additionally, the use of advanced statistical analysis techniques may require a significant investment in time and resources, which may not be feasible for all organizations.
Overall, the proposed method represents an important step forward in the field of human resource management. By incorporating advanced statistical analysis techniques and new technologies, we can develop a more nuanced understanding of the performance appraisal process and how it can be improved to meet the needs of modern enterprises. While there are potential drawbacks to the proposed method, we believe that the benefits of the approach outweigh the costs, and we encourage further research and development in this area.
Disclosure statement
No potential conflict of interest was reported by the author(s).
Additional information
Funding
References
- Alsaleh, M. N., E. Al-Shaer, S. Jajodia, G. Cybenko, V. S. Subrahmanian, and V. Swarup. 2020. Automated cyber risk mitigation: making informed cost-effective decisions. In Adaptive autonomous secure cyber systems, C. Wang and M. Wellman. ed., 131–1075. Cham: Springer International Publishing. doi:10.1007/978-3-030-33432-1_7.
- Atienza, D., C. Bielza, and P. Larranaga. 2022. Semiparametric Bayesian networks. Information Sciences 584:564–82. doi:10.1016/j.ins.2021.10.074.
- Atienza, D., P. Larranaga, and C. Bielza. 2022. Hybrid semiparametric Bayesian networks. TEST 31 (2):299–327. doi:10.1007/s11749-022-00812-3.
- Beliveau, A., and P. Gustafson. 2021. A theoretical investigation of how evidence flows in Bayesian network meta-analysis of disconnected networks. Bayesian Analysis 16 (3):803–23. doi:10.1214/20-BA1224.
- Canonne, C. L., I. Diakonikolas, D. M. Kane, and A. Stewart. 2020. Testing Bayesian networks. IEEE Transactions on Information Theory 66 (5):3132–70. doi:10.1109/TIT.2020.2971625.
- Cengiz, M. A., E. Dunder, and T. Senel. 2018. Energy performance evaluation of OECD countries using Bayesian stochastic frontier analysis and Bayesian network classifiers. Journal of Applied Statistics 45 (1):17–25. doi:10.1080/02664763.2016.1257586.
- Chiang, H. Y., and B. M. T. Lin. 2020. A decision model for human resource allocation in project management of software development. IEEE Access 8:38073–81. doi:10.1109/ACCESS.2020.2975829.
- Gao, X. G., Y. Yang, and Z. G. Guo. 2019. Learning Bayesian networks by constrained Bayesian estimation. Journal of Systems Engineering and Electronics 30 (3):511–24. doi:10.21629/JSEE.2019.03.09.
- Goes, A. S., and R. C. Oliveira. 2020. A process for human resource performance evaluation using computational intelligence: an approach using a combination of rule-based classifiers and supervised learning algorithms. IEEE Access 8:39403–19. doi:10.1109/ACCESS.2020.2975485.
- Hu, W. X., and S. K. Zhao. 2020. StrategiC human management evaluation for marine enterprises. Journal of Coastal Research 107 (sp1):57–60. doi:10.2112/JCR-SI107-015.1.
- Li, L. T., and B. Pahlevanzadeh. 2022. Evaluation of the trust values among human resources in the enterprise cloud using an optimization algorithm and fuzzy logic. KYBERNETES 51 (6):2008–29. doi:10.1108/K-04-2021-0280.
- Lidinska, L., and J. Jablonsky. 2018. AHP model for performance evaluation of employees in a Czech management consulting company. Central European Journal of Operations Research 26 (1):239–58. doi:10.1007/s10100-017-0486-7.
- Luo, S. Z., L. N. Xing, and T. Ren. 2022. Performance evaluation of human resources based on Linguistic Neutrosophic Maclaurin Symmetric mean Operators. Cognitive Computation 14 (2):547–62. doi:10.1007/s12559-021-09963-1.
- Nasar, N., S. Ray, S. Umer, and H. M. Pandey. 2021. Design and data analytics of electronic human resource management activities through internet of things in an organization. Software: Practice & Experience 51 (12):2411–27. doi:10.1002/spe.2817.
- Scanagatta, M., G. Corani, C. P. Campos, and M. Zaffalon. 2018. Approximate structure learning for large Bayesian networks. Machine Learning 107 (8–10):1209–27. doi:10.1007/s10994-018-5701-9.
- Scutari, M. 2020. Bayesian network models for incomplete and dynamic data. Statistica Neerlandica 74 (3):397–419. doi:10.1111/stan.12197.
- Shen, B. 2020. Construction of performance evaluation system of human resource management in port foreign trade enterprises. Journal of Coastal Research 103 (sp1):217–21. doi:10.2112/SI103-047.1.
- Sohail, A., D. D. Dominic, M. Hijji, and M. A. Butt. 2021. Competency driven resource evaluation method for business process intelligence. Computers, Materials & Continua 69 (1):1141–57. doi:10.32604/cmc.2021.018023.
- Wang, L. J., X. Yuan, and M. Elhoseny. 2019. Research on human resource performance and decision-making evaluation based on Fuzzy mathematics and Clustering model. Journal of Intelligent & Fuzzy Systems 37 (1):171–84. doi:10.3233/JIFS-179075.
- Zhao, C., Y. Xue, T. Niu, X. Yuan, and M. Elhoseny. 2021. Enterprise human resource management index based on fuzzy system. Journal of Intelligent & Fuzzy Systems 40 (2):3137–46. doi:10.3233/JIFS-189352.
- Zhao, H., X. S. Ding, and J. C. Wang. 2020. Optimize the evaluation index system of internal human resource management and control of ocean shipping companies. Journal of Coastal Research 112 (sp1):461–64. doi:10.2112/JCR-SI112-122.1.
- Zhou, L. L., R. Mao, and P. Li. 2020. Performance evaluation of environmental management for sustainable development of enterprises based on human resource management. International Journal of Environment and Pollution 68 (3/4):178–98. doi:10.1504/IJEP.2020.120158.
- Zhou, L. T., Q. G. Wu, and H. Chen. 2022. Multifactor evaluation approach for human performance. Arabian Journal for Science and Engineering 47 (3):3763–72. doi:10.1007/s13369-021-06091-8.