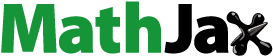
Abstract
Food cooperatives are gaining popularity due to consumers’ desire to eat healthy and source locally. Mainly run by private citizens, such groups present an interesting additional income source to regional organic farmers. However, small order quantities and substantial logistics efforts challenge operations. To facilitate efficient and sustainable food transports from farms to food cooperatives, this work investigates impacts of collaborative logistics activities through the development of a simulation and optimization-based decision support system. Results of computational experiments considering fresh food transports in Austria highlight potentials of such joint activities. Particularly, if orders are infrequent and quantities small, collaboration results in a substantial reduction of travel distances and reduces the number of required vehicles. Nevertheless, delivered food quality may deteriorate as consolidating shipments results in longer travel durations as well as additional loading and unloading activities.
1. Background and motivation
In Austria, food cooperatives are gaining popularity, representing an interesting alternative distribution channel for small regional organic farmers. Further referred to as voluntary food co‐operatives (Freathy and Hare Citation2004) or food-buying groups (Little, Maye, and Ilbery Citation2010), such organizations are mainly self-organized by end consumers and often fuelled by the desire to source locally and eat fresh and organic produces (IG-FoodCoops Citation2018). They are characterized by a face-to-face contact between customers and farmers and offer seasonable, regional as well as organic food. Closely related to other Alternative Food Networks (AFNs) such as farmer’s markets or community supported agriculture, food cooperatives offer consumers an alternative to large scale, industrial food systems (Jarosz Citation2008).
Nevertheless, food cooperatives still play a subordinate role for Austrian farmers as only 1% of all farmers who sell products directly trade with food cooperatives (KeyQUEST Citation2016). Particularly, substantial additional logistics efforts are required to serve such groups as orders are of small quantities and occur infrequently. Consequently, logistics and deliveries were identified as the main challenge of farmers supplying food cooperatives within Austria (Jaklin, Kummer, and Milestad Citation2015).
One potential way to support related logistics operations is through horizontal cooperation, i.e. the joint organization of delivery activities of multiple competing farmers serving various independent food cooperatives. To achieve successful cooperation, trust is a major success factor that needs to be built over time by the cooperating actors (Serrano-Hernandez et al. Citation2018). Computer-aided decision support systems (DSS) facilitate such activities as they allow one to estimate impacts of cooperation and visualize results in a risk-free and flexible manner (Fikar, Hirsch, and Gronalt Citation2018). Additionally, various order picking and delivery strategies can be investigated to support sustainable implementations and a reduction in food waste and travel distances (Fikar Citation2018).
plots the considered collaborative distribution strategies investigated within this work. To visualize, an example with three food cooperatives and three farms is considered. Food cooperative A orders from farm 1, food cooperative B from farms 1 and 2 and food cooperative C from farms 2 and 3. Arrows indicate driving distances. Strategies where food cooperatives collect orders from farms are compared with ones where farmers or third-party logistics providers deliver items. Both collaborative settings, i.e. where food cooperatives or farmers share transport resources, and non-collaborative ones are investigated, focussing on the impact on travel distances, required number of vehicles and resulting losses in food quality due to suboptimal cooling conditions and additional loading activities. For instance, if food cooperatives collaborate to jointly collect products from farms, both total travel distances and the number of required cars can be reduced if multiple orders of various food cooperatives are picked up by a single vehicle. Product quality, however, may decrease as products are transported longer due to additional stops and detours.
The contribution of this work is twofold: (i) a simulation and optimization-based DSS to model coordinated supply of food cooperatives is introduced; and (ii) managerial implications of joint distribution strategies derived from a computational study are highlighted to facilitate future implementations.
The remainder of the paper is structured as follows. The subsequent Section 2 lists related work. Section 3 describes the studied problem setting in detail. The developed DSS is presented in Section 4 and performed computational experiments on the supply of food cooperatives in Austria are introduced in Section 5. Section 6 reports results of the computational study and discusses managerial implications. Concluding remarks are given in Section 7.
2. Related work
Various related works focus on AFNs and investigate collaboration within such networks. Two types of food cooperatives are distinguished: (i) agricultural food cooperatives, where farmers cooperate for joint production or to share infrastructure; and (ii) consumer-driven food cooperatives such as food-buying groups, where end consumers jointly order food products.
An analysis of the relation between cooperative associations and AFNs in Italy is performed in Fonte and Cucco (Citation2017). The authors study the history of Italian cooperative movements focussing on agricultural ones and point out that such cooperatives should closer collaborate with AFNs in order to achieve sustainable food systems. Vlajic, Mijailovic, and Bogdanova (Citation2018), who investigate value recovery strategies in fresh fruit supply chains, further highlight the importance of alternative customers. Particularly, surplus inventory and remanufactured products often flow to such markets to generate additional income. To successfully participate in AFNs, Brunori, Rossi, and Malandrin (Citation2011) state that it requires appropriate farming styles and that direct interaction with consumers as well as cooperation and coordination with other farmers is crucial. The importance of supply chain collaboration is further noted in Dreyer et al. (Citation2016). Through an in-deep investigation of speciality food produced in Norway, the authors highlight, among others, the importance of developing joint distribution agreements to mitigate scale burdens. With a special focus on logistics and regional food supply chains, Mittal, Krejci, and Craven (Citation2018) review the existing literature to identify best practices. The authors conclude that high costs associated with distributing food from many small-scale producers to consumers are a major barrier to long-term success of such systems. Strengths and weaknesses associated with the implementation of local food logistics services in Italy are analysed in Paciarotti and Torregiani (Citation2018). Focussing on local deliveries to restaurants, the authors state that interaction between actors is hindered by poor communication and unstructured processes. Nevertheless, producers as well as restaurant operators are interested in cooperatives to improve local food logistics. Such interest is further indicated in Jaklin, Kummer, and Milestad (Citation2015), who interviewed 14 farmers supplying a food cooperative in Vienna, Austria. The farmers point out that the logistics of food cooperatives should be managed more efficiently and adapted to the farmers’ needs, particularly concerning pickups and requested delivery time windows. Additionally, limited transportation resources are noted as a main challenge. As most food cooperative members live in cities and may not own a car, difficulties arise when large amounts of products need to be picked up.
To facilitate cooperation and optimize regional food delivery processes, various authors use simulation and optimization techniques. Mittal and Krejci (Citation2015) study scheduling of deliveries to food hubs. Using a hybrid agent-based and discrete event simulation, the authors analyze the scheduling behaviour of farmers and the implementation of incentives to motivate farmers to schedule their routes in advance. By integrating food quality data in a discrete event simulation, Leithner and Fikar (Citation2020) analyze a regional and organic fruit supply chain in order to improve supply chain performance. The consideration of shelf life data in supply chain decisions enables one to reduce food quality losses and improve product availability. Tavella and Papadopoulos (Citation2017) use soft operation research methods to address various communication and coordination problems in a Danish consumer-driven food cooperative to alleviated ill-structured problem situations and facilitate coordination. Among others, the purchasing and logistics groups within the food cooperative are considered. High distribution costs, uncertainty in demand and delivery times as well as a lack of coordination between the individual actors are, among others, identified as major challenges within daily operations.
Consequently, while various works focus on food cooperatives and identify key challenges within daily logistics activities, limited work has been performed on providing decision support to facilitate collaborative distribution strategies and to quantify impacts. Given the various specific characteristics and features of related logistics operations, tailored solutions are required to support successful implementations in the future.
3. Problem description
The problem setting studied in this work considers a single day of operation. Within the study region, various food cooperatives order fresh food from local farmers. Due to different food characteristics, an order within the DSS consists of a single food type only, however, multiple orders are placed by food cooperatives on a given day. Each order needs to be fulfilled within a specified delivery time window, i.e. the opening times of the food cooperatives. For distribution, multiple vehicles are available. If collection by food cooperatives is considered, private cars are considered with no cooling options. In contrast, both farmers and the considered logistics provider use refrigerated vans or refrigerated trucks, respectively. Vehicle capacities of such trucks and vans are larger than the ones of private vehicles.
Food products are stored in cold stores at farms with each farmer offering one or more food types. It is assumed that all products ordered by the food cooperatives are available at the respective farmer, i.e. no stock-outs occur. The specific storage and transport temperatures along the delivery processes influence food quality losses over time. Additionally, food quality is affected by loading and unloading activities. As noted in James, James, and Evans (Citation2006), this impacts all products on board of a vehicle due to heat and mass transfer, particularly in road transportation where products can be subject to as many as 50 door openings before final delivery.
A simplified visualization of the modelled business process is provided in . Food cooperatives place orders from multiple farmers for various products, which subsequently need to be shipped. Depending on the investigated problem setting, products are either collected by food cooperatives, delivered by farmers or shipped through a third-party logistics provider. At final arrival at the food cooperatives, product quality is checked and items are stored for distribution to the members. The objective is to minimize travel distances throughout delivery processes to reduce costs.
Based on common challenges within the supply of food cooperatives described in Tavella and Papadopoulos (Citation2017) and Jaklin, Kummer, and Milestad (Citation2015), uncertainties are considered during the day of operations to evaluate the quality of the logistics solutions. Due to sudden demand, dynamic orders may occur at any time, which need to be fulfilled on the same day. Additionally, due to limited capacities at organic farmers and food cooperatives, it is assumed that only a single vehicle can be served at any given time. Consequently, if multiple vehicles are present at a location at the same time, the vehicle, which arrived the earliest, is served first. Once loading or unloading processes of this vehicle are finished, the next vehicle is served, resulting in additional delays and potential negative impact on food quality.
4. Method
The developed DSS combines simulation techniques to estimate demand and food quality losses with a metaheuristic optimization procedure to generate delivery routes. An overview of the DSS is given in . To initiate the system, the user defines the study area (i.e. locations of farmers and food cooperatives), specifies food quality characteristics and sets various parameters stating expected demand, available delivery vehicles, temperature ranges and considered time windows. This data is loaded into the DSS, which generates demand, plans vehicle routes and performs deliveries. Throughout the day, plans are adjusted in case of sudden demand. Various key performance indicators such as travel distances, delivery food quality and the number of used vehicles are recorded. Results are visualized to the user, who can adjust the problem setting and parameters for subsequent runs to derive managerial implications and facilitate understandings of key influencing factors. The individual components are introduced in detail in the subsequent subsections.
4.1. Generating demand
At the start of each simulation run, demand is randomly generated. Based on user-defined parameters, food cooperatives place orders. Each order is assigned to a farmer and defined by a load factor measured in roll containers to consider limited storage capacities within the various vehicle classes. Due to the specific focus of food cooperative to source products locally, farmers in close proximity of food cooperatives are favoured. Therefore, all farmers offering the requested food product are sorted in ascending order based on the travel duration from food cooperative to farm. The specific location to order from is subsequently selected based on a geometric distribution with parameter where a higher p value favours top ranked candidates. provides an example for this ordering procedure.
4.2. Modelling of delivery and storage processes
Once all orders are generated, a routeing procedure is started. The problem is modelled as a pickup and delivery problem (Parragh, Doerner, and Hartl Citation2008), i.e. each order consists of a pickup request and a separate delivery one, to enable the consideration of both collaborative and non-collaborative settings. Due to the focus of the DSS to generate solutions swiftly in dynamic settings, an adaptive large neighbourhood search (ALNS) based on Ropke and Pisinger (Citation2006) was implemented. ALNS is a metaheuristic that repeatedly partially destroys and repairs solutions in order to find improvements. Therefore, multiple removal and insertion heuristics are considered. To visualize, a simplified example of an ALNS iteration is given in . A setting where farms collaboratively supply food cooperatives is considered. Food cooperative A again orders from farm 1, food cooperative B from farm 1 and 2 and food cooperative C from farms 2 and 3. Each iteration starts with a current solution. A removal heuristic is selected, removing multiple stops from the vehicle tours. These missing stops are subsequently reinserted at the most promising positions. The altered solution is evaluated and kept if an improvement in the objective value was found. Additionally, keeping worse solutions is allowed in some cases based on an acceptance criterion to diversify the search. To further guide the search, removal and insertion heuristics which performed well are favoured in subsequent iterations. The procedure stops once a given time limit is reached and returns the best found solution.
This procedure is, depending on the investigated collaboration setting, either run independently for each farmer or food cooperative, or jointly for all orders within the collaborative settings. Consequently, if a non-collaborative setting is considered, the ALNS is run multiple times for each actor independently, while in collaborative settings, a single run with all orders is performed. Furthermore, the procedure is rerun each time dynamic orders occur. In such settings, the currently scheduled vehicle routes act as a starting solution for the procedure. A high-level description of the implemented routeing algorithm is shown in .
To construct an initial solution, a best insertion heuristics is run entering all requests sequentially at their best position. Therefore, the orders to schedule are sorted by their respective time window starting with the earliest one. This solution is subsequently improved by the ALNS. The implemented version employs four removal operators, namely a worst removal, random removal and two removals grouping requests either by their end location or time window. All requests are sorted based on the selected removal operator. A random number of requests is subsequently selected based on a geometric distribution and removed from the current vehicle routes. To reinsert these requests, a best insertion strategy is employed entering requests either in order of their time windows, randomly or based on the respective demand location. To enable diversification, a random noise value is further added to each evaluation within the insertion heuristic. Once all removed requests are reinserted, the objective value is calculated and compared to the current one. If better, the solution is accepted and acts as a basis for the next iteration. Otherwise, an acceptance criteria based on simulated annealing is implemented, deciding if a solution is kept or not. Furthermore, statistics are updated highlighting promising removal and insertion operators as well as how many requests should be removed to achieved improvements. Such values are favoured in subsequent iterations.
After a user-defined time limit or after a certain number of unsuccessful iterations is reached, i.e. iterations without a global improvement, the best solution of the ALNS is returned. This solution is further improved by a local search evaluating benefits of relocating each shipment. If an improvement is found, the solution is saved and this procedure is repeated until no further improvements are found. At this point, vehicle routes are performed within the DSS to estimate food quality losses.
4.3. Estimating food quality throughout storage and transportation
The DSS includes 11 fresh vegetables sold by farmers in Austria during the autumn season. The quality of such products throughout storage and transportation is estimated based on food quality models developed by Hertog and Tijskens (Citation1998). The deterioration of fresh fruits and vegetables is mainly affected by temperature conditions and the time the products are exposed to these temperatures. Consequently, during a simulation run, the total time each food product spends in transit and during loading operations is recorded. At final delivery of the item, these durations are evaluated with the respective storage temperatures to estimate quality losses. Therefore, Arrhenius’ law, indicated in EquationEquation (1)(1)
(1) , constitutes the dependence between spoilage behaviour and temperature (Laidler Citation1984). If a product is stored at a high temperature or for a long duration, quality losses increase.
(1)
(1)
displays the spoilage rate constant at reference temperature
(10 °C/283.15 K),
the energy of activation (J/mol),
the gas constant (8.314 J/mol/K) and
the absolute temperature.
EquationEquation (2)(2)
(2) represents the remaining keeping quality of a product at a certain temperature after this product was stored for
time spans in dynamically changing temperatures affecting the rate constants
(2)
(2)
represents the initial keeping quality at reference temperature. To calculate the remaining keeping quality
the rate constant
at a certain temperature is used. Tijskens and Polderdijk (Citation1996) list spoilage parameters for over 50 fresh fruits and vegetables, including parameters for all products considered in this work.
5. Computational experiments
To compare different distribution strategies, organic vegetables farmers and food cooperatives located in Upper Austria, Lower Austria and Vienna are considered within the presented computational experiments. In total, 60 food cooperatives are considered as reported online by IG-FoodCoops (Citation2018), the interest group of food cooperatives in Austria. Organic farmers within the study region are based on publicly available data from BioAustria (BioAustria Citation2018), the Association of Austrian organic farmers. Fifty-nine farms are considered, which sell fresh vegetables harvested in autumn. These considered produces are cabbage, carrots, cauliflower, celeriac, gherkin, kale, kohlrabi, leek, lettuce, onions and radishes. One farm can offer either one or multiple of these products to food cooperatives. Based on a major food logistics company providing less-than-truckload shipping, the third party logistics provider within the computational study operates two vehicle depots, placed in close proximity to Vienna and Linz, the two major cities in the study region.
An overview of the considered problem setting is given in . While food cooperatives are mainly located within urban regions, farms are distributed throughout the study region. To consider real world street networks, openly available OpenStreetMap data (OpenStreetMap Citation2018) are integrated in the DSS. Distances and durations are calculated with the open source routeing library GraphHopper (Citation2018).
5.1. Parameter setting
The DSS was developed with AnyLogic 8.3.2 (AnyLogic Citation2018) with all optimization procedures coded in Java. Findings are based on the average results of 100 simulation replications for each problem setting.
Parameters for the ALNS are based on Ropke and Pisinger (Citation2006) and performed parameter tests. A maximum of 15% of the current solution is destroyed at each insertion and a noise value of 20% is added to each evaluation for diversification. To select which stops are removed, the geometric distribution is run with p = 0.05. The starting temperature is set to allow a 35% decrease in objective value within the first iteration with a 50% probability. Subsequent temperatures are multiplied by 0.99 at each iteration to represent the cooling scheme. The optimization procedure stops after either 60 s or once no improvement was found for 4n iterations, where n indicates the number of newly added shipment requests at the given point in time.
5.2. Test setting
In the base scenario, 180 orders are considered, i.e. an average three orders per food cooperative. Each order is placed by a uniformly randomly selected food cooperative. It is further associated with a random food type and requires between 0.1 and 0.5 roll containers to be transported. The local ordering bias is set to 20%, indicating that the closest farm that offers this product type is selected with a 20% probability. If unsuccessful, the next closest farm is tested with the given probability until a draw is successful. Delivery operations start at 8 a.m. and all delivery time windows of food cooperatives are set to 4 h and uniformly distributed to start at any full hour between 9 a.m. and 4 p.m. The likelihood of an additional dynamic order of a food cooperative is set to 10% and such orders may occur at a uniformly random time prior to the time window of the food cooperative.
Until pickup, food items are stored at cold stores. Food quality losses are recorded from the moment the product gets loaded onto a vehicle. Concerning the available vehicle fleet, one car that can transport a maximum of one roll container at any given time is placed at each food cooperative. Due to a lack of cooling equipment, transport temperature is set to 295.15 K, i.e. 22 °C or 71.6 °F. The same temperature is set for all loading and unloading operations, affecting all products currently loaded on the vehicle. For each farm, a single van with a transport temperature of 277.15 K, i.e., 4 °C or 39.2 °F, and a capacity of five roll containers is available. The logistics provider is assumed to have an unlimited number of trucks with transport temperatures of 277.15 K available, each able to carry a maximum of 11 roll containers. Travel speed is based on speed limits of the individual streets segments, independent of the vehicle type.
5.3. Limitations
As food cooperatives differ substantially depending on the geographic and regulative settings, deriving general implications is challenging. Within the computational study, only organic farmers and food cooperatives who agreed to publish their data online are considered. The focus is set on fresh produces, i.e. goods stored at ambient temperatures such as flour or bread are not included. Additionally, it is assumed that food cooperatives drive privately with their cars to pick up goods from local farmers. To act more sustainable, the food cooperatives handbook in Austria (Dax Citation2017) recommends to combine trips with other activities such as regular commutes to work of members or to use public transport. Due to lack of data, such potential synergies are not considered in this study. Additionally, the estimation of food quality losses during transports and loading is simplified in this study by focussing on temperature impacts. Specific heat and mass transfers during transport are additionally subject to a wide range of influencing factors such as vehicles configurations, cooling equipment, packaging and loading layouts. For detailed information on this topic, the reader is referred to James, James, and Evans (Citation2006).
6. Results and discussion
To derive beneficial settings for collaboration, individual parameters of the base scenario are adjusted. gives the notation used in the subsequent presentation of results. Detailed numeric results of all runs and each simulation replication are available online at http://short.boku.ac.at/instances for further investigations.
Table 1. Notation of the investigated logistics concepts with the results.
6.1. Impact of the number of orders
Few and infrequent orders are a major challenge for farmers supplying food cooperatives (Jaklin, Kummer, and Milestad Citation2015). To investigate this impact, plots results with the total number of orders varied on a given day between 60 and 300.
Comparing collaborative settings (i.e. Coop-HC, Farm-HC and 3PL) with noncollaborative ones (i.e. Coop and Farm) shows that shared logistics enables one in all cases to substantially decrease travel distances and the number of vehicles used. Food quality losses during transport, however, increase due to additional loading activities. Concerning the number of orders, a higher amount results in a reduction in the average travel distance per order for all logistics concepts as multiple shipments can be consolidated in a single vehicle tour. This further leads to additional food quality losses due to additional loading and unloading activities. Only in the setting where multiple food cooperatives collaborate, this negative impact is offset by shorter travel durations if the number of orders increases.
6.2. Impact of the size of orders
In addition to the amount of orders, the size of individual orders is of importance to evaluate the individual distribution strategies. varies the maximum size of a single order between 0.1 and 1.0 roll containers. The minimum order size is set to 0.1 roll containers in all settings.
Particularly for the setting where food cooperatives collaborate, such an increase in order quantity leads to a major increase in travel distances and number of vehicles used as fewer orders can be consolidated within a single vehicle tour. Larger vehicle capacities at farms and of the logistics provider result in more robust solutions for all other settings. Consequently, if order quantities are large, it is beneficial to employ a logistics provider or facilitate cooperation among farmers.
6.3. Impact of sourcing locally
Food cooperatives often prefer to order products from farmers located closely to them (IG-FoodCoops Citation2018). shows results of adjusting this assumption by varying the modelled local buying bias. If 0, food cooperatives order at a uniformly randomly selected farmer independent of the travel distance, while with a value of 1, the closest one is always selected, i.e. a high focus on local produces is placed.
As is implied by the number of vehicles used, if the local bias is high, orders are placed at a subset of farmers resulting in a reduction of vehicles used in the non-collaborative delivery setting. Such farms are mostly located in the proximity of main cities and, consequently, experience a high amount of demand resulting in additional loading delays if multiple food cooperatives are simultaneously picking up orders. This negatively impacts food quality. Concerning the average travel distance of an order, horizontal cooperation or employing a third party logistics provider is particularly beneficial if orders are distributed equally among the farmers.
6.4. Impact of dynamic orders
Uncertainty and complexity in demand is a further major challenge for food cooperatives (Tavella and Papadopoulos Citation2017). To investigate such impacts on the individual delivery concepts, the likelihood that an additional sudden order occurs for each food cooperative during the day of operations was varied between 0 and 1 in increments of 0.05. Results, shown in , only indicate minor impacts of such uncertainty on average travel distances and food quality losses per order. Some impacts are seen concerning the number of vehicles used, particularly for the collaborative settings were currently idle vehicles are facilitated to fulfill such sudden requests.
6.5. Impact of number of collaborating partners
The settings so far assumed that all famers or all food cooperatives collaborate. While beneficial, it is difficult to achieve in practice due to limited information sharing or different organizational cultures and objectives. To closer study this point, the number of collaborative actors was varied from noncollaborative settings to full collaborative ones. In each step, one additional actor joins the collaboration. The actors that collaborate were selected randomly in the first experiments and clustered in the second one. For this clustering, the same procedure employed for the local ordering process was used, with parameter p set to 0.2 and starting from a randomly selected actor. Additionally, due to the vast amount of potential collaborative settings, the number of replication was increased to 1.000 for these experiments. plots the achieved savings of the various collaboration sizes compared to a non-collaborative setting.
Starting at a minimum size of four collaborating partners, major benefits in terms of travel distance and vehicles used can be achieved. Such benefits are the largest if multiple farmers in close proximity to each other jointly distribute their products to food cooperatives. Increasing the number of collaborating partners is further beneficial if products are jointly collected by food cooperatives, however, results indicate that it is less important in such settings that the collaborating actors are located in close proximity to one another.
6.6. Managerial implications
The performed computational experiments highlight various benefits and drawbacks of collaborative pickups or deliveries. Additionally, promising settings where the individual collaborative logistics concepts can be employed are identified. These key findings are summarised below.
6.6.1. Trade-off between travel distance and food quality
Facilitating collaboration is highly beneficial in all settings to reduce travel distances and lower the number of vehicles used. Food quality losses, however, are increased due to detours and additional loading activities, requiring special attention when implementing such logistics concepts. If food quality is of focus, farmers are advised to directly deliver their products to food cooperatives. This further provides additional options to react efficiently to dynamic orders throughout the day.
6.6.2. Small-scale settings favour collaboration among food cooperatives
Due to an assumed lack of cooling equipment and smaller vehicle capacities, pickups by food cooperatives perform worse in nearly all considered settings compared to delivery concepts. Nevertheless, particularly in settings common in current real-world operations, i.e. if few orders are placed, order sizes are small and a local bias is present, facilitating collaboration among food cooperatives leads to substantial benefits compared to non-collaborative settings. Consequently, joint collections of multiple food cooperatives can act as a promising initial step to set-up sustainable operations.
6.6.3. Large-scale settings favour collaboration among farmers
With an increased amount of orders, larger order sizes and a lower local bias, benefits of employing collaborative logistics among farmers increase. Outsourcing deliveries to a third-party logistics provider performs similar in such cases, however, one has to consider additional trips required to leave and return to the provider’s vehicle depots. Therefore, providing collaborative deliveries can be seen as a follow-up step once order sizes and quantities increase or if goods are sourced from distanced farmers.
6.6.4. Size of cooperation
To achieve major benefits of collaboration, a minimum number of participating partners is required. Furthermore, to reduce travel distances, it is beneficial for farmers if the cooperating partners are locally clustered, while for food cooperatives such local clusters are of less importance to achieve benefits.
Through the development of a simulation and optimization-based DSS, such effects of collaborative actions can be identified in a flexible and risk-free environment. This enables decision makers to test various configurations of the systems to estimate impacts, build trust and facilitate future real-world implementations.
7. Conclusions
This work investigates benefits of facilitating collaborative logistics activities to supply food cooperatives with fresh regional vegetables. A simulation and optimization-based DSS is developed. It includes horizontal cooperation strategies for both farmers and food cooperatives by combining a metaheuristic vehicle routeing procedure with simulation techniques and food quality models. Results of a sample setting in Austria show that such joint actions allow one to reduce travel distances substantially, particularly if many orders are placed, quantities are small and products are not sourced locally. Both collaborations among food cooperatives and between multiple farmers show promising results. However, delivery food quality potentially deteriorates because of such joint activities due to additional loading activities.
Future work should focus on expanding the DSS to allow investigating additional types of cooperation. Of particular interest is the option to offer joint storage. This further enables one to consider shipping goods between farms to save travel distances and improve food quality. To facilitate such collaborative logistics activities, various related legal and regulative settings need to be investigated in more detail. Including additional food groups, e.g. frozen items and products stored at ambient temperature, and considering interdependencies such as ethylene production further results in a wide range of interesting research questions. Furthermore, studies focussing on social aspects and group decision-making are of interest to support successful and sustainable real-world implementations of collaborative logistics activities among food cooperatives.
Acknowledgements
At WU Vienna University of Economics and Business, support by the WU Jubiläumsstiftung is acknowledged. We further like to thank our project partner BIO AUSTRIA for providing us with data and feedback as well as the anonymous reviewers for their valuable input.
Additional information
Funding
Notes on contributors
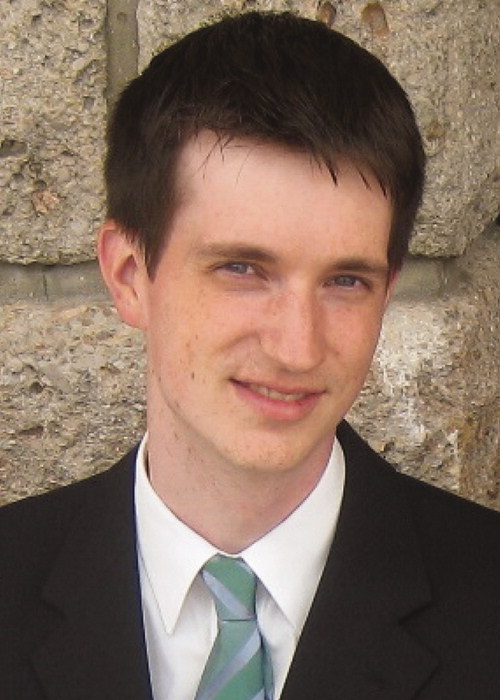
Christian Fikar
Christian Fikar is an Assistant Professor at the Institute for Production Management at WU Vienna University of Economics and Business. He holds a Habilitation in Business Administration and a doctoral degree in Social and Economic Sciences from University of Natural Resources and Life Sciences, Vienna as well as a Master’s degree in Supply Chain Management from WU. His research fields include supply chain management and related model-driven decision support systems to investigate and optimize time-critical delivery processes, particularly for food logistics and humanitarian operations. He teaches various courses related to operations research, data mining and decision support systems.
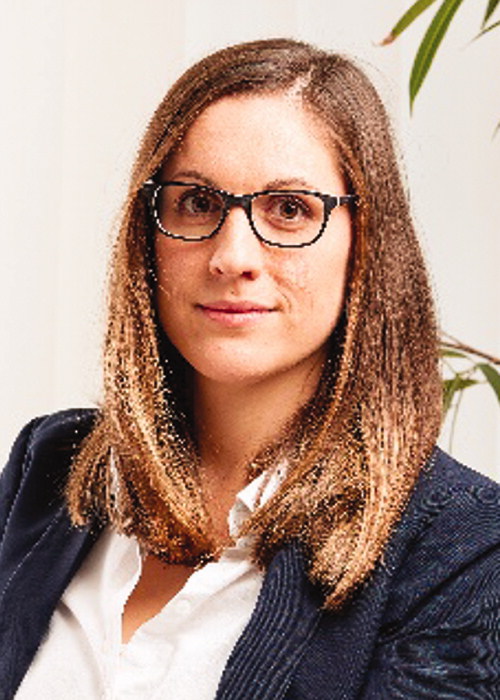
Magdalena Leithner
Magdalena Leithner is a PhD candidate at the Institute of Production and Logistics at University of Natural Resources and Life Sciences, Vienna (BOKU). She holds a Master in Environment and Bio-Resources Management from BOKU. As a key member of the BioHub4all project team, she facilitated collaboration within focal points of fresh fruit and vegetable supply chains in close cooperation with various industrial partners and interest groups within Austria. Her research interests include food logistics, particularly the simulation and optimization of regional fresh food supply chains as well as the integration of food quality models in related model-driven decision support systems.
References
- AnyLogic. 2018. Anylogic – multimethod simulation software. Accessed 8 November 2018. www.anylogic.com/
- BioAustria. 2018. Biomaps. Accessed 8 November 2018. https://www.bio-austria.at/biomap
- Brunori, G., A. Rossi, and V. Malandrin. 2011. “Co-producing Transition: Innovation Processes in Farms Adhering to Solidarity Based Purchase Groups (GAS) in Tuscany, Italy.” International Journal of Sociology and Agriculture and Food 18: 28–53.
- Dax, D. 2017. Das Handbuch zum Gründen und Betreiben von Food Coops [Handbook to Establish and Run Food Coops]. Linz, Austria: BioAustria.
- Dreyer, H. C., J. O. Strandhagen, H.-H. Hvolby, A. Romsdal, and E. Alfnes. 2016. “Supply Chain Strategies for Speciality Foods: A Norwegian Case Study.” Production Planning & Control 27 (11): 878–893. doi:https://doi.org/10.1080/09537287.2016.1156779.
- Fikar, C. 2018. “A Decision Support System to Investigate Food Losses in e-Grocery Deliveries.” Computers & Industrial Engineering 117: 282–290. doi:https://doi.org/10.1016/j.cie.2018.02.014.
- Fikar, C., P. Hirsch, and M. Gronalt. 2018. “A Decision Support System to Investigate Dynamic Last-Mile Distribution Facilitating Cargo-Bikes.” International Journal of Logistics Research and Applications 21 (3): 300–317. doi:https://doi.org/10.1080/13675567.2017.1395830.
- Fonte, M., and I. Cucco. 2017. “Cooperatives and Alternative Food Networks in Italy. The Long Road Towards a Social Economy in Agriculture.” Journal of Rural Studies 53: 291–302. doi:https://doi.org/10.1016/j.jrurstud.2017.01.019.
- Freathy, P., and C. Hare. 2004. “Retailing in the Voluntary Sector: The Role of the Scottish Food co‐Operatives.” European Journal of Marketing 38 (11/12): 1562–1576. doi:https://doi.org/10.1108/03090560410560245.
- GraphHopper 2018., Graphhopper route planner. Accessed 8 November 2018. https://graphhopper.com/
- Hertog, M., and L. M. M. Tijskens. 1998. “Modelling Modified Atmosphere Packaging of Perishable Produce: Keeping Quality at Dynamic Conditions.” Acta Aliment 27: 53–62.
- IG-FoodCoops 2018. FoodCoops. Accessed 17 July 2018. https://foodcoops.at/
- Jaklin, U., S. Kummer, and R. Milestad. 2015. “Why Do Farmers Collaborate with a Food Cooperative? Reasons for Participation in a Civic Food Network in Vienna.” Austria International Journal of Sociology of Agriculutre and Food 22: 41–61.
- James, S. J., C. James, and J. A. Evans. 2006. “Modelling of Food Transportation Systems – A Review.” International Journal of Refrigeration 29 (6): 947–957. doi:https://doi.org/10.1016/j.ijrefrig.2006.03.017.
- Jarosz, L. 2008. “The City in the Country: Growing Alternative Food Networks in Metropolitan Areas.” Journal of Rural Studies 24 (3): 231–244. doi:https://doi.org/10.1016/j.jrurstud.2007.10.002.
- KeyQUEST. 2016. Landwirte-Befragung zu Direktvermarktung [Farmer survey on direct marketing]. Garsten, Austria.
- Laidler, K. J. 1984. “The Development of the Arrhenius Equation.” Journal of Chemical Education 61 (6): 494. doi:https://doi.org/10.1021/ed061p494.
- Leithner, M., and C. Fikar. 2020. “A Simulation Model to Investigate Impacts of Facilitating Quality Data within Organic Fresh Food Supply Chains.” Annals of Operations Research.doi:https://doi.org/10.1007/s10479-019-03455-0.
- Little, R., D. Maye, and B. Ilbery. 2010. “Collective Purchase: Moving Local and Organic Foods beyond the Niche Market.” Environment and Planning A: Economy and Space 42 (8): 1797–1813. doi:https://doi.org/10.1068/a4262.
- Mittal, A., and C. C. Krejci. 2015. “A Hybrid Simulation Model of Inbound Logistics Operations in Regional Food Supply Systems.” 2015 Winter Simulation Conference (WSC); 1549–1560.
- Mittal, A., C. C. Krejci, and T. J. Craven. 2018. “Logistics Best Practices for Regional Food Systems: A Review.” Sustainability 10 (1): 168. doi:https://doi.org/10.3390/su10010168.
- OpenStreetMap. 2018. OpenStreetMap. Accessed 8 November 2018. https://www.openstreetmap.org/
- Paciarotti, C., and F. Torregiani. 2018. “Short Food Supply Chain Between Micro/Small Farms and Restaurants.” British Food Journal 120 (8): 1722–1734. doi:https://doi.org/10.1108/BFJ-04-2018-0253.
- Parragh, S. N., K. F. Doerner, and R. F. Hartl. 2008. “A Survey on Pickup and Delivery Problems.” Journal Für Betriebswirtschaft 58 (2): 81–117. doi:https://doi.org/10.1007/s11301-008-0036-4.
- Ropke, S., and D. Pisinger. 2006. “An Adaptive Large Neighborhood Search Heuristic for the Pickup and Delivery Problem with Time Windows.” Transportation Science 40 (4): 455–472. doi:https://doi.org/10.1287/trsc.1050.0135.
- Serrano-Hernandez, A., J. Faulin, P. Hirsch, and C. Fikar. 2018. “Agent-Based Simulation for Horizontal Cooperation in Logistics and Transportation: From the Individual to the Grand Coalition.” Simulation Modelling Practice and Theory 85: 47–59. doi:https://doi.org/10.1016/j.simpat.2018.04.002.
- Tavella, E., and T. Papadopoulos. 2017. “Applying or to Problem Situations within Community Organisations: A Case in a Danish Non-Profit, Member-Driven Food Cooperative.” European Journal of Operational Research 258 (2): 726–742. doi:https://doi.org/10.1016/j.ejor.2016.08.065.
- Tijskens, L. M. M., and J. J. Polderdijk. 1996. “A Generic Model for Keeping Quality of Vegetable Produce During Storage and Distribution.” Agricultural Systems 51 (4): 431–452. doi:https://doi.org/10.1016/0308-521X(95)00058-D.
- Vlajic, J. V., R. Mijailovic, and M. Bogdanova. 2018. “Creating Loops with Value Recovery: empirical Study of Fresh Food Supply Chains.” Production Planning & Control 29 (6): 522–538. doi:https://doi.org/10.1080/09537287.2018.1449264.