ABSTRACT
This paper presents a methodology to assess the internal and external coherence, and the robustness to future uncertainty, of place-based interventions addressing spatial (in)justice. The methodology merges elements of Theory of Change (ToC) and mechanism mapping with scenario planning. It was designed based on analysis of a range of European interventions (public policies and bottom-up initiatives), selected to highlight the ways spatial injustices have been tackled across different scales. The first phase of the methodology uses a ToC mechanism map to illustrate the logic of the intervention, including its baseline assumptions (internal) and contextual conditions (external). In the second phase, scenarios for the locality are developed based on potential states of global and local macro-trends, revealing how the contextual conditions are expected to change, and whether the intervention's baseline assumptions are likely to hold. This then allows the elaboration of spatial justice trajectories, and for the ToC mechanism map to be updated. To effectively illustrate the methodology, we show how it has been applied using a case study intervention implemented in a territorially disadvantaged area. We present the methodology as a flexible tool allowing detection of more general stylised facts, and spatial comparisons between a broad range of interventions.
1. Introduction
Spatial justice has been defined as ‘an equitable spatial distribution of resources and opportunities, and fairness in the relations of power that shape and transform the social space’ (Madanipour et al. Citation2020, 75). Spatial justice focuses primarily on the implication for justice of spatiality, and the concept draws upon but is distinguished from social justice, which instead focuses on the distribution of resources across social groups.
In terms of how spatial justice is understood and responded to in EU policy, it is not systematically addressed by the European Social Model. This model pursues social justice instead, though there is no official definition of this concept, but rather a ‘conceptual abstraction’ incorporating the EU’s attempt to strike a balance between individuals, the state and the market (Madanipour et al. Citation2020, 21). The EU Cohesion Policy seems more closely related to spatial justice given its focus on wellbeing across space. The Barca Report (2009) recognised that ‘place-based development strategies’ are more effective in promoting the catch-up of lagging areas than neo-liberal approaches, which ignore spatial specificities and can thus be argued to be spatially-blind. Place-based policy is defined in the Report as a ‘long-term strategy aimed at tackling persistent underutilisation of potential and reducing persistent social exclusion in specific places through external interventions and multilevel governance’ (Barca Citation2009: viii). The EU Community-led Local Development programme (previously LEADER) exemplifies how place-based approaches have been applied in practice. But despite place-based development becoming more prominent in the Cohesion Policy rhetoric, cohesion programmes are often shaped by strategic choices of national and regional authorities rather than being bottom-up (Madanipour et al. Citation2020).
The EU covers a large diversity of places, and place-based interventions addressing spatial justice therein. However, a systematic approach to assess the internal and external ‘coherence’ of such interventions, including their response to changing contextual conditions in increasingly uncertain local, national and international situations, is lacking. Such an approach would help enhance the coherence of policy design and evaluation by showing what it is and is not working well, thus delivering sustainable outputs and, ultimately, outcomes (Nilsson and Weitz Citation2019).
To answer these questions, the interventions need to be considered within their contexts and conditions over time. The EU Horizon 2020 RELOCAL project (‘Resituating the local in cohesion and territorial development’), from where this paper derives, sought to assess how far spatial justice can be achieved through place-based strategies, and whether achievements are place-bound or can be extended to other places and times. For doing this, it examined a number of case studies employing policy evaluation approaches integrated with scenario analysis. Policy evaluation approaches like Theory of Change (ToC) and mechanism mapping (see ‘Section 2’) can be used to analyse the interaction of ‘current’ complex contextual conditions with the working logic of an intervention, but do not routinely integrate the impact of future uncertainty on these contextual conditions (Vogel Citation2012). Scenario planning (also detailed below) can help identify the impact of future uncertainty on existing conditions and develop a broad picture of the future of a locality. However, it cannot itself identify the logic mechanism through which an intervention has or will lead to contextual change (Bradfield et al. Citation2005), and to the best of our knowledge, it has not been used to analyse spatial injustice. A systematic methodology integrating these two approaches is thus required.
This paper responds to this evidence gap and aims to present a combined ToC mechanism mapping – scenario methodology for assessing the current and long-term coherence and sustainability of place-based interventions tackling spatial (in)justice. It goes on to demonstrate the value of such a methodology by applying it to a RELOCAL case study.
In RELOCAL, the methodology was adopted in a work package that aimed to formulate future spatial justice scenarios for specific types of EU regions and typologies of spatial injustice, represented by 33 case studies in 11 EU countries. The case studies were selected either for their relevance to place-based development, or as coping strategies for improving living conditions and promoting more balanced and sustainable development. Full details can be found in Copus et al. (Citation2020) and Piras et al. (Citation2020).
Our methodology is designed to enable monitoring and refinement of interventions during their implementation, but can also be used for ex-post evaluation, to inform the design of future policies in the same locality or elsewhere. Given the analytical complexity of the methodology, its use is intended for the policy or research practitioners (henceforth ‘analysts’), whilst policy-makers are likely to be interested in the outputs produced (specifically the ToC mechanism maps and the scenarios). Also, important in an effective application of the methodology is the involvement of local stakeholders, as ‘there is no single true, objective understanding of a policy problem that can be discovered through analytical work’ (Nilsson and Weitz Citation2019, 261). Besides better policy design, a likely benefit of involvement for stakeholders is the self-reflection exercise.
2. Theoretical background
Our methodology integrates elements of ToC, mechanism mapping and scenario building. This section describes the theoretical background underpinning such approaches.
ToC (Taplin and Clark Citation2012; Blamey and Mackenzie Citation2007; Connell and Kubisch Citation1998) is part of a suite of approaches to policy design and evaluation which emphasize the need to clearly specify the ‘internal’ logic underpinning an intervention, that is, the rationale behind it and the causal pathway(s) from the initial input to the final impact under certain assumptions. It aims to facilitate subsequent monitoring of the policy process in relation to one or more logical chains of cause-and-effect links, and evaluate the changes achieved against explicit goals. Related approaches include ‘realistic evaluation’ (Pawson et al. Citation2001; Pawson and Tilley Citation2005; Tilley Citation2000), ‘logic models’ (Anderson et al. Citation2011; Kaplan and Garrett Citation2005; McLaughlin and Jordan Citation1999), ‘theory-based evaluation’ (Stame Citation2004) and ‘results-based management’ (Spreckley Citation2009). ToC was selected over other approaches for its strong focus on complex socio-economic and institutional processes underlying social change, which facilitates its integration with mechanism mapping, and for the key role assigned to stakeholder participation.
While the ToC of an intervention focuses on its ‘internal’ logic, mechanism mapping compares the ToC against underlying contextual conditions. Incorporating mechanism mapping with ToC allows both ‘internal’ consistency and ‘external’ validity (i.e. the transferability of the intervention between contexts) to be considered. For example, if a successful concept for the delivery of health services in urban areas had been adopted, a combined ToC and mechanism mapping approach could be used to consider the consequences of replicating this concept in a sparsely populated region. Integration between Toc and mechanism mapping was devised by Williams (Citation2017, Citation2020). Such integration favours a focus upon the assumptions underpinning the intervention logic, and how promoters and inhibitors (whose impact has usually been taken into account at the design stage) affect the outcome. Although Williams (Citation2017, Citation2020) uses mechanism mapping to assess the validity of an intervention logic between different geographic or policy contexts, this paper argues that the same approach can be applied to future scenarios. Indeed, if the contextual conditions vary when the intervention is transposed to a different time, the internal assumptions can also be affected and the logical chains in the ToC may begin to alter. Building on Williams (Citation2017, Citation2020), we propose a graphic convention to illustrate an intervention’s ToC, and to integrate ToC mechanism mapping with scenario planning to include temporal assessment of the robustness of the intervention.
Scenario planning began as an instrument to support public policy, emerging as a formal research method in the 1960s. It owes much to pioneering futurists Gaston Berger, who founded the prospective school and worked in a systematic manner towards ‘clarifying present action in the light of possible and desirable futures’ (Durance and Godet Citation2010, 1488), and Herman Kahn whose mantra to ‘think the unthinkable’ (Kahn Citation1962) is associated with Cold War military planning. Current research methods have been further influenced by the appropriation and expansion of the approach by the corporate world, notably the Shell oil corporation (Shell.com Citation2014; Bradfield et al. Citation2005).
From these three distinct traditions, a range of techniques has emerged (see Foresight Horizon Scanning Centre Citation2009; Bishop, Hines, and Collins Citation2007), and scenario planning today should be regarded as a suite of methods integrating applied knowledge with theoretical underpinnings (Duckett et al. Citation2017). Depending on their use, scenarios have different foci and can assume a range of forms (Bishop, Hines, and Collins Citation2007; Börjeson et al. Citation2006). To situate our approach within this diverse field, a brief summary of the main forms of scenario planning follows.
Quantitative models incorporating mathematical assumptions about the future state of key variables are often deployed to make ‘base case’, ‘best case’ and ‘worst case’ scenarios. These approaches are not well suited to participatory engagements due to a tendency to lack transparency. Critics further identify challenges around making assumptions sufficiently reliable to handle unexpected discontinuities. Probability-based scenarios like cross-impact matrices (e.g. Martelli Citation2014), also grounded in a mathematical treatment of variables, typically incorporate greater commitment to identify assumptions and to force a wide variability on the key trends. It is argued that the effort taken to determine each cross-impact stimulates participants’ thinking about unlikely combinations of events. Qualitative approaches, also widely deployed, are generally defined by a strong narrative element or story. Finally, the combination of qualitative descriptions with quantitative calculations of systemic consequences results in ‘hybrid’ scenarios, like the ‘Story and Simulation’ approach in environmental scenario analysis (Weimer-Jehle et al. Citation2016).
Quantitative, qualitative or hybrid scenarios can take three forms: ‘normative’, which describe an aspirational future condition in which goals are usually cast in terms of changes that the scenario planners would like to see come about; ‘predictive’, which aim at identifying most likely futures and ‘exploratory’, which seek foresight and flexibility by constructing scenarios of what may happen (Elsawah et al. Citation2020; Van Notten et al. Citation2003). An important proviso is that participatory exploratory scenarios may anticipate future states that are also desirable, thereby problematizing a clear distinction between these forms.
Scenarios incorporating narrative elements, such as ours, proceed by considering permutations of the macro-level forces, otherwise known as ‘drivers of change’, that define the boundary conditions of a future world. In their simplest form, a two-axis method is used based upon two macro-level forces, each in one of two possible states. Because choosing just two drivers risks overemphasizing their importance, and delimiting two states restricts their potential development, the morphological scenario approach was developed. This approach uses a matrix to consider a wider range of drivers of change in a greater number of states, with the added advantages of foregrounding more real-world complexity and allowing systematic assessment of combinations of driver states (Johansen Citation2018; Ritchey Citation2009). This form of scenario planning, adopted in our methodology, usually identifies context-specific drivers of change. The morphological approach is often combined with a quantitative analysis (for a good example, see Mora et al. Citation2020), and while the RELOCAL project did not extend to this task, this is entirely compatible with the method we propose.
Regarding scenarios’ chronological horizon, there is great flexibility to consider timeframes from two-to-three years and upwards to the far future; however, policy cycles tend to influence planners to set horizons between 5 and 10 years in the future. In terms of the geographical scope, scenarios are produced at the global, international, national, sub-national and regional or local levels (Kosow and Gaßner Citation2008, 36). Since our methodology was developed for analysing place-based interventions, it is well-suited to develop local scenarios accounting for wider trends.
3. The methodology and its application
Building on the above theoretical premises, our methodology consists of three phases: (i) an integrated ToC mechanism map framework representing the intervention, and the spatial justice-enhancing mechanism it triggers; (ii) elaborating future scenario(s) by identifying plausible changes over a given period in a number of domains; (iii) reviewing the baseline ToC mechanism map based on the scenario(s). These three phases are closely interrelated, and characterised by significant feedback loops between them, and between the steps of each phase. This turns the overall exercise into an iterative learning process. Therefore, analysts must familiarise with all the phases before undertaking it. provides an overview of the three phases, while a step-by-step demonstration of how the ToC mechanism maps and the scenarios are developed is provided as Supplementary Material.
The first phase integrates ToC and mechanism mapping. The two methods are overlapping and complimentary as the assumptions identified through the ToC are a crucial foundation for mechanism mapping. When the assumptions are specified, their relationship with contextual conditions is also considered. For example, the assumption that ‘better qualifications lead to better employment for everyone’ would be questionable in a context of ‘minority groups suffering systematic discrimination’. Furthermore, if analysts already have an idea of which contextual conditions are likely to change in the future, their choice should reflect this. For example, ‘limited accessibility’ could be included among the conditions if the building of a new road is foreseen. Our approach deviates from the original ToC mechanism map because the process is implemented ex post on an existing (or ended) intervention. Henceforth, the joint output of the ToC and mechanism mapping exercises is identified as ‘mechanism map’.
The second phase is to formulate scenarios to explore how the intervention is likely to perform within a potential future context. While the mechanism map is aimed at analysing the intervention, the scenario itself focuses on the context presented in the locality.
The final phase is to re-assess the mechanism map by taking account of the changed external conditions specified in the scenario, and exploring their implications for the ability of the intervention to improve spatial justice in the locality. This phase includes subjectivity with regards to the selection of changes to incorporate in the new assumptions; however, as long as analysts are explicit about their choices, this will not undermine the value of the mechanism mapping scenario exercise as a ‘learning machine’ to explore the effectiveness, potential and limitations of interventions.
Our methodology needs to be understood not as a prediction exercise, but rather as a strategic planning process that takes account of future uncertainties. Given the complexity and the high level of abstraction required, analysts are meant to lead this exercise. The amount of evidence collected, and the number and types of stakeholders involved can vary considerably depending on the nature of the intervention itself as well as on time and resource constraints. Williams (Citation2017, 2) highlights that data gathering could range from ‘a systematic process of seeking empirical evidence […] through descriptive statistics, qualitative data, and […] relevant impact evaluations’, to ‘a quick and informal desk exercise undertaken by a single policy-maker’. When participatory policy-making processes are concerned, inputs from impacted populations and implementing staff are key (Williams Citation2020).
We suggest that the three methodological phases are all led by the same team to ensure consistency. Following the approach used in RELOCAL, analysts could first gather information about the legal, administrative, and socio-economic context of the intervention through desk research. Second, they could organise in-depth qualitative interviews with key stakeholders, including the institutions and organisations responsible of implementing the intervention, and its final recipients. Focus groups could be used to gather the view of ordinary citizens if the resources allow this. The scenario elaboration is best achieved as a collective exercise of the analysts team. The leading role of analysts, who use their expertise to interpret and integrate information collected from different sources, is aimed at reducing the bias due to speculation or intuition. However, it is key that stakeholders are consulted in each phase to provide feedback, and possibly review some aspects before starting the next phase.
Along with the description of the methodology, in the following, we show how this has been applied to a place-based intervention addressing ‘territorial disadvantage’ – which is one of the three types of spatial (in)justice defined in RELOCAL, the other two being ‘neighbourhood effects’ and ‘place disempowerment’ (Copus et al. Citation2020). Territorially disadvantaged localities are characterised by difficulties, compared to elsewhere, in seeking employment; achieving equivalent levels of income and wellbeing; and accessing services. The reason may be geographical, for example, remoteness or lack of resources; historical, like some form of structural inertia or lack of control over resources; or ‘softer’, such as the weakness of social capital, which inhibits cooperation between individuals and businesses. Here, we present the intervention ‘Strengthening Communities’, implemented in the Isle of Lewis, part of the Outer Hebrides, Scotland (Currie, Pinker, and Copus Citation2019). The time horizon in our case study is set to 2030, while the baseline mechanism maps date back to 2018. Examples of application to interventions addressing ‘neighbourhood effects’ and ‘place disempowerment’ are provided as Supplementary Material.
3.1. Phase one: Baseline ToC mechanism mapping
The baseline mechanism map centres around a systematic diagram that deconstructs the logic underpinning the intervention, and the assumptions upon which this logic is conditional, using graphic conventions. This, in turn, allows the charting of family resemblances between interventions and implied concepts of spatial justice.
The mechanism map for ‘Strengthening Communities’ was elaborated by research practitioners, who first collected evidence through desk research, and then led the fieldwork in Lewis, where stakeholders were interviewed and focus groups organised. The mechanism map was then circulated to stakeholders for feedbacks.
To build their mechanism map, analysts must follow the seven steps below. These are identified by numbered red circles in the mechanism map for ‘Strengthening Communities’ in Lewis ().
A concise description of the long-term goal of the intervention must be entered at the top of the diagram; this must be realistically achievable and thus below the ‘accountability ceiling’.
Figure 2. Baseline ToC mechanism map for ‘Strengthening Communities’ in Lewis, with the specification of the steps to elaborate it.
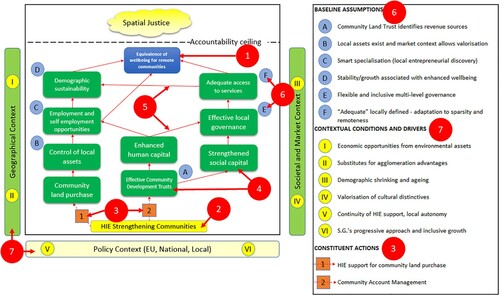
The intervention ‘Strengthening Communities’, administered by the regional development agency Highlands and Islands Enterprise (HIE), aims at fostering socio-economic development in ‘fragile areas’. Its long-term goal is to achieve a broad ‘equivalence of wellbeing for remote communities’.
(2) The name of the intervention must be added at the bottom.
In the Lewis case study, this corresponds to ‘HIE Strengthening Communities’.
(3) One or more constituent actions of the intervention must be added above its name; these are elementary ‘blocks’ in which the intervention can be decomposed, for example, a ‘soft’ and a ‘hard’ one.
‘Strengthening Communities’ includes two actions: (i) supporting groups of residents (Community Land Trusts) in the process of community land purchase in the context of the Scottish Government’s land reform policy; (ii) legally assisting the Trusts as they explore and develop opportunities for land-based economic activities (Community Account Management).
(4) Intermediate outcomes must be specified in the middle; these represent stepping stones (achievements) between the initial intervention and the long-term goal.
The first stepping stone of action (i) is the purchase of community land, while action (ii) starts with the creation of effective Community Development Trusts. Overall, nine intermediate outcomes were identified for ‘Strengthening Communities’.
(5) The intermediate outcomes must be linked using arrows to form causal pathways or logic chains; it is possible to have multiple causal links from one intermediate outcome to other intermediate outcomes; ideally, each of the constituent actions would generate a separate causal pathway.
The two constitutive actions of ‘Strengthening Communities’ generate two causal pathways: (i) one focused on distributional spatial justice which should lead to additional economic activity and employment, and thus enhanced demographic sustainability; (ii) one focused on procedures, which is about developing local, community and individual capacity to deal with administrative structures.
(6) Most of the causal links between intermediate outcomes are not ‘automatic’ but conditional upon cause-and-effect relationships intrinsic to the place and time analysed; in other words, whether the causal pathways ‘work’ or not depends on baseline assumptions, which must be added to the diagram alongside the causal link(s) or the intermediate outcome(s) affected; these represent either drivers boosting a certain causal pathway, or inhibitors hindering it.
The main baseline assumptions of ‘Strengthening Communities’ are that the Trusts can identify a revenue stream (which proved problematic for one of them); that enhanced wellbeing is associated with demographic sustainability; and that ‘adequateness’ of local conditions is defined by stakeholders relatively to local constraints.
The previous steps are internal to the ToC; however, by ‘zooming out’, we would find that these are conditional upon contextual conditions and drivers (CC&D) within the wider context, leading to the last step:
(7) Key CC&D which affect the current behaviour of the ToC must be added in the boxes surrounding the diagram. They represent the environment where the intervention is implemented. This ‘external environment’ can be divided up into various domains, among which we propose three: geography; policy; and society and market. The CC&D represent the link between the mechanism map and the scenario(s) developed in the next phase; therefore, analysts should select them carefully, possibly deriving them from the ‘states’ of the ‘nexuses of changes’ defined in the following.
In Lewis, CC&D include the wealth of environmental assets; the strong relation with a local customer base as a replacement for agglomeration; demographic shrinking; cultural uniqueness (the Gaelic language, the Free Church); and, on the policy side, continuity of HIE support and of the Scottish Government’s inclusive growth approach.
3.2. Phase two: the scenarios
Once the baseline mechanism map has been finalised, the next phase is to elaborate plausible scenario(s) for the locality. The final goal of the scenario exercise is to assess how changes in key CC&D affect the ability of the intervention to deliver spatial justice in a given term (from 2018 to 2030 in the ‘Strengthening Communities’ case study). For a locality, a scenario elaborated on the basis of our methodology is represented by a combination of states of eight or nine nexuses of change included in a synthetic matrix called ‘nexus-state array’. To capture the level of uncertainty, analysts are required to assess the likelihood of each state of every nexus, except for those deemed of limited relevance for their locality, that is, lacking external consistency based on real-world assessment (Johansen Citation2018). The nexus–state array is the final output of a process of synthesis that starts with the definition of global macro-trends, the DEPEST domains, and combines them into ‘vectors of change’ whose cross-tabulation generates the nexuses and their states. The stages of this process are illustrated in the following.
3.2.1. The DEPEST domains and the nexuses of change
Since spatial justice is affected by the changing socio-economic context, we identified relevant spatio-temporal domains defining this context, and associated macro-trends. The structure chosen for systematising these macro-trends was initialised as DEPEST, an acronym for the domains chosen: Demography, Economy, Policy and Governance, Environment, Society, Technology. The structure follows Aguilar (Citation1967) who coined ETPS (Economic, Technical, Political, Social), a framework which has been subsequently extended in various guises, including PEST, PESTEL, etc (Pestle Analysis Citation2015). This has been used in management sciences within SWOT (Strengths, Weaknesses, Opportunities and Threats) and similar analytical methods. The rationale of ETPS and all its derivatives is to obtain a comprehensive coverage of heterogenous topics by explicitly structuring macro-trends. For each DEPEST domain, potential macro-trends for the period 2020–2030 were first identified to guide analysts in their reflections about plausible futures.
Due to their high level of abstraction and rather global or national than local character, the DEPEST domains could not be used directly to formulate local spatial justice scenarios. Also, the large number of macro-trends generated a myriad combinations and corresponding potential scenarios, impractical for detailed elaboration. The domains were thus disentangled and re-assembled to identify eight ‘scenario building blocks’ yielding more explicit spatial implications that we named ‘nexuses of change’ (a ‘set of parameters defining the problem’, Johansen Citation2018, 118). The nexuses are neither deterministic nor normative, and uncertainty about the evolution of the local context in each nexus’ domain is expressed in terms of two dichotomous vectors whose cross-tabulation generates four mutually exclusive states. Five of the nexuses (N1–N5) are intrinsically spatial; two relate to more aspatial issues of equity (N6) and governance (N7); and one focuses on policy change that, nonetheless, has spatial repercussions (N8). The states were defined in RELOCAL based on expected macro-trends in 2020–2030, and can thus be reviewed by analysts based on the timeframe they are considering. Instead, the nexuses are chosen for their relevance for spatial justice at the local level, and should thus be used as such.
The nine nexuses are the following:
Changing Distribution of Economic Activity (N1) captures the change towards agglomeration or dispersal and servitisation of economic activities. It results from two vectors: (i) dispersal away from current hubs, into rural and remote areas vs. increasing agglomeration; and (ii) the economic development policy approach, ranging from place-based approaches and evolutionary economics to space-blind neo-liberal regional policy and free trade.
Changing Central Places and Services (N2) captures the implications of new mobilities and digitization for individuals’ access to central places and services of general interest. It results from (i) digital dispersion, which may lead to revitalization of rural and remote areas as digitization brings new employment and better services, or further constraints in case of lagged digitalization; and (ii) decarbonized mobilities: new technology may bring them at affordable prices and facilitate counter-urbanisation, or decarbonization may limit the range of daily mobility, favouring population concentration.
Neighbourhood Diversity and Segregation (N3) indicates the degree to which neighbourhoods are increasingly segregated or diverse. This complex situation is mapped through (i) a mobility-driven vector ranging from concentration and city centre gentrification to sprawl and increasing commuting, primarily of middle-class employees; and (ii) a vector reflecting the capacity (and will) of city planning policy to counter segregation, ranging from the promotion of diversity to laissez-faire development.
Demographic Change (N4) is well documented in the EU analytical sphere. The states of this nexus result from cross tabulation of two vectors: (i) growth vs. shrinkage; and (ii) migration patterns, leading to either a balanced age structure and heathy labour markets or ageing and increased demand for healthcare services.
Economic and Social Implications of Climate Change (N5) reflects the spatial changes in economic activity associated with climate change mitigation and adaptation. The associated vectors capture respectively (i) the negative or positive impact upon land-based industries and (ii) the degree to which climate change will open up opportunities for new economic activities, or decarbonisation will introduce constraints on Fordist industrial models.
Equity (N6) identifies whether policy approaches will shift towards more inclusion or exclusion through the interplay of two vectors: (i) macro-economic trends ranging between strong growth and recession and (ii) economic policy, ranging between progressive inclusion through service provision, and non-distributional policy and austerity.
Governance systems (N7) reflects changes in power configurations. It is about the distribution of influence and decision-making competence between strata of multi-level governance systems. The two vectors capture respectively (i) the dichotomy between increasing local autonomy and centralisation, including at EU level and (ii) the tension between scale economies advocated by the neo-liberal concept of efficiency, and competing citizens’ rights-based concepts like ‘territorial equivalence’.
Policy approaches (N8) captures changes in the EU, national and local policy. The two vectors reflect (i) the dichotomy between expansionary and contractionary EU policy and (ii) the programme or project-based approach to development of national and local institutions. Regional horizontal programmes imply a holistic vision for a territory, while project-based approach relying on EU or other funds entails a stronger role for local institutions but less vertical coordination.
A local nexus (N9) can be added by analysts to reflect aspects that are so influential, and unique to the locality, that they deserve the consideration afforded by a separate nexus. It might be a new piece of transport infrastructure (e.g. a highway, a metro station), a new aspect of governance or policy (e.g. a reform of local administration), etc.
3.2.2. The nexus–state array
The states of the above nexuses are combined to form a nexus–state array, that is, a version of the ‘sector factor array’ used by Coyle and Yong (Citation1996), or the ‘morphological box’ of Johansen (Citation2018). Indeed, the process up to this point is loosely inspired to the first three ‘steps’ of the morphological scenario analysis illustrated by the latter. The nexus–state array serves as a ‘palette’ of scenario elements from which analysts are invited to select as a framework for their scenario narrative(s). The four states of each nexus are designed to be mutually exclusive, so only one state must be chosen from each column. Not all nexuses are relevant to a specific context, and therefore it is not necessary to select one state from every column.
Apart from providing a framework for the scenario, the nexus–state array is intended to introduce an element of flexible standardisation, facilitating subsequent comparison of scenarios for different localities. For this purpose, a scoring procedure is included to capture the relevance of each nexus, and the relative likelihood of each of its states.
Each potential combination of the states (one per each nexus) represents a different scenario. However, only the combinations that present internal logical consistency, that is, that are free from contradictions between the states (Elsawah et al. Citation2020) should be considered. This is a concern particularly for qualitative scenarios. Elsawah et al. (Citation2020) list a number of methods that have been developed to address this challenge, among which Cross Impact Balances (CIB) (Weimer-Jehle Citation2006), well-suited for socio-environmental systems scenarios. A similar method, recommend by Coyle and Yong (Citation1996) to eliminate the combinations that are highly unlikely or lack external consistency with real-world assessment, is Field Anomaly Relaxation (FAR). In turn, Johansen (Citation2018) suggests reducing the overwhelming number of combinations arising from even a small number of factors by eliminating those including ‘inconsistent value pairs’, that is, pairs of states that are assessed ex ante as lacking logical or empirical consistency.
Given the high diversity implied in a place-based approach, we suggest that analysts omit consistency checks as a preliminary stage, and carry out the process of exclusion ex post, on a case-by-case basis. A second reason for taking this case-by-case approach is that analysts and stakeholders would otherwise face many combinations of states to be assessed jointly, while the nexus-state array involves judging a few nexuses. Finally, since the states of the nexuses represent the link with the re-mapping phase, it is key that analysts reflect on them separately and not only on the overall picture.
The nexus-state array should be completed according to five steps. These are identified by numbered green circles in , which is a nexus-states array compiled with relevance and likelihood scores for the Lewis case study in 2030. For Lewis, the table was compiled by the same team of researchers who developed the baseline mechanism map, and then sent to key stakeholders for feedbacks. Compared to other methodological phases, this one is also suitable for implementation during a participatory event. The five steps are the following:
Analysists must identify whether there are specific local dynamics that deserve to be represented using a local nexus and, if so, define two dichotomous vectors, and input the states generated through their cross-tabulation in the last column of the nexus-state array.
Lewis is characterised by specific cultural features, primarily the Gaelic language and local Free Church. The role of these specificities in future local development depends on the Scottish Government, which is currently keen to protect Scottish culture but is also committed to an open and cosmopolitan society. This is captured by the vector ‘strengthened local identity – homogenisation’. Meanwhile, the British identity and approach to local development will be shaped by ‘Brexit’ and the form it will take, either hard or soft, representing the second vector. The cross-tabulation of these two vectors generates the four states of a local ‘identity’ nexus.
(2) For each nexus, analysis must indicate in the row ‘relevance’ how relevant a change in the states of this nexus would be for the locality, from 1 being ‘totally irrelevant’ to 5 ‘very relevant’.
The changing distribution of economic activity, central places and services, new governance systems and identity issues are very relevant for Lewis, while neighbourhood diversity and segregation is totally irrelevant.
(3) For each state of every nexus, in the row ‘likelihood’ it must be indicated how likely is that the nexus will assume this particular state in the locality in the future, from 1 being ‘very unlikely’ to 5 ‘very likely’.
In Lewis, the future in terms of demography shows limited uncertainty, with depletion being the most likely state; the climate change and identity nexuses also show limited uncertainty, leaning towards a double dividend, and strengthened local identity in a hard Brexit context, respectively. Instead, there is high uncertainty concerning policy approaches, resulting in very similar scores for more states.
(4) By combining, for each nexus, the state (or one of the states) to which a high likelihood has been assigned, analysts can generate one or more scenarios for the locality; if a low relevance of 1 or 2 was assigned to a certain nexus, there is no need to select a state from this nexus.
In , a single, ‘most plausible’ scenario for Lewis is identified by highlighting the most likely states of each nexus in blue and connecting them with a red line. The scenario reveals a persistence of a neo-liberal economic paradigm favouring agglomeration, partially counterbalanced by the Scottish Government’s progressive response to decline, improved rural digitalization, and opportunities generated by a milder climate. The local identity is expected to become a point of increasing strength even in the Brexit context. Although future governance and policy approaches remain highly uncertain, more centralization in Edinburgh is foreseen. Such trends are likely to foster the ongoing demographic depletion.
(5) Since no consistency check is implemented ex ante, it is important that for each scenario generated, analysts assess the joint coherence of the states selected. This could be done by identifying inconsistent pairs ex ante, as suggested by Johansen (Citation2018), and excluding any combinations resulting in the selection of one of these pairs.
The scenario for Lewis presented in has already been controlled for coherence, and no major issues were identified after selecting the most likely states of the nine nexuses.
The combination(s) of states identified according to the above instructions represent(s) the scenario(s) for the locality, and can be qualitatively illustrated in ‘pen picture(s)’ where analysts, in consultation with local stakeholders, creatively envisage the future of the locality if the combination(s) are realized. Additionally, the ‘scenario table’ is used as such in the next methodological phase, where the states selected are contrasted with existing CC&D.
In RELOCAL, the methodology was used to assess ongoing interventions, or interventions whose impacts were not yet fully realized but that could be replicated in other studies subject to limited adjustments. For this reason, and to keep the number of scenario manageable (given the need to compare 33 case studies afterwards), local analysts were asked to generate a single exploratory, most plausible scenario for their locality, typically referred to as a predictive scenario (Börjeson et al. Citation2006). This was done by combining the most likely (or one of the most likely) state(s) of each nexus – a choice replicated in the example here. Scenario planning is essentially a contingent and modular exercise (Durance and Godet Citation2010), and our particular enquiry was designed to explore probable outcomes rather than the kind of more aspirational outcomes often associated with normative scenario building. This approach allows consideration of critical uncertainties while moving the back-casting elements to the following phase. Nevertheless, this choice does not represent a constitutive aspect of our methodology, and the nexus–state array allows selection of more than one scenario, for example, a possible ‘best case’, and a possible ‘worst case’ scenario.
Similarly, additional modules, beyond the scope of our method, can be added to address further questions that analysts may require. Scenario analyses, for weakly structured problems around highly complex real-world systems, including the question of spatial justice, often turn to Cross Impact Analyses, attempting to model the system dynamics. The aim is to equip strategic planning with multidisciplinary analyses of correlations between relevant problem areas that can be expressed quantitively. Both probabilistic and deterministic techniques, using quantitative variables, have been developed. For a fuller discussion, see Weimer-Jehle (Citation2006) or Mora et al. (Citation2020).
Finally, while the RELOCAL team elected to elaborate short-term, 10-year scenarios, our methodology is not prescriptive, and analysts could consider a different timeframe – keeping in mind that longer-term scenarios are subject to wider uncertainty (Taylor Citation1988).
3.3. Phase three: mechanism re-mapping
The final phase of our methodological protocol consists of reviewing the baseline mechanism map by assessing how the changes in the CC&D triggered by the scenario could impact on the underpinning logic of the intervention, and hence on its ability to deliver its long-term goal. If more than one scenario is generated in the previous phase, a new mechanism map must be created from each of them.
The re-mapping exercise proceeds in reverse order compared to the elaboration of the map in the first methodological phase. The four steps of this procedure are identified with numbered purple circles in the upper part of , which illustrates the impact of the most likely scenario for Lewis on the future of ‘Strengthening Communities’. In RELOCAL, this last phase was implemented by the same teams which developed the scenario, and stakeholders’ feedbacks were collected afterwards.
Figure 4. Scenario ToC mechanism map for ‘Strengthening Communities’ in Lewis, with the specification of the steps to review the baseline version (upper part) and consolidated (lower part).
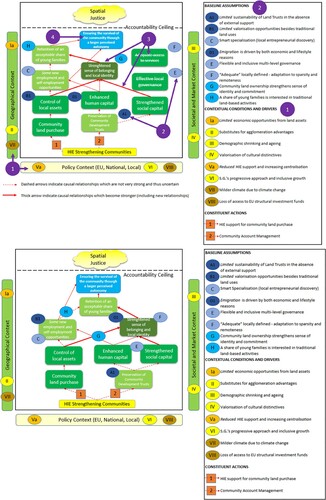
The four steps for updating the mechanism map are as follows:
The CC&D must be reviewed by identifying those which will not be in place anymore in the scenario year; those which will exist but in a slightly revised version; and new CC&D that could emerge. To ensure consistency with the previous phase of the methodology, all change should be linked to one or more of the future states selected for the scenario. In an ‘optimal’ situation, each CC&D corresponds to a potential nexus state, so that changes in states correspond directly to changes in CC&D.
In the mechanism map for ‘Strengthening Communities’, two CC&D were revised, and two were added. Economic opportunities from environmental assets will be limited by remoteness; competition with other areas; and reduction of rents due to depopulation (N4). HIE support will decline due to centralisation, thus limiting local autonomy of decision (‘place-based city-led growth’, N1). Brexit (‘strengthened local identity in a hard Brexit context’, N9) will result in loss of access to EU structural investment funds, namely Cohesion Policy and CAP Pillar II. In turn, in line with ‘double climate change dividend’ (N5), climate change can benefit farming through a longer growing season, and create more opportunities for outdoor activities and thus tourism, at least until 2030.
(2) Based on the changes in CC&D, the baseline assumptions underpinning the intervention (inhibitors and promoters) may cease to hold in their current form, and must thus undergo a similar process of revision, consisting in erasing or reviewing those affected, and possibly adding new ones.
Given the above less favourable conditions, three baseline assumptions of ‘Strengthening Communities’ (financial sustainability of the Land Trusts; the existence of market opportunities; and population stability enhanced by economic growth) are expected not to hold; and two new assumptions are required: that community land ownership enhances place attachment; and that there will be young households interested in land-based activities.
(3) The intermediate outcomes and the causal links between them must also be reconsidered in the light of the changes in the baseline assumptions, by erasing or reviewing those affected; adding new intermediate outcomes; and identifying the causal links which become more robust (by means of ‘thicker’ arrows) or uncertain (by means of ‘dashed’ arrows).
The new, ‘softer’ mechanism activated by ‘Strengthening Communities’ will be centred on a renovated sense of belonging and identity, rather than on economic growth. Retention of population will be driven by community land ownership and social capital through this renovated sense of belonging (the more robust causal pathway), while the pathway from asset control, through new employment opportunity, to demographic sustainability will become very uncertain.
(4) Finally, analysts must reflect whether the long-term goal will still be valid in its current form or must be reconsidered (scaled up, down, re-focused, or has become unachievable).
The long-term goal of ‘Strengthening Communities’ needs to become less ambitious: ensuring the survival of the community by leveraging on a locally-defined sense of ‘adequateness’ and ‘autonomy’, rather than achieving ‘equivalence of wellbeing’ with non-disadvantaged areas.
A consolidated version of the reviewed mechanism map for ‘Strengthening Communities’ is shown in the lower part of .
The CC&D, baseline assumptions, and intermediate outcomes reviewed or added should be identified with specific colours in the new map. While the intervention’s constituent actions are not expected to change in this phase, analysts, local experts, or stakeholders might be aware of future developments in this sense, which must thus be implemented before reviewing the intermediate outcomes.
The re-mapping exercise allows identification of the baseline assumptions more vulnerable to changes in the CC&D, which can thus undermine the causal pathways and hinder the delivery of the long-term goal in its current form. This sort of ‘risk assessment’ can help consolidate the intervention by reinforcing the more uncertain causal pathways or envisaging new, more robust alternatives. If the intervention is already concluded, analysts could instead envisage future adaptation to critical dynamics identified, and use the lessons learned to design more effective interventions in the future.
4. Discussion and conclusions
We have presented a systematic methodology to assess the internal and external coherence of place-based interventions addressing spatial justice in Europe, including their robustness to changing contextual conditions. Although local social justice scenarios have been discussed, for example, in the degrowth literature (Jackson Citation2014), to the best of our knowledge this is the first attempt to develop an ad hoc methodology for assessing spatial justice interventions. Hence, this paper fills an increasingly relevant knowledge gap. The added value of this methodology is that, by integrating policy evaluation and scenario approaches, it allows an account to be taken of both spatial and temporal complexities. Besides assessing a single intervention, analysts can also draw upon our methodology to provide comparative assessments across space, as we have done in RELOCAL.
We have also presented how the methodology has been applied to analyse an intervention addressing territorial disadvantage in remote rural Scotland, namely ‘Strengthening Communities’ in Lewis. The two other case studies in Supplementary Material show that it is flexible enough to address very different examples of spatial injustice. The application has highlighted the methodology’s effectiveness in disentangling the spatial and temporal complexities of place-based policy. Specifically, we were able to identify a mismatch between a stated spatial justice goal, and intermediate outcomes and assumptions built around an economic growth paradigm poorly fitting a remote locality unable to benefit from agglomeration. This misalignment calls for decoupling economic growth and spatial justice goals in policy-making. We further found that the aspirations of local stakeholders must be considered if we wish to achieve a more realistic prediction of long-term outputs and outcomes.
Our semi-qualitative scenario approach (with numeric scores assigned to each state in the nexus-state array, and a qualitative ‘pen picture’ crafted around the scenario selected) proved well-suited to address the two main challenges characterizing scenario development, namely uncertainty and complexity (Elsawah et al. Citation2020), and was well-received by RELOCAL analysts and stakeholders. The choice of not formally implementing ex ante consistency checks, preferring to discuss the coherence of the combinations of states ex post, allowed complexity to be kept at a manageable level, avoiding joint assessment of large numbers of combinations and facilitating stakeholder involvement. The risk of generating incoherent scenarios could represent a limitation of our approach, especially due to the difficulty in identifying ‘triangular interactions’ of states (Elsawah et al. Citation2020). Nevertheless, RELOCAL analysts and stakeholders successfully overcame it thanks to their familiarity with the context, pointing to the importance of including the right expertise and of facilitating skills (Elsawah et al. Citation2020). As a robustness check, future works could analyse ‘relevance’ and ‘likelihood’ scores quantitatively (e.g. with principal component analysis) to detect underlying macro-dynamics, especially if comparing several interventions.
Our methodology requires elaboration of an exploratory scenario (Van Notten et al. Citation2003). Normative (back-casting) aspects are approximated in mechanism mapping, where analysts must plan for robustness to changing external conditions. It must be borne in mind that while the baseline map is aimed at visualising how the intervention should work if all the baseline assumptions hold, that is, the vision of the policy-makers when designing the intervention, the reviewed map illustrates how the intervention is expected to work if the scenario materialises. The need to balance normative and exploratory approaches in different phases of the methodology could be challenging – a dilemma familiar in participatory scenario building. The RELOCAL case studies showed that analysts should pay particular attention to this aspect.
The methodology was developed to study place-based interventions implemented in the EU, and to generate 10-year scenarios. Therefore, we suggest its applicability particularly for this timeframe, this scale and in the same or similar regions of the world. Nevertheless, the short-to-mid-term horizon is not constitutive, and analyst can set a different one. Equally, the development of a single, most plausible scenario is not constitutive of the methodology. In RELOCAL, it was applied to 33 case studies; hence, we opted to focus on the variation of scenarios across localities rather than overloading our policy audience with additional cross comparisons from within individual localities, which allowed manageable assessment of the internal and external coherence of interventions. Future applications should pay particular attention to the scaling issue (Elsawah et al. Citation2020), by ensuring that the states selected represent local manifestation of higher-level macro-trends. Another challenge, especially if more than one scenario is developed, is to ensure sufficient diversity between them, although this could cause problems in terms of consistency (Elsawah et al. Citation2020). The states in the nexus–state array, and their scores, could be used to generate measures of distance for this purpose.
The elaboration of the mechanism map could represent a challenge due to the need to be highly abstractive. Although our novel, user-friendly graphical convention facilitated this exercise, for some RELOCAL case studies a further round of revisions was needed to achieve a satisfactory deconstruction of the intervention’s logic. This complexity implies that the methodology cannot be applied directly by stakeholders without policy analysis expertise, and this could represent a limitation. Nevertheless, the methodology was successfully applied by analysts other than the developers, and to 33 different EU contexts.
In some RELOCAL case studies, analysts had difficulty identifying clear assumptions, intermediate outcomes and the CC&D of their interventions. This difficulty may also suggest that the interventions had been poorly designed (indeed, this was more pronounced for less successful ones), and that more robust tools for ex ante impact assessment and course monitoring are needed, as advocated by Nilsson and Weitz (Citation2019). While these authors focus on trade-offs and coherence between policies, other interventions can be factored in as baseline assumptions, or CC&D. Moreover, our methodology is well-suited to integrate the ‘analytical-deliberative approaches’ deemed ‘essential’ by them (Nilsson and Weitz 2019, 261). Involvement of local stakeholders is key to improve the reliability of the exercise.
The RELOCAL case studies were developed before the outbreak of the COVID-19 pandemic that began affecting Europe in March 2020. Therefore, the direct impact of COVID-19 and of the national measures taken to limit its spread is not factored in. Such events are likely to make spatial injustices even more pronounced (due to interventions being implemented quickly and without due consideration of the spatial justice implications), calling for systematic approaches to identify where future support might be required. In this sense, the presentation of our methodology is timely.
By integrating mechanism mapping and scenario planning, our methodology encourages a more structured design of policy interventions and the adoption of a more long-term view. Building on it, policy practitioners might address shortcomings in both the design and execution stages of place-based policies. Future applications could take advantage of the potentialities of scenario planning, for example, by using the nexus-state array to identify more possible scenarios, characterized by unlikely yet disruptive states of the nexuses; and by assessing the robustness of the interventions in these future conditions. Additionally, they could test the sensitiveness of baseline assumptions to uncertainty by allowing the state of one or more nexuses to vary. Finally, they could explore different time horizons, providing allowances for variable levels of uncertainty are adequately factored in.
Supplemental Material
Download MS Word (1.4 MB)Supplemental Material
Download PDF (1.1 MB)Acknowledgments
The authors would like to thank the RELOCAL project partners who implemented the case studies and applied the methodology to their interventions, particularly Estelle Evrard for her report on ‘EPA Alzette-Belval’, as well as all the stakeholders involved in the Lewis case study. They are also grateful to Ali Madanipour for a first revision of the manuscript.
Disclosure statement
No potential conflict of interest was reported by the author(s).
Additional information
Funding
References
- Aguilar, F. J. 1967. Scanning the Business Environment. New York: Macmillan.
- Anderson, L., Mark Petticrew, Eva Rehfuess, Rebecca Armstrong, Erin Ueffing, Phillip Baker, Daniel Francis, and Peter Tugwell. 2011. “Using Logic Models to Capture Complexity in Systematic Reviews.” Research Synthesis Methods 2 (1): 33–42. doi:https://doi.org/10.1002/jrsm.32.
- Barca, F. 2009. An Agenda for a reformed Cohesion Policy –A place-based approach to meeting European Union challenges and expectations, Independent report prepared at the request of Danuta Hübner, Commissioner for Regional Policy, April 2009.
- Bishop, P., A. Hines, and T. Collins. 2007. “The Current State of Scenario Development: An Overview of Techniques.” Foresight – The Journal of Future Studies, Strategic Thinking, and Policy 9 (1): 5–25.
- Blamey, A., and M. Mackenzie. 2007. “Theories of Change and Realistic Evaluation: Peas in a Pod or Apples and Oranges?” Evaluation 13 (4): 439–455. doi:https://doi.org/10.1177/1356389007082129
- Börjeson, L., M. Höjer, K. Dreborg, T. Ekvall, and G. Finnveden. 2006. “Scenario Types and Techniques: Towards a User’s Guide.” Futures 38: 723–739. doi:https://doi.org/10.1016/j.futures.2005.12.002
- Bradfield, R., G. Wright, G. Burt, G. Cairns, and K. Van Der Heijden. 2005. “The Origins and Evolution of Scenario Techniques in Long Range Business Planning.” Futures 37 (8): 795–812. doi:https://doi.org/10.1016/j.futures.2005.01.003
- Connell, J., and A. Kubisch. 1998. “Applying a Theory of Change Approach to the Evaluation of Comprehensive Community Initiatives: Progress, Prospects, and Problems.” New Approaches to Evaluating Community Initiatives 2 (15–44): 1–16.
- Copus, A., S. Piras, P. Tobiasz-Lis, K. Dmochowska-Dudek, M. Wójcik, and T. Napierała. 2020. D8.2 Synthesis Report: Towards an operational concept of spatial justice, Project Report, H2020 RELOCAL, Version 1, 30 September 2019.
- Coyle, R. G., and Y. C. Yong. 1996. “A Scenario Projection for the South China Sea: Further Experience with Field Anomaly Relaxation.” Futures 28 (3): 269–283. doi:https://doi.org/10.1016/0016-3287(96)00001-8
- Currie, M., A. Pinker, and A. Copus. 2019. Strengthening Communities on the Isle of Lewis in the Western Isles, United Kingdom: RELOCAL Case Study N°33/33. Joensuu: University of Eastern Finland.
- Duckett Dominic, McKee Annie, Sutherland Lee, Kyle Carol, Boden Lisa, Auty Harriet, Bessell Paul, McKendrick Iain. 2017. “Scenario Planning as Communicative Action: Lessons from Participatory Exercises Conducted for the Scottish Livestock Industry.” Technological Forecasting and Social Change 114: 138–151. doi:https://doi.org/10.1016/j.techfore.2016.07.034
- Durance, P., and M. Godet. 2010. “Scenario Building: Uses and Abuses.” Technological Forecasting and Social Change 77 (9): 1488–1492. doi:https://doi.org/10.1016/j.techfore.2010.06.007
- Elsawah Sondoss, Hamilton Serena, Jakeman Anthony, Rothman Dale, Schweizer Vanessa, Trutnevyte Evelina, Carlsen Henrik, Drakes Crystal, Frame Bob, Fu Baihua, Guivarch Celine, Haasnoot Marjolijn, Kemp-Benedict Eric, Kok Kasper, Kosow Hannah, Ryan Mike, van Delden Hedwig. 2020. “Scenario Processes for Socio-environmental Systems Analysis of Futures: A Review of Recent Efforts and a Salient Research Agenda for Supporting Decision Making.” Science of the Total Environment 729: 138393. doi:https://doi.org/10.1016/j.scitotenv.2020.138393
- Foresight Horizon Scanning Centre. 2009. Scenario Planning: Guidance note. http://www.scribd.com/doc/129432607/Foresight-Scenario-Planning#scribd. Accessed May 22, 2020
- Jackson, T. 2014. “New Economy.” In Degrowth: A Vocabulary for a New Era, edited by G. D’Alisa, F. Demaria, and G. Kallis, 178–181. New York: Routledge, Taylor and Francis.
- Johansen, I. 2018. “Scenario Modelling with Morphological Analysis.” Technological Forecasting and Social Change 126: 116–125. doi:https://doi.org/10.1016/j.techfore.2017.05.016
- Kahn, H. 1962. Thinking About the Unthinkable. New York, NY: Horizon Press.
- Kaplan, S., and K. Garrett. 2005. “The Use of Logic Models by Community-based Initiatives.” Evaluation and Program Planning 28 (2): 167–172. doi:https://doi.org/10.1016/j.evalprogplan.2004.09.002
- Kosow, H., and R. Gaßner. 2008. Methods of Futures – and Scenario-analysis: Overview, Assessment, and Selection Criteria. DIE Studies 39. Bonn: Deutsches Institut für Entwicklungspolitik.
- Madanipour, A., M. Shucksmith, H. Talbot, J. Crawford, and E. Brooks. 2020. D1.2 Revised Conceptual Framework for the Project, H2020 RELOCAL, April 2020 [unpublished document].
- Martelli, A. 2014. “From Scenario Building to Scenario Planning: Cross-impact Analysis and Morphological Analysis.” In Models of Scenario Building and Planning: Facing Uncertainty and Complexity, edited by A. Martelli, 157–180. London: Palgrave Macmillan.
- McLaughlin, J., and G. Jordan. 1999. “Logic Models: A Tool for Telling Your Programs Performance Story.” Evaluation and Program Planning 22 (1): 65–72. doi:https://doi.org/10.1016/S0149-7189(98)00042-1
- Mora Olivier, Le Mouël Chantal, de Lattre-Gasquet Marie, Donnars Catherine, Dumas Patrice, Réchauchère Olivier, Brunelle Thierry, Manceron Stéphane, Marajo-Petitzon Elodie, Moreau Clémence, Barzman Marc, Forslund Agneta, Marty Pauline, Bui Elisabeth 2020. “Exploring the Future of Land Use and Food Security: A New Set of Global Scenarios.” PloS one 15 (7): e0235597. doi:https://doi.org/10.1371/journal.pone.0235597
- Nilsson, M., and N. Weitz. 2019. “Governing Trade-offs and Building Coherence in Policy-making for the 2030 Agenda.” Politics and Governance 7 (4): 254–263. doi:https://doi.org/10.17645/pag.v7i4.2229
- Pawson, R., T. Greenhalgh, G. Harvey, and K. Walshe. 2005. “Realist Review – A New Method of Systematic Review Designed for Complex Policy Interventions.” Journal of Health Services Research and Policy 10 (1_suppl): 21–34. doi:https://doi.org/10.1258/1355819054308530
- Pawson, R., and N. Tilley. 2001. “Realistic Evaluation Bloodlines.” American Journal of Evaluation 22 (3): 317–324. doi:https://doi.org/10.1177/109821400102200305
- Pestle Analysis. 2015. Difference between SWOT, PEST, STEEP and STEEPLE analysis. Available at https://pestleanalysis.com/difference-swot-pest-steep-steeple-analysis/ [accessed 29 May 2020]
- Piras, S., M. Currie, D. Duckett, A. Copus, P. Tobiasz-Lis, and K. Dmochowska-Dudek. 2020. D8.3 Scenario Report: Trajectories of Spatial Justice and Actions to achieve it across Europe, Project Report, H2020 RELOCAL, Version 2.2, 31 March 2020.
- Ritchey, T. 2009. “Morphological Analysis.” In Futures Research Methodology — Version 3.0, edited by J. C. Glenn and T. J. Gordon. Washington, DC: Millennium Project.
- Shell.com. 2014. 40 Years of Shell Scenarios. http://s05.static-shell.com/content/dam/shell-new/local/corporate/corporate/downloads/pdf/shell-scenarios-40yearsbook080213.pdf. Accessed May 22, 2020
- Spreckley, F. 2009. Results Based Monitoring and Evaluation. A Toolkit. Local Livelihoods, St Oswalds Barn Hertfordshire, UK. http://www.locallivelihoods.com/cmsms/index.php?page=publications. Accessed April 4, 2019
- Stame, N. 2004. “Theory-based Evaluation and Types of Complexity.” Evaluation 10 (1): 58–76. doi:https://doi.org/10.1177/1356389004043135
- Taplin, D., and H. Clark. 2012. Theory of Change Basics. A Primer. Actknowledge, New York. https://www.actknowledge.org/PDFs/PACETheoryofChangeDiscussionPaper.pdf. Accessed March 9, 2020
- Taylor, C. 1988. Alternative World Scenarios for a New Order of Nations. Carlisle Barracks, PA: Army War College Strategic Studies Institute.
- Tilley, N. 2000. Realistic Evaluation: An Overview. In Founding conference of the Danish Evaluation Society (Vol. 8).
- Van Notten, P., J. Rotmans, M. Van Asselt, and D. Rothman. 2003. “An Updated Scenario Typology.” Futures 35 (5): 423–443. doi:https://doi.org/10.1016/S0016-3287(02)00090-3
- Vogel, I. 2012. Review of the Use of ‘Theory of Change’ in International Development. Review Report. London: Department for International Development (DFID).
- Weimer-Jehle, W. 2006. “Cross-impact Balances: A System-theoretical Approach to Cross-impact Analysis.” Technological Forecasting and Social Change 73 (4): 334–361. doi:https://doi.org/10.1016/j.techfore.2005.06.005
- Weimer-Jehle Wolfgang, Buchgeister Jens, Hauser Wolfgang, Kosow Hannah, Naegler Tobias, Poganietz Witold, Pregger Thomas, Prehofer Sigrid, von Recklinghausen Andreas, Schippl Jens, Vögele Stefan 2016. “Context Scenarios and Their Usage for the Construction of Socio-technical Energy Scenarios.” Energy 111: 956–970. doi:https://doi.org/10.1016/j.energy.2016.05.073
- Williams, M. 2017. External Validity and Policy Adaptation: A Five Step Guide to Mechanism Mapping. Policy Memo. Blavatnik School of Government. University of Oxford. https://www.bsg.ox.ac.uk/sites/www.bsg.ox.ac.uk/files/documents/BSG-WP-2017-019_0.pdf. Accessed July 10, 2017
- Williams, M. 2020. “External Validity and Policy Adaptation: From Impact Evaluation to Policy Design.” The World Bank Research Observer 35 (2): 158–191. doi:https://doi.org/10.1093/wbro/lky010