ABSTRACT
Technology is now being developed that is able to handle vast amounts of structured and unstructured data from diverse sources and origins. These technologies are often referred to as big data, and open new areas of research and applications that will have an increasing impact in all sectors of our society. In this paper we assessed to which extent big data is being applied in the food safety domain and identified several promising trends. In several parts of the world, governments stimulate the publication on internet of all data generated in public funded research projects. This policy opens new opportunities for stakeholders dealing with food safety to address issues which were not possible before. Application of mobile phones as detection devices for food safety and the use of social media as early warning of food safety problems are a few examples of the new developments that are possible due to big data.
Introduction
A huge volume of data is being produced worldwide in nearly all sectors of the society including business, government, health care, and research disciplines such as natural sciences, life science, engineering, humanities, and social sciences. As more and more of this big data become available, it can be used to enable new insights, improve decision-making, and enhance the quality of products and services. The aggregation and the speed at which big data is generated, however, requires to overcome challenges related to efficient collection, storage and processing of data. The applications of big data are highly diverse and vary from recommendation systems of www.Amazon.com (Linden et al., Citation2003b) to real-time surveillance of influenza outbreaks (Ginsberg et al., Citation2009). Several publications have presented many potential applications of big data (Ebeling, Citation2016; Klous and Wielaard, Citation2016; Li et al., Citation2016; Lin et al., Citation2016; Richterich, Citation2016; Ueti et al., Citation2016).
The term “big data” is seldom used in relation to food safety mainly because food safety data and information are scattered across the food, health and agriculture sectors. The application of big data in the food safety domain requires the establishment and implementation of interoperability standards and confidentiality safeguards. Traditional food safety data such as national monitoring data are relatively limited but well structured, although generally not harmonized between regions. To investigate where and how food safety can benefit from the big data approach, we analyzed the applicability in food safety of tools developed within the various stages of big data research (e.g., data collection, data storage and transferring, data analysis and data visualization).
In this study we analyze if and to which extent big data play a role in food safety. Examples will be provided to demonstrate future developments and opportunities.
Big data definition
Many definitions of big data exist. The World Health Organization (WHO) uses the definition of (Ward and Barker, Citation2013): “The emerging use of rapidly collected, complex data in such unprecedented quantities that terabytes (1012 bytes), petabytes (1015 bytes) or even zettabytes (1021bytes) of storage may be required.” Data management challenges for big data are described by Gartner (Citation2012) as having three-dimensional characteristics, i.e., “Big Data is high volume, high velocity, and high variety information assets that require new forms of processing to enable enhanced decision making, insight discovery and process optimization.” The European Commission (EC) has issued a similar definition (EC, Citation2014), referencing the three Vs of Volume, Velocity and Variety: “Big Data refers to large amounts of different types of data produced with high velocity from a high number of various types of sources. Handling today's highly variable and real-time data sets requires new tools and methods, such as powerful processors, software and algorithms.” (De Mauro et al., Citation2015) proposed the consensual definition: “Big data represents the information assets characterized by such a High Volume, Velocity and Variety to require specific technology and analytical methods for its transformation into Value.”
In (all) definitions volume refers to the amount of data (e.g., terabytes to exabytes of existing data to process), velocity is the speed of information generated and how fast the data is processed, and variety represents the variation in data formats (e.g., structured and unstructured data). Structured data refers to a variety of data formats and types that can be fitted neatly into rows and columns (traditional text/numeric information). Unstructured data is information that is not organized such as Twitter tweets, and other social media postings (Arthur, Citation2013). Value is referred to as the costs of data generation and its intrinsic value (Hazeleger, Citation2015), as well as the transformation of big data into valuable new insights, solutions or decisions that otherwise have remained undiscovered and unknown (De Mauro et al., Citation2015). In addition to the four Vs mentioned above (Volume, Velocity, Variety and Value), Veracity and Validity can be considered as big data characteristics as well. Veracity is the uncertainty due to incompleteness, approximations and inconsistencies (IBM, Citation2012). Validity is the question if the data is valid for the problem and has the data sound basis in logic or fact.
Big data as described in the definitions has become a reality in many sectors and the ability to tackle the challenges related to handling and integrating huge amounts of data will provide opportunities to increase competitive advantages.
Application of big data in food safety
The WHO has recently embraced the big data approach to support decision-making in food safety which has resulted in the food safety platform “FOSCOLLAB” to provide integration of different sources from various disciplines (WHO, Citation2015a). In this platform, structured and nonstructured data from multiple sectors such as animal, agriculture, food, public health and economic indicators are integrated and available to the user via several dedicated dashboards (WHO, Citation2015a). The data sources connected in FOSCOLLAB are Evaluations of the Joint FAO/WHO Expert Committee on Food Additives (JECFA) and The Joint FAO/WHO Meeting on Pesticide Residues (JMPR) databases on chemical risk assessment, the WHO database on Collaborating Centres and the GEMS food databases on food consumption and chemical occurrence in food (see ). Information from additional data sources will be integrated as the FOSCOLLAB platform develops, which will support actors operating in the risk analysis of food and feed (WHO, Citation2015a). shows the different stages that can be distinguished when managing big data and which has been adapted for food safety from health sciences (Huang et al., Citation2015). In the next section, each stage will be discussed.
Table 1. Examples of food safety databases.
Data collection in food safety
Various types of sources can be distinguished that may contain or generate information useful for food safety such as (online) databases, internet, omics profiling, mobile phones, and social media. The challenge is to identify relevant data within a data source and to link it to other data sources. In this regard, especially challenging is the use of nontraditional data sources such as social media. Next we will discuss the various types of data sources and how they may be used to generate additional value for food safety.
(Online) database
provides an overview of (online) data sources that contain information related to food safety (directly/indirectly) such as information on a hazard (i.e., monitoring programmes, alert systems, chemical data), exposure (i.e., consumption databases), and surveillance reports on animal and plant diseases. gives an example on which elements in the various types of data sources may be used to connect the data sources (e.g., hazard, (food) product and country) to generate an added value. The data linkages shown in are similar to the ones used in FOSCOLLAB by WHO (WHO, Citation2015a), albeit from different data sources.
Figure 2. An example of possible data linkages between data sources that can provide an added value in the analysis of food safety risks.
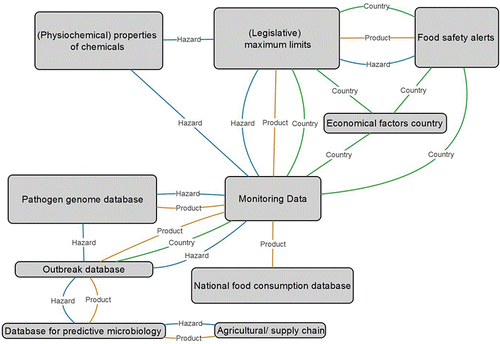
For monitoring data of hazards in food products a few large databases can be identified (e.g., GEMS/ Food, RASFF, see ). The Global Environment Monitoring System (GEMS/food) database (WHO, Citation2015b) contains millions of global monitoring data entries. Given the relatively large volume of entries (600–800 entries/ month), the data are structured in a logical manner and is easily retrievable. Information on the properties of chemicals, growth conditions of microorganisms and weather reports can be of importance for food safety research or can be used in models to predict the presence of certain hazards, for example, mycotoxins in wheat (van der Fels-Klerx et al., Citation2012). These weather reports contain large volumes of data generated with high velocity, just as data collected in the agricultural and supply chain. Food safety incidences are collected in structured databases such as RASFF, but also on websites of the International food safety authorities (e.g., recalls) and in media reports (see MedISys [http://medusa.jrc.it/medisys/homeedition/en/home.html]). These latter data sources are unstructured and scattered over the internet, and therefore harder to retrieve. A similar example is the registration of foodborne outbreaks (e.g., by the CDC). These incidences can be found on the internet or social media as well.
Internet
Internet is a huge source of information and may be exploited to assist risk managers and or risk assessors in maintaining food safety. Web crawling systems have been developed that search the internet for publications on food safety related reports. A typical example of such system is MedISys which is part of the European Media Monitor (EMM) developed by the joint Research Centre (JRC) of the European Commission (Steinberger et al., Citation2013). MedISys is a fully automatic surveillance system that collects 24/7 reports from the internet on human and animal infectious diseases (Linge et al., Citation2009), which also has been adapted to collect food safety related publications (Rortais et al., Citation2010). Analysis of this system showed that it can be used as an early warning system for the detection of food and feed-borne hazards (Rortais et al., Citation2010).
(Online) archives of functional genomics data
Omics is a term that covers multiple disciplines, including genomics (studies on effects of nucleotide variations within genes), transcriptomics (mRNA expression), metabolomics (levels of metabolites), and proteomics (levels of peptides and proteins).
The principle approach for developing toxicogenomics-based predictive assays for chemical safety, and in particular for the purpose of hazard identification, involves that large-scale genomic databases () are derived from exposure of cells or animals to known toxicants (Goetz et al., Citation2011). Toxicogenomics aims to elucidate molecular mechanisms involved in the expression of toxicity and to derive molecular expression patterns (i.e., molecular biomarkers) that predict in vitro and in vivo toxicity using “animal-based” and in vitro (cellular) models (Embry et al., Citation2014). This so-called read-across approach is based on the assumption that similar gene expression profiles dictate similar physiological responses that are used to discover the toxicological properties of a biological or chemical entity. This task, although conceptually simple, is far from easily performed. Toxicogenomics tends to generate “big data” requiring extensive bioinformatics and biostatistics efforts for actually retrieving toxicologically meaningful results. The amount of toxicogenomics data generated internationally is vast, complex, and difficult to interpret statistically and biologically (Suter-Dick et al., Citation2014). It has proven vital to be able to store and manage voluminous toxicogenomics data sets in databases, as linking data resources would improve toxicogenomics research and data analysis (Hendrickx et al., Citation2014). Large amounts of transcriptomics data on toxicogenomics, but also on other types of data like cancer research, are stored in very large databases that are freely accessible. Two examples of these are Gene Expression Omnius (GEO) (Clough and Barrett, Citation2016) and ArrayExpress (Kolesnikov et al., Citation2015).
Presently, a comprehensive knowledge base is being developed as part of the Organisation for Economic Co-operation and Development (OECD) Adverse Outcome Pathway (AOP) program (http://aopkb.org/) that will serve as a central repository for exploratory analyses and predicting human health risks (Oki et al., Citation2016).
Mobile phones
The use of mobile phones is widespread and new applications appear rapidly including food safety and health related applications. Reports have appeared on the use of Smartphones in combinations with other handheld devices to measure (i) Mercury contamination in water (Wei et al., Citation2014), (ii) Ochratoxin A contamination in beer (Bueno et al., Citation2016), (iii) allergens in a variety of food products (Coskun et al., Citation2013), and (iv) microbial contamination (Escherichia coli) in water and food samples (Zhu et al., Citation2012). Collected data can be processed on the phone or via a Wi-Fi connected computer for own purpose but may also be transferred to data clouds or other data centers. An example of such process has been provided by Dzantiev et al. (Citation2014) for nonlaboratory analyses based on immuno-chromatography. Challenges and opportunities of such data in the open source arena should be carefully evaluated to determine the direction such development should be guided.
Social media
Food safety agencies and food associated organizations already are using social media such as Facebook, Twitter and YouTube to communicate with the general public on food safety related issues (Shan et al., Citation2014). By monitoring users' conversations on social media, food agencies will better understand their audience and may detect new issues. Web mining and social media analysis approaches are being developed to exploit the huge amount of data as an early warning system for identification of potential health and food safety issues that may develop into a crisis (Meyer et al., Citation2015).
Data storage and transferring
Generally, data storage is achieved using data management systems, such as MySQL, Oracle, and PostgreSQL (see ). However, such systems are not sufficient to support big data handling. Much more speed, flexibility and reliability are needed in these cases than these traditional systems can deliver. Therefore, next generation databases have been developed which are nonrelational, open source and horizontal scalable and are referred to as NoSQL. Examples of such systems are MongoDB, Cassandra, and HBase.
Table 2. Examples of data storage, processing, transferring and visualisation.
Following storage, the next challenge is moving big data from different sources of data into a NoSQL cluster for processing. For this, transferring software is needed and examples of such software used to handle big data are Aspera and Talend.
Data analysis
Following storage and moving the data to the processing unit in NoSQL, the data should be processed. A list of the most used analysis methods for big data is shown in . These methods can be classified in two categories: (1) Recommendation System and (2) Machine Learning.
Table 3. Examples of data analysis methods.
Recommendation systems are information filtering systems that elicit the preferences, interest, or observed behavior of consumers and make recommendations accordingly. They have the potential to support the decisions consumers make while searching for and selecting products online (Chenguang and Wenxin, Citation2010; Konstan and Riedl, Citation2012). These systems are used by e-commerce organizations to advice their customers based for example on the top sellers on a site, demographics of the customer, analysis of the past buying behavior of the customer, etc. (Mishra et al., Citation2015). These systems are developed using data mining techniques (collaborative filtering, content based filtering and hybrid approaches (Goldberg et al., Citation2001) and heuristics (Nakamura and Abe, Citation1998). Examples of recommendation systems in various applications are shown in : Amazon, Netflix, etc. To the author's knowledge, these systems are not yet applied in food safety.
Machine Learning explores algorithms that can learn from and make predictions on data. Machine learning is employed in cases where designing algorithms is complex and to build models from data in order to make predictions or decisions (Kim et al., Citation2015). Several machine learning algorithms are proposed to solve classification problems in the literature: Auto Encoder (Bengio, Citation2009), Restricted Bolzmann Machine (Montavon et al., Citation2012), Bayesian networks (Mkrtchyan et al., Citation2015), Neural networks (Ata, Citation2015), etc. (). Several of these technologies have been used in food safety applications (Beaudequin et al., Citation2015; Bouzembrak and Marvin, Citation2016; Marvin et al., 2016; Esser et al., Citation2015; Lin and Block, Citation2009) and have also been proposed as tool in big data handling in food safety (Wang et al., Citation2015).
Visualization
Several visualization tools are available to analyze and present summaries of the big amount of data, which all have their own advantages and disadvantages (see ). Most commonly used are R and Cicos. R (Schumacker and Tomek, Citation2013) is an open source programming language used in data science to visualize and analyze data that provide plot functions and network plot functions. Circos (Xiao et al., Citation2013) allows to visualize data in a circular layout and to explore relationships between objects or positions. This software has become the standard of visualizing genome chromosomes. For commercial visualization software which does not require programming skills, IBM Many Eyes (see ) and Tableau are good choices.
Examples of big data in food safety
Agricultural chain and food supply chain
In the agricultural chain, big data can be used to predict the presence of pathogens or contaminants by linking information on environmental factors with pathogen growth and/or hazard occurrence. For example, by monitoring the conditions of crops in the field, the areas with an increased incidence of aflatoxins can be identified before entering the food chain (Armbruster and MacDonell, Citation2014). In another study, quantitative models were developed to predict the contamination of the mycotoxin deoxynivalenol (DON) on wheat in northwestern Europe using a variety of models and databases, including weather data (van der Fels-Klerx et al., Citation2012). By characterizing the presence of pathogens on farm fields and by combining this with environmental and meteorological data, the presence of Listeria monocytogenes could be predicted (Strawn et al., Citation2013).
Several big data collection and analytics systems have been developed to support farmers in decision making such as SemaGrow (http://www.semagrow.eu/). This system uses algorithms and tools for the efficient querying of large-scale data sets and independent data sources. It specifically focused on the agriculture domain and its use cases through merging and integrating a large and very diverse spatio-temporal data sets. One of the use cases explored in SemaGrow is regional agro-climatic modelling in the frame of climate adaptation (Lokers et al., Citation2016).
Another example is the system developed in the European research project “Trees4future” (www.trees4future.eu). In the Trees4Future project, forestry scientific data was made accessible for scientists and decision makers and several models (The ForGEM model (Kramer et al., Citation2013), the EFISCEN model (Nabuurs et al., Citation2000) and the Tosia model (Lindner et al., Citation2010)) were linked to assess climate change impacts and explore climate adaptation strategies.
In the supply chain, tracking and tracing of food is mandatory to ensure quick recalls. This can be broadened by using GPS, sensor-based and RFID technologies. In this way, near or real-time data can be collected on the location and other attributes of the food (e.g., temperature). A large U.S. restaurant chain (The Cheesecake Factory) collects large volumes of data on transportation temperature, shelf life, and food withdrawals which is analyzed by IBM Big Data Analytics. When something is amiss, the affected food can quickly be recalled from all the restaurants (HACCPEurope, Citation2013). Wal-Mart Stores Inc. uses a Sustainable Paperless Auditing and Record Keeping (SPARK) system that automatically uploads data (like food temperature) to a web-based recordkeeping system. In one month, internal cooking temperatures of rotisserie chickens were measured 10 times by health officers, 100 times by private investigators and 1.4 million times by SPARK (Yiannas, Citation2015). In this way a lot of data are collected and can be used to quickly identify undercooked chicken.
Outbreaks and source identification
During a food safety outbreak a large number of samples are collected and analyzed, leading to large volumes of data and information that is used in identifying the source of the outbreak. Development of techniques in rapid screening of pathogen genomes (whole genome sequencing, next-generation sequencing) results in a collection of the specific genomic information and the (historical) occurrence of pathogenic strains or subtypes (Lienau et al., Citation2011). For example, during and after the pathogen “EHEC” outbreak in Germany in 2011, information was gathered on the presence of the bacteria in several areas. Homes of healthy individuals were screened for harboring the pathogen and families were monitored to screen for secondary infections. It is expected that such monitoring information may help to detect a problem at an early stage allowing timely preventive measures and consequently preventing an outbreak (Kupferschmidt, Citation2011).
Identification of outbreaks with alternative data sources
In addition to the genomic information, other factors can be used to establish the source of contamination. Gardy et al. (Citation2011) concluded from a study on a tuberculosis outbreak that “genotyping and contact tracing alone did not capture the true dynamics of the outbreak.” Socio-environmental information in combination with whole-genome sequencing of existing and historical isolates were used by these authors to determine the source and cause of the outbreak (Gardy et al., Citation2011). Although the data were not big in “Volume” (36 isolates), the “Variety” of the data was increased by using a social network (interviews with patients).
Doerr et al. (Citation2012) used proactive geospatial modelling to identify the wholesalers involved in the distribution of contaminated food based on the food supply chain. They included in the model the distribution network of wholesalers, the population density, retailer's locations, and consumer behavior.
One study analyzed online customers' reviews of restaurants (yelp.com) for key words related to food poisoning. They compared the results to the Centers for Disease Control and Prevention (CDC) outbreak controls database. The authors postulate that these reviews provide near-real time information on outbreaks and can complement traditional surveillance systems (Nsoesie et al., Citation2014). In addition, (Newkirk et al., Citation2012) also see the potential of using social media to augment food surveillance systems, however, these authors think that the methods are not fully developed yet. big data analysis can provide the resolution for this problem.
Future of big data in food safety
In Europe, the European Commission has developed a strategy on big data and supports a data-driven economy (EC, Citation2014). They support open access of data, e.g., free of charge online access to EU-funded research results, including scientific publications and research data. This involves EU funded projects on (i) crop monitoring for developing countries (e-Agri), (ii) monitoring the whole product lifecycle (LinkedDesign), and (iii) improving the efficiency and quality of the product development process (iprod). Also national governments in Europe such as the Dutch Government are stimulating public–private projects to explore the potentials of big data (Rijksoverheid, Citation2015). In the United States, the Obama Administration launched a “Big Data Research and Development Initiative” to “greatly improve the tools and techniques needed to access, organize, and glean discoveries from huge volumes of digital data” (Obama Administration, Citation2012). The Initiative increased government support and accelerated the Federal agencies' ability to extract knowledge from large and complex digital data. This is also, encouraged private companies, academia, local governments, and foundations to collaborate on new big data projects such as “Data to Knowledge to Action” in 2013 (Whitehouse, Citation2013).
It is clear that these strong driving sources will boost the availability and use of big data in many sectors of our society. It is expected, however, that food safety will not be at the forefront of these developments. The success of new applications and approaches in food safety, such as use of smart phones to measure food safety hazards, combining data from a large variety of sources, including climate data, to analyze food safety risks or the use of social media such as Twitter as information source will strongly influence the future use of big data tools. Finally, the availability of huge amount of data from public funded research projects such as aimed for by the European Commission for H2020 funded projects will provide a new opportunity to generate new insight to food safety issues provided that tools are available to handle the diversity and complexity of such data supply.
In the RICHFIELDS project (www.richfields.eu) innovative consumer support tools will be developed to select healthy food (personalized nutrition). The developed tools will utilize food products data, food intake data, lifestyle and health data, including real time consumer-generate data through the use of mobile apps or tech-wear (consumer information, purchase, preparation and consumer-generated real-time data, etc.) (Van den Puttelaar et al., Citation2016).
Bayesian Networks (BNs) are capable of dealing with such data diversity and have been used for this purpose in many domains, albeit very limited in food safety. We expect that BNs may be useful to implement system or holistic approach in food safety where data from influencing drivers on food safety such as climate change, economy, and human behavior are combined to predict further events of food safety risks (Marvin et al., Citation2016).
Conclusions
A huge amount of data directly and indirectly linked to food safety is being produced worldwide. Currently, only limited numbers of tools developed within the big data domain are applied in food safety. The trend to make data from public funded research projects available on internet opens new opportunities for stakeholders dealing with food safety to address issues not possible before. Especially, the use of mobile phones and advanced traceability systems in food safety monitoring and the use of social media may require tools and infrastructure that have more big data characteristics than currently.
Acknowledgment
The authors would like to thank Dr. L.A.P. Hoogenboom for critical reading the manuscript and his valuable suggestions.
Funding
This research was subsidized by the Dutch ministry of Economic Affairs in the KB programme.
References
- Agerri, R., Artola, X., Beloki, Z., Rigau, G. and Soroa, A. (2015). Big data for natural language processing: A streaming approach. Knowl.-Based Syst. 79:36–42.
- Armbruster, W. J. and MacDonell, M. M. (2014). Informatics to support international food safety. Proceedings of the 28th Conference on Environmental Informatics - Informatics for Environmental Protection, Sustainable Development and Risk Management, pp. 127–134.
- Arthur, L. (2013). Big Data Marketing: Engage Your Customers More Effectively and Drive Value. John Wiley & Sons.
- Ata, R. (2015). Artificial neural networks applications in wind energy systems: A review. Renew. Sustain. Energy Rev. 49:534–562.
- Beaudequin, D., Harden, F., Roiko, A., Stratton, H., Lemckert, C. and Mengersen, K. (2015). Beyond QMRA: Modelling microbial health risk as a complex system using Bayesian networks. Environ. Int. 80:8–18.
- Bengio, Y. (2009). Learning deep architectures for AI. Found. Trends® in Mach. Learn. 2:1–127.
- Bouzembrak, Y. and Marvin, H. J. P. (2016). Prediction of food fraud type using data from rapid alert system for food and feed (RASFF) and bayesian network modelling. Food Control. 61:180–187.
- Bueno, D., Muñoz, R. and Marty, J. L. (2016). Fluorescence analyzer based on smartphone camera and wireless for detection of Ochratoxin A. Sensors Actuators B: Chem. 232:462–468.
- Chen, Y. and Qiao, J. (2015). Protein-protein interaction network analysis and identifying regulation microRNAs in asthmatic children. Allergol. Immunopathol. (Madr). 43:584–592.
- Chenguang, P. and Wenxin, L. (2010). Research paper recommendation with topic analysis. In: Computer Design and Applications (ICCDA), 2010 International Conference on, 25-27 June 2010, pp. V4-264-V264-268.
- Clough, E. and Barrett, T. (2016). The gene expression omnibus database. Methods Mol. Biol. 1418:93–110.
- Coskun, A. F., Wong, J., Khodadadi, D., Nagi, R., Tey, A. and Ozcan, A. (2013). A personalized food allergen testing platform on a cellphone. Lab Chip. 13:636–640.
- De Mauro, A., Greco, M. and Grimaldi, M. (2015). What is big data? A consensual definition and a review of key research topics. AIP Conf. Proc. 1644:97–104.
- Doerr, D., Hu, K., Renly, S., Edlund, S., Davis, M., Kaufman, J. H., Lessler, J., Filter, M., Käsbohrer, A. and Appel, B. (2012). Accelerating investigation of food-borne disease outbreaks using pro-active geospatial modeling of food supply chains. In: Proceedings of the First ACM SIGSPATIAL International Workshop on Use of GIS in Public Health. ACM, Redondo Beach, California.
- Dzantiev, B.B., Byzova, N.A., Urusov, A.E. and Zherdev, A.V. (2014). Immunochromatographic methods in food analysis. TrAC Trends Anal. Chem. 55:81–93.
- Ebeling, M. F. E. (2016). The rise of the databased society. In: Healthcare and Big Data: Digital Specters and Phantom Objects, pp. 27–48. Palgrave Macmillan US, New York.
- EC, E. C. (2014). Towards a thriving data-driven economy. Brussels: Communication from the Commission to the European Parliament, the Council, the European Economic and social committee and the committee of the regions.
- Embry, M. R., Bachman, A. N., Bell, D. R., Boobis, A. R., Cohen, S. M., Dellarco, M., Dewhurst, I. C., Doerrer, N. G., Hines, R. N., Moretto, A., Pastoor, T. P., Phillips, R. D., Rowlands, J. C., Tanir, J. Y., Wolf, D. C. and Doe, J. E. (2014). Risk assessment in the 21st century: roadmap and matrix. Crit. Rev. Toxicol. 44(Suppl. 3):6–16.
- Esser, D., Leveau, J. J. and Meyer, K. (2015). Modeling microbial growth and dynamics. Appl. Microbiol. Biotechnol. 99:8831–8846.
- Gardy, J. L., Johnston, J. C., Ho Sui, S. J., Cook, V. J., Shah, L., Brodkin, E., Rempel, S., Moore, R., Zhao, Y., Holt, R., Varhol, R., Birol, I., Lem, M., Sharma, M. K., Elwood, K., Jones, S. J. M., Brinkman, F. S. L., Brunham, R. C. and Tang, P. (2011). Whole-genome sequencing and social-network analysis of a tuberculosis outbreak. New England J. Med. 364:730–739.
- Gartner (2012). The importance of ‘Big Data': A definition. Available from]. https://www.gartner.com/doc/2057415/importance-big-data-definition.
- Ginsberg, J., Mohebbi, M. H., Patel, R. S., Brammer, L., Smolinski, M. S. and Brilliant, L. (2009). Detecting influenza epidemics using search engine query data. Nature. 457:1012–1014.
- Goetz, A. K., Singh, B. P., Battalora, M., Breier, J. M., Bailey, J. P., Chukwudebe, A. C. and Janus, E. R. (2011). Current and future use of genomics data in toxicology: Opportunities and challenges for regulatory applications. Regul. Toxicol. Pharmacol. 61:141–153.
- Goldberg, K., Roeder, T., Gupta, D. and Perkins, C. (2001). Eigentaste: A constant time collaborative filtering algorithm. Inf. Retriev. 4:133–151.
- HACCPEurope (2013). IBM Big Data Helps to Control Food Safety in Restaurant Chain.
- Hazeleger, W. (2015). Is Big Data a Big Deal? What Big Data Does to Science. Generale, W. S. (Ed.). Available at http://www.wur.nl/nl/activiteit/Is-Big-Data-a-Big-Deal-What-Big-Data-Does-To-Science-1.htm. Accessed 12 January 2016.
- Hendrickx, D. M., Boyles, R. R., Kleinjans, J. C. and Dearry, A. (2014). Workshop report: Identifying opportunities for global integration of toxicogenomics databases, 26-27 June 2013, Research Triangle Park, NC, USA. Arch. Toxicol. 88:2323–2332.
- Hu, Y. and Nie, L. (2016). An aerial image recognition framework using discrimination and redundancy quality measure. J. Visual Commun. Image Represent. 37:53–62.
- Huang, T., Lan, L., Fang, X., An, P., Min, J. and Wang, F. (2015). Promises and challenges of big data computing in health sciences. Big Data Res. 2:2–11.
- IBM (2012). Solutions Big Data IBM. Isabelle Claverie-Berge, I. A. (Ed.). http://www-05.ibm.com/fr/events/netezzaDM_2012/Solutions_Big_Data.pdf. Accessed 12 January 2016.
- Kim, S., Yu, Z., Kil, R. M. and Lee, M. (2015). Deep learning of support vector machines with class probability output networks. Neural Netw. 64:19–28.
- Klous, S. and Wielaard, N. (2016). Big, bigger, biggest data. In: We are Big Data: The Future of the Information Society, pp. 1–15. Atlantis Press, Paris.
- Kolesnikov, N., Hastings, E., Keays, M., Melnichuk, O., Tang, Y. A., Williams, E., Dylag, M., Kurbatova, N., Brandizi, M., Burdett, T., Megy, K., Pilicheva, E., Rustici, G., Tikhonov, A., Parkinson, H., Petryszak, R., Sarkans, U. and Brazma, A. (2015). ArrayExpress update–simplifying data submissions. Nucleic Acids Res. 43:D1113–1116.
- Konstan, J. A. and Riedl, J. (2012). Recommender systems: From algorithms to user experience. User Model. User-Adapt. Interact. 22:101–123.
- Koren, Y. (2008). Tutorial on recent progress in collaborative filtering. In: Proceedings of the 2008 ACM conference on Recommender systems. ACM, Lausanne, Switzerland.
- Kramer, K., Hengeveld, G. M., Schelhaas, M. J., Werf, D. C. v. d. and Winter, W. d. (2013). Genetic adaptive response: Missing issue in climate change assessment studies.
- Kupferschmidt, K. (2011). As E. coli outbreak recedes, new questions come to the fore. Science. 333:27.
- Li, B.-Q., Zhang, J., Huang, T., Zhang, L. and Cai, Y.-D. (2012). Identification of retinoblastoma related genes with shortest path in a protein–protein interaction network. Biochimie. 94:1910–1917.
- Li, Q., Xu, B., Ma, Y. and Chung, T. (2016). Real-time monitoring and forecast of active population density using mobile phone data. In: Big Data Technology and Applications: First National Conference, BDTA 2015, Harbin, China, December 25-26, 2015. Proceedings, pp. 116–129. Chen, W., Yin, G., Zhao, G., Han, Q., Jing, W., Sun, G., and Lu, Z. (Eds.), Springer Singapore, Singapore.
- Lienau, E. K., Strain, E., Wang, C., Zheng, J., Ottesen, A. R., Keys, C. E., Hammack, T. S., Musser, S. M., Brown, E. W., Allard, M. W., Cao, G., Meng, J. and Stones, R. (2011). Identification of a salmonellosis outbreak by means of molecular sequencing. New Engl. J. Med. 364:981–982.
- Lin, C.-H., Huang, L.-C., Chou, S.-C. T., Liu, C.-H., Cheng, H.-F. and Chiang, I.-J. (2016). Temporal event tracing on big healthcare data analytics. In: Big Data Applications and Use Cases, pp. 95–108. Hung, P. C. K. (Ed.), Springer International Publishing, Cham.
- Lin, W.-C. and Block, G. (2009). Neural network modeling to predict shelf life of greenhouse lettuce. Algorithms. 2:623.
- Linden, G., Smith, B. and York, J. (2003a). Amazon.com recommendations: Item-to-item collaborative filtering. Internet Comput., IEEE. 7:76–80.
- Linden, G., Smith, B. and York, J. (2003b). Amazon.com recommendations: Item-to-item collaborative filtering. IEEE Internet Comput. 7:76–80.
- Lindner, M., Suominen, T., Palosuo, T., Garcia-Gonzalo, J., Verweij, P., Zudin, S. and Päivinen, R. (2010). ToSIA-A tool for sustainability impact assessment of forest-wood-chains. Ecol. Model. 221:2197–2205.
- Linge, J. S., Steinberger, R., Weber, T. P., Yangarber, R., van der Goot, E., Al Khudhairy, D. H. and Stilianakis, N. I. (2009). Internet surveillance systems for early alerting of health threats. Eurosurveillance 14.
- Liu, J. and Yang, G.-Z. (2015). Robust speech recognition in reverberant environments by using an optimal synthetic room impulse response model. Speech Commun. 67:65–77.
- Lokers, R., Knapen, R., Janssen, S., van Randen, Y. and Jansen, J. (2016). Analysis of Big Data technologies for use in agro-environmental science. Environ. Model. Softw. 84:494–504.
- Marvin, H. J. P., Bouzembrak, Y., Janssen, E. M., van der Fels- Klerx, H. J., van Asselt, E. D. and Kleter, G. A. (2016). A holistic approach to food safety risks: Food fraud as an example. Food Res. Int. 89(Part 1):463–470.
- Meyer, C. H., Hamer, M., Terlau, W., Raithel, J. and Pongratz, P. (2015). Web data mining and social media analysis for better communication in food safety crises. Int. J. Food Syst. Dyn. 6:129–138.
- Miller, B. N., Albert, I., Lam, S. K., Konstan, J. A. and Riedl, J. (2003). MovieLens unplugged: experiences with an occasionally connected recommender system. In: Proceedings of the 8th International Conference on Intelligent user Interfaces. ACM, Miami, Florida, USA.
- Mishra, R., Kumar, P. and Bhasker, B. (2015). A web recommendation system considering sequential information. Decis. Support Syst. 75:1–10.
- Mkrtchyan, L., Podofillini, L. and Dang, V. N. (2015). Bayesian belief networks for human reliability analysis: A review of applications and gaps. Reliab. Eng. Syst. Safety. 139:1–16.
- Montavon, G., Orr, G., Müller, K.-R. and Hinton, G. (2012). A practical guide to training restricted boltzmann machines. In: Neural Networks: Tricks of the Trade, pp. 599–619. Springer Berlin, Heidelberg.
- Nabuurs, G. J., Schelhaas, M. J. and Pussinen, A. (2000). Validation of the European forest information scenario model (EFISCEN) and a projection of Finnish forests. Silva Fennica 34(2):167–179.
- Nakamura, A. and Abe, N. (1998). Collaborative filtering using weighted majority prediction algorithms. In: Proceedings of the Fifteenth International Conference on Machine Learning. Morgan Kaufmann Publishers Inc.
- Newkirk, R. W., Bender, J. B. and Hedberg, C. W. (2012). The potential capability of social media as a component of food safety and food terrorism surveillance systems. Foodborne Pathogens Dis. 9:120–124.
- Nsoesie, E. O., Kluberg, S. A. and Brownstein, J. S. (2014). Online reports of foodborne illness capture foods implicated in official foodborne outbreak reports. Prev. Med. 67:264–269.
- Obama Administration (2012). Obama Administration unveils “Big Data” initiative: announces $200 million in new R&D investments. Office of Science and Technology Policy Executive Office of the President.
- Oki, N. O., Nelms, M. D., Bell, S. M., Mortensen, H. M. and Edwards, S. W. (2016). Accelerating adverse outcome pathway development using publicly Available Data Sources. Curr. Environ. Health Rep. 3:53–63.
- Parikh, D. and Zitnick, C. L. (2011). Finding the weakest link in person detectors. In: Computer Vision and Pattern Recognition (CVPR), 2011 IEEE Conference on, 20-25 June 2011, pp. 1425–1432.
- Richterich, A. (2016). Using transactional big data for epidemiological surveillance: google flu trends and ethical implications of ‘infodemiology’. In: The Ethics of Biomedical Big Data, pp. 41–72. Mittelstadt, B. D. and Floridi, L. (Eds.), Springer International Publishing, Cham.
- Rijksoverheid (2015). http://www.rijksoverheid.nl/onderwerpen/doorbraakprojecten-met-ict/economische-groei-met-doorbraakprojecten-ict.
- Rortais, A., Belyaeva, J., Gemo, M., van der Goot, E. and Linge, J. P. (2010). MedISys: An early-warning system for the detection of (re-)emerging food- and feed-borne hazards. Food Res. Int. 43:1553–1556.
- Schumacker, R. and Tomek, S. (2013). R Fundamentals. In: Understanding Statistics Using R, pp. 1–10. Springer, New York.
- Shan, L. C., Panagiotopoulos, P., Regan, Á., De Brún, A., Barnett, J., Wall, P. and McConnon, Á. (2014). Interactive communication with the public: Qualitative exploration of the use of social media by food and health organizations. J. Nutr. Educ. Behav. 47:104–108.
- Steinberger, R., Pouliquen, B. and Goot, E. V. d. (2013). An introduction to the Europe Media Monitor family of applications. CoRR. abs/1309.5290.
- Strawn, L. K., Fortes, E. D., Bihn, E. A., Nightingale, K. K., Gröhn, Y. T., Worobo, R. W., Wiedmann, M. and Bergholz, P. W. (2013). Landscape and meteorological factors affecting prevalence of three food-borne pathogens in fruit and vegetable farms. Appl. Environ. Microbiol. 79:588–600.
- Suter-Dick, L., Pretot, R. F. and Chen, G. J. (2014). Molecular and in vitro Toxicology at the FHNW. Chimia (Aarau). 68:329–330.
- Ueti, R. d. M., Espinosa, D. F., Rafferty, L. and Hung, P. C. K. (2016). Case studies of government use of big data in Latin America: Brazil and Mexico. In: Big Data Applications and Use Cases, pp. 197–214. Hung, P. C. K. (Ed.), Springer International Publishing, Cham.
- Van den Puttelaar, J., Verain, M. C. D. and Onwezen, M. C. (2016). The potential of enriching food consumption data by use of consumer generated data: A case from RICHFIELDS. Proceedings of Measuring Behavior 2016.
- van der Fels-Klerx, H. J., Olesen, J. E., Madsen, M. S. and Goedhart, P. W. (2012). Climate change increases deoxynivalenol contamination of wheat in north-western Europe. Food Additives and Contaminants—Part A Chemistry, Analysis, Control, Exposure and Risk Assess. 29:1593–1604.
- Wang, Y., Yang, B., Luo, Y., He, J. and Tan, H. (2015). The application of big data mining in risk warning for food safety. Asian Agric. Res. 07:83–86.
- Ward, J. S. and Barker, A. (2013). Undefined by data: A survey of big data definitions. CoRR. abs/1309.5821.
- Wei, Q., Nagi, R., Sadeghi, K., Feng, S., Yan, E., Ki, S. J., Caire, R., Tseng, D. and Ozcan, A. (2014). Detection and spatial mapping of mercury contamination in water samples using a smart-phone. ACS Nano. 8:1121–1129.
- Whitehouse (2013). Data to Knowledge to Action: Event Highlights Innovative Collaborations to Benefit Americans https://www.whitehouse.gov/sites/default/files/microsites/ostp/Data2Action%20Press%20Release.pdf.
- WHO (2015a). FOSCOLLAB: Global platform for food safety data and information. http://www.who.int/foodsafety/foscollab_dashboards/en/.
- WHO (2015b). Global environment monitoring system - food contamination monitoring and assessment programme. GEMS/food. https://extranet.who.int/gemsfood/Default.aspx (Ed.).
- Xiao, M., Prabakaran, P., Chen, W., Kessing, B. and Dimitrov, D. S. (2013). Deep sequencing and Circos analyses of antibody libraries reveal antigen-driven selection of Ig VH genes during HIV-1 infection. Exp. Mol. Pathol. 95:357–363.
- Yiannas, F. (2015). How Walmart's SPARK Keeps Your Food Fresh. In: Walmart today. http://corporate.walmart.com/_blog_/sustainability/20150112/how-walmarts-spark-keeps-your-food-fresh.
- Zhu, H., Sikora, U. and Ozcan, A. (2012). Quantum dot enabled detection of Escherichia coli using a cell-phone. Analyst. 137:2541–2544.