Abstract
We use the Location Affordability Index (LAI) and the newly developed Child Opportunity Index (COI) to assess, for the first time, the tradeoff between neighborhood opportunity and housing/transportation affordability facing low-income renter families in the 100 largest metropolitan areas. In addition to describing the opportunity/affordability relationship, we explore the level of balance between neighborhoods’ relative cost burden and their corresponding opportunity levels to determine whether children of different racial/ethnic groups are more (or less) likely to experience cost-opportunity imbalance. Our multilevel analyses show that housing affordability is largely accounted for by the neighborhood opportunity structure within each metropolitan area. The metropolitan characteristics examined account for only a small proportion of the between-metro variance in the opportunity/affordability gradient for housing, presumably because the neighborhood opportunity structure already reflects metro area factors such as fragmentation and segregation. On the other hand, transportation affordability shows a weaker association with neighborhood opportunity. The COI/LAI association is much weaker for transportation than for housing, and a large part of the variation in the transportation gradient occurs at the metropolitan area level, not the neighborhood level. Sprawl is particularly associated with transportation affordability, with lower sprawl areas having lower transportation-cost burden. We discuss the implications of the empirical findings for defining affordability in housing assistance programs. We recommend that housing policy for low-income renter families adopt an expanded notion of affordability (housing, transportation, and opportunity) and explicitly consider equity (e.g. cost-opportunity imbalance) in the implementation of this expanded affordability definition.
This article examines the tradeoffs that low-income renter families face between affordability and neighborhood opportunity in the 100 largest U.S. metropolitan areas. How much more do we expect families to have to pay to live near good schools, quality child care, parks and green space, and in a favorable socioeconomic climate? Research has established that neighborhood contexts have a direct influence on child healthy development, emphasizing the importance of both housing unit quality and neighborhood quality for low-income families. Whereas past research suggests that lower cost neighborhoods are generally of lower quality, past studies measure just a single dimension of affordability (typically, rent levels), and a single dimension of neighborhood quality (e.g., poverty or crime). This article combines, for the first time, new multidimensional measures of both affordability and neighborhood quality. Together these measures move us beyond the relationship between neighborhood poverty rates and rent levels, to a more complete analysis of the tradeoffs between an expanded notion of neighborhood affordability (that includes both housing and transportation costs), and multiple dimensions of neighborhood opportunity (socioeconomic, educational, and health/environmental opportunity) that matter for children.
We also examine racial/ethnic differences in affordability/opportunity tradeoffs for low-income renter families. Black and Hispanic families, a growing share of low-income renter families, experience high levels of residential segregation from white families, and are more likely to be concentrated in lower opportunity neighborhoods (Acevedo-Garcia et al., Citation2014). Building on the vast evidence of limited access to fair housing opportunities for blacks and Hispanics (Ellen & Yager, Citation2015), we examine whether minorities also face higher costs than whites to access neighborhood opportunity.
Both lines of analysis have clear policy implications. The analysis of the tradeoff between opportunity and affordability can show whether prioritizing housing and/or transportation affordability in rental assistance for low-income renters may land families in lower opportunity neighborhoods. Second, the analysis of racial/ethnic inequities in opportunity/affordability tradeoffs can inform whether assessments of fair housing should consider neighborhood opportunity alongside affordability. Our analysis focuses on families with children—who represent about 35% of low-income households receiving rental housing assistance—because of the strength of the evidence that neighborhoods matter for child development (Chetty, Hendren, & Katz, Citation2015). Additionally, partly because of this evidence, a quality neighborhood environment for families with children is increasingly being recognized as an important objective of rental assistance. Finally, for fair housing purposes, not only race but family status (i.e., families with children under 18) is a protected class under federal law (Fair Housing Amendments Act of Citation1988).
The analysis includes all neighborhoods (census tracts) in the 100 largest U.S. metropolitan areas, and explores variation in affordability/opportunity tradeoffs both within and between metropolitan areas, using a multilevel analysis. We use the Location Affordability Index (LAI; U.S. Department of Housing and Urban Development, & U.S. Department of Transportation, Citation2014) to measure neighborhood affordability and the newly developed Child Opportunity Index (COI; Acevedo-Garcia et al., Citation2014) to capture the bundle of neighborhood amenities important for children and families to thrive. The LAI is unique in that it includes both housing and transportation costs, giving us a more comprehensive measure of how much a neighborhood costs a family. The COI combines 19 individual indicators across three domains of neighborhood opportunity—educational opportunity, health and environmental opportunity, and socioeconomic opportunity—offering a first-of-its-kind multidimensional measure of neighborhood factors most relevant for children and families.
We begin the analysis by examining the opportunity/affordability gradient—defined as the stepwise relationship between neighborhood opportunity level and neighborhood affordability level—to assess the tradeoffs faced by low-income renter families. We then explore racial/ethnic differences in the level of balance (or imbalance) between neighborhoods’ relative cost levels and their corresponding opportunity levels. Finally, we discuss the implications of our findings for defining affordability in housing assistance programs for low-income families.
The Neighborhood Opportunity/Affordability Tradeoff for Low-Income Families
Neighborhood quality is reflected in housing prices, but we do not know the extent of the opportunity/housing affordability tradeoff for low-income families with children. Housing is a bundled good: it includes the housing unit but also the amenities associated with its location. Previous research shows that neighborhood attributes are associated with housing prices in the expected direction—that is, after controlling for housing unit and other neighborhood variables, housing in neighborhoods with higher quality schools (more open space, more access to healthy food, or less air pollution) is more expensive (Beron, Hanson, Murdoch, & Thayer, Citation2004; Brasington & Haurin, Citation2006; Brown & Yarnell, Citation2013; Chiodo, Hernández-Murillo, & Owyang, Citation2010; Nguyen-Hoang & Yinger, Citation2011). For example, across the 100 largest metropolitan areas, housing costs are, on average, 2.4 times higher ($11,000 more per year) near a high-scoring public school than near a low-scoring public school. This has important implications for children in families that have affordability constraints, as they may be priced out of neighborhoods with amenities that matter for children (Ellen & Glied, Citation2015).
Increasing evidence suggests that neighborhood conditions matter for child development and later outcomes. For example, children who live in low-poverty neighborhoods from a young age (under age 13) have better outcomes, such as increased college attendance and income as adults, compared with children growing up in high-poverty neighborhoods (Chetty et al., Citation2015). However, as described above, community resources, such as better performing schools, often bring higher housing costs, as they are capitalized into housing prices (Rothwell, Citation2012). When faced with high housing-cost burdens, families are forced to make tradeoffs between paying for housing and paying for food, health care, and other resources vital to children (Smith & Child Health Impact Working Group, Citation2005). For example, housing affordability affects child enrichment expenditures in families with incomes at or below 200% of the poverty line (Newman & Holupka, Citation2014a, Citation2014b). Spending about 30% of family income on housing (compared with spending over 30%, or too little) is associated with better child cognitive achievement outcomes in reading and math (Newman & Holupka, Citation2014a, Citation2014b). Although we expect housing prices to be higher in higher opportunity areas (as those higher opportunities are capitalized into price), until now we have lacked systematic analysis of how affordability—that is, family housing-cost burden (defined as housing costs as a share of family income)—increases as neighborhood opportunity level increases. We offer the first comprehensive assessment of the opportunity/affordability tradeoff across the 100 largest metropolitan areas for low-income renter families, a population that is one of the main targets of rental housing assistance. For example, until now, there have been no comprehensive data on the prevalence of high-opportunity neighborhoods that have affordable housing-cost burdens for low-income families.
The present study makes three advancements to this area of research. First, it looks specifically at housing affordability, defined as a family’s housing-cost burden (rental costs as a percentage of income). Whereas past studies predominantly focus on housing prices, cost burden as a proportion of income is an indicator of the share of family resources dedicated to housing, and allows us to compare how this share varies for families of different income levels. Cost burden is also a more policy-relevant measure as it is the way affordability is defined in housing assistance. Second, whereas previous research has looked at a single aspect of neighborhood quality, like school quality or air pollution, the COI aims to capture the bundle of neighborhood attributes that matter for children. Finally, by disaggregating opportunity into three domains (socioeconomic, educational, and health and environmental opportunity), we examine the relative affordability of various dimensions of child neighborhood opportunity.
Little is known about the relationship between neighborhood quality and transportation costs. Whereas evidence strongly suggests that living in higher opportunity neighborhoods leads to higher housing costs for low-income families, the evidence on the tradeoff between neighborhood opportunity and transportation costs is limited. A study of the Low Income Housing Tax Credit (LIHTC) showed that by two opportunity indicators (neighborhood poverty rate and school district proficiency rate), LIHTC developments close (< 0.5 mile) to transit stations had worse opportunity levels than developments not close to transit (> 0.5 mile; Zuk & Carlton, Citation2015). An analysis of transportation costs in Chicago, Illinois, showed that whereas suburban areas have higher transportation costs than urban areas do, costs vary within suburbs, and many suburban locations are both high opportunity and have relatively affordable transportation costs. Therefore, the authors concluded that for siting of affordable housing, “there are significant options for maximizing opportunity while keeping transportation costs low” (Bernstein, Citation2011). They recognized, though, that other metropolitan areas may differ from Chicago in their transportation infrastructure and opportunity structure.
Transportation costs are increasingly a focus for policymakers and researchers concerned about location-related expenses. Recent federal policies (e.g., U.S. Department of Housing and Urban Development's Sustainable Communities Initiative) and the LAI have enlisted an expanded notion of affordability costs that considers an expanded cost of living in a place, which includes not only the housing costs, but the related transportation costs as well (U.S. Department of Housing and Urban Development, & U.S. Department of Transportation, Citation2014). This expanded definition of affordability will influence both federal and local housing policies in the years ahead. Therefore, it is important to examine whether applying this new definition of affordability may direct low-income families to neighborhoods with inadequate conditions and resources for children.
Racial/Ethnic Equity in Opportunity and Affordability
Despite steady declines over the past several decades, residential segregation between blacks and whites remains high, especially in large Northeastern and Midwestern cities with large black populations. Hispanic segregation, although lower, has been essentially unchanged since at least 1980 (Logan & Stults, Citation2011). Not only are the neighborhoods of blacks and Hispanics separate from those of whites, research has also indicated that racial/ethnic inequities in the quality of neighborhood environment are so staggering that they constitute a “difference of kind, not degree” (Sampson, Citation2012, p. 113). These differences in neighborhood quality persist even after accounting for families’ poverty status. On average, across the 100 largest metropolitan areas, relatively small proportions of poor white (22%) and poor Asian (29%) children live in very low-opportunity neighborhoods within their metropolitan areas, as measured by the COI. On the other hand, much larger proportions of poor Hispanic (45%) and poor black (57%) children live in very low-opportunity neighborhoods (authors’ calculations of data from diversitydatakids.org, Citation2014).
This article builds on prior evidence of large racial/ethnic inequities in children’s locational distribution across levels of neighborhood opportunity, and further examines whether minorities may pay more than whites to live in better quality neighborhoods. Evidence of excess cost burden for minorities has been shown in some metropolitan areas for households receiving Section 8 vouchers (Early, Citation2011). We combine the COI and the LAI to examine whether there is congruence between neighborhood opportunity level and neighborhood affordability level for low-income renter families, and whether such congruence varies by race/ethnicity.
The Role of Housing Policy in Promoting Affordability and Access to Opportunity
Addressing affordability has been the main focus of rental housing assistance programs for low-income households. However, fair housing calls for a critical examination of all housing programs to ensure that they also reduce segregation and exposure to poverty concentration for protected classes (U.S. Department of Housing and Urban Development, Citation2015a). More recently, another explicit objective of fair housing has been to ensure that protected classes (e.g., families with children) have residential choices that give them access to opportunity neighborhoods or community assets such as high-performing schools (U.S. Department of Housing and Urban Development, Citation2012, 2013). Barriers to housing opportunity include exclusionary zoning by municipal jurisdictions, which limits the availability of affordable rental housing, persistent housing discrimination, and lack of incentives for housing agencies to help families find housing in high-opportunity communities. Another major barrier is the set of incentives historically built into subsidized housing regulations and procedures. For example, to determine subsidies, the Section 8 program uses fair market rents for entire metropolitan areas, which are usually lower than rent levels in neighborhoods with better conditions and resources for children (Sard & Rice, Citation2014).
Whereas achieving fair housing objectives may require improving the supply of affordable housing in higher opportunity communities, in the last two decades concerns about environmental sustainability have created incentives for housing development near transit. There has also been a push for expanding the traditional measure of housing affordability, which includes only housing costs, to include transportation costs (Center for Neighborhood Technology, & Center for Transit-Oriented Development, Citation2006). Low-income and minority families are disproportionately concentrated in lower opportunity neighborhoods, which are often in or near the urban core and thus in closer proximity to transit. There is evidence that low-opportunity neighborhoods have more favorable sustainability indicators, such as closer proximity to transit, which implies that prioritizing sustainability may reinforce racial/ethnic and income residential segregation (Been et al., Citation2010).
Therefore, it is important to assess whether prioritizing housing affordability and transportation affordability for low-income families is compatible with the fair housing goal of promoting access to opportunity neighborhoods. This article offers an empirical examination of this question to inform policy discussions about the opportunity/affordability tradeoff.
Research Approach
Our analysis addresses two main research questions. First, what is the relationship between neighborhood affordability and neighborhood opportunity for low-income renter families? In this analysis, we consider:
• | Whether the opportunity/affordability relationship is different for three definitions of affordability: housing-cost burden alone, transportation-cost burden alone, and housing and transportation-cost burden combined; | ||||
• | Whether the opportunity/affordability relationship is different for four definitions of neighborhood opportunity: overall opportunity, socioeconomic opportunity, educational opportunity, and health/environmental opportunity; | ||||
• | Whether there is variation in the opportunity/affordability relationship across metropolitan areas, and if so, whether certain metro-area characteristics, such as higher levels of residential segregation, help explain between-metro area differences. |
The second research question is an equity question—that is, what is the distribution of the (poor) child population by race/ethnicity across levels of neighborhood opportunity/affordability? We examine whether different racial/ethnic groups face different degrees of balance (or imbalance) between the cost-burden level and the opportunity level of the neighborhoods where they live.
Levels of Analysis
We examine the relationship between child opportunity and locational affordability at the neighborhood (i.e., census tract) level across the 100 largest U.S. metropolitan areas (U.S. Office of Management and Budget Citation2009; metropolitan area definitions; approximately 46,200 neighborhoods for which LAI data were available), where over two thirds of U.S. children reside. Metropolitan areas approximate regional context, and are formally defined as core urban areas of at least 50,000 in population. They include the counties containing the core urban area as well as adjacent counties that have a high degree of social and economic integration with the urban core. Census tracts each contain about 4,000 people and 1,600 housing units (U.S. Census Bureau, Citation2014).
Data
Our analysis utilizes both the LAI, which provides neighborhood-level data on estimated housing-cost burden and transportation-cost burden for 942 core-based statistical areas and county-level data for nonmetropolitan counties, and the recently developed COI, which provides data on neighborhood-based conditions and resources for healthy child development for all neighborhoods in the 100 largest metropolitan areas (Acevedo-Garcia et al., Citation2014). We recognize that there are critiques of both the LAI and neighborhood opportunity indices. Whereas alternative definitions and measures can be considered, the focus of this article is not methodological. Our objective is to establish as a baseline the first comprehensive, multilevel analysis of the relation between neighborhood opportunity and locational affordability for low-income renter families in the 100 largest U.S. metropolitan areas. Both the LAI and the COI are relative measures. The LAI indicates the housing/transportation costs for a given neighborhood as a percentage of the median income for a representative household type in the metropolitan area. The COI indicates the relative position of a given neighborhood within the neighborhood opportunity distribution of its metropolitan area.
Location Affordability Index
The LAI (Version 2) was developed as part of an initiative by the federal Partnership for Sustainable Communities, composed of the Department of Housing and Urban Development, the Department of Transportation, and the Environmental Protection Agency (U.S. Department of Housing and Urban Development, & U.S. Department of Transportation, Citation2014). Because housing and transportation costs vary considerably within and across metropolitan areas, the LAI estimates block group-level housing/transportation costs. It provides estimates of the affordability of a given location defined as the percentage of a household’s income that would be dedicated to housing costs, transportation costs, and combined housing and transportation costs if a household was to live in that location.
The LAI does not measure the cost burden of households currently living in each location. Instead, the LAI is based on the predicted cost burden that a household of a particular composition would incur if it lived in a specified location. Using the characteristics (household size, income, and number of commuters) of households already living in each block group would lead to an index where most places would be characterized as relatively affordable, since people generally live in places they can afford. Therefore, the model is estimated for eight representative household profiles. These household profiles, defined in terms of number of family members, income, and number of commuters, are unique to each metro area (or rural county). Since the LAI is defined as housing/transportation costs as a proportion of household income, higher values of the index denote higher cost burden—that is, lower affordability. In the following, we briefly describe the content and methodology of the LAI (see U.S. Department of Housing and Urban Development, & U.S. Department of Transportation, Citation2014 for more detailed information and motivation behind empirical choices).
The LAI uses 18 demographic and structural characteristics of neighborhoods to predict housing and transportation costs. Most data are measured at the block group level, some at the tract level. Measures of housing costs, auto ownership, and transit use (endogenous variables) are modeled as a function of 18 exogenous predictors in a simultaneous equation model (SEM). The exogenous variables include demographic characteristics of households (e.g., household size, number of commuters, income) and variables describing spatial and economic neighborhood amenities, such as access to jobs and walkability.
After the SEM is run, parameter estimates from the SEM are used in combination with observed data to predict housing and transportation costsFootnote1 for each block group. For variables measuring structural neighborhood amenities, the observed values for each block group are multiplied with their respective coefficient estimates. Demographic variables characterizing renter or owner households, however, are held constant across block groups at eight specific sets of values that define eight household profiles. These profiles are defined in terms of household size, household income relative to the area median, and number of commuters (for example, a family of four with two commuters with a household income equal to the area median income, or a single-parent household with three members and one commuter and an income of 50% of the area median income). Predicted costs therefore vary across block groups because of variation in spatial and economic amenities, while the contribution of demographic variables characterizing household profiles is held constant across block groups at eight specific sets of values. In this manner, eight predicted cost estimates are obtained for each block group, one for each household profile. Finally, these household profile-specific cost estimates are divided by the metro area-wide income of each household profile.
The resulting index can be interpreted as the percentage of the specified household profile’s income that would be needed to cover housing, transportation, or combined costs if that household type were to live in the specified block group. So, for example, a household profile (such as single-parent family) with a housing LAI of 40% would be expected to pay 40% of their income for housing if they lived in the specified location.
For the purposes of the analysis in this article, LAI estimates at the census block group level were weighted up to census tracts based on the number of households in each block group, as per the LAI technical guidance.Footnote2 We used the LAI household type single-parent family renters (defined as having a household size of three, one commuter, and income at 50% of the metro area median) since this is the household type with the most policy relevance both in terms of affordability (e.g., rental housing assistance) and access to neighborhood opportunity (e.g., fair housing, housing mobility).
Although the LAI represents an advance over measuring housing affordability alone, it does have several shortcomings. Given our objective to assess the relationship between opportunity and affordability across metropolitan areas, an important limitation is that the LAI model has considerable unexplained variation—it accounts for 52.6%–68% of the variation observed across the country’s neighborhoods in housing costs, and 69.5%–80.1% in transportation costs. The unexplained variation likely results from several factors including (but not limited to) derivation of a significant number of the model input variables from the 2008–2012 American Community Survey, which has relatively high margins of error for block group-level estimates. For example, across all block groups, the average margin of error for selected monthly owner costs is 37% of the estimated cost level.
Although we recognize limitations to the LAI methodology (about which the reader can learn more in the Introduction to this special issue), for the purposes of our analysis, the main limitation noted above (i.e., unexplained variation) should not affect our ability to analyze aggregate patterns in the relationship between opportunity and affordability across metropolitan areas. Although there is large unexplained variation in housing/transportation costs per the LAI, to our knowledge there are no regional patterns (or metro outliers) in the extent of unexplained variation. Therefore, it is possible to model the relationship between the explained portion of affordability given by the LAI and our measure of neighborhood opportunity across the 100 largest metropolitan areas.
Child Opportunity Index
Child neighborhood opportunity is the context of neighborhood-based opportunities that influence children’s health and development. The COI is a measure of relative opportunity across all neighborhoods in a metropolitan area. Each neighborhood is assessed relative to the distribution of opportunity in the metropolitan area—that is, other neighborhoods in the same metropolitan area. We calculate the COI for all census tracts in the 100 largest metropolitan areas based on 19 indicators in three domains of neighborhood opportunity: socioeconomic opportunity, educational opportunity, and health and environmental opportunity (see Table ). All indicators are vetted for their relevance to child development based on extant empirical literature on neighborhood effects and/or conceptual frameworks of neighborhood influences on children. In addition to relevance, indicator selection is guided by data availability. Certain factors, such as exposure to crime and neighborhood violence, are not included because of a lack of comparable neighborhood-level data across metropolitan areas (Acevedo-Garcia et al., Citation2014).
Table 1. Opportunity indicators in the Child Opportunity Index.
The COI includes indicators of factors that may impede opportunity (e.g., high neighborhood poverty) or facilitate opportunity (e.g., presence of quality early childhood centers). The opportunity indicators are analyzed relative to other neighborhoods in the region through the use of z scores, which indicate how far and in what direction a particular indicator value deviates from its distribution’s mean, expressed in units of its distribution’s standard deviation. This allows neighborhood data to be measured based on their relative distance from the data average for the metro area.
The z scores for the 19 indicators are first averaged to create opportunity indices for each of the three domains, and then the domain indices are averaged to form the final overall opportunity index. The corresponding level of opportunity (very low, low, moderate, high, very high) is determined by sorting all neighborhoods into quintiles based on their opportunity-index scores. Very high-opportunity neighborhoods represent the top 20% of opportunity scores within the metropolitan area. Conversely, very low-opportunity neighborhoods represent the bottom 20% of opportunity scores (diversitydatakids.org & Kirwan Institute for the Study of Race & Ethnicity, Citation2014). Additional details on the methodology for the COI are available at diversitydatakids.org.Footnote3
Metropolitan Area Variables
Housing prices reflect local conditions such as neighborhood quality and transportation access, but also the characteristics of their metropolitan region (Jud & Winkler, Citation2002). Therefore, we analyze whether the relationship between neighborhood opportunity and locational affordability varies between metropolitan areas. We further examine whether variation between metro areas can be accounted for by four metropolitan area characteristics: municipal fragmentation, residential segregation between blacks and whites, residential segregation between Hispanics and whites, and sprawl.
We hypothesize that the extent of municipal fragmentation and the extent of residential segregation at the metropolitan area level are positively associated with the magnitude of the housing affordability gradient between low- and high-opportunity neighborhoods. Municipal fragmentation (and segregation) likely increase housing costs in higher opportunity areas. Municipal fragmentation is related to the fragmentation of school districts and school financing, which results in a link between school quality and housing prices along municipal boundaries (Loubert, Citation2005). Municipal fragmentation also facilitates the segmentation of the housing market because jurisdictions are able to enact exclusionary zoning regulations that limit housing density and consequently affordability. In turn, these zoning regulations price out low-income families and racial/ethnic minority families, which are more likely to be low-income and renters than are nonminority families. Thus, exclusionary zoning both increases housing price differentials and facilitates economic and racial/ethnic residential segregation (Pendall, Citation2000; Pendall, Puentes, & Martin, Citation2006; Rothwell, Citation2012; Rothwell & Massey, Citation2010).
In our analysis, fragmentation is measured using the Metropolitan Power Diffusion Index (MPDI), a univariate score based on 2007 Census of Governments individual government data, that measures the extent to which the provision and funding of 11 common public services are distributed across separate governments in a metro area (Miller, Citation2012). We also examined whether segregation, as proxied by the dissimilarity index, accounts for metro variation in the opportunity/affordability relationship. Residential segregation between blacks and whites is measured using the dissimilarity index and 2010 Census population estimates, with dissimilarity values below 60 denoting low-to-moderate segregation and values of 60 and above denoting high segregation (Massey & Denton, Citation1989). Segregation between Hispanics and whites (non-Hispanic) is also measured using the dissimilarity index, with values below 43 (the median value for the 100 largest metropolitian areas) denoting lower segregation and values of 43 and above denoting higher segregation.
At the metropolitan area level, transportation expenses are positively related to the degree of sprawl. Households in metropolitan regions with more sprawl spend more than 20% of their expenditures on transportation ($8,500 annually), whereas those in areas with less sprawl spend less than 17% ($5,500 annually; McCann, Citation2000). Therefore, we hypothesize that metro areas with lower levels of sprawl have lower transportation-cost burdens, and that the transportation affordability gradient between low- and high-opportunity neighborhoods is less pronounced in areas with less sprawl. Sprawl is measured with the Sprawl Index score, developed by the Metropolitan Research Center at the University of Utah, which incorporates four dimensions: (a) development density; (b) land-use mix; (c) activity centering; and (d) street accessibility (Ewing & Hamidi, Citation2014).Footnote4 Although the Sprawl Index as originally constructed assigns lower scores to more sprawling areas, to make our results more intuitive, we multiply the originally constructed score by −1 so that more sprawling metro areas have higher sprawl values.
In addition, we control for metropolitan region and population size, following previous research that found both variables to be associated with metropolitan neighborhood patterns such as residential segregation (Iceland & Sharp, Citation2013).
Analytic Methods
To answer our first research question—What is the relationship between neighborhood affordability and neighborhood opportunity for low-income renter families?—we examine the median cost burden for neighborhoods at each opportunity level for all neighborhoods in the 100 largest metro areas. We look separately at three definitions of affordability (housing (LAI-H), transportation (LAI-T), and housing and transportation combined (LAI)) and four definitions of neighborhood opportunity (overall, socioeconomic, educational, and health/environmental). We then calculate the COI–LAI gradient as the difference between the metro area median estimated cost burden for very low-opportunity neighborhoods and the median estimated cost burden for very high-opportunity neighborhoods. We next look at opportunity/affordability correlations by metropolitan area to get an initial sense of whether the association between opportunity and affordability varies by metro. The indication of strong between-metro variation from both the gradient and the correlation analyses motivates a multilevel analysis (neighborhoods nested in metro areas) to quantify the average relationship between neighborhood opportunity and affordability, as well as the extent of variation in this association across metro areas. Finally, we explore whether metro area characteristics (fragmentation, segregation and sprawl) may help explain observed between-metro area differences, and thus point to potential drivers to inform policy efforts. The multilevel analysis methods are described in more detail below and in the Appendix.
To answer the second research question—What is the distribution of the (poor) child population by race/ethnicity across levels of neighborhood opportunity/affordability?—we use a newly developed measure called the cost–opportunity balance score. The measure helps us assess whether a neighborhood’s cost level exceeds (or is below) its relative opportunity level (i.e., whether a neighborhood has a cost burden level that is higher (or lower) than its opportunity level would suggest). We then look at the distribution of children across neighborhoods to examine racial/ethnic differences in the degree of cost–opportunity balance (or imbalance) they experience—see Results for more details on the cost–opportunity balance score.
Multilevel Analysis
We conducted a two-level hierarchical linear regression model (Raudenbush & Bryk, Citation2002), of neighborhoods (census tracts) nested within metropolitan areas to predict LAI outcomes, using Stata I/C, version 13.1. A multilevel model accommodates variables operationalized at different levels (in this case, variables at the neighborhood and metropolitan levels). The model accounts for correlation in the outcome occurring within nested contexts, and adjusts standard errors accordingly. It can partition the variance at which the outcome occurs between the levels of analysis: either between metro areas, or within metro areas between neighborhoods. If a substantial variance operates at the higher (metropolitan) level, this would suggest that metropolitan-level characteristics have a strong influence on housing/transportation affordability at the neighborhood level.
We ran a series of models to address the following questions:
(1) | Does affordability (i.e., the average LAI) vary across metro areas? | ||||
(2) | Does the relationship (gradient) between neighborhood opportunity (COI) and affordability (LAI) vary across metro areas? | ||||
(3) | Does the neighborhood opportunity/affordability gradient vary according to metro-area characteristics such as fragmentation, segregation, and sprawl? |
The Appendix provides details on the multilevel model-building strategy, variable specifications, statistical equation, and model interpretation.
Results
Low-Income Renter Households Face High Cost Burdens Even in Low-Opportunity Neighborhoods
Using the LAI cost-burden data for single-parent renters,Footnote5 we calculated the median cost burden by neighborhood opportunity level for each metropolitan area, and on average across the 100 largest metropolitan areas. We examined housing-, transportation-, and combined-cost burdens separately for four measures of neighborhood opportunity (overall, socioeconomic, educational, and health and environmental).
We find not only a positive gradient between neighborhood opportunity and location affordability, but also that low-income renter families face high housing- and transportation-cost burdens at all levels of neighborhood opportunity. In other words, whereas higher opportunity neighborhoods are less affordable, even in very low-opportunity neighborhoods, the median estimated cost burden for low-income families is 40% of household income for housing and 26% for transportation (across the 100 largest metro areas), well above the recommended cost-burden thresholds of 30% for housing and 15% for transportation (see Table ).
Table 2. Median cost burden by neighborhood opportunity level for single-parent and median-income renter households.
Across opportunity levels, the estimated cost burden for low-income families is greater than that for middle-income familiesFootnote6 (see Table ). For middle-income families, estimated housing- and transportation-cost burdens at each opportunity level are lower than for low-income families. For middle-income families, the housing-cost burden at each opportunity level is below the recommended threshold of 30%, but the transportation-cost burden is about 5 percentage points higher than the threshold of 15%. Both the housing and transportation affordability/opportunity gradients are steeper for low-income families than for middle-income families. In other words, for low-income families, affordability decreases more rapidly as the neighborhood opportunity level increases.
The Gradient Between Child Neighborhood Opportunity and Housing/Transportation Affordability
Whereas cost burdens for low-income households are high for both housing and transportation, living in a higher opportunity (compared with lower opportunity) neighborhood is more expensive in terms of housing than it is in terms of transportation (see Table ). For low-income renter families, housing- and combined-cost burdens increase, linearly, from very low- to very high-opportunity neighborhoods. On average, across the 100 largest metro areas, this gradient is present for the three domains of neighborhood opportunity but it is slightly more pronounced for educational opportunity.
Table 3. Median cost burden by neighborhood opportunity level for three domains of the Child Opportunity Index for single-parent renter households.
Transportation-cost burden also shows a positive gradient with the educational domain of the COI. In contrast, the gradients for the socioeconomic and the health and environmental domains are essentially flat. We attribute this primarily to the effects of the proximity to employment component of the socioeconomic domain and the proximity to health providers component of the health and environmental domain, both of which lift the opportunity index scores of their respective domains in more dense urban cores, which are often locations which also have lower transportation-cost burdens.
There is considerable variation in the magnitude of the neighborhood opportunity/affordability gradient by metro areas. Summary measures of the gradient spread, including enumeration of the 10 steepest and 10 shallowest metropolitan area gradients, are presented in Table A1 in the Appendix. Across the 100 largest metropolitan areas, the median opportunity/transportation affordability gradient (2.3) is less pronounced than the median opportunity/housing affordability gradient (7.6). As an example, Miami, Florida has the highest opportunity/housing affordability gradient. A low-income renter family living in a very high-opportunity neighborhood in Miami will have an additional housing-cost burden of 19.1 percentage points compared with a family living in a very low-opportunity neighborhood (the respective cost burdens in very low- and very high-opportunity neighborhoods in Miami are 42.4% and 61.5%). In contrast, Springfield, Massachusetts, has the lowest opportunity/housing-affordability gradient with a difference of only 0.1 percentage points in housing-cost burden between its very low- and very high-opportunity neighborhoods (the respective cost burdens in very low- and very high-opportunity neighborhoods in Springfield are 40.8% and 40.9%).
Table A2 in the Appendix presents data on the neighborhood affordability/opportunity gradients for all of the 100 largest metropolitan areas for the overall COI and the three COI subdomains relative to overall, housing, and transportation affordability (LAI).
Variation Across Metropolitan Areas in the Opportunity/Affordability Gradient
We calculated the correlations between neighborhood opportunity (defined as each neighborhood’s COI expressed as a percentile of its metropolitan area COI distribution) and both housing and transportation affordability (using the LAI cost-burden measures) within each metropolitan area, to assess metropolitan variation in the strength of this correlation. The strength of the correlation between the COI and the LAI varies markedly across metropolitan areas. Figure shows box plots of the distribution of the correlation between the COI (overall, educational, socioeconomic, health and environmental) with the LAI across metropolitan areas. Figure a shows the correlation for housing LAI, and Figure b shows the distribution of correlations for transportation LAI. The color of the dot indicates the census region of each metro area.
Figure 1. Correlation of cost burden with the child opportunity index, 100 largest metropolitan areas. (A) Housing-cost burden. (B) Transportation-cost burden. Authors’ calculations based on data from: U.S. Department of Housing and Urban Development and Department of Transportation, Location Affordability Portal Version 2, 2014, and diversitydatakids.org and Kirwan Institute, Child Opportunity Index, 2014.
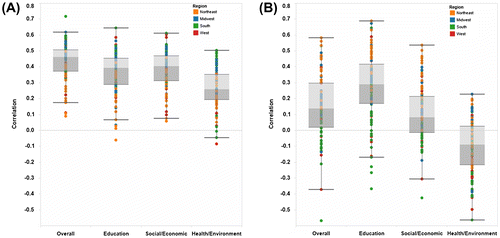
There is substantial variation in the strength of the correlation between opportunity and housing-cost burden across metropolitan areas, but this correlation is almost always positive. For example, the correlation between neighborhood opportunity and housing-cost burden ranges from 0.08 (Lancaster, Pennsylvania) to 0.72 (McAllen, Texas). Correlations with housing LAI are highest for educational and socioeconomic opportunity and lowest for health and environmental opportunity, indicating that educational and socioeconomic neighborhood conditions have a stronger association with housing costs than do neighborhood conditions related to health and environmental aspects (the respective median correlations are 0.39, 0.40, and 0.26).
The correlations between opportunity and transportation-cost burden are considerably lower, suggesting that transportation costs are less strongly associated with neighborhood opportunity. Correlations are highest for educational opportunity and lowest for health and environmental opportunity, with the median correlation for the latter being negative. Across all opportunity domains, correlations with transportation-cost burden are highest in Northeastern metro areas and lowest in Southern metro areas.
The substantial between-metro area variation in the correlation between neighborhood opportunity and affordability suggests the need for a multilevel analysis.
Multilevel Analysis
Tables and and Tables A3 and A4 in the Appendix show the results from multilevel models examining the association between neighborhood opportunity (COI) and affordability (LAI). We interpret the models below.
Table 4. Multilevel model predicting Location Affordability Index: Regression results for Models 1 through 4.
Table 5. Multilevel model predicting Location Affordability Index: Regression results for Model 5.
Affordability varies considerably between metropolitan areas, but there is vastly higher variation in transportation affordability than in housing affordability between metropolitan areas. Results from the random-effects model (Table A3) show that there is sizable, significant variation in affordability between metropolitan areas, indicated by a significant MSA-level random intercept ( =25.14, standard error (SE) = 3.63). We then found that 18.4% of the total variation in overall LAI occurs at the metro area level, with the remaining variance at the tract level (estimated by the intraclass correlation coefficient, ICC.Footnote7); however, the proportion of variance at the metro area level is much larger for transportation costs (ICC = 52.4%) than for housing costs (ICC = 6.1%).
Child neighborhood opportunity is strongly positively associated with affordability, especially housing affordability. As the multilevel regression results in Table show, whereas the COI coefficient is strongly significant and positive for all three measures of affordability, the opportunity/affordability gradient is much steeper for housing than for transportation. A one-quintile increase in the COI is associated with a 3.1-point change in the combined LAI and a 2.5-point change in LAI-H but only a 0.6-point change in LAI-T (see Model 1, Table ).
Housing affordability of high-opportunity neighborhoods varies more between metro areas than housing affordability of low-opportunity neighborhoods does.Footnote8 Although between-metro area variation is smaller for housing than for transportation affordability, for housing there is much more variation between metro areas in affordability for very high-opportunity neighborhoods than for very low opportunity-neighborhoods (Table A4). The percentage of variance at the MSA level for LAI-H is 4.4% in the lowest quintile of COI, but 18.5% in the highest quintile of COI (Model 2, LAI-H). This indicates that the opportunity structure of the metro accounts for more of the housing-cost variation in the lower part of the neighborhood opportunity distribution, but factors other than neighborhood opportunity drive cost variation in the higher end of the opportunity distribution. In contrast, the percentage of variance at the MSA level for transportation LAI is 59.7% in the lowest quintile of COI, but 50.2% in the highest quintile (Model 2, LAI-T).
Models 3 adds region and population size to COI in the model, which lowers the ICC and accounts for 3–5 percentage points of the MSA variance in LAI (Table A4, Model 3). However, the addition of these variables does not influence the COI gradient with any of the LAI outcomes (Table , Model 3).
We examined whether metropolitan factors (fragmentation and segregation for housing, and sprawl for transportation) are associated with affordability. First, we assessed whether metropolitan characteristics are associated with affordability levels across all neighborhoods—that is, whether, for example, higher segregation raises the housing-cost burden level across all neighborhoods in the metro (i.e., main effect of segregation). Second, we examined whether metropolitan characteristics are associated with the opportunity/affordability gradient—for example, whether in more fragmented metro areas, higher opportunity neighborhoods are even more unaffordable compared with lower opportunity neighborhoods (i.e., interaction effect between fragmentation and COI). Models 4a–d (Table and Table A4) add explanatory variables at the metro area level in separate models. Models 5a–d (see Table ) add an interaction effect between the explanatory variables at the metro-area level and the COI (again, with each metro-area variable modeled separately for models a through d).
Contrary to our expectation, metro areas with more fragmentation have lower housing-cost burdens, and segregation is not associated with housing-cost burden (the main effects, Table ). Also, contrary to our expectation in more fragmented/segregated metro areas, higher opportunity neighborhoods are not less affordable housing-wise than in less fragmented/segregated metro areas—there is not a significant interaction between fragmentation/segregation and the opportunity/affordability gradient (see Table ).
More sprawling metro areas have higher transportation-cost burdens. Sprawl is positively and significantly associated with LAI-T (main effect, Table ). Adding sprawl to the model reduces the share of variance between metro areas for LAI-T, lowering the ICC from 55.8% to 47.2% for the lowest COI quintile (compared with Model 3 without sprawl) and from 46.7% to 35.8% for the highest COI quintile (Table A4). We also found a sprawl/COI interaction (interaction, Table ), which indicates that in lower sprawl metro areas, the opportunity/affordability gradient for LAI-T is much larger than it is in higher sprawl areas.
Cost–Opportunity Balance by Race/Ethnicity
To assess issues of racial/ethnic equity, we examined the distribution of poor children by race/ethnicity across combined levels of neighborhood opportunity and affordability. We also calculated cost–opportunity balance scores by race/ethnicity. The cost–opportunity balance score measures the difference between a neighborhood’s cost-burden quintile and its opportunity quintile. Using these scores, we can see whether there are differences, on average, in cost–opportunity balance for neighborhoods where poor children of different racial/ethnic groups reside. The cost–opportunity balance score is calculated, for each census tract i, as follows:(1)
where the LAI and COI quintiles for tract i represent the quintile (1, 2…5) that the tract falls into based on the metropolitan-area distribution of LAI and COI values, respectively. The cost–opportunity balance score can range from −4 to + 4. A score of zero means that a neighborhood’s cost burden and opportunity quintiles are the same, indicating balance between the neighborhood’s relative cost level and its relative opportunity level. A positive cost–opportunity balance score indicates that a neighborhood’s cost quintile value is greater than its opportunity quintile value, suggesting an imbalance, in which the neighborhood’s cost level exceeds its opportunity level. A negative cost–opportunity balance score indicates the opposite—i.e., that a neighborhood’s cost quintile value is less than its opportunity quintile value, suggesting an imbalance where the opportunity level of the neighborhood is greater than its cost level.
Cost–opportunity balance scores characterize neighborhoods and can also be used to examine the extent to which different populations, such as children by race/ethnicity and poverty status, are exposed to neighborhoods with different cost–opportunity balance. These population-specific scores are created by averaging opportunity balance scores across all neighborhoods, weighted by the proportion of the population group of interest that resides in each neighborhood. For example, a score of 0.74 for poor black children would be interpreted as: on average, poor black children live in a neighborhood with a cost–opportunity balance score of 0.74.
Balance scores comparing housing-/transportation-cost burden and opportunity reveal substantial racial/ethnic differences. Whereas poor white children have a balance between housing-cost burden and opportunity level of their neighborhoods, poor black and Hispanic children are more likely to live in neighborhoods where housing-cost burdens are higher than the neighborhood opportunity level of the neighborhood would suggest (see Figure a). Poor white children have scores close to zero, indicating balance between the housing-cost level and opportunity level of their neighborhoods. On the other hand, poor black children experience the highest level of imbalance, with positive balance scores close to 1 (0.74), indicating that the housing-cost level of their neighborhoods is almost a full quintile above the opportunity level of their neighborhoods. In other words, poor black families live in neighborhoods where they are likely to overpay for housing relative to the opportunities for children those neighborhoods provide. Poor Hispanic children also have a positive balance score of 0.51, indicating that their families are also likely overpaying, albeit not as much as poor black families. Poor Asian children have a score of 0.33.
Figure 2. Cost–opportunity balance scores for poor children by race/ethnicity. (A) Housing-cost burden. (B) Transportation-cost burden. (C) Combined-cost burden. Authors’ calculations based on data from: U.S. Department of Housing and Urban Development and Department of Transportation, Location Affordability Portal Version 2, 2014, and diversitydatakids.org and Kirwan Institute, Child Opportunity Index, 2014. Child population below the federal poverty threshold from U.S. Census Bureau, 2007–2011 American Community Survey (U.S. Census Bureau, Citation2011).
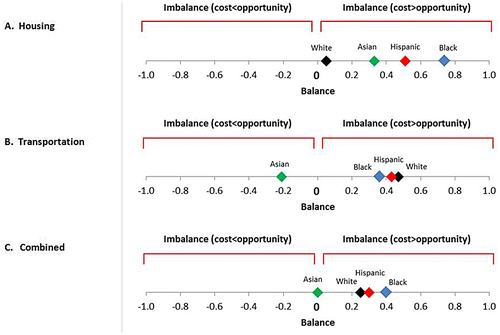
Balance scores comparing LAI-transportation-cost quintiles and opportunity quintiles tell a different story, although racial/ethnic differences are still present (see Figure b). Poor white, black, and Hispanic children experience positive balance scores around 0.40, suggesting that all three groups of children are experiencing imbalance in which their neighborhood transportation-cost burden level exceeds their neighborhood opportunity level. Poor Asian/Pacific Islander children have a negative balance score, suggesting a beneficial imbalance where the opportunity level in their neighborhoods is greater than the estimated transportation-cost burden level of their neighborhoods.
For poor black children, the cost–opportunity balance scores based on housing-cost burdens are more than twice the magnitude of the balance scores based on transportation-cost burdens. Scores for poor Hispanic children follow a similar pattern, although to a lesser extent. Poor Asian children also experience substantially lower (actually negative) cost–opportunity balance scores based on transportation costs as compared with scores based on housing costs. Meanwhile, poor white children experience a contrasting pattern where balance scores based on housing-cost burdens are close to zero (i.e., achieving cost–opportunity balance), but transportation cost-based balance scores are large and positive. This may reflect that poor white children are more likely than other poor children to live in suburban neighborhoods, which may be more transportation-costly.
In sum, poor black and Hispanic children are most likely to live in neighborhoods where the cost burdens exceed the neighborhood opportunity level (see Figure c). In contrast, white children achieve the most favorable cost–opportunity balance based on housing costs, but experience imbalance in transportation-based (and resulting combined) scores. Poor Asian children achieve the most favorable cost–opportunity balance based on housing/transportation combined scores, but experience contrasting patterns for housing-based and transportation-based measures, that net out to zero when combined.
Discussion
An Expanded Notion of Affordability Should Include Not Only Housing and Transportation Costs but Also Child Neighborhood Opportunity Costs
Our analysis shows a positive gradient between neighborhood opportunity and housing-cost burden, consistent with our hypothesis that neighborhood resources (and absence of stressors) have a strong association with higher housing costs. In contrast, transportation-cost burden shows a weaker association with neighborhood opportunity. Because housing costs represent a larger share of income than do transportation costs, the combined housing and transportation burden measure is dominated by the housing component, and exhibits a positive association between opportunity and cost burden, and thus a negative association between opportunity and affordability. Therefore, when considering housing and transportation costs combined, low-income families do face a tradeoff between location affordability and opportunity and are often limited to lower opportunity neighborhoods.
The LAI accounts for housing and transportation costs, an improvement over measures of housing affordability alone. However, the notion of affordability could be further enriched with the addition of an opportunity measure to more fully reflect the true costs of location for the wellbeing of children. Policies that rely on a definition of affordability that combines housing and transportation costs alone, such as the LAI, risk directing low-income families to low-opportunity neighborhoods, which may eventually result in poorer child outcomes. The costs of these poorer outcomes, such as worse child development and health and reduced earnings as adults, may not be readily apparent to families. These hidden costs may also be passed on to other sectors such as education and health.
Three features are needed for federal and state housing policies to promote better locational outcomes for families with children. First, the LAI or other measures of housing and transportation affordability could be combined with measures of child neighborhood opportunity (such as the COI or measures of school performance) to account for the full set of costs and benefits of living in a neighborhood, both in the near and longer terms. Second, housing subsidy levels need to increase because factoring in neighborhood opportunity will direct families to neighborhoods with more expensive housing. Third, the affordability threshold of 30% of household income currently used in housing policy should be combined with affordability thresholds for transportation and, eventually, for opportunity. Below, we discuss examples of policies that could be expanded to include the three features above.
Expanding the Notion of Affordability by Incorporating Child Neighborhood Opportunity Into the Objectives of Rental Housing Assistance
Consistent with an expanding notion of locational costs, researchers and policymakers have made a case for broadening our policy definitions of neighborhood quality to include opportunities for healthy child development (Acevedo-Garcia et al., Citation2014; Sard & Rice, Citation2014). Housing policy researchers increasingly agree that rental assistance programs should facilitate location in neighborhoods with better opportunities for children, but recognize the tradeoff between addressing affordability and improving location (Sard & Rice, Citation2014). An important question is whether and how the costs and benefits of child outcomes associated with children’s exposure to neighborhoods with more/fewer resources should be factored into definitions of affordability for low-income housing families. This article represents a first step in answering this question by contributing to our understanding of the relationship between neighborhood attributes that matter for children and housing/transportation affordability.
Developing a methodology to include child opportunity costs in a measure of affordability will likely require years of technical research, expert consultation, and stakeholder vetting. For example, an expanded measure of affordability may include the net present value of housing costs, the net present value of children’s projected lifetime earnings, and the net present value of savings in medical spending from improved health status associated with a given neighborhood location. In the meantime, though, child opportunity costs can be factored in by (a) integrating neighborhood-based opportunity for healthy child development into the locational objectives of low-income housing programs such as rental assistance and the LIHTC, (b) including measures of child opportunity in estimation of locational costs per measures such as the fair market rent, and (c) enhancing affordability thresholds to include transportation and opportunity.
Given the unaffordability of child neighborhood opportunity facing low-income renters, an objective of housing assistance programs for low-income families should be to address affordability for specific locational outcomes, such as neighborhoods with low poverty rates or high-performing schools. To date, however, with a few exceptions (e.g., Gautreaux, Moving to Opportunity, Thompson; Goering & Feins, Citation2003; Powell, Citation2005; Rosenbaum, Citation1995), access to high-opportunity neighborhoods has not been a major part of rental housing assistance. Some recent place-based federal programs (e.g., Promise Neighborhoods, Choice Neighborhoods), however, have a strong focus on improving educational opportunities for children, and about 15 states have begun adding points for locational opportunity in their Qualified Allocation Plans for assessment of LIHTC (Zuk & Carlton, Citation2015). The same principles could guide rental assistance. For example, better neighborhood outcomes per child-specific measures of opportunity such as school quality could be given more weight in assessing the performance of local housing agencies. Additionally, as described below, payment standards should facilitate opportunity moves.
Increasing Rental Subsidies to Allow Families to Afford Child Neighborhood Opportunity
Our analysis shows that location affordability is very limited for low-income families across opportunity levels, but even more so in high-opportunity neighborhoods. This suggests that in order to incentivize moves to higher opportunity neighborhoods, housing subsidies should be higher in those neighborhoods. HUD has recognized this issue and, as a result, is currently proposing the use of Small Area Fair Market Rents (SAFMR) that would increase subsidy levels for families that want to move to higher opportunity neighborhoods, defined as neighborhoods with low poverty rates. SAFMR would replace fair market rents defined for the entire metropolitan area and would be calculated as a ratio between the median rent in a target low-poverty neighborhood and the rate for the metropolitan area (capped at 150%). In other words, under SAFMR, a family attempting to rent in a high-opportunity neighborhood would have a higher subsidy than a family attempting to rent in a low-opportunity neighborhood. This is an improvement over the status quo which provides a maximum subsidy based on an average figure for the entire metro area, ignoring the large differences in opportunity and housing costs between neighborhoods within the same metro area. Evidence from HUD’s demonstration study suggests that SAFMR may facilitate moves to higher opportunity neighborhoods (U.S. Department of Housing and Urban Development, Citation2015b). Our analysis supports the use SAFMR instead of metro-level measures. Whereas HUD is presently using neighborhood poverty rates to establish SAFMR, conceptually an extension of their framework could include a composite measure of neighborhood opportunity such as the COI or a particular dimension of opportunity. Calculation of SAFMR could include not only housing (rents) but a multiplier that would reflect the small area child opportunity level. The COI could be enlisted as part of the definition of SAFMR, acting as an adjustment factor that would raise or lower rent levels to reflect small area-level opportunity. An alternative to using the entire COI to adjust SAFMR would be to use specific dimensions or indicators of opportunity to adjust FMR. Presently, HUD uses poverty rates. Conceptually and practically, a logical extension could be to use other dimensions of opportunity such as school quality to determine SAFMR. Our analysis indicates that the educational domain of neighborhood opportunity has an even stronger positive association with affordability than the socioeconomic domain does, which suggests that families may need higher subsidy levels to afford educational opportunity than to afford low-poverty neighborhoods. SAFMR could be different depending on household type since not all dimensions of opportunity are relevant for all households. For example, SAFMR determined based on school quality (or school quality and other dimensions of opportunity such as poverty rates) could be applied to housing subsidies for households with school-age children. School quality can be operationalized as the percentage of students with proficient scores (or large student growth) per state standardized testing for neighborhood schools. For example, for two neighborhoods with different educational opportunity levels (e.g., per the percentage of students proficient in standardized assessments in the neighborhood schools), the FMR for the neighborhood with better schools could be adjusted to be higher than that for the neighborhood with worse schools to provide an incentive to families to locate in neighborhoods with better educational opportunity.
In addition to improving cost-of-living measures such as the FMR to reflect opportunity costs, our analysis supports an enhancement of the 30% income standard for affordability to include transportation and, eventually, opportunity. Calls for enhancement are by no means new, beginning with Stone (Citation1993). Both the LAI and COI enlist the notion of area affordability from Fisher, Pollakowski, and Zabel (Citation2009). The current 30% of income standard assumes that this level of expenditure on housing leaves sufficient household income for other critical nonhousing needs such as food, clothing, transportation, childcare, and medical care (U.S. Department of Housing and Urban Development, Citation2012). A suggested affordability threshold for transportation is 15% of income. The LAI-T provides an estimate of the percentage of income a given type of household would be spending on transportation if they lived in that location. Therefore, when estimating the maximum household contribution toward rent (SAFMR) under a housing subsidy such as the Section 8 voucher, the household’s contribution could be capped at 45% (housing (30%) + transportation (15%)). The housing voucher would cover the difference between the FMR and 30% of the household’s income. Another subsidy could cover the difference between 15% of household income and the estimated transportation expenses for the location where the household would rent a unit—or the household’s contribution to the rent could be lower to keep the combined housing and transportation costs at less than 45% of household income.
Using an Expanded Definition of Affordability That Includes Child Neighborhood Opportunity Would Contribute to Further Fair Housing
Above and beyond the limited affordability and access to child neighborhood opportunity facing all low-income families, black and Hispanic families have additional challenges. As shown in this article, even after controlling for poverty status, black and Hispanic families both are more isolated from high neighborhood opportunity and also pay relatively more for neighborhood opportunity than do white families. At a minimum, assisted housing programs should ensure that families are not paying excessive costs relative to the neighborhood opportunity level. As our cost–opportunity balance measure analysis shows, many poor families are in neighborhoods where the housing costs are nearly a full quintile above the concomitant opportunity level. Ideally, housing assistance should allow families to afford high-opportunity neighborhoods that will enhance their life chances. Directing families to the best available neighborhoods (e.g., per school performance measures), given affordability constraints, would especially help black and Hispanic families, as these families tend to locate in neighborhoods where housing is too expensive relative to their opportunity level.
Therefore, identifying and correcting racial/ethnic inequity in neighborhood cost–opportunity balance should be added to the scope of efforts to affirmatively further fair housing (AFFH). Under HUD’s new AFFH rule and the new AFFH data-reporting requirements for housing jurisdictions, there is room to add the cost–opportunity balance score discussed in this article (or a similar measure) to the indicators used to assess segregation and unequal access to neighborhood opportunity. Our findings also emphasize the important role of housing search counseling in rental assistance, especially for black and Hispanic families who experience the highest imbalance between cost burden and neighborhood opportunity.
Future research should explore factors that may explain the observed racial/ethnic locational differences in the balance between neighborhood opportunity and neighborhood affordability. First, black and Hispanic families may be more likely to experience cost–opportunity imbalances than white and Asian families because of underlying racial discrimination in local housing markets. Second, familiarity with neighborhoods is an important aspect of housing decisions and has been shown to vary by race/ethnicity. For instance, black and Hispanic families may be less familiar with affordable higher opportunity neighborhoods, because of more geographically constrained information and social networks, which in turn are a result of longstanding patterns of residential segregation (Harris & McArdle, Citation2004; Krysan & Bader, Citation2009). Third, low-income families may base housing decisions more on the quality and fit of the housing unit itself (e.g., number of bedrooms relative to household size) than on the quality of the neighborhood (Rosenblatt & DeLuca, Citation2012). It therefore could be that black and Hispanic families are more likely to find units that meet their requirements in lower opportunity neighborhoods. However, the extent to which the unit requirements of black and Hispanic families differ from those of white and Asian families, and the extent to which there are shortages of units that meet the needs of black and Hispanic families in higher opportunity neighborhoods, is an understudied topic.
Policies Should Address Structural Metropolitan Factors Such as Sprawl Which Contribute to Lack of Affordability
In addition to examining the association between neighborhood opportunity and neighborhood housing/transportation costs, our analysis explored the influence of metropolitan-level factors on affordability at the neighborhood level. We find that housing affordability is largely accounted for by the neighborhood opportunity structure within each metro area, which is consistent with our hypothesis that neighborhood features have a strong association with housing costs. The metro area-level characteristics we examined in relation to housing-cost burden (i.e., metropolitan fragmentation and segregation) did not show the expected effects. A possible explanation is that the neighborhood opportunity structure already reflects metro-area patterns of fragmentation and segregation.
We found that metropolitan sprawl is positively associated with higher transportation-cost burdens. Unlike for housing, a large part of the variation in the opportunity/transportation affordability gradient occurs at the metropolitan-area level, not the neighborhood level, and sprawl accounts for a large part of the variation between metro areas in transportation affordability. This suggests that sprawl is a driver of transportation costs, and that policies that address metropolitan sprawl may contribute to improving affordability.
Conclusion
Based on our findings, we recommend that housing policymakers concerned about the wellbeing of low-income renter families adopt an expanded notion of affordability (housing, transportation, and opportunity) and explicitly consider equity (e.g., racial/ethnic segregation, cost–opportunity imbalance) in the implementation of this expanded affordability definition.
In addition to helping all children access higher opportunity neighborhoods, expanding the concept of affordability may help address racial/ethnic equity issues in neighborhood cost–opportunity balance. By making it explicit how much a family would pay to locate in a neighborhood with a given opportunity level, it would be possible to see if the cost (even if affordable according to established affordability thresholds) is congruent with the neighborhood opportunity (e.g., school quality) the family would attain. This would disproportionately benefit minority families, especially black and Hispanic families, who are more likely to live in neighborhoods with excessive cost burden relative to their opportunity level. In the future, HUD’s proposed assessment tool for AFFH (U.S. Department of Housing & Urban Development, Citation2013) could incorporate a cost–opportunity balance measure, by race/ethnicity, into the data they provide jurisdictions to identify segregation and poverty concentration patterns.
Housing unit and transportation costs are both critical to families’ locational decisions and have major implications for their ability to pay for other basic necessities. The LAI is a major step forward in reflecting the importance of both costs. However, when considered in isolation, it risks guiding families to neighborhoods with unmeasured or hidden costs which are no less real and which have profound consequences for children and their futures. Taking these neighborhood opportunity costs into account is the necessary next step in more fully measuring and reflecting true affordability.
Disclosure Statement
No potential conflict of interest was reported by the authors.
Funding
The research for this article was funded by the Robert Wood Johnson Foundation (Grant 71192); the W. K. Kellogg Foundation (Grant P3020864); the National Institute of Child Health and Human Development (Grant R03 HD080848).
Notes on Contributors
Dolores Acevedo-Garcia is a Samuel F and Rose B Gingold Professor of Human Development and Social Policy, and Director of the Institute for Child, Youth and Family Policy at the Heller School for Social Policy and Management, Brandeis University, Waltham, MA. Her research focuses on the social determinants (e.g., residential segregation, neighborhood inequality, immigrant adaptation) of racial/ethnic inequities in health, and the role of social policies (e.g., housing policies, immigrant policies) in reducing those inequities. She is the project director for diversitydatakids.org, a comprehensive database of indicators on child well-being and opportunity by race/ethnicity across multiple sectors and geographies. She received her master’s in Public Affairs and Urban and Regional Planning and PhD in Public Affairs with a concentration in demography from Princeton University.
Nancy McArdle is a senior research consultant with diversitydatakids.org and an independent researcher in Somerville, MA focusing on housing policy and demographics, racial segregation, and the intersection between civil rights and opportunity. She is a contributor to the books Twenty-First Century Color Lines: Multiracial Change in Contemporary Society and The Integration Debate: Competing Futures for American Cities and has been published in journals such as Health Affairs, Urban Affairs Review, and Social Science & Medicine. She received a master’s of Public Policy from Harvard University’s Kennedy School of Government.
Erin Hardy is a fellow at the Institute for Child, Youth and Family Policy at the Heller School for Social Policy and Management at Brandeis University, Waltham, MA. She is the research director for diversitydatakids.org, an indicator and policy research project focused on using data to advance child well-being and racial/ethnic equity. She is a co-principal investigator for a Child Care Research Partnership Grant evaluation study and for “Child-Focused Community Profiles of WKKF Priority Areas,” a data research project for the W K Kellogg Foundation. Her work focuses on connecting research in the areas of racial and ethnic stratification and child development, with particular emphasis on neighborhoods, early childhood, and spatial methods. She is also focused on the development of nationally comparable indicators related to child health equity. She earned a Master of Science in Political Science at MIT and a bachelor’s in Government and Economics at Wesleyan University.
Keri-Nicole Dillman is an independent evaluation and learning consultant in Boston, MA. She conducts mixed-method evaluation and applied research, with considerable focus on community-based and coalition-driven social change initiatives for community revitalization, public health, and health disparities eradication in our cities. Her evaluation work has included a focus on place-based initiatives such as the Robert Wood Johnson Foundation’s (RWJF) Urban Health Initiative and Invest Health Initiative, the MacArthur Foundation’s New Communities Program, and the Living Cities-funded Integration Initiative. Her work has been published in journals such as the Journal of Urban Health, the American Journal of Evaluation, and the Journal of Urban Affairs. She received her PhD in Public Administration from New York University.
Jason Reece is assistant professor of land use policy and law in the City & Regional Planning Program, Knowlton School of Architecture at the Ohio State University, Columbus, OH. Jason was formerly the senior associate director and director of research for the Kirwan Institute, an engaged research institute based at the Ohio State University. Jason has acted as an advisor and capacity builder to foundations, nonprofits, community organizations, and government agencies in more than 30 states on topics of community development, fair housing, social equity in planning, and health equity. From 2010 to 2014, he was a capacity builder for the U.S. Department of Housing and Urban Development (HUD), supporting more than 70 HUD sustainable communities’ grantees across the nation. His research focuses on fair housing, asset-based community development and models to further social and racial equity in planning policy and law. Jason received his PhD in City & Regional Planning and MCRP from The Ohio State University.
Unda Ioana Crisan is a research associate at Human Services Research Institute in Cambridge, MA. She analyzes aggregated state-wide health care data and develops data quality standards. She also provides data management and quantitative research support for projects focused on racial/ethnic equity in child well-being. She received her bachelor’s degree in psychology from West University of Timisoara, Romania, and her master’s degree in Community Social Psychology from the University of Massachusetts Lowell.
David Norris is a senior researcher at the Kirwan Institute for the Study of Race and Ethnicity at the Ohio State University, Columbus, OH. His research focuses on the uneven geographic distribution of social determinants of health and the racial/ethnic health inequities that result. He manages Kirwan's Health Equity Mapping projects. He received his bachelor's in Zoology from Ohio University and master's in Public Policy and Management from the John Glenn College of Public Affairs at the Ohio State University.
Theresa L Osypuk, SD, SM, is an associate professor in the Division of Epidemiology and Community Health, at the University of Minnesota School of Public Health, Minneapolis, MN. She is a social epidemiologist, and her research examines why place and social policy influence health and health disparities. She studies the influence of racial residential segregation, neighborhood context, and social and economic policies implemented outside of the health sector, for their effects on health disparities across the life course. She is currently the principal investigator of several National Institutes of Health grants investigating how Housing Choice Vouchers and neighborhood context influence the health of families. She earned her master’s and doctoral degrees from Harvard School of Public Health, and trained as a Robert Wood Johnson Foundation Health and Society Scholar at the University of Michigan.
Notes
1. In addition to the SEM, an auxiliary analysis was used to model vehicle miles traveled, for which only data from two metro areas were available. Estimates from this auxiliary regression were used in combination with the estimates related to transportation in the SEM and information on the costs of public transit and car use to calculate the transportation cost component of the LAI.
2. For purpose of this analysis, we define neighborhoods using census tracts, and both affordability and opportunity are measured and compared at this geographic scale throughout. Using census tracts to represent neighborhoods is a widely accepted standard in social science research. Whereas LAI data are available at smaller levels of geography (i.e., census block groups), this analysis requires that affordability and opportunity be compared at the same level of geography. For both practical and conceptual reasons, the census tract was deemed to be the preferred geographic unit of analysis. When interpreting results, note that affordability and opportunity measures represent the average levels of affordability/opportunity across entire tracts, and are not meant to speak to patterns of affordability and opportunity at smaller levels of geography within census tracts, such as census block groups.
3. The COI data and maps—as well as child population overlays by race/ethnicity—for the 100 largest metropolitan areas are available from diversitydatakids.org (diversitydatakids.org, Citation2014). Detailed technical documentation on the COI is available at http://www.diversitydatakids.org/files/CHILDOI/DOCS/DDK_KIRWAN_CHILDOI_OVERVIEW.pdf.
4. The original index was calculated for 221 metro areas and has an average index value of 100, meaning that areas with scores higher than 100 tend to be more compact and connected and areas with scores lower than 100 are more sprawling. Note that for all analysis, we multiply the original index by −1, so that high values denote more sprawl. Of the 100 large metro areas analyzed in this article, sprawl data were not available for four metro areas: Boston, Springfield, and Worcester, Massachusetts, and Honolulu, Hawaii. Therefore models incorporating sprawl as a predictor are based on only 96 metro areas. Further, we computed aggregated indices (based on population weights) for each of 10 large metropolitan areas for which sprawl indices were originally computed only for their component metropolitan divisions.
5. LAI analysis was weighted by the number of households in the neighborhood.
6. Middle-income families are defined based on the LAI-defined housing type Median Income Family, modeled as having four household members, two commuters, and income equal to the median for the metro area.
7. We calculated the ICC from the multilevel variance components to determine the proportion of variance in LAI operating at the metro area level, as opposed to at the neighborhood level. The ICC is a measure of contextual variation that theoretically ranges from 0% to 100%, although in organizational and educational research it typically ranges from 5% to 20%.
8. When we allow the slope between the COI and LAI to vary across metro areas (random-slope model), we find the slope variance is significant in all models (not shown), which indicates (along with the covariance) that the variation in LAI increases at higher values of COI.
References
- Acevedo-Garcia, D., McArdle, N., Hardy, E. F., Crisan, U. I., Romano, B., Norris, D., … Reece, J. (2014). The child opportunity index: Improving collaboration between community development and public health. Health Affairs, 33, 1948–1957. doi:10.1377/hlthaff.2014.0679.
- Been, V., Cunningham, M., Ellen, I. G., Gordon, A., Parilla, J., Turner, M. A., & Zimmerman, K. (2010). Building environmentally sustainable communities: A framework for inclusivity. Washington, DC: Furman Center, Urban Institute, What Works Collaborative.
- Bernstein, S. (2011). Transportation and fair housing part 2: Consider transportation cost to make fair housing practical. Shelterforce, 165. Retrieved from http://www.shelterforce.org/article/2175/transportation_and_fair_housing_part_2_consider_transportation_cost_to_make/P0/
- Beron, K. J., Hanson, Y., Murdoch, J. C., & Thayer, M. A. (2004). Hedonic price functions and spatial dependence: Implications for the demand for urban air quality. In L. Anselin, R. Florax & S. J. Rey (Eds.), Advances in spatial econometrics: Methodology, tools and applications (pp. 267–281). Berlin: Springer-Verlag.10.1007/978-3-662-05617-2
- Brasington, D., & Haurin, D. R. (2006). Educational outcomes and house values: A test of the value added approach. Journal of Regional Science, 46, 245–268. 10.1111/jors.2006.46.issue-2
- Brown, H. S., & Yarnell, L. M. (2013). The price of access: Capitalization of neighborhood contextual factors. International Journal of Behavioral Nutrition and Physical Activity, 10, 95–95. doi:10.1186/1479-5868-10-95.
- Center for Neighborhood Technology, & Center for Transit-Oriented Development. (2006). The affordability index: A new tool for measuring the true affordability of a housing choice. In Urban Markets Initiative, Market Innovation Brief. Washington, DC: Brookings Institution, Metropolitan Policy Program. Retrieved from: http://www.brookings.edu/research/reports/2006/01/01-affordability-index.
- Chetty, R., Hendren, N., & Katz, L. F. (2015). The effects of exposure to better neighborhoods on children: New evidence from the Moving to Opportunity experiment. Cambridge, MA: Harvard University and NBER. 10.3386/w21156
- Chiodo, A. J., Hernández-Murillo, R., & Owyang, M. T. (2010). Nonlinear effects of school quality on house prices. Federal Reserve Bank of St. Louis Review, 92, 185–204.
- diversitydatakids.org (2014). Waltham, MA: diversitydatakids.org, Institute for Child, Youth and Family Policy, Heller School for Social Policy & Management, Brandeis University. Retrieved on April 4, 2016 from http://www.diversitydatakids.org
- diversitydatakids.org, & Kirwan Institute for the Study of Race and Ethnicity. (2014). Child opportunity index mapping. Waltham, MA: Brandeis University.
- diversitydatakids.org, Institute for Child, Youth and Family Policy, Heller School for Social Policy & Management, Brandeis University. 2016. Retrieved on April 4, 2016 from http://www.diversitydatakids.org/files/CHILDOI/DOCS/DDK_KIRWAN_CHILDOI_OVERVIEW.pdf
- Early, D. W. (2011). Racial and ethnic disparities in rents of constant quality units in the Housing Choice Voucher program: Evidence from HUD’s customer satisfaction survey assisted housing research cadre report. Washington, DC: U.S. Department of Housing and Urban Development.
- Ellen, I. G., & Glied, S. (2015). Housing, neighborhoods, and children’s health. The Future of Children, 25, 135–153.
- Ellen, I. G., & Yager, J. (2015). Race, poverty, and federal rental housing policy. In Ross, L.M. (Ed.), HUD at 50: Creating Pathways to Opportunity (pp. 103–132). Washington, DC: Department of Housing and Urban Development.
- Ewing, R., & Hamidi, S. (2014). Measuring urban sprawl and validating sprawl measures. Salt Lake City, UT: Metropolitan Research Center, University of Utah. Prepared for the National Cancer Institute, National Institutes of Health, Ford Foundation, Smart Growth America.
- Fair Housing Amendments Act of 1988. (1988). 42 § 3601 et seq
- Fisher, L., Pollakowski, H., & Zabel, J. (2009). Amenity-based housing affordability indexes. Real Estate Economics, 37, 705–746. 10.1111/reec.2009.37.issue-4
- Goering, J., & Feins, J. D. (Eds.) (2003). Choosing a better life? Evaluating the Moving to Opportunity social experiment. Washington, DC: The Urban Institute Press.
- Harris, D. J., & McArdle, N. (2004). More than money: The spatial mismatch between where homeowners of color in metro boston can aford to live and where they actually reside. Cambridge, MA: The Civil Rights Project at Harvard University.
- Hox, J. (2002). Multilevel analysis: Techniques and applications. Mahwah, NJ: Lawrence Erlbaum Associates.
- Iceland, J., & Sharp, G. (2013). White residential segregation in U.S. metropolitan areas: Conceptual issues, patterns, and trends from the U.S. census, 1980 to 2010. Population Research and Policy Review, 32, 663-686. doi: 10.1007/s11113-013-9277-6
- Jud, G. D., & Winkler, D. T. (2002). The dynamics of metropolitan housing prices. Journal of Real Estate Research, 23, 29–45.
- Krysan, M., & Bader, M. D. (2009). Racial blind spots: Black-white-Latino differences in community knowledge. Social Problems, 56, 677–701. 10.1525/sp.2009.56.4.677
- Logan, J. R., & Stults, B. J. (2011). The persistence of segregation in the metropolis: New findings from the 2010 census, census brief prepared for project US2010 U.S. 2010: Discover America in a new century: American communities project. New York, NY: Russell Sage Foundation.
- Loubert, L. (2005). Housing markets and school financing. Journal of Education Finance, 30, 412–429.
- Massey, D. S., & Denton, N. A. (1989). Hypersegregation in U.S. metropolitan areas: Black and Hispanic segregation along five dimensions. Demography, 26, 373–391. 10.2307/2061599
- McCann, B. (2000). Driven to spend: The impact of sprawl on household transportation expenses. Washington, DC: Surface Transportation Policy Project.
- Miller, D. Y. (2012). Methodological framework for the metropolitan power diffusion index. In Research Note. Pittsburgh, PA: University of Pittsburgh Graduate School of Public and International Affairs, Center for Metropolitan Studies. Retrieved from: https://www.metrostudies.pitt.edu/Projects/Metropolitan-Power-Diffusion-Index
- Newman, S. J., & Holupka, C. S. (2014a). Housing affordability and child well-being. Housing Policy Debate, 25, 116–151. doi:10.1080/10511482.2014.899261
- Newman, S. J., & Holupka, C. S. (2014b). Housing affordability and investments in children. Journal of Housing Economics, 24, 89–100. doi:10.1016/j.jhe.2013.11.006.
- Nguyen-Hoang, P., & Yinger, J. (2011). The capitalization of school quality into house values: A review. Journal of Housing Economics, 20, 30–48. 10.1016/j.jhe.2011.02.001
- Pendall, R. (2000). Local land use regulation and the chain of exclusion. Journal of the American Planning Association, 66, 125–142. 10.1080/01944360008976094
- Pendall, R., Puentes, R., & Martin, J. (2006). From traditional to reformed: A review of the land use regulations in the nation’s 50 largest metropolitan areas (pp. 39). Washington, DC: The Brookings Institution, Metropolitan Policy Program.
- Powell, J. (2005). Expert remedial report in Thompson v. HUD. Columbus, OH: Kirwan Institute for the Study of Race & Ethnicity.
- Raudenbush, S., & Bryk, A. (2002). Hierarchical linear models: Applications and data analysis methods. Thousand Oaks: Sage Publications.
- Rosenbaum, J. E. (1995). Changing the geography of opportunity by expanding residential choice: Lessons from the Gautreaux program. Housing Policy Debate, 6, 231–269. 10.1080/10511482.1995.9521186
- Rosenblatt, P., & DeLuca, S. D. (2012). “We don’t live outside, we live in here”: Neighborhoods and residential mobility decisions among low-income families. City and Community, 11, 254–284. 10.1111/cico.2012.11.issue-3
- Rothwell, J. T. (2012). Housing costs, zoning, and access to high-scoring schools. Washington, DC: Brookings Institution, Metropolitan Policy Program.
- Rothwell, J. T., & Massey, D. S. (2010). Density zoning and class segregation in U.S. metropolitan areas. Social Science Quarterly, 91, 1123–1143. doi:10.1111/j.1540-6237.2010.00724.x.
- Sampson, R. J. (2012). Great American city. Chicago, IL: University of Chicago Press. 10.7208/chicago/9780226733883.001.0001
- Sard, B., & Rice, D. (2014). Creating opportunity for children: How housing location can make a difference. Washington, DC: Center on Budget and Policy Priorities.
- Smith, L. A., & Child Health Impact Working Group (2005). Affordable housing and child health– A child health impact assessment of the Massachusetts rental voucher program. Boston, MA: Boston Medical Center.
- Stone, M. (1993). Shelter poverty: New ideas on housing affordability. Philadelphia, PA: Temple University Press.
- U.S. Census Bureau. (2010). Census 2010 summary file 1 (SF1), Table P1, total population. Retrieved 10 June 2015 from U.S. Census http://factfinder.census.gov.
- U.S. Census Bureau. (2011). American community survey, 5 year estimates. Tables B17001B, B17001D, B17001H, B17001I, poverty in the last 12 months by sex by age. Retrieved 18 June 2014 from U.S. Census http://factfinder.census.gov.
- U.S. Census Bureau. (2014). 2010 geographic terms and concepts. Retrieved from https://www.census.gov/geo/reference/gtc/gtc_ct.html
- U.S. Department of Housing and Urban Development. (2012). Program policy guidance OSHC-2012-2013. Fair housing equity assessment. Retrieved from http://portal.hud.gov/hudportal/documents/huddoc?id=OSHC2012-03FHEAss.pdf.
- U.S. Department of Housing and Urban Development. (2012). Affordable housing. Washington, DC: Author.
- U.S. Department of Housing and Urban Development. (2013). Affirmatively furthering fair housing data documentation. Retrieved from http://www.huduser.org/portal/publications/pdf/FR-5173-P-01_AFFH_data_documentation.pdf
- U.S. Department of Housing and Urban Development. (2015a). Affirmatively furthering fair housing (final rule). Federal Register, 80, 42272–42371.
- U.S. Department of Housing and Urban Development. (2015b). Establishing a more effective fair market rent (FMR) system; using small area fair market rents (SAFMRs) in Housing Choice Voucher program instead of the current 50th percentile FMRs. Advanced Notice of Proposed Rulemaking Federal Register, 80, 31332–31336.
- U.S. Office of Management and Budget. (2009). Update of statistical area definitions and guidance on their uses. OMB Bulletin, No. 10-12. Retrieved from https://www.whitehouse.gov/sites/default/files/omb/assets/bulletins/b10-02.pdf
- U.S. Department of Housing and Urban Development, & U.S. Department of Transportation. (2014). The location affordability portal version 2. Retrieved from http://www.locationaffordability.info/default.aspx
- Zuk, M., & Carlton, I. (2015). Equitable transit oriented development: Examining the progress and continued challenges of developing affordable housing in opportunity and transit-rich neighborhoods. Washington, DC: Poverty & Race Research Action Council.
Appendix A.
Multilevel Model Methodology
We conducted a two-level hierarchical linear regression model (Raudenbush & Bryk, Citation2002), of neighborhoods (census tracts) nested within metropolitan areas to predict Location Affordability Index (LAI) outcomes, using Stata I/C, version 13.1.
After presenting a null random-intercept model with no covariates to determine the proportion of variance in LAI operating at the metro area level, our model-building is as follows:
(1) | A random intercept model, adding Child Opportunity Index (COI) as a predictor in the fixed portion of the model only, but allowing the LAI intercept to vary across metro areas; | ||||
(2) | A random slope model, with COI as the predictor of interest, allowing the COI/LAI slope to be random (varying) across metro areas; | ||||
(3) | Building on Model 2, we introduce controls for metro area region and population size; | ||||
(4) | Building on Model 3, we introduce four metropolitan-level predictors (one by one): black–white segregation, Hispanic–white segregation, municipal fragmentation and sprawl; | ||||
(5) | Building on each sub-model in Model 4, we test cross-level interactions between the COI and the metropolitan-level predictor. |
In each case the dependent variable was the LAI for single-parent family renters. Models were run separately for the LAI, LAI-H, and LAI-T. The COI was entered as an ordinal variable, modeled linearly. COI values were ordered from 0 to 4, representing each of the COI quintiles (very low-opportunity to very high-opportunity) within each metro area. Regions were the four primary census regions, with the Northeast as the omitted variable. Metro-area population size was taken from the 2010 Decennial Census (U.S. Census Bureau, Citation2010), measured in thousands of people. The remaining metropolitan area predictors (segregation, sprawl, and fragmentation) are described in the body of the article.
The multilevel statistical model for our most complex model, Model 5, the cross level interaction, is:(1)
We adopt the notation by Hox (Citation2002), where indicates the outcome, LAI, which varies by tract (denoted by subscript i) and metro area (subscript j).
indicates the tract-level COI variable, modeled ordinally from 0 to 4 for each quintile, again varying by tract and metro. Metro-level variables are denoted by Z, numbered sequentially and subscripted by j but not i, since they only vary at the metro level. For example,
indicates the metro-level variable for black–white dissimilarity (segregation),
indicates the metro population size, and
and
indicate the three contrast-coded regional variables for West, South, and Midwest, respectively (where Northeast is omitted). Gamma (
) indicates the regression-derived fixed-effect coefficient for the average relationship between each predictor variable and
, with Gamma’s first nonzero subscript number indicating a coefficient associated with the tract level, the second nonzero subscript number indicating a coefficient associated with the metro level, and both nonzero subscript numbers indicating a cross-level interaction (e.g.,
denotes the cross-level interaction coefficient for tract-level COI with metro-level black–white dissimilarity).
represents the constant, or the fixed intercept, interpreted as the grand-mean average LAI when all predictor variables in the equation are set to zero.
indicates the metro level random effects:
indicates the metro level intercept variance, which allows each metro area to have a different mean value of LAI, and
indicates the slope variance associated with COI, which allows the association of COI with LAI to vary differently for each metro area. These metro-level random effects are assumed to be normally distributed with mean of zero, and uncorrelated with the tract-level (residual) variance, represented by
.
Table A1.
Metropolitan areas with the highest and lowest affordability gradient between very low-opportunity neighborhoods and very high-opportunity neighborhoods, for single-parent renter households, 100 largest metropolitan areas.
Note.
VL COI = very low-opportunity neighborhoods; VH COI = very high-opportunity neighborhoods; Grad = gradient (difference) between very low- and very high-opportunity neighborhoods.The Child Opportunity Index–Location Affordability Index gradient is calculated as the difference between the metro area median estimated cost burden for very low-opportunity neighborhoods and the median estimated cost burden for very high-opportunity neighborhoods. Estimated cost burdens at the 25th, 50th, and 75th percentile across the metro-level values for the 100 largest metro areas were obtained after weighting by number of households of any type in metro areas. Table rows for top 10 and bottom 10 metro areas are sorted by the gradient value. Metro area names are abbreviated to include first named city only; see Appendix Table 3 for complete name.
Authors’ calculations based on data from: U.S. Department of Housing and Urban Development and Department of Transportation, Location Affordability Portal Version 2, 2014, and diversitydatakids.org and Kirwan Institute, Child Opportunity Index, 2014.
Table A2.
Affordability gradient between very low-opportunity neighborhoods and very high-opportunity neighborhoods, single-parent renter households, 100 largest metropolitan areas (sorted high to low by gradient between Child Opportunity Index and Location Affordability Index).
Note.
The COI–LAI gradient is calculated as the difference between the metro area median estimated cost burden for very low-opportunity neighborhoods and the median estimated cost burden for very high-opportunity neighborhoods. Median estimated cost burden within a metro area was obtained after weighting by number of households of any type in tracts. Estimated cost burden at the 25th, 50th, and 75th percentiles across the metro-level values for the 100 largest metro areas were obtained after weighting by number of households of any type in metro areas. For a description of the measures included in the overall COI and the component domains, please consult the COI technical documentation available here. LAI rows display information on the gradient based on the combined-cost burden (housing- and transportation-cost burden); values in the LAI-H rows are based on housing-cost burden only; values in the LAI-T rows are based on transportation-cost burden only. Metropolitan areas are sorted by the gradient value calculated for the combined LAI, between the very low- and very high-opportunity neighborhoods on the overall COI.
Authors’ calculations based on data from: U.S. Department of Housing and Urban Development and Department of Transportation, Location Affordability Portal Version 2, 2014, and diversitydatakids.org and Kirwan Institute, Child Opportunity Index (COI), 2014.
Table A3.
Multilevel model predicting Location Affordability Index: variance components of Null Model and Model 1.
Note.
LAI = combined-cost burden (housing and transportation); COI = Child Opportunity Index; CI = confidence interval; LAI-H = housing-cost burden; LAI-T = transportation-cost burden; SE = standard error. This table shows results of the variance components (the random effects) from two random intercept models, a null model (Model 0) with no predictor variables, and a second model (Model 1) including COI as the only predictor. ICC is the intraclass correlation coefficient which reflects the proportion of variance in LAI operating at the metro level, as opposed to at the neighborhood (tract) level.
Authors’ calculations based on data from: U.S. Department of Housing and Urban Development and Department of Transportation, Location Affordability Portal Version 2, 2014, and diversitydatakids.org and Kirwan Institute, Child Opportunity Index, 2014.
Table A4.
Multilevel model predicting Location Affordability Index: variance components of Models 2 through 5.
Note.
LAI = Location Affordability Index, i.e., combined-cost burden (housing and transportation); COI = Child Opportunity Index; LAI-H = housing-cost burden; LAI-T = transportation-cost burden. Sprawl is the Sprawl Index score, developed by the Metropolitan Research Center at the University of Utah. Segregation indices computed using 2010 Decennial Census population counts. Fragmentation is the 2007 Metropolitan Power Diffusion Index (MPDI), developed by the Center for Metropolitan Studies at the University of Pittsburgh.
The intraclass correlation coefficient reflects the proportion of variance in LAI operating at the metro-area level, as opposed to at the neighborhood (tract) level. Model 2 is a random slope model, with COI in the fixed portion of the model and both COI and metro area in the random portion of the model. Model 3 builds on Model 2, adding metropolitan region and population size controls to the fixed portion of the model. Alternate Model 3 is similar to Model 3 but estimated for only the 96 metro areas for which sprawl data was available. It does not contain sprawl itself, but is included for comparison purposes with further models that include sprawl. Sprawl is a continuous variable, with higher values denoting greater sprawl.
Authors’ calculations based on data from: U.S. Department of Housing and Urban Development and Department of Transportation, Location Affordability Portal Version 2, 2014, and diversitydatakids.org and Kirwan Institute, Child Opportunity Index, 2014.