ABSTRACT
This study investigates the discrete effects of inquiry-based instructional practices that described the PISA 2015 construct ‘inquiry-based instruction’ and how each practice, and the frequency of each practice, is related to science achievement across 69 countries. The data for this study were drawn from the PISA 2015 database and analysed using hierarchical linear modelling (HLM). HLMs were estimated to test the contribution of each item to students’ science achievement scores. Some inquiry practices demonstrated a significant, linear, positive relationship to science achievement (particularly items involving contextualising science learning). Two of the negatively associated items (explaining their ideas and doing experiments) were found to have a curvilinear relationship to science achievement. All nine items were dummy coded by the reported frequency of use and an optimum frequency was determined using the categorical model and by calculating the inflection point of the curvilinear associations in the previous model e.g. students that carry out experiments in the lab in some lessons have higher achievement scores than students who perform experiments in all lessons. These findings, accompanied by detailed analyses of the items and their relationships to science outcomes, give stakeholders clear guidance regarding the effective use of inquiry-based approaches in the classroom.
Introduction
The effectiveness of inquiry-based instruction in science education has been widely investigated in the research literature for both experimental studies (see Furtak, Seidel, Iverson, & Briggs, Citation2012; Minner, Levy, & Century, Citation2010) and correlational studies (see Cairns & Areepattamannil, Citation2019; Jerrim, Oliver, & Sims, Citation2019). This study will use data from the 2015 Programme for International Student Assessment (PISA) cycle. The PISA project is an OECD initiative that takes place as part of a three year cycle in which 15-year-old students, across all participating countries, are assessed in terms of their reading-, mathematics- and science-literacy to determine their work-place readiness at (or near) the end of their compulsory schooling experience (OECD, Citation2017a). This correlational study will investigate the items that were used to generate the inquiry-based instruction scale in the 2015 PISA student questionnaire and analyse the individual approaches they represent and their apparent effectiveness over a range of exposure frequencies, with regards to student achievement.
Inquiry-based instruction
As this literature review will illustrate, there is an ongoing debate regarding the effectiveness of inquiry-based instruction in terms of student outcomes. Despite this uncertainty, there is a strong theoretical case for using inquiry-based instructional approaches (see Constantinou, Tsivitanidou, & Rybska, Citation2018; Spronken-Smith, Citation2008). Inquiry-based instruction is largely grounded in constructivist theory and is an inductive teaching (‘bottom-up’) approach (Prince & Felder, Citation2006). That is, the individual learner constructs their own understandings during the learning process. Furthermore, inquiry-based instruction approaches enable learners to develop higher-order cognitive skills that allow for the application of their deeper understanding of scientific principles to everyday phenomena (Anderson, Citation2002; Constantinou et al., Citation2018). Also, including the features of authentic science inquiry (such as generating research questions and controlling for multiple dependent variables) in school-based tasks encourages the development of epistemological awareness and a better understanding of how scientific knowledge is created (Chinn & Malhotra, Citation2002). Finally, the active, more self-directed, and student-centred nature of inquiry-based instruction allows students to act as participants in knowledge generation rather than as passive audiences that receive knowledge. This shift in responsibility combined with increased levels of autonomy and self-direction, improved higher order thinking skills, and a more sophisticated epistemological understanding encapsulates the theoretical benefits of inquiry-based instruction (Constantinou et al., Citation2018).
As mentioned above, the term ‘inquiry-based instruction’ is not clearly conceptualised in the literature (Anderson, Citation2002; Furtak et al., Citation2012; Hmelo-Silver, Duncan, & Chinn, Citation2007; Kirschner, Sweller, & Clark, Citation2006; Minner et al., Citation2010). However, each conceptualisation usually consists of two or three of the following core elements. Firstly, inquiry-based instruction often includes activities that teach science investigation skills that are required pre-requisites for handling scientific equipment, generating and manipulating data and making inferences. Also, in an inquiry-oriented approach, students learn about the nature of scientific knowledge and the processes used to generate scientific knowledge. Finally, there is a simulation of the scientific inquiry process in the classroom, whereby students develop conceptual understanding through investigating phenomena using methods similar to a practicing scientist.
As such, science educators usually agree that inquiry-based instructional approaches are an suitable model for teaching science. Theoretically, it is a methodology that not only delivers science content in an interactive and engaging manner, it also simulates the working processes of real scientists. However, the research literature regarding the effectiveness of inquiry-based instruction is inconclusive.
Experimental studies of inquiry-based instruction
The body of research measuring the effectiveness of inquiry-based instruction, implemented as a treatment condition, indicates that this approach produces positive results. Meta-analyses carried out in the 1980s that analysed the implementation of inquiry-oriented (reform) curricula in the preceding decades, demonstrated moderate, positive effect sizes for inquiry-based instruction when compared with ‘traditional’ approaches. For example, Shymansky, Kyle, and Alport (Citation1983) reported a ‘general achievement’ effect size of 0.43, Wise and Okey (Citation1983) showed an effect size of 0.41 for ‘inquiry-discovery’ approaches and Weinstein, Boulanger, and Walberg (Citation1982) found a positive effect size for innovative, inquiry-oriented curricula of 0.31. Another study that distinguished between overall science achievement and science process skills discovered a mean effect size of 0.35 and a larger effect size of 0.52 for outcomes relating only to science skills (Bredderman, Citation1983).
In more recent times an educational technology focused inquiry-based instruction project reported an effect size of 0.44 for their first cohort and 0.37 for their second cohort (Geier et al., Citation2008). The positive effects of inquiry-based instruction were also demonstrated over a three-year period, for novice teachers undertaking a professional development and coaching project (Borman, Gamoran, & Bowdon, Citation2008). In his comprehensive analysis of teaching practices Hattie (Citation2009) analysed over 800 meta-analyses. Included in his research synthesis was the teaching approach of ‘inquiry-based teaching’ and a moderate overall mean effect size (reported as 0.31) was determined. A more recent meta-analysis using studies from 1996 to 2006 reported an effect size for inquiry teaching of 0.50 (Furtak et al., Citation2012).
Although these studies are positive, the operationalisation of inquiry-based instruction is important when interpreting their results. As an example, Furtak et al. (Citation2012) categorised inquiry-based instructional studies in terms the level of support the students received. Guided-inquiry involves the teacher completing or leading certain elements of the inquiry cycle such as providing the research question and supporting the development of their investigation (Colburn, Citation2000). Open-inquiry demands more input from the students as they are responsible for developing their own research questions, designing an experiment and drawing their own conclusions (Barman, Citation2002). However, the open-inquiry approach has been heavily criticised in the literature (Kirschner et al., Citation2006; Klahr & Nigam, Citation2004; Sweller, Kirschner, & Clark, Citation2007). One common criticism is the concept of cognitive overload arising from students not possessing the underlying knowledge and skills to access the inquiry process (Kirschner et al., Citation2006). As (Hmelo-Silver et al., Citation2007) pointed out, open-inquiry does not necessarily mean unsupported inquiry and recommended that, through the use of various scaffolds, elements of the open-inquiry process can be effectively supported.
Inquiry-based instruction can also be operationalised in terms of the conceptual domains covered during its implementation. Furtak et al. (Citation2012) categorised inquiry-based instruction into cognitive domains. The effect size was calculated using the contrast of the domains present in the treatment and the control conditions. The studies that contrasted the epistemic domain yielded the highest effect size (0.75). This implied that during the inquiry-based instructional process, attention should be focused on developing the epistemic element.
Correlational studies of inquiry-based instruction
In contrast to the generally positive effects of inquiry-based instruction illustrated by the experimental literature, several cross-sectional research studies that use PISA data have reported an inverse relationship between the frequency of student exposure to inquiry teaching and science literacy (Areepattamannil, Citation2012; Areepattamannil, Freeman, & Klinger, Citation2011; Cairns & Areepattamannil, Citation2019; Lavonen & Laaksonen, Citation2009; Organisation for Economic Co-operation and Developmen [OECD], Citation2016b). These studies use the inquiry-based instruction scaled index as a predictor measure and assume a linear relationship with science achievement. For example, as stated by Cairns and Areepattamannil (Citation2019), the relationship between the frequency of exposure to inquiry-based instruction is negatively associated with science achievement, this implies that the less inquiry-based instruction students experience, the better they will score in science assessments. However, the relationship between the variables in large-scale international assessments is likely more complex (Caro, Lenkeit, & Kyriakides, Citation2016) and possibly non-linear. Methods of addressing possible non-linear associations include the use of quadratic predictors (Teig, Scherer, & Nilsen, Citation2018) and categorising the variables in question (Jerrim et al., Citation2019). For example, a study of Norwegian TIMSS 2015 data investigated the relationship between the equivalent inquiry-based instruction scale and science achievement in terms of linear and curvilinear relationships (Teig et al., Citation2018). The study reported that the linear relationship was positive and non-significant, yet the curvilinear relationship was negative and significant. This suggested that there was an optimum frequency of inquiry-based instruction after which, the relationship with science achievement was negative (Teig et al., Citation2018). A longitudinal study investigating the effects of inquiry-based instruction on science examination results in England categorised their adapted inquiry scale into quartiles relating to the reported frequency of inquiry-based instruction (Jerrim et al., Citation2019). They reported that, although the frequency of exposure to inquiry approaches were not significantly associated with science achievement, a small, positive association was demonstrated for students that experienced high frequencies of inquiry-based instruction and high levels of teacher support (Jerrim et al., Citation2019).
Some researchers have raised questions regarding the nature of the PISA 2015 inquiry-based instruction scale itself (Lau & Lam, Citation2017). The nine items in the inquiry-based instruction construct in the 2015 questionnaire originated from a range of scales (interactive teaching, hands-on activities, student investigations, and models or applications) in the PISA 2006 questionnaire (OECD, Citation2009). Of these, 5 items were included in the 2015 questionnaire, unchanged, and 2 items were modified slightly. The items used in the PISA 2015 questionnaire to represent inquiry-based instruction are listed in Appendix A.
Why these items were selected and then considered to capture the inquiry-based instruction construct is not made completely clear in the PISA documentation. Lau and Lam (Citation2017) opined that the ‘new construct is neither clearly defined not well justified with the theories and literature’. However, according to the PISA 2015 Assessment and Analytical Framework (OECD, Citation2017a) the nine items were selected to cover elements of inquiry-based instruction such as contextualised learning (Fensham, Citation2009), scientific argumentation (Osborne, Citation2012), active thinking and drawing conclusions from data (Minner et al., Citation2010). These decisions appear have also been informed by analyses of the PISA 2006 results in terms of the instructional methods included in the PISA 2006 scales mentioned above (Taylor, Stuhlsatz, & Bybee, Citation2009). For example, Taylor et al. (Citation2009) found that three items later included in the 2015 scaled index were correlated with high science achievement.
There are also cross-sectional studies that analyse the inquiry-based instruction scaled index by exploring the items as individual explanatory variables, revising the PISA index or further investigating nonlinear relationships. Using an index constructed based on their own conceptual framework, Jiang and McComas (Citation2015) categorised the PISA 2006 student background questionnaire inquiry-based instruction statements by the likely level of teacher support received at varying reported frequencies. The level of support was derived for each activity based on the pioneering work of Schwab (Citation1962). Students that experienced the highest level of open inquiry had lower scientific literacy scores than students that experienced a medium level of guidance. In a report produced by McKinsey & Company regarding the PISA 2015 results for Europe, a non-linear relationship between inquiry-based instruction and PISA science scores was identified (Denoël et al., Citation2018). In EU countries the optimum frequency of experiencing inquiry-based instruction approaches (using the original IBTEACH scale) was ‘in some classes’ with teacher-directed instruction recommended as a pre-requisite. This report also investigated the discrete effects of practices described by the individual inquiry items and identified structured teaching techniques as more successful than unstructured methods. Using data for the ten top performing regions in PISA 2015, Lau and Lam (Citation2017) developed two new constructs from the PISA inquiry-based instruction scale: interactive investigation and interactive application. They reported that, across the ten regions, interactive application was the most positive teaching practice and interactive investigation was the most negative teaching approach. Using structural equation modelling, Aditomo and Klieme (Citation2019) separated the inquiry-based instruction scaled index into two factors (‘guided’ and ‘independent’ inquiry). The study reported a positive relationship with science achievement across the 16 regions where guided-inquiry practices were observed and a negative relationship with independent-inquiry approaches.
This study
Despite the considerable level of scrutiny of the inquiry-based instruction scaled index, the research in this area has yet to investigate the individual effect of each discrete item from the index in terms of the reported frequency of experiencing each practice, on student science achievement, across countries. In this study the discrete effects of inquiry-based instructional practices that were used to generate the ‘inquiry-based instruction’ construct and the reported frequency of each are related to PISA science scores across 69 countries. Data from all participating countries in the PISA 2015 cycle were utilised, however, some countries were removed from the analysis due to high levels of missing data for the variables of interest. The remaining 69 countries are listed in Appendix B. This study aims to address the following research questions:
What is the relationship between the frequency of experiencing specific inquiry-based instruction approaches and science achievement?
What is the relationship between specific frequencies of exposure to components of inquiry-based instruction and science achievement?
Analysis
Data
The school and student level data for this study were drawn from the questionnaires in the PISA 2015 database (http://www.oecd.org/pisa/data/2015database). The questionnaires used in this study include student-level data (the assessment results for science literacy and the demographic, attitudinal and background questionnaire for each student) and school-level data (a background questionnaire completed by principals relating to whole school issues). Country-level data was drawn from the World Bank database (https://data.worldbank.org/indicator) and the Central Intelligence Agency (CIA) world fact book (https://www.cia.gov/library/publications/the-world-factbook). Log GDP per capita was used to provide a comparison of wealth generation per person on a manageable, linear scale and the income Gini coefficient was included as a measure of income distribution within a country.
The sample data included in the present study consisted of 514,119 15-year-old students (male = 183,695 (49%), female = 192,061 (51%); Mage = 15.78 years, SD = .29) from 17,681 schools in 69 countries. The list of countries and economies included is given in Appendix (Table B1) and due to the high sample size, a conservative significance criterion was used (p < .001).
Measures
Outcome measures
The outcome measure for this study was student science achievement as measured by domain-specific science assessments in PISA 2015. The rotational test design employed allowed for the construction of a continuous proficiency scale based on the relative difficulty of the items they answered (OECD, Citation2016a). In other words, item response theory (IRT) was used to scale the student’s proficiency score resulting in plausible values, which are a range of multiply imputed values derived from the student’s performance according to the procedure discussed above (IRT scaling), combined with a regression model based on the student’s answers to the background questionnaire (OECD, Citation2009). PISA 2015 generated 10 plausible values per student that were used to run 10 separate analyses and pooled to yield the parameter estimates for a given model.
Predictor measures
For the purposes of this study, individual items of the inquiry-based instruction construct were used because the effects of the individual approaches were the focus of this research. This approach was also driven by the problematic nature of the construct itself (as discussed above). The predictor measures used in this study were the nine individual items (see Appendix A) from the inquiry-based instruction scaled index (IBTEACH) in the PISA 2015 student questionnaire (OECD, Citation2017b).
The raw scores of individual inquiry scale items were reverse-coded [1 = never or hardly ever, 4 = in all lessons] as a means of determining the relationship between science achievement and the frequency of each discrete practice described by the item. In order to investigate the relationship of these items in terms of specific descriptors of frequency and the relationship with science achievement, dummy coded inquiry items were generated with three categories per item [in some lessons, in most lessons and in all lessons]. The reference category was ‘never or hardly ever’.
Student-, school-, and country-level control variables
The student-, school-, and country-level demographic and socioeconomic control variables included in the study were gender (1 = female, 0 = male), immigration status (1 = non-immigrant, 0 = immigrant), the index of economic, social, and cultural status (ESCS; a composite score of highest level of education of parents, highest parental occupational status, and home possessions; see OECD, Citation2017b), school ownership type (1 = public, 0 = other), school location (1 = rural, 0 = urban), index of schools’ science-specific resources, income Gini coefficient, and log GDP per capita.
Statistical analyses
To calculate descriptive statistics and to check for multicollinearity of the predictors the IEA IDB Analyzer: Analysis Module (version 4.0.23) (https://www.iea.nl/data) combined with the IBM© SPSS© software package was used. I employed hierarchal linear modelling (HLM) analyses using the software package HLM 7.03 for Windows (Stephen W. Raudenbush, Bryk, Cheong, & Toit, Citation2011) to test the hypothesised model and to answer the research questions. The parameter estimation technique used in the three-level HLM analysis full information maximum likelihood (FIML) (McCoach, Citation2010; Stephen W. Raudenbush & Bryk, Citation2002). In all models, the continuous variables were grand-mean centred to facilitate interpretation of the beta coefficients (Heck & Thomas, Citation2015) and to also mitigate the effects of multicollinearity (Tabachnick & Fidell, Citation2014).
Data from the student- and school-level questionnaires were used with the country-level data drawn from the World Bank database and the CIA world factbook to build a series of three-level models. Prior to running these models an intercept only model was estimated to partition the variance in the outcome measure (science achievement) into student-, school- and country-level variance components (level 1, 2 and 3, respectively).
Prior to the disaggregation of the of the inquiry-based instruction scaled index (IBTEACH) the relationship between the frequency of experiencing inquiry-based instructional approaches and science achievement was estimated using a three-level HLM which predicted a significant, negative relationship (B = −6.53, p < 0.001). Further to this, a two-level HLM was estimated for each country/region included in the study. The relationship between inquiry-based instruction and science achievement was found to be negative and significant (e.g. Estonia, B = −17.91, p < 0.001), or non-significant (e.g. Finland) for every region. No regions predicted a positive, significant relationship. For more detailed information regarding these analyses, see the supplementary material provided. As a result of this high level of isomorphism across countries in terms of inquiry-based instruction and science achievement, and in order to retain the maximum quantity of data for the analyses, all countries with sufficient data under the variables of interest were included in the models described below.
To answer research question 1 a three-level model was estimated that included the inquiry-based instruction items (as described above) as predictors and the student-, school- and country-level variables as covariates. A further model was estimated, informed by theory, which included the quadratic terms for ST098Q01TA and ST098Q02TA to test for a curvilinear relationship with the outcome variable. To answer research question 2, a three-level model was built that included the dummy-coded inquiry-based instruction items (coded for each level of frequency; ‘in some lessons’, ‘in most lessons’, ‘in all lessons’ and ‘never or hardly ever’ as the reference category). This final categorical model contained 27 explanatory variables.
The multiple imputation process was employed to account for the missing data (missing data per item, M = 6%) and 10 imputed data sets were generated using the multiple imputation function in the IBM© SPSS© software package. This method was used (instead of approaches such as list wise deletion) to avoid a reduction in power and to reduce parameter estimate bias caused by data that is not missing completely at random (Enders, Citation2010). The 10 imputed data sets were merged with the 10, previously imputed, plausible values for each student outcome measure (science literacy) as described in the PISA documentation (OECD, Citation2016a) and normalised student weights were included in the analysis to account for the two-stage stratified sampling methods employed (OECD, Citation2017b).
Results
shows the means, standard deviations and correlations between the reverse-coded items used in the inquiry-based instruction scale. Each item is significantly, positively correlated with the other items suggesting that as the frequency of each item increases so do the other items in the scale. In terms of multicollinearity, the correlations between the independent variables (<0.7) and the corresponding tolerance statistics (Mtolerance = 0.51) were moderate (Hutcheson & Sofroniou, Citation1999). In light of this, all items were included in the model. Similar statistics were calculated for the dummy-coded variables in the categorical model and, again, only moderate correlations were detected.
Table 1. Descriptive results and correlations between the reverse-coded items used in the inquiry-based instruction scale.
The relationship between individual inquiry items and science achievement was estimated using a three-level HLM approach. The multi-level intercept-only model estimated a grand mean science achievement score γ0.00 = 460.71, and statistically significant variation in the random effects for science achievement score values between students (level 1: σ2 = 5150.27), across schools (level 2: τπ000 = 2718.29), and across countries (level 3: τβ000 = 2616.82). The intra-class correlation coefficients (ICCs) indicated that 49% of the science achievement score variance occurred between students, 26% of the variance occurred across schools, and 25% occurred across countries.
Research question 1
The full HLM model using the reverse-coded inquiry-based instruction scale items as explanatory variables, after accounting for student-, school-, and country-level demographic characteristics as well as students’ dispositions toward science, indicated some considerable differences in the associations between each of the variables and science achievement (). The strategy of giving students opportunities to explain their ideas was significantly, positively associated with science achievement (B = 3.55, p < 0.001) suggesting that a one-unit increase in the frequency of experiencing this approach corresponded to a 3.55-point increase in science achievement score. Similar effects were observed for the approaches of the teacher explaining how a science idea can be applied (B = 11.99, p < 0.001) and the teacher clearly explaining the relevance of science concepts to the lives of the students (B = 6.25, p < 0.001). For several strategies, a significant, negative relationship with science achievement was observed. When students are allowed to design their own experiments a one-unit increase in the frequency of this approach corresponded to a 11.03-point decrease in science achievement (B = −11.03, p < 0.001). The approaches of having a class debate about investigations (B = −7.48, p < 0.001) and asking students to do an investigation to test ideas (B = −4.59, p < 0.01), had a similar, negative effect. The strategies of allowing students to spend time in the laboratory doing practical experiments, requiring students to argue about science questions and, asking students to draw conclusions from an experiment they have conducted had no significant, linear relationship with science achievement.
Table 2. Inquiry-based instruction by item predicting science achievement.
The full HLM model accounted for 62% of the variance in science achievement at level 1, 22% of the variance at level 2, and 17% of the variance at level 3 as compared to the demographic model that accounted for only 60% of variance at level 1 and 23% and 16% of the variance at level 2 and 3, respectively (see ).
To test for the hypothesised non-linear relationship of two of the approaches (ST098Q01TA and ST098Q02TA), another three-level HLM was estimated that included the quadratic terms for the two items. The relationship with science achievement, in both cases, was found to be negative and significant (B = −5.01, p < 001 and B = −5.81, p < 0.001, respectively) suggesting a curvilinear relationship (Teig et al., Citation2018). The inflection point, for each item, was calculated from the first derivative of the quadratic function and found to be 2.94 (approximately equivalent to a frequency of in most lessons) when giving students opportunities to explain their ideas and 2.02 (approximately equivalent to a frequency of in some lessons) when students spend time in the laboratory doing practical experiments.
Research question 2
The results of the full HLM model, accounting for the same demographical covariates as the previous model but including the dummy-coded inquiry-based instruction items (the categorical model) are shown in . In terms of the frequencies of experiencing these approaches the categories involved are never, or almost never (the reference category), in some lessons, in most lessons and in all lessons. The significant individual approaches and their frequency of use, as related to science achievement, emerged as three main groups. The first group contained strategies that had a positive relationship to science achievement and also, generally, increased with the frequency of use: the teacher explaining how a science idea can be applied and the teacher clearly explaining the relevance of science concepts to their everyday lives (see ). The second group consisted of strategies that had a negative relationship to science achievement at all frequencies of use, or had a non-significant relationship at lower frequencies (in most and in some lessons) and a negative, significant relationship at high frequencies (in all lessons): students are allowed to design their own experiments, there is a class debate about investigations and students are asked to do an investigation to test ideas (see ). The final group consisted of approaches where the frequency of exposure to these strategies appeared to have a non-linear relationship with science achievement: students are given opportunities to explain their ideas; students spend time in the laboratory doing practical experiments (see ). For these two approaches the optimum frequency of use appeared to be in most lessons (B = 12.78, p > 0.001) and in some lessons (B = 5.56, p > 0.01), respectively.
Figure 1. Inquiry-based approaches associated with increased levels of achievement.
Note: The error bars represent ±1 SD.
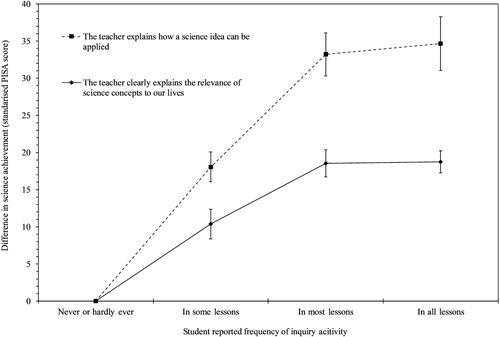
Figure 2. Inquiry-based approaches associated with decreased levels of achievement.
Note: The error bars represent ±1 SD.
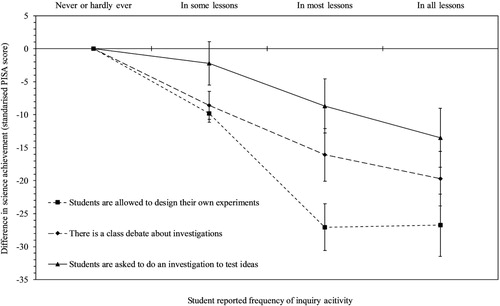
Figure 3. Inquiry-based approaches exhibiting a curvlinear relationship to achievement.
Note: The error bars represent ±1 SD.
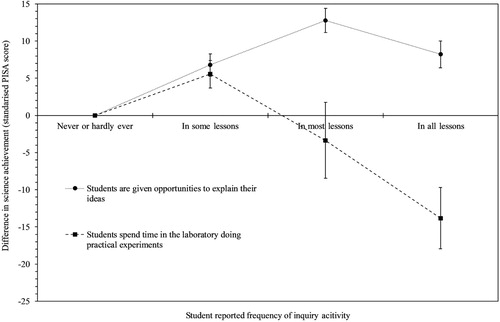
Table 3. Inquiry-based instruction by dummy-coded item predicting science achievement.
Discussion
The approaches found to be associated with the highest levels of achievement at all frequencies of reported exposure involved the teacher explaining how a science idea can be applied and the teacher clearly explaining the relevance of science concepts to their everyday lives. Both approaches had a significant, positive relationship with science achievement. The categorical model () demonstrates that their relationship with science achievement steadily increases with frequency, at least until the maximum frequency of in all lessons. At this level of reported exposure there is little or no difference in science achievement compared to the previous level (in most lessons) (see ). The inclusion of these two items in the inquiry-based instruction index scale indicates that real-world contextualisation and application are considered to be components of inquiry-based teaching and learning by the OECD. Certainly, in an analysis of the 2006 PISA results Taylor et al. (Citation2009) referred to the necessity of ideas to be relevant in order for students to perceive a reason for, and therefore a use for, new knowledge. Further, the authentic education movement has long espoused the benefits of contextualised learning in terms of improved conceptual understanding and the development of higher-order thinking skills (Friesen & Jardine, Citation2009). In essence, approaches to inquiry that reflect the principles of authentic education allow for students to experience a junior version of knowledge generation in a specific discipline (Scott, Smith, Chu, & Friesen, Citation2018). However, whether the practice of contextualising learning and providing real-life applications of knowledge is specific to inquiry-based instruction is questionable. For example, it is considered the good practice to provide real-world examples and contexts in science lessons in order to engage and enthuse students (Whitelegg & Parry, Citation1999; Wieman & Perkins, Citation2006), regardless of the other instructional approaches employed. Concerns regarding these two items were also voiced by Jerrim et al. (Citation2019) in a longitudinal study of inquiry-based instruction that involved the use of the PISA 2015 data. ST098Q06TA and ST098Q09TA were removed from their analysis and described as relating to teacher-directed instruction (Jerrim et al., Citation2019), particularly in light of the teacher-centred focus of the statements. Further, previous conceptualisations of inquiry-based instruction have not explicitly referred to real-life application or real-life relevance of knowledge as integral to this instructional approach. A commonly quoted standard regarding the elements of inquiry-based instruction is the definition provided in the 1996 National Science Education Standards (National Research Council, Citation1996). Although this document stated that ‘inquiry learning’ should be authentic the features of inquiry-based instruction outlined in a supplementary publication named ‘Inquiry and the National Science Education Standards’ (Loucks-Horsley & Olson, Citation2000) contained no mention of the real-life application of science concepts. Minner et al. (Citation2010) and Furtak et al. (Citation2012) operationalised inquiry-based instruction resulting in the identification of inquiry domains (and sub-domains). Neither of these major studies mentioned the real-life application of scientific knowledge. In an analysis of articles that relate to the phases of inquiry-based instruction Manoli et al. (Citation2015) classified future-oriented elements of inquiry, such as applying new knowledge to practical (real-life) problems, as not directly related to the inquiry-learning cycle. In light of this, there are certainly ample reasons to question the inclusion of these approaches in the inquiry-based instruction scaled index.
Another group of activities in this analysis generally demonstrated a negative relationship with science achievement. These included the following: students are allowed to design their own experiments, there is a class debate about investigations, and students are asked to do an investigation to test ideas. The relationship with science achievement for these strategies was significant and negative at all levels of reported exposure (see and ). In other words, the more frequently students experienced these approaches the lower their related science achievement (see ). Two of the items in this group were included in the 2006 PISA cycle and analysed by Jiang and McComas (Citation2015). They reported that students that were frequently allowed to design their own experiments and do an investigation to test out their own ideas experienced an open-inquiry approach (level 3 out of 4 on a scale of ‘openness’). This level of openness was found to be less effective than level 2 where designing experiments and asking questions were teacher-directed. According to (Bell, Smetana, & Binns, Citation2005) students must master the previous (more guided) levels of inquiry-based instruction before tackling the higher levels. There is not sufficient information in the PISA questionnaires to ascertain if these preparation steps have taken place. There is some agreement in the research literature that open, unguided inquiry-based instruction is largely ineffective and perhaps even detrimental, in terms of student outcomes (Denoël et al., Citation2018; Furtak et al., Citation2012; Hmelo-Silver et al., Citation2007; Jiang & McComas, Citation2015; Kirschner et al., Citation2006; Klahr & Nigam, Citation2004). In a report by McKinsey & Company, unstructured inquiry-based practices were related to negative effects on student outcomes, in EU and non-EU countries alike (Denoël et al., Citation2018). The ‘harmful’ unstructured activities identified in the report included all three items found to have a negative association with science achievement, at all frequencies, in the present study (ST098Q07TA, ST098Q08NA and ST098Q10NA). This body of evidence suggests that open inquiry-based instructional activities should be used sparingly or only when students have sufficient foundational preparation to access these methods of learning (Bell et al., Citation2005; Denoël et al., Citation2018; Kirschner et al., Citation2006). For example, there is some evidence suggesting an interplay between direct-instruction and inquiry-based instruction where clear explanations of science phenomena delivered by the teacher (in many or in all lessons) are precursors to effective structured and unstructured inquiry-based instruction (Denoël et al., Citation2018). Another concern regarding open-inquiry approaches is the question of teaching quality. The PISA questionnaires only provide the student-reported frequency of these strategies yet, open inquiry requires considerable skill to deliver effectively as it is essentially a simulation of the work of practicing scientists (Colburn, Citation2000). This was illustrated in a study involving 34 ‘early adopter’ teachers in Australia implementing practices that align with open-inquiry. They described how the quality of these approaches was undermined by limitations such as, a lack of teacher self-efficacy, health and safety concerns, IT limitations, a lack of effective professional development and time constraints within the curriculum (Fitzgerald, Danaia, & McKinnon, Citation2019). A final issue I considered is the causal direction of this relationship. In most discussions relating to the negative association of inquiry-based instruction practices and science achievement (as above) the implicit assumption is that lower science achievement is a result of students experiencing inquiry approaches more frequently. A suggestion in the PISA 2015 report (OECD, Citation2017b) and further discussed by (Lau & Lam, Citation2017) is that approaches such as asking students to carry out an investigation to test ideas may be used at higher frequencies as active engagement strategies for low achieving students. However, the results of this study do not support this. For example, if the causal relationship is reversed using the rationale described above, we would expect to see a linear, negative relationship for all the ‘hands-on’ activities included in the scale, but this is not the case.
A further group that exhibited a non-linear relationship with science achievement included: students are given opportunities to explain their ideas and students spend time in the laboratory doing practical experiments. This curvilinear relationship was verified using quadratic terms and from the coefficients estimated by the categorical model (see ). By calculating the inflection point using the first derivative of the quadratic function and comparing these values to the results of the categorical model the optimum frequency of exposure was obtained. When students were given opportunities to explain their ideas in most lessons the highest association with science achievement was observed. This was verified by the categorical model (see ) and the calculated value of the inflection point (2.94). This suggests that student explanations and argumentation are effective approaches and should be employed in most lessons. However, using these approaches in all lessons could have a detrimental effect. In order to effectively develop students’ scientific explanations and arguments, McNeill and Krajcik (Citation2008) reported that this skill first requires the sharing of an explicit rationale. Also, students explaining their ideas falls into the epistemic domain of inquiry-based instruction (Furtak et al., Citation2012; Sandoval & Reiser, Citation2004). The largely positive relationship demonstrated in this study aligns with findings in the experimental literature where studies that experienced inquiry approaches that included the epistemic domain reported higher effect sizes (Furtak et al., Citation2012). When students spent time in laboratory doing experiments in some lessons the relationship with science achievement was positive, yet at higher frequencies (in most or all lessons) the relationship is non-significant and becomes significant and negative at the highest frequency of exposure. This relationship was verified by the categorical model (see ) and the calculated value of the inflection point (2.01). Intuitively, science educators may argue that carrying out experiments in every science lesson might not be an efficient use of instructional time. However, in educational scenarios that focus heavily on inquiry-based instructional approaches, there is an expectation that students should develop scientific knowledge and understanding through carrying out experiments in the laboratory. Developing scientific conceptual understanding is a very complex process that requires considerable opportunities for reflection (Gunstone, Citation1991) and metacognitive activities such as the elaboration and application of learning (Hofstein & Lunetta, Citation2004). The evidence from this study suggests that in the average science lesson the use of experimental work is likely to be most effective when used in some lessons to target specific scientific concepts or inquiry skills. This would then allow sufficient instructional time to supplement the experimental work with reflection opportunities and possibly, teacher-directed approaches.
A final group of teaching methods found to have no significant relationship with science achievement (at any level of reported exposure), consisted of the following: students are required to argue about science questions; and students are asked to draw conclusions from an experiment they have conducted (see and ). It is unclear how students completing the PISA background questionnaire would perceive the statement ‘students are required to argue about science questions’. Scientific questions, in this sense, could well mean questions that are testable. In other words, the statement could be referring to arguing about scientific research questions or students may perceive this statement as arguing about conceptual scientific questions. This ambiguity could contribute to inconsistent self-reported responses in the questionnaire and lead to a net effect on achievement that is not significantly different to zero. The second item in the group, regarding students drawing conclusions, also has no significant relationship to science achievement. This is a surprising result considering that the process of drawing conclusions from experimental data is epistemic in nature (Furtak et al., Citation2012). However, the item used in the inquiry-based instruction index specifically states that students draw conclusions from experiments that they have conducted. As such, the issues regarding the accuracy and precision of student measurements and students’ general competencies in terms of manipulating scientific apparatus, could explain this insignificant relationship. For example, a study or the effectiveness of laboratory work in school science lessons for 11–16 year-olds reported that teachers almost exclusively focused on science content knowledge when students carried out experiments rather than developing inquiry skills (Abrahams & Millar, Citation2008). As such, the focus of these laboratory-based lessons was to reproduce a scientific phenomenon to illustrate a concept using a largely inductive approach, whereby the conceptual understanding would be enabled by an observable relationship in the data. However, in many cases the students reported different data values than those intended by the teacher and the desired understanding of the phenomena failed to emerge (Abrahams & Millar, Citation2008). If students must draw conclusions from experiments that they have conducted but the data they generate does not support the conceptual understanding of the phenomenon in question, then if follows that this teaching approach is unlikely to have a significant effect on scientific literacy.
Implications for teacher development
Despite the debate regarding the inclusion of the teacher-directed, contextualised learning items in the inquiry-based instruction scaled index, this study provides evidence that this is an effective practice and should take place in most or all science lessons, and science teacher training and professional development should reflect this. Although there is some evidence for the effectiveness of allowing students to explain their ideas and give opportunities for students to work in the laboratory, the results of this study suggest that these approaches should be used in moderation (in some lessons or in most lessons, respectively). Teacher training related to these strategies should include guidance for teachers regarding the amount of curriculum time to allocate to student explanations and laboratory work, to optimise student learning.
This study also provides evidence that students designing their own experiments, using experiments to test ideas, and drawing conclusions from their experiments are not effective uses of instructional time. However, in most education systems, science teachers are expected to provide such experiences for their students. In order to improve learning gains when using these approaches a greater level of scaffolding may be required for students. This increased level of support (in open-inquiry type activities) can be achieved using a range of methods. For example, effective scaffolds were developed in a study involving the implementation of inquiry-based instruction using the 5Es model (Bybee et al., Citation2006) as part of a lesson study professional development project (Hofer & Lembens, Citation2017). The iterative lesson improvement process (embedded in the lesson study cycle, Cajkler, Wood, Norton, & Pedder, Citation2014) led to the development of ever-more structured approaches as the teachers planned to address observed issues in the previous inquiry-based instruction lessons. Scaffolding strategies included offering a range of pre-made hypotheses outlines for students to choose from; providing templates for tables, diagrams and graphs; and explicit instructions and strategies to support data interpretation and drawing conclusions (Hofer & Lembens, Citation2017). In an inquiry-based instruction teaching professional development programme in the Netherlands, the notion of hard and soft scaffolds was used to support the students in open-inquiries (van Uum, Peeters, & Verhoeff, Citation2019). Hard scaffolds included ‘concrete’ learning aids such as the question machine that provided specific criteria for developing research questions and soft scaffolds involved the teacher guiding students to the appropriate hard scaffold (provided either pro-actively or in response to student difficulties). In addition to conceptual scaffolds, students require explicit teaching and ongoing support when using scientific apparatus, in order to increase the likelihood of generating results (during open-inquiries) that lead to the development of the correct understanding of scientific phenomena (Abrahams & Millar, Citation2008). Professional development in this area should focus on training teachers to develop and deploy suitable and effective scaffolding techniques to support all aspects of the open-inquiry process.
Limitations
The limitations of this study are three-fold. Firstly, the measures used are based on self-reported data. Issues then arise regarding social desirability bias (Holtgraves, Citation2004), adolescents not taking the questionnaire seriously (Fan et al., Citation2006), and the general lack of introspective ability and understanding of the participants. Secondly, the results of the questionnaire only stated the reported frequency of exposure to inquiry-based instruction approaches and provided no information regarding the quality of the teaching and learning experiences. Lastly, the correlational nature of the study means that causality cannot be assumed without collecting data directly from schools and students. For example, evidence gathered from lesson observations, educational policy and curriculum analysis, and interviews with stakeholders could be used to verify the assumptions made from correlational data.
Conclusions
Despite these limitations, the findings of the present study provide frequency-specific empirical evidence regarding the effectiveness of the teaching approaches included in the inquiry-based instruction scaled index in PISA 2015. The teacher-directed items, which included approaches related to contextualising students’ science learning, were consistently related to high science achievement and this suggests that these strategies should be integrated into most, if not all, science lessons. The general use of laboratory work is encouraged, but it should take place in moderation. This allows time for the consolidation of conceptual understanding as students reflect on their concrete experiences in the laboratory and use them to conceptualise abstract scientific knowledge. Strategies that required students to generate their own inquiry research questions, experiments and conclusions were consistently associated with low science achievement (or found to have no significant relationship). These results highlighted the need for well-planned, scaffolded, open-inquiry approaches that directly support students with the underlying processes used to independently generate science knowledge.
By taking this analytic approach, the apparent disparity between experimental and correlational studies, in the field of inquiry-based instruction, has been challenged and, to some extent, reconciled. The findings of this study, in terms of the individual approaches, are largely in line with findings in the experimental literature regarding the effectiveness of inquiry-based instruction. Detailed analyses and suggestions were also presented to explain the remaining differences between the correlational and experimental data and to address the possible practice-based short-comings in inquiry-focused science classrooms.
Finally, including most of the educational regions participating in PISA 2015 in this analysis likely masks some between-country differences due to variation in social and economic characteristics. In future studies, this ‘disaggregation’ approach should be carried out for the context of interest to more accurately inform policy decisions for a specific country or region.
Acknowledgments
I would like to sincerely thank Dr Shaljan Areepattamannil for his patience.
Disclosure statement
No potential conflict of interest was reported by the author.
ORCID
Dean Cairns http://orcid.org/0000-0002-5262-868X
References
- Abrahams, I., & Millar, R. (2008). Does practical work really work? A study of the effectiveness of practical work as a teaching and learning method in school science. International Journal of Science Education, 30(14), 1945–1969.
- Aditomo, A., & Klieme, E. (2019). Forms of inquiry-based science instruction and their relations with learning outcomes: Evidence from high and low-performing education systems. doi: 10.31235/osf.io/aqsbj
- Anderson, R. D. (2002). Reforming science teaching: What research says about inquiry. Journal of Science Teacher Education, 13(1), 1–12.
- Areepattamannil, S. (2012). Effects of inquiry-based science instruction on science achievement and interest in science: Evidence from Qatar. The Journal of Educational Research, 105(2), 134–146.
- Areepattamannil, S., Freeman, J., & Klinger, D. (2011). Influence of motivation, self-beliefs, and instructional practices on science achievement of adolescents in Canada. Social Psychology of Education, 14(2), 233–259.
- Barman, C. (2002). How do you define inquiry. Science and Children, 26, 8–9.
- Bell, R. L., Smetana, L., & Binns, I. (2005). Simplifying inquiry instruction. The Science Teacher, 72(7), 30–33.
- Borman, G. D., Gamoran, A., & Bowdon, J. (2008). A randomized trial of teacher development in elementary science: First-year achievement effects. Journal of Research on Educational Effectiveness, 1(4), 237–264.
- Bredderman, T. (1983). Effects of activity-based elementary science on student outcomes: A quantitative synthesis. Review of Educational Research, 53(4), 499–518.
- Bybee, R. W., Taylor, J. A., Gardner, A., Van Scotter, P., Powell, J. C., Westbrook, A., & Landes, N. (2006). The BSCS 5E instructional model: Origins and effectiveness. Colorado Springs, Co: BSCS, 5, 88–98.
- Cairns, D., & Areepattamannil, S. (2019). Exploring the relations of inquiry-based teaching to science achievement and dispositions in 54 countries. Research in Science Education, 49(1), 1–23.
- Cajkler, W., Wood, P., Norton, J., & Pedder, D. (2014). Lesson study as a vehicle for collaborative teacher learning in a secondary school. Professional Development in Education, 40(4), 511–529.
- Caro, D. H., Lenkeit, J., & Kyriakides, L. (2016). Teaching strategies and differential effectiveness across learning contexts: Evidence from PISA 2012. Studies in Educational Evaluation, 49, 30–41.
- Chinn, C. A., & Malhotra, B. A. (2002). Epistemologically authentic inquiry in schools: A theoretical framework for evaluating inquiry tasks. Science Education, 86(2), 175–218.
- Colburn, A. (2000). An inquiry primer. Science Scope, 23(6), 42–44.
- Constantinou, C. P., Tsivitanidou, O. E., & Rybska, E. (2018). What is inquiry-based science teaching and learning? In O. E. Tsivitanidou, P. Gray, E. Rybska, L. Louca, & C. Constantinou (Eds.), Professional development for inquiry-based science teaching and learning (pp. 1–23). Cham: Springer International Publishing.
- Denoël, E., Dorn, E., Goodman, A., Hiltunen, J., Krawitz, M., & Mourshed, M. (2018). Drivers of student performance: Insights from Europe. Retrieved from https://www.mckinsey.com/industries/social-sector/our-insights/drivers-of-student-performance-insights-from-europe
- Enders, C. K. (2010). Applied missing data analysis. New York, NY: Guilford Press.
- Fan, X., Miller, B. C., Park, K.-E., Winward, B. W., Christensen, M., Grotevant, H. D., & Tai, R. H. (2006). An exploratory study about inaccuracy and invalidity in adolescent self-report surveys. Field Methods, 18(3), 223–244.
- Fensham, P. J. (2009). Real world contexts in PISA science: Implications for context-based science education. Journal of Research in Science Teaching: The Official Journal of the National Association for Research in Science Teaching, 46(8), 884–896.
- Fitzgerald, M., Danaia, L., & McKinnon, D. H. (2019). Barriers inhibiting inquiry-based science teaching and potential solutions: Perceptions of positively inclined early adopters. Research in Science Education, 49(2), 543–566.
- Friesen, S., & Jardine, D. (2009). 21st century learners. Calgary, AB: Galileo Educational Network.
- Furtak, E. M., Seidel, T., Iverson, H., & Briggs, D. C. (2012). Experimental and quasi-experimental studies of inquiry-based science teaching a meta-analysis. Review of Educational Research, 82(3), 300–329.
- Geier, R., Blumenfeld, P. C., Marx, R. W., Krajcik, J. S., Fishman, B., Soloway, E., & Clay-Chambers, J. (2008). Standardized test outcomes for students engaged in inquiry-based science curricula in the context of urban reform. Journal of Research in Science Teaching, 45(8), 922–939.
- Gunstone, R. F. (1991). Reconstructing theory from practical experience. In B. Woolnough (Ed.), Practical Science (pp. 67–77). Milton Keynes, UK: Open University Press.
- Hattie, J. (2009). Visible learning. London: Routledge.
- Heck, R. H., & Thomas, S. L. (2015). An introduction to multilevel modeling techniques: MLM and SEM approaches using Mplus (3rd ed.). New York, NY: Routledge.
- Hmelo-Silver, C. E., Duncan, R. G., & Chinn, C. A. (2007). Scaffolding and achievement in problem-based and inquiry learning: A response to Kirschner, Sweller, and Clark (2006). Educational Psychologist, 42(2), 99–107.
- Hofer, E., & Lembens, A. (2017). Implementing aspects of inquiry-based learning in secondary chemistry classes: A case study. Paper presented at the ESERA 2017 Conference. Research, Practice and Collaboration in Science Education, Dublin: Dublin City University.
- Hofstein, A., & Lunetta, V. N. (2004). The laboratory in science education: Foundations for the twenty-first century. Science Education, 88(1), 28–54.
- Holtgraves, T. (2004). Social desirability and self-reports: Testing models of socially desirable responding. Personality and Social Psychology Bulletin, 30(2), 161–172.
- Hutcheson, G. D., & Sofroniou, N. (1999). The multivariate social scientist: Introductory statistics using generalized linear models (1st ed.). Thousand Oaks, CA: SAGE.
- Jerrim, J., Oliver, M., & Sims, S. (2019). The relationship between inquiry-based teaching and students’ achievement. New evidence from a longitudinal PISA study in England. Learning and Instruction, 61, 35–44.
- Jiang, F., & McComas, W. F. (2015). The effects of inquiry teaching on student science achievement and attitudes: Evidence from propensity score analysis of PISA data. International Journal of Science Education, 37(3), 554–576.
- Kirschner, P. A., Sweller, J., & Clark, R. E. (2006). Why minimal guidance during instruction does not work: An analysis of the Failure of constructivist, discovery, problem-based, experiential, and inquiry-based teaching. Educational Psychologist, 41(2), 75–86.
- Klahr, D., & Nigam, M. (2004). The equivalence of learning paths in early science instruction: Effects of direct instruction and discovery learning. Psychological Science, 15(10), 661–667.
- Lau, K.-c., & Lam, T. Y.-p. (2017). Instructional practices and science performance of 10 top-performing regions in PISA 2015. International Journal of Science Education, 39(15), 2128–2149.
- Lavonen, J., & Laaksonen, S. (2009). Context of teaching and learning school science in Finland: Reflections on PISA 2006 results. Journal of Research in Science Teaching, 46(8), 922–944.
- Loucks-Horsley, S., & Olson, S. (2000). Inquiry and the national science education standards: A guide for teaching and learning. Washington, DC: National Academies Press.
- Manoli, C., Pedaste, M., Mäeots, M., Siiman, L., De Jong, T., Van Riesen, S. A., … Tsourlidaki, E. (2015). Phases of inquiry-based learning: definitions and the inquiry cycle. Educational Research Review, 14, 47–61.
- McCoach, D. B. (2010). Hierarchical linear modeling. In G. R. Hancock & R. O. Mueller (Eds.), The reviewer’s guide to quantitative methods in the social sciences (pp. 123–140). New York, NY: Routledge.
- McNeill, K. L., & Krajcik, J. (2008). Scientific explanations: Characterizing and evaluating the effects of teachers’ instructional practices on student learning. Journal of Research in Science Teaching: The Official Journal of the National Association for Research in Science Teaching, 45(1), 53–78.
- Minner, D. D., Levy, A. J., & Century, J. (2010). Inquiry-based science instruction-what is it and does it matter? Results from a research synthesis years 1984 to 2002. Journal of Research in Science Teaching, 47(4), 474–496.
- National Research Council. (1996). National science education standards. Washington, DC: Author.
- OECD. (2009). PISA 2006 Technical Report, PISA. Paris: OECD Publishing. doi:10.1787/9789264048096-en
- OECD. (2016a). PISA 2015 results (volume I): Policies and practices for successful schools: PISA. Paris: OECD Publishing.
- OECD. (2016b). PISA 2015 results (volume II): Policies and practices for successful schools: PISA. Paris: OECD Publishing.
- OECD. (2017a). PISA 2015 Assessment and Analytical Framework: Science, Reading, Mathematic, Financial Literacy and Collaborative Problem Solving, PISA. Paris: OECD Publishing. doi: 10.1787/9789264281820-en
- OECD. (2017b). PISA 2015 technical report. Retrieved from http://www.oecd.org/pisa/data/2015-technical-report/
- Osborne, J. (2012). The role of argument: Learning how to learn in school science. In B. J. Fraser, K. Tobin, & C. McRobbie (Eds.), Second international handbook of science education (Vol. 24, pp. 933–949). Dordrecht: Springer.
- Prince, M. J., & Felder, R. M. (2006). Inductive teaching and learning methods: Definitions, comparisons, and research bases. Journal of Engineering Education, 95(2), 123–138.
- Raudenbush, S. W., & Bryk, A. S. (2002). Hierarchical linear models: Applications and data analysis methods (2nd). Thousand Oaks, CA: Sage.
- Raudenbush, S. W., Bryk, A. S., Cheong, Y. F. Jr., Congdon, R. T., & Toit, M. d. (2011). HLM7 hierarchical linear and nonlinear modeling user manual: User guide for scientific software international’s (S.S.I.) program. Stokie, IL: Scientific Software International.
- Sandoval, W. A., & Reiser, B. J. (2004). Explanation-driven inquiry: Integrating conceptual and epistemic scaffolds for scientific inquiry. Science Education, 88(3), 345–372.
- Schwab, J. J. (1962). The teaching of science as enquiry. In J. J. Schwab & P. F. Brandwein (Eds.), The teaching of science (pp. 1–103). Cambridge, MA: Harvard University Press.
- Scott, D. M., Smith, C. W., Chu, M.-W., & Friesen, S. (2018). Examining the efficacy of inquiry-based approaches to education. Alberta Journal of Educational Research, 64(1), 35–54.
- Shymansky, J. A., Kyle, W. C., & Alport, J. M. (1983). The effects of new science curricula on student performance. Journal of Research in Science Teaching, 20(5), 387–404.
- Spronken-Smith, R. (2008). Experiencing the process of knowledge creation: The nature and use of inquiry-based learning in higher education. Dunedin, NZ: University of Otago.
- Sweller, J., Kirschner, P. A., & Clark, R. E. (2007). Why minimally guided teaching techniques do not work: A reply to commentaries. Educational Psychologist, 42(2), 115–121.
- Tabachnick, B. G., & Fidell, L. S. (2014). Using multivariate statistics (6th ed.). Essex: Pearson Education.
- Taylor, J., Stuhlsatz, M., & Bybee, R. (2009). Windows into high-achieving science classrooms. In R. W. Bybee & B. McCrae (Eds.), PISA science 2006: Implications for science teachers and teaching (pp. 123–132). Arlington, VA: NSTA Press.
- Teig, N., Scherer, R., & Nilsen, T. (2018). More isn’t always better: The curvilinear relationship between inquiry-based teaching and student achievement in science. Learning and Instruction, 56, 20–29.
- van Uum, M. S., Peeters, M., & Verhoeff, R. P. (2019). Professionalising primary school teachers in guiding inquiry-based learning. Research in Science Education, 1–28. doi: 10.1007/s11165-019-9818-z
- Weinstein, T., Boulanger, F. D., & Walberg, H. J. (1982). Science curriculum effects in high school: A quantitative synthesis. Journal of Research in Science Teaching, 19(6), 511–522.
- Whitelegg, E., & Parry, M. (1999). Real-life contexts for learning physics: Meanings, issues and practice. Physics Education, 34(2), 68–72.
- Wieman, C. E., & Perkins, K. K. (2006). A powerful tool for teaching science. Nature Physics, 2(5), 290–292.
- Wise, K. C., & Okey, J. R. (1983). A meta-analysis of the effects of various science teaching strategies on achievement. Journal of Research in Science Teaching, 20(5), 419–435.
Appendices
Appendix A
The PISA 2015 inquiry-based instruction scaled index items.
ST098Q01TA – Students are given opportunities to explain their ideas.
ST098Q02TA – Students spend time in the laboratory doing practical experiments.
ST098Q03NA – Students are required to argue about science questions.
ST098Q05TA – Students are asked to draw conclusions from an experiment they have conducted.
ST098Q06TA – The teacher explains how a science idea can be applied.
ST098Q07TA – Students are allowed to design their own experiments.
ST098Q08TA – There is a class debate about investigations.
ST098Q09TA – The teacher clearly explains the relevance of science concepts to our lives.
ST098Q10NA – Students are asked to do an investigation to test ideas.
Appendix B
Table B1. List of countries/economies included in the study.
The relationship between the inquiry-based instruction scaled index (IBTEACH) to science achievement, by country.