Abstract
Visible and near infrared spectroscopy was investigated to identify the varieties of rice vinegars based on back propagation neural network and least squares-support vector machine. Five varieties of rice vinegars were prepared. Partial least squares discriminant analysis was implemented for calibration and extraction of partial least squares factors. The factors were used as inputs of back propagation neural network and least squares-support vector machine. Finally, back propagation neural network and least squares-support vector machine models were achieved. The threshold value of prediction was set as ±0.1. An excellent precision and recognition ratio of 100% was achieved by both methods. Simultaneously, certain effective wavelengths were proposed by x-loading weights and regression coefficients. The performance of effective wavelengths was validated and an acceptable result was achieved. The results indicated that visible and near infrared spectroscopy could be used as a rapid and high precision method for the identification of different varieties of rice vinegars.
INTRODUCTION
Vinegars can be produced from various materials, such as wine, cider, fruit musts, malted barley, and pure alcohol. Specific to rice vinegar, it is mainly produced by rice fermentation. The commercial rice vinegar is composed of water, acetic acid, and other secondary constituents that contribute to the aroma, taste, and preserving qualities. It is a long history that may be more than 5000 years to produce vinegar in China, and Chinese people treat vinegar as their favorite condiments, health-care products, and even medicines.[Citation1] In the market of rice vinegar, the price and quality of vinegars are different to different brands. Product adulteration also happens in the market and it is harmful to consumers. To some extent, the brand stands for the quality of the merchandise. However, studies of variety evaluation of rice vinegars are scarce. In some countries, some chemical analyses or sensory analyses were applied for the evaluation of vinegars, such as pyrolysis-mass spectrometry,[Citation2,Citation3] ion-selective electrodes,[Citation4] gas chromatography,[Citation5–10] electronic nose,[Citation1,Citation2] atomic absorption spectrum,[Citation11] and so on. Caligiani et al.[Citation12] studied the identification and quantification of the main organic components of vinegars by high resolution 1H NMR spectroscopy. However, these methods were time consuming, laborious, and costly. Therefore, a new and fast discrimination method is necessarily needed for the variety identification of rice vinegars.
With the development of modern chemometric methods and instrumentation, near infrared spectroscopy is widely applied with the characteristics of high speed, low cost, and reliable detection for quantitative and qualitative analysis in industries, such as agriculture, pharmaceuticals, food, textiles, cosmetics, and polymer production industry.[Citation13] Some researchers had applied visible and near infrared (VIS/NIR) spectroscopy for the variety discrimination or internal quality evaluation. He et al.[Citation14] studied the variety discrimination of yogurt using near infrared spectra based on principal component analysis and an artificial neural network model. For rice vinegar, Casale et al.[Citation15] discriminated the aging of vinegars during storage by near infrared spectroscopy. Sáiz-Abajo et al.[Citation16] applied near infrared spectroscopy to predict the organic acids and other quality parameters of wine vinegar. Liu et al.[Citation17] applied near infrared spectroscopy to discriminate the varieties of fruit vinegars. Some other researchers applied near infrared spectroscopy for the determination of reducing sugars,[Citation18] total procyanidins,[Citation19] ethanol and acetic acid,[Citation20] soluble solids content, and pH.[Citation21] However, there were few reports on the variety identification of rice vinegars using visible and near infrared spectroscopy without considering the chemical or flavor constituents.
The objective of this study was to investigate the potential feasibility of using VIS/NIR spectroscopy combined with different chemometrics to identify different varieties of rice vinegars. This preliminary study was based on the spectral data and brands without considering the internal chemical constituents. Simultaneously, certain effective wavelengths (EWs) were proposed for the identification of rice vinegar variety according to x-loading weights and regression coefficients.
MATERIALS AND METHODS
Preparation of Rice Vinegar Samples
In this study, five brands of rice vinegars were purchased in a local market, including Amoy, Haitian, Hengshun, Beau Ideal, and Shuangyu. They were stored in the laboratory with a constant temperature at 25 ± 1°C for more than 48 h to equalize the temperature. Three batches of the same brand were included in the rice vinegar samples. The sample number for each variety was 60 (20 for one batch), and a total of 300 samples were prepared for further analysis. Before the calibration model, some calculations were processed and there was no significant difference among these three batches of the same brand. Hence, all 60 samples of one brand were considered together for the calibration models. Two hundred twenty-five rice vinegar samples (45 samples for each variety) were randomly selected for the calibration set, while the rest (75 samples, 15 for each variety) were separated for the validation set. No single sample was used both in calibration and validation sets.
Spectral Acquisition and Preprocessing
For each sample, three transmission spectra were scanned by a handheld FieldSpec Pro FR (325–1075 nm)/A110070, Trademarks of Analytical Spectral Devices, Inc. (Analytical Spectral Devices, Boulder, CO, USA). The light source consists of a Lowell pro-lam interior light source assemble/128930 with Lowell pro-lam 14.5V bulb/128690 tungsten halogen bulb that could be used both in visible and near infrared region. The field-of-view (Analytical Spectral Devices, Boulder, CU, USA) of the spectroradiometer was 25°. The light source was placed at a height of approximately 300 mm above the sample, and the space between the light source and sample was dependant on the energy of the light source and what kind of sample was detected. The light source of the halogen bulb brought much heat, if the distance was too close to the sample, the temperature of the sample would rise. The changes of temperature would insert some noise, which was bad for the spectral scanning. Hence, the distance of 300 mm was settled after some trials. Using this distance, the halogen bulb could supply enough light energy and the sample temperature kept the same during the spectral scanning time. The probe was under the sample, and the distance between the sample and probe was 50 mm and the value was fixed by the spectroradiometer. The rice vinegar sample was placed in a cuvette with a 2-mm light path length. The transmission spectra from 325 to 1075 nm were measured at 1.5 nm intervals with an average reading of 30 scans for each spectrum. Three spectra were collected for each sample and the average spectrum of these three measurements was used in a later analysis. All spectral data were stored in a computer and processed using the RS3 software for Windows (Analytical Spectral Devices, Boulder, CO, USA) designed with a Graphical User Interface.
The transmission spectra were first transformed into ASCII format by using the ASD ViewSpecPro software (Analytical Spectral Devices, Boulder, CO, USA). Then three spectra for each sample were averaged into one spectrum and transformed by log(1/T) into an absorbance spectrum. The pretreatments were implemented by “The Unscrambler V 9.5” (CAMO AS, Oslo, Norway). The commonly used pretreatment methods were smoothing, standard normal variate (SNV), multiplicative scatter correction, first and second derivative. After some trial computations, the optimal smoothing way of a moving average with three segments was applied to decrease the noise. SNV was applied for light scatter correction and reducing the changes of light path length. To some extent, perturbations in the conditions could be reduced or removed. To avoid low signal-to-noise ratio, only the region of wavelengths (400–1000 nm) was employed for the calculations.
Partial Least Squares Discriminant Analysis
Partial least squares discriminant analysis (PLSDA), a multianalysis method, is presently widely utilized near infrared spectroscopy analysis. PLSDA opened the possibility to unravel and interpret the optical properties of rice vinegar and allowed a classification without the use of chemical information. Herein, it was used to develop the calibration model as well as a way to extract the PLS factors. The PLS factors could be used as new eigenvectors to present the original spectra and reduce the dimensionality and compress the original spectra data. PLS considers simultaneously variable matrix Y (varieties of rice vinegars) and variable matrix X (spectral data of rice vinegars). The varieties of rice vinegar were set as dummy variables, such as 1, 2, 3, 4, and 5, corresponding to five brands, respectively. In the development of the PLS model, full cross-validation was used to validate the quality and to prevent overfitting of the calibration model. The total explained variance could explain the variance of the original spectra data. According to the total explained variance, the selected PLS factors were employed as inputs of back propagation neural network (BPNN) and least squares-support vector machine (LS-SVM).
The effective wavelengths could describe the features of spectra for the identification of variety, and they were proposed by x-loading weights and regression coefficients. PLS loading weights were specific to PLS and expressed by the information at each wavelength ( X -variable) related to the variation in varieties ( Y ) summarized by the u-scores. The loading weights were normalized, so that their lengths could be interpreted as well as their directions. Wavelengths (variables) with large loading weight values were important for the prediction of variety identification ( Y ). With a similar function, regression coefficients were primarily used to check the effects of different wavelengths ( X -variables) in predicting the variety ( Y ). Large absolute values indicate the importance and the significance of the effect.
Back Propagation Neural Network
In this research, a basic error back propagation neural network (BPNN), one of the most popular neural network topologies, was employed for the development of calibration models. The PLS factors obtained from compressing the raw spectra were processed by the neural network. The network output expresses the resemblance that an object corresponds with a training pattern. The training pattern can express the dissimilarity between the desired (i.e., 0 or 1) and calculated values. Along with every process of a training pattern and adjustments of the weight factors, the difference between the desired and calculated network output value, defined as the network output error, will gradually become less and less until it meets the desired value. One cycle through all the training patterns is defined as an epoch. Before the optimal accordance of the network output error is achieved for all training patterns, many epochs are required for the back propagation. The transfer function of node can be expressed as follows:
In the function, Q is the parameter of function sigmoid, x is the input variables. BP neural network is a highly non-linear mapping from input to output, i.e., Rn → Rm, h( X ) = Y . Taking a set, for example, an input xi (xi ∈ Rn ) and the output, there is a mapping g, where
The aim is to find a mapping h, which is the best approximation to g. The architecture of BP neural network with four layers is shown in .
Least Squares-Support Vector Machine
Least squares-support vector machine (LS-SVM) is a new and attractive statistical learning method. LS-SVM has the capability of dealing with linear and nonlinear multivariate calibration and resolving these problems in a relatively fast way.[Citation22,Citation23] Moreover, SVM is capable of learning in high-dimensional feature space with fewer training data. It employs a set of linear equations instead of quadratic programming (QP) problems to obtain the support vectors. The details of the LS-SVM algorithm could be found in the literature.[Citation22,Citation24,Citation25] Radial basis function (RBF) kernel function was used in this study and is shown as follows:
RESULTS AND DISCUSSION
Overview of the Rice Vinegar Spectra
shows the transmission spectra of five varieties of rice vinegar. Some evident differences exist within the wavelength region 400–600 nm, which might be caused by the color difference or pigments.[Citation26] There are also some crossovers and overlapping among these samples. Therefore, different varieties of rice vinegar with different internal qualities could be reflected in the VIS/NIR spectra. After further analysis, the latent features of the spectra could be applied for the identification of rice vinegar variety.
PLSDA Models and Selection of PLS Factors
The PLSDA model was developed using the preprocessed spectra by moving average and SNV. The variety matrix ( Y variables) was set as dummy variables using the Arabic numbers 1, 2, 3, 4, and 5 corresponding to Amoy, Haitian, Hengshun, Beau Ideal, and Shuangyu, respectively. Five PLS factors were used in the PLSDA model. The threshold error of recognition was set as ±0.1. Take Amoy, for example, the standard value for Amoy was 1, and if the predictive value was lower than 0.9 or more than 1.1, it would be marked as a wrong identification. The recognition ratio in validation set by PLSDA was 40% when the threshold value was set as ±0.1. The total recognition ratio was 97.3% when the threshold value was set as ±0.5. The accuracy of PLSDA was not good enough for further analysis using the threshold value of ±0.1. Hence, PLSDA model was not good enough for further analysis. The reason might be that PLSDA only made use of the linear information between the spectra and rice vinegar varieties. Some later nonlinear information in the spectra might contribute to the variety identification. Therefore, the BPNN and LS-SVM methods, which could handle nonlinear problems, were needed for further studies. Simultaneously, PLSDA could be used to extract the PLS factors that could be used as the inputs of BPNN and LS-SVM models.
In order to improve the training speed and reduce the training error, the PLS factors were applied as inputs of BPNN and LS-SVM model. Hence, PLS was used as the first step to enhance the features of spectra and reduce the dimensionality of the spectra. Another PLSDA model was developed using all 300 samples for the extraction of PLS factors. Five PLS factors were used in this model according to the lowest value of root mean squares error of cross validation (RMSECV) by full cross-validation.[Citation27] The scatter plots of the top three PLS factors showed the characteristic information of clustering, as shown in . From , it could be found that rice vinegars of different varieties were distributed separately in the 3-dimensional area. But a certain brand, like Amoy, distributed not closely enough to be one brand. It seems like two brands for Amoy. Some samples of Amoy are quite close to the Haitian brand. Furthermore, the total recognition ratio was only 42% when the threshold value was set as ±0.1. The total recognition ratio was 93.3% when the threshold value was set as ±0.5. Hence, this PLSDA model was also not good enough for further analysis.
Figure 3 Cluster plot with top three PLS factors of five varieties of rice vinegars (color figure available online).
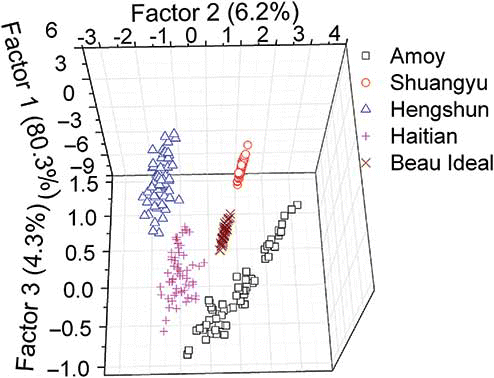
shows the total explained variance of the first six PLS factors. It indicated that the explained variance of the first five PLS factors could explain 97.1% of the total variance. The sixth PLS factor only interpreted an additional 0.2%, which contributed not so much as the aforementioned PLS factors. The first 5 PLS factors were also used in the PLSDA model. The five PLS factors could achieve the lowest value of RMSECV by full cross-validation. So the first five PLS factors were considered as the inputs of the BPNN and LS-SVM models.
Table 1 The first six PLS factors and their explained variance
BPNN Models
The selected top five PLS factors were applied to develop BPNN models. The matrix of calibration set was composed of 225 samples and 5 variables. After the adjustment of the parameters, a four-layer BP model was achieved in order to reduce the training time and simplify the adjustment of the parameters of BPNN. The BPNN model was developed with a sigmoid transfer function. The nodes of the input layer, the first and second hidden layers, and the output layer were with the structure of 5-5-3-1. The momentum was set as 0.75, which was determined after several trials in the range of 0.6–0.9, and the least learning rate was set as a default value of 0.1.[Citation28] The threshold residual error was set as 0.00001. The time of training was set as 1500. The optimal architecture of the neural networks was achieved by using the parameters mentioned above. The node of the output layer showed the results in the form of Arabic numbers from 1 to 5, which were used as a dummy variable as reference values for the separation of varieties. The specification was 1 for Amoy, 2 for Haitian, 3 for Hengshun, 4 for Beau Ideal, and 5 for Shuangyu. Seventy-five unknown samples in the validation set were used to validate the performance of this model. The threshold error of recognition was set as ±0.1. The residual error of the prediction was 7.4 × 10−5. The identification results of prediction set are shown in . The prediction results demonstrated that no sample exceeded the limit. The absolute error in the validation set is shown in , and the total recognition ratio was 100%. The results indicated that the BPNN model outperformed the PLSDA model.
Table 2 The identification results of rice vinegars in validation set by BPNN and LS-SVM models
LS-SVM Models
In this study, five brands of rice vinegars were classified by LS-SVM. This was a problem of multi-class classification, and usually it could be solved by a decomposing and reconstruction process using two-class decision machines. In the standard decomposing scheme, a multi-classification problem could be transformed into dichotomies.[Citation29] LS-SVM was trained over all the training patterns: the ith 1-v-r (short for ‘one versus the rest’). In this process, LS-SVM assigned the label +1 to the samples in the ith brand, and label −1 to the other four brands. The prediction results directly assigned the sample with a label +1 or −1 to identify which category it belonged to. To obtain a good calibration and validation performance, three crucial problems were required to solve, including the selection of the optimal input feature subset, proper kernel function (classifier), and the optimal LS-SVM parameters. Currently, there was no systematic methodology for the selection of kernel function. However, compared with other feasible kernel functions, radial basis function (RBF) as a nonlinear function was a more compacted supported kernel and able to reduce the computational complexity of the training procedure. RBF kernel could give a good performance under general smoothness assumptions. RBF function also could handle the nonlinear relationships between the spectra and target attributes. Thus, RBF kernel was recommended as the kernel function of LS-SVM in this article. The empirical trade-off parameter gam (γ) of LS-SVM determined the tradeoff between minimizing the training error and minimizing model complexity. The parameter sig2 (σ2) of RBF kernel function was the bandwidth and implicitly defined the nonlinear mapping from input space to some high dimensional feature space. A grid search technique with leave-one-out cross validation was employed to obtain the optimal parameter values. The ranges of γ within (10−2–104) and σ2 within (10−2–104) were set based on experience and previous researches.[Citation24,Citation25,Citation30] Grid search technique tried the values of each parameter across the specified search range using geometric steps. Leave-one-out cross validation was used to avoid over fitting. A two-step grid search was applied in the process. The first step of grid search was a crude search with a large step size and the second step was a specified search with a small step size. Therefore, the optimal combination of (γ, σ2) was achieved.
The first five PLS factors were used as the input data set to develop LS-SVM model for the brand classification of rice vinegars. Seventy-five samples in the validation set were predicted by the LS-SVM model with different optimal combinations of (γ, σ2). The outputs would be +1 or −1 to show which category the samples belonged to. The overall results indicated that the recognition ratio of 100% was achieved (as shown in ). With a comparison of BPNN and LS-SVM, both models achieved a perfect prediction precision and recognition ratio. Therefore, the results indicated that VIS/NIR spectroscopy combined with chemometric methods could be utilized as a rapid method for the identification of different varieties of rice vinegars. For commercial applications, more samples with different brands, batches, and storage time could be used for the calibration model. More specific work would be done to develop a more robust model with the full wavelength region (400–1000 nm) or certain selected wavelength bands.
Selection of Effective Wavelengths and Performance Validation
The plots of x-loading weights and regression coefficients, as previously mentioned, are shown in and , respectively. The first five PLS factors were explored for x-loading weights and regression coefficients, which were calculated by “The Unscrambler V 9.5” software (CAMO AS, Oslo, Norway).
Figure 5 The plots of (a) x-loading weights of first five PLS factors and (b) regression coefficients of rice vinegars (color figure available online).
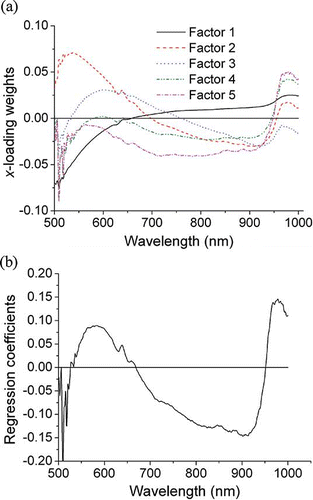
With a close observation, shows some strong peaks and valleys at certain wavelengths, which were thought to be more significant for identification of rice vinegar, such as 532–542, and 973–984 nm. shows that the wavelengths of 572–593, 891–916, and 973–984 nm are the most effective wavelengths for the variety identification of rice vinegar. In the visible region, 532–542 nm might represent the color differences since 540 nm was related to the pigments.[Citation26] Wavelengths around 891–916 and 973–984 nm might be produced by the O–H stretch with a second overtone from sugars, organic acids, and flavonoids.[Citation31] Taking x-loading weights and regression coefficients into consideration, wavelengths around 532–542, 572–593, 891–916, and 973–984 nm were considered as the effective wavelengths for variety identification of rice vinegar. In order to validate the performance of selected wavelength bands, five EWs (538, 584, 899, 902, 978 nm) were selected as the inputs of the BPNN and LS-SVM models. These five EWs had the highest absolute value of x-loading weights or regression coefficients in aforementioned wavelength bands.
The EW-BPNN model was developed using 5 EWs. The nodes of the input layer, the first and second hidden layers and the output layer were with the structure of 5–8–3–1. The momentum was set as 0.8, which was determined after several trials in the range of 0.6–0.9, and the least learning rate was set as default values of 0.1.[Citation28] The threshold residual error was set as 0.00001. The time of training was set as 1500. The threshold error of recognition was set as ±0.1. The residual error of the prediction was 1.6 × 10−3. The identification results of prediction set are shown in . The completely wrong identification for Haitian might be due to the strict threshold value of ±0.1. All 15 samples of Haitian were wrongly identified with the threshold value of ±0.1, but only one sample exceeded the cutoff value of 0.5. The other reason might be the generalization and robustness of EW-BPNN model for the specific brand of Haitian. As can be seen in , only the Haitian brand had large undulate absolute errors by the EW-BPNN model. Therefore, more samples of the Haitian brand would be considered to develop a general and robust EW-BPNN model in future works. The total recognition ratio in the validation set was 66.7% with a threshold value of ±0.1. However, the total recognition ratio in the validation set was 98.7% with a threshold value of ±0.5. The prediction result of EW-BPNN was better than that of PLSDA.
Table 3 The identification results of rice vinegars in validation set by EW-BPNN and EW-LS-SVM models
The EW-LS-SVM model was developed using 5 EWs. Different values of (γ, σ2) were achieved using a grid search technique. The overall results indicated that the recognition ratio of 100% was achieved (as shown in ). Both the EW-BPNN and EW-LS-SVM models outperformed the PLSDA models. Both the EW-LS-SVM and EW-BPNN models were good to deal with non-linearity, but the better performance of the EW-LS-SVM models compared with the EW-BPNN models might be due to the following three reasons. First, it might be that the RBF kernel was used in the EW-LS-SVM model, which was more powerful for nonlinear calibrations than sigmoid function in the EW-BPNN model. The second reason might be that a large sample set was needed for a stable and robust BPNN model, whereas the LS-SVM model was more suitable for small sample set calibrations. The third reason might be that the five independent effective wavelengths contained the main features and information of all spectral region (400–1000 nm), but they did not contribute equally useful information to the first PLS factors. The results indicated that EWs selected by x-loading weights and regression coefficients were powerful for the variety discrimination of rice vinegars. The wavelength selection method might be useful for the development of portable instruments for commercial applications of adulteration detection.
CONCLUSION
VIS/NIR spectroscopy was successfully utilized for variety identification of rice vinegar based on chemometric methods of BPNN and LS-SVM models. The PLSDA model was developed, and the recognition ratio was 40% for the validation set when the threshold value was set as ±0.1. BPNN and LS-SVM models were developed using the first five PLS factors as inputs. An excellent recognition ratio of 100% was achieved by both BPNN and LS-SVM models. The results demonstrated that VIS/NIR spectroscopy has the potential ability to identify different varieties of rice vinegar with different internal qualities. Furthermore, according to x-loading weights and regression coefficients, certain wavelengths were proposed as the effective wavelengths for the discrimination of varieties of rice vinegar. The performance was validated by BPNN and LS-SVM. The total recognition ratio was 66.7 and 100% for BPNN and LS-SVM, respectively. The effective wavelengths might be important for the development of portable instruments for commercial applications of adulteration detection. Therefore, more samples of different brands, batches, and storage time should be included to expand the model and improve the model generalization, specificity, and accuracy.
ACKNOWLEDGMENTS
This study was supported by the National Science and Technology Support Program (2006BAD10A09), the Teaching and Research Award Program for Outstanding Young Teachers in Higher Education Institutions of MOE, P. R. C., and Zhejiang Provincial Science & Technology Innovation Team Project (2009R50001).
REFERENCES
- Zhang , Q.Y. , Zhang , S.P. , Xie , C.S. , Zeng , D.W. , Fan , C.Q. , Li , D.F. and Bai , Z.K. 2006 . Characterization of Chinese vinegars by electronic nose . Sensors and Actuators B , 119 : 538 – 546 .
- Anklam , E. , Lipp , M. , Radovic , B. , Chiavaro , E. and Palla , G. 1998 . Characterization of Italian vinegar by pyrolysis-mass spectrometry and a sensor device (‘electronic nose’) . Food Chemistry. , 61 : 243 – 248 .
- Lipp , M. , Radovic , B.S. and Anklam , F. 1998 . Characterisation of vinegar by pyrolysis-mass spectrometry . Food Control. , 9 : 349 – 355 .
- Lapa , R.A.S. , Lima , J.L.F.C. , Pérez-Olmos , R. and Pilar Ruiz , M. 1995 . Simultaneous automatic potentiometric determination of acidity, chloride and fluoride in vinegar . Food Control. , 6 : 155 – 159 .
- Cocchi , M. , Durante , C. , Foca , G. , Manzini , D. , Marchetti , A. and Ulrici , A. 2004 . Application of a wavelet-based algorithm on HS-SPME/GC signals for the classification of balsamic vinegars . Chemometrics and Intelligent Laboratory Systems. , 71 : 129 – 140 .
- Cocchi , M. , Durante , C. , Grandi , M. , Lambertini , P. , Manzini , D. and Marchetti , A. 2006 . Simultaneous determination of sugars and organic acids in aged vinegars and chemometric data analysis . Talanta , 69 : 1166 – 1175 .
- Del Signore , A. 2001 . Chemometric analysis and volatile compounds of traditional balsamic vinegars from Modena . Journal of Food Engineering. , 50 : 77 – 90 .
- Durante , C. , Cocchi , M. , Grandi , M. , Marchetti , A. and Bro , R. 2006 . Application of N-PLS to gas chromatographic and sensory data of traditional balsamic vinegars of Modena . Chemometrics and Intelligent Laboratory Systems , 83 : 54 – 65 .
- Mejías , R.C. , Marín , R.N. , De Moreno , M.V.G. and Barroso , C.G. 2002 . Optimization of headspace solid-phase microextraction for analysis of aromatic compounds in vinegar . Journal of Chromatograph A , 953 : 7 – 15 .
- Tesfaye , W. , Morales , M.L. , Benítez , B. , García-Parrilla , M.C. and Troncoso , A.M. 2004 . Evolution of wine vinegar composition during accelerated aging with oak chips . Analytica Chimica Acta , 513 : 239 – 245 .
- Guerrero , M.I. , Herce-Pagliai , C. , Cameán , A.M. , Troncoso , A.M. and González , A.G. 1997 . Multivariate characterization of wine vinegars from the south of Spain according to their metallic content . Talanta , 45 : 379 – 386 .
- Caligiani , A. , Acquotti , D. , Palla , G. and Bocchi , V. 2007 . Identification and quantification of the main organic components of vinegars by high resolution 1H NMR spectroscopy . Analytica Chimica Acta , 585 : 110 – 119 .
- Yan , Y.L. , Zhao , L.L. , Han , D.H. and Yang , S.M. 2005 . The Foundation and Application of Near Infrared Spectroscopy Analysis , Beijing, , China : China Light Industry Press .
- He , Y. , Feng , S. J. , Deng , X. F. and Li , X. L. 2006 . Study on lossless discrimination of varieties of yogurt using the visible/NIR-spectroscopy . Food Research International , 39 : 645 – 650 .
- Casale , M. , Sáiz-Abajo , M.J. , González-Sáiz , J.M. , Pizarro , C. and Forina , M. 2006 . Study of the aging and oxidation processes of vinegar samples form different origins during storage by near-infrared spectroscopy . Analytica Chimica Acta , 557 : 360 – 366 .
- Sáiz-Abajo , M.J. , González-Sáiz , J.M. and Pizarro , C. 2006 . Prediction of organic acids and other quality parameters of wine vinegar by near-infrared spectroscopy . A feasibility study. Food Chem. , 99 : 615 – 621 .
- Liu , F. , He , Y. and Wang , L. 2008 . Determination of effective wavelengths for discrimination of fruit vinegars using near infrared spectroscopy and multivariate analysis . Analytica Chimica Acta , 615 : 10 – 17 .
- Fu , X.G. , Yan , G.Z. , Chen , B. and Li , H.B. 2005 . Application of wavelet transforms to improve prediction precision of near infrared spectra . Journal of Food Engineering. , 69 : 461 – 466 .
- García-Parrilla , M.C. , Heredia , F.J. , Troncoso , A.M. and González , A.G. 1997 . Spectrophotometric determination of total procyanidins in wine vinegars . Talanta , 44 : 119 – 123 .
- Yano , T. , Aimi , T. , Nakano , Y. and Tamai , M. 1997 . Prediction of the concentrations of ethanol and acetic acid in the culture broth of a rice vinegar fermentation using near-infrared spectroscopy . Journal of Fermentation and Bioengineering. , 84 : 461 – 465 .
- Liu , F. , He , Y. and Wang , L. 2008 . Comparison of calibrations for the determination of soluble solids content and pH of rice vinegars using visible and short-wave near infrared spectroscopy . Analytica Chimica Acta , 610 : 196 – 204 .
- Vapnik , V.N. 1995 . The Nature of Statistical Learning Theory , Springer-Verlag : New York .
- Suykens , J.A.K. and Vanderwalle , J. 1999 . Least squares support vector machine classifiers . Neural Processing Letters. , 9 : 293 – 300 .
- Guo , H. , Liu , H.P. and Wang , L. 2006 . Method for selecting parameters of least squares support vector machines and application . Journal of System Simulation. , 18 : 2033 – 2036 .
- Chen , Q.S. , Zhao , J.W. , Fang , C.H. and Wang , D.M. 2007 . Feasibility study on identification of green, black and oolong teas using near-infrared reflectance spectroscopy based on support vector machine (SVM) . Spectrochinica Acta Part A , 66 : 568 – 574 .
- De Jong , S. SIMPLS . 1993 . An alternative approach to partial least squares regression . Chemometrics and Intelligent Laboratory Systems , 18 : 251 – 263 .
- Somers , C. 1998 . The Wine Spectrum: An Approach Towards Objective Definition of Wine Quality , 50 Winetitles : Adelaide, Australia .
- Shao , Y.N. , He , Y. and Hu , X.Y. 2007 . Optical system for tablet variety discrimination using visible/near-infrared spectroscopy . Applied Optics , 46 : 8379 – 8384 .
- Angulo , C. , Parra , X. and Català , A. K-SVCR. 2003 . A support vector machine for multi-class classification . Neurocomputing , 55 : 57 – 77 .
- Belousov , A.I. , Verzakov , S.A. and Von Frese , J. 2002 . Applicational aspects of support vector machines . Journal of Chemonetrics. , 16 : 482 – 489 .
- Sasic , S. and Ozaki , Y. 2001 . Short-wave near-infrared spectroscopy of biological fluids. 1. Quantitative analysis of fat, protein, and lactose in raw milk by partial least-squares regression and band assignment . Analytical Chemistry , 73 : 64 – 71 .