Abstract
Anthocyanin content and antioxidant activity are believed to display an array of beneficial actions on human health and well-being. This article presents an innovative time and cost economizing method to determine these important attributes of sour cherry during the ripening stage by combining image processing and artificial neural network techniques. For this purpose, six different stages of ripening were selected based on homogeneous size and color of the cherries. The measuring technique consisted of a charge coupled device camera for image acquisition, fluorescent illuminants, capture card, and MATLAB software for image analysis. DPPH and pH differential methods were used to determine antioxidant activity and anthocyanin content, respectively. Several artificial neural networks were designed, trained, and generalized with a back propagation neural network using ‘trainlm’ as a training function. Among these networks, two networks with 12-20-6-1 and 12-15-19-1 architectures had the highest correlation coefficients (R = 0.987 and R = 0.904) and the lowest values of MSE (0.027 and 0.186) for modeling anthocyanin content and antioxidant activity values, respectively, from laboratory results and the machine vision method. It was found that anthocyanin content was constantly increased during ripening stages of sour cherry, but antioxidant activity decreased during the early stages of sour cherry development but increased from the second stage.
INTRODUCTION
Sour cherries (Prunus cerasus), having an attractive color, are consumed in both fresh and processed form. Iran is the fifth largest producing country of sour cherry around the world after Turkey, Russian Federation, Ukraine, and Poland.[Citation1] A major role of the phytochemicals is protection against oxidation. Both cardiovascular disease and cancer are thought to be, in particular, the result of oxidative stress, which can lead to damage of the larger biomolecules, such as DNA, lipids, and proteins. It has been estimated that there are 10,000 oxidative hits to DNA per cell per day in humans.[Citation2,Citation3] Furthermore, in order to control the oxidation processes in foods, previous researchers have adopted a range of strategies that involve an appropriate choice of raw material, processing technologies, packing materials, and storage conditions, and it is often necessary to use antioxidants. These inhibitory substances, when added to the product, may preserve the lipid fraction from oxidation without reducing the food quality.[Citation4] More recently, much attention has been paid to the antioxidant properties of polyphenols, which seem to protect tissues against oxygen free radicals and lipid peroxidation.[Citation5] Fruits, such as cherries, are high in antioxidants, and a diet high in this fruit should help prevent oxidative stress, and may therefore prevent chronic disease and slow aging.
One of the most common plant pigments are anthocyanins, which appear to have multi functions; for example, in addition to coloring property, they may be used as a quality control marker of food stuffs.[Citation6] Due to our increasing understanding and awareness of the potential beneficial human health effects, research on anthocyanins has recently intensified.[Citation7] Anthocyanin pigment content has a critical role in the color quality of many fresh and processed fruits and vegetables. Thus, accurate measurement of anthocyanins, along with their degradation indices, is very useful to food technologists and horticulturists in assessing the quality of raw and processed foods. In addition, studies have demonstrated that anthocyanin extracts can improve sight acuteness; display antioxidative, radical-scavenging, and anticancer activities; and act as chemoprotective agents. Anthocyanins also play a role in anti-diabetic properties, such as lipid lowering, insulin secretion, and vasoprotective effects.[Citation7 − Citation14]
Polder et al.,[Citation15] by using an independent component analysis (ICA) on spectral images of tomatoes, determined the predicted percentage variation this way: 0.78 for the lycopene concentration and 0.80 for the chlorophyll concentration. For the supervised method these values were 0.95 and 0.73, respectively. Machine vision (MV) is a technique to acquire and analyze an image of a real scene by computers to obtain information or control processes.[Citation16] Rapid advances in hardware and software for digital image processing have caused several studies on the development of MVs for the food industry during recent years.[Citation16,Citation17] The examples of successful applications of MV in the food industry are quality evaluation; detection of defects; identification; grading; and sorting of fruits and vegetables, meat and fish, bakery products, and prepared goods.[Citation18
−
Citation35]
Various techniques, such as artificial neural network (ANN), statistical learning (SL), fuzzy logic (FL) and genetic algorithm (GA) have been applied increasingly for agri-food quality evaluation using MV in recent years.[Citation21,Citation36 − Citation38] Du and Sun[Citation21] reviewed the latest advances in learning techniques, such as ANNs, SL, FL, and GA, for food quality evaluation using MV. Makino et al.[Citation39] estimated oxygen uptake rate of tomato fruits by ANNs modeled using near-infrared spectral absorbance and fruit mass. Wang et al.[Citation40] employed machine vision and back-propagation NN to grade chestnuts. Unlike conventional laboratory methods, which are expensive, destructive, and time consuming, MV systems can be more useful for food analysis. In this work, an ANN-assisted image processing technique was used for determining antioxidant activity and anthocyanin content of sour cherries during ripeness of the fruit.
MATERIALS AND METHODS
Samples Preparation
Sour cherry fruits were taken from a garden located in Shahrestanak village in Alborz province of Iran and transported to the Agricultural Machinery Department, University of Tehran (Iran) and stored in a refrigerator after detaching their stems. The senescence periods of cherries changed in six stages: from green to yellow, pink, light red then to red, and finally to black. Fruits in different ripening stages were chosen based on their colors by visual inspection.
Analytical Methods
Anthocyanin content
At first, sour cherries were divided into homochromatic groups; fourteen fruits were in each group. Juices were extracted from each group and centrifuged at 6000 rpm for 20 min. A pH differential method using two buffer systems, potassium chloride buffer, pH 1.0 (0.025 M) and sodium acetate buffer, pH 4.5 (0.4 M) were used to determine the total anthocyanin content of sour cherry juice.[Citation41 − Citation43] Briefly, 0.4 mL of a sour cherry juice sample was mixed with 3.6 mL of the appropriate buffer and the absorbance was read against a water blank at 510 and 700 nm. Total absorbance (A) was calculated as:
The total anthocyanin content of the samples (mgr of cyanidin-3-rutinoside per 100 mL of cherry juice) was then calculated by the following equation:
The visible spectra of samples were determined by scanning the absorbance between 350 and 700 nm using a Cecil CE 2502 UV–vis spectrophotometer (Cecil Ins., England). Quartz cuvettes with a 1-cm path length were used, and all measurements were carried out at room temperature (∼22°C) in triplicate. Absorbance readings were made against distilled water as a blank.
Antioxidant activity
DPPH (2,2-diphenyl-1-picrylhydrazyl) was obtained from Fluka (Buchs, Switzerland), and 2.5 mgr of DPPH was dissolved in 100 mL of methanol (≈0.0625 mmol L−1). This stock solution was prepared daily and kept in the dark at ambient temperature when not used. EC50 value was defined as the amount of sample necessary to decrease the initial DPPH . concentration by 50%. EC50 was expressed as volume of sample (mL) to the amount of DPPH (gr). EC50 was determined according to the method of Cam.[Citation41] Of the appropriately diluted sour cherry juice samples, 0.1 mL was mixed with 3.9 mL of the above mentioned DPPH solution. The control sample was prepared with the same volume of methanol. Absorbance at 515 nm was measured at different time intervals using a Cecil CE 2502 UV–vis spectrophotometer (Cecil Ins., England) until the reaction reached steady-state condition. The DPPH concentration in the reaction medium was calculated using a calibration equation. The remaining amount of DPPH (percentage) at steady-state condition was calculated as follows:
Image Processing
Machine vision setup
Setup consists of a charge coupled device (CCD) camera (Model 565s with 510 by 492 pixel resolutions; PROLINE, UK), capture card (WinFast DV2000 with a 320H×240V resolution), two fluorescent tubes around the camera as a lighting system providing uniform light and eliminating shadow images of fruits, and a personal computer (PC). The camera was mounted 10 cm above the white background and powered by a 24 V power supply. The light source and camera were mounted on a frame and attached to the measurement table. A tarpaulin cover was used to omit the effects of surrounding lights. Generally, the system was under controlled conditions (illumination, light source, camera angle, and distance between camera and sour cherries). Captured signals by the camera were transferred to the PC through the video capture cards, digitized, and stored on the PC into RGB coordinates for further analysis[Citation46] (). Using this setup, images of sour cherries were taken.
Cherry fruit segmentation
When the fruit is well contrasted against the background, fruit localization could be undertaken by classical supervised or unsupervised threshold techniques.[Citation32] After trial and error, a white background was chosen because of it has good and acceptable contrast with cherries. The fruit area was separated from the background with the thresholding technique and algorithm of Otsu, which is a nonparametric and unsupervised method of automatic threshold selection for image segmentation, based on statistical and space information of histograms.[Citation32,Citation47] The saturation parameter (S) was effective to segment the cherry from the background, although, being a non-linear combination of the red, green, and blue values, it requires more computation. It was found that a simple thresholding was not enough because of the varying color of cherries in different ripening stages, possible juice spot, reflection, and possible noises due to shadows. Accordingly, the ‘if’ command in MATLAB software and morphological operators were applied.
Characterization of fruit images
At first, 34 attributes in RGB and L*a*b* (CIE) spaces were extracted from the acquired images. These included some statistical parameters, such as mean, skewness, kurtosis, and variance of color matrices (R, G, and B) or their differences and ratios as given by Eqs. (4) to (15). CIE parameters, including L*, a*, b*, were also extracted by using ‘cform’ in an image processing toolbox in MATLAB software.
Accelerating procedure
An effective procedure to reduce the dimension of the input vector is using sensitivity analysis available in NeuroSolutions 5.0 software.[Citation48] By means of sensitivity analysis, we determined the most effective attributes on outputs (antioxidant activity and anthocyanin content). Accordingly, the original data set (from the characterization of fruit images) was subjected to sensitivity analysis and 12 features were finally selected as inputs for developing ANN models. Selected features are shown in . This means by using sensitivity analysis, a 64.70% reduction in features was achieved. These features were then fed to ANN models as input vectors.
Table 1 Selected features of cherries by using sensitivity analysis
Artificial Neural Networks Modeling
ANNs are composed of interconnecting artificial neurons (programming constructs that mimic the properties of biological neurons). ANNs may either be used to gain an understanding of biological neural networks, or for solving artificial intelligence problems without necessarily creating a model of a real biological system. In this research, ANN, as implemented in MATLAB, was used for modeling the relationship between antioxidant activity and anthocyanin content measured by means of the laboratory results and characteristics extracted from the images of cherries. Levenberg-Marquardt (LM) algorithm, ‘trainlm’ function was used to train the networks. Image analysis routines are integrated into software programmed in MATLAB that converts the image attributes of the cherry images over the whole fruit pixels shown in the images into desired outputs.
Accuracy Assessment and Calibration
The correlation coefficient, mean absolute error, mean square error, and mean absolute percentage error are the statistical criterions defined as Eqs. (16)–(19)[Citation49,Citation50] and were used for measurement of accuracy and differences between the normalized values achieved by MV setup and the actual antioxidant activity and anthocyanin content achieved in laboratory methods.
RESULTS AND DISCUSSION
At first, data were divided into three sets; among 720 and 750 batches for antioxidant and anthocyanin modeling, 75, 10, and 15% of the data were used for training, validation, and testing, respectively. Several ANNs were designed, trained, and generalized, using the MATLAB Neural Network Toolbox. Back propagation algorithm was chosen to build the prediction models. The results obtained from the six models and their characteristics are illustrated in for antioxidant activity. Among these, the best performance, without overtraining, was achieved with a back propagation neural network used ‘trainlm’ as training function and with 14 neurons in the first hidden layer and 9 in the second one, both layers use ‘tansig’ as their transfer function (). This two hidden layer network has 12-15-19-1 architecture for, i.e., the best models were consisted of an input layer with 12 input variables, two hidden layers with 15 and 19 neurons in each layer, and an output layer with one output variable, highlighted in . This architecture has the highest correlation coefficient (0.904) () and the lowest values of MAE (0.328), MSE (0.186), and MAPE (3.4%) for antioxidant activity indicating that the ANN predicted antioxidant activity by this model tends to follow the corresponding actual ones.
Table 2 Performance of neural networks results related to antioxidant activity
Figure 2 Architecture of the used artificial neural networks for (a) anthocyanin content and (b) antioxidant activity.
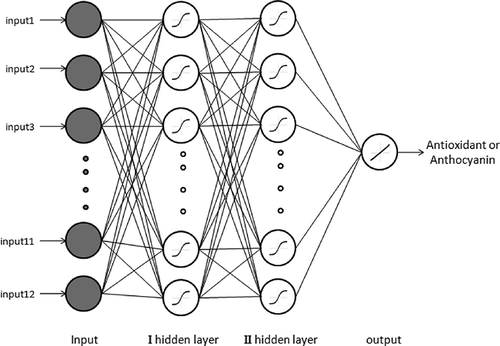
Similar results were observed for anthocyanin content as highlighted in . For anthocyanin content, a back propagation neural network had the best performance without overtraining also. It used ‘trainlm’ as a training function with 20 neurons in the first hidden layer and 6 in the second one, just like antioxidant modeling network, both layers use ‘tansig’ as their transfer function and the NN was consisted of an input layer with 12 input variables and an output layer with one output variable (). The 12-20-6-1 architecture has the highest correlation coefficient (0.987) () and the lowest values of MAE (0.082), MSE (0.027), and MAPE (3.5%) for anthocyanin modeling. These results show that our system can be used to determine anthocyanin content and antioxidant activity of sour cherry well with a very low cost CCD camera and without destructive, time consuming, and expensive tests. Figures 3a and 3b compare the predicted values with the desired output values for anthocyanin content and antioxidant activity using the optimal ANNs (12-20-6-1 and 12-15-19-1 architecture, respectively).
Figure 3 Correlation between (a) anthocyanin content and (b) antioxidant activity achieved by laboratory methods and predicted values.
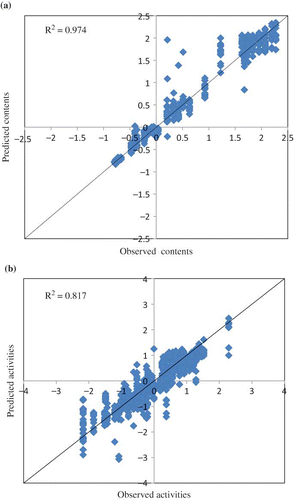
Table 3 Performance of neural networks results related to anthocyanin content
Physiologically, at the red stage, cherry fruit is mature and reaches its maximum weight and size. At stage ‘d’, fruit ripening starts, its color changes from red to black, and its texture becomes soft. Anthocyanin content is constantly increased during ripening stages and changing color from green to red, as can be seen in ; but antioxidant activity decreased during the early stages of sour cherry development but increased from stage 2, which coincided with the anthocyanin accumulation and fruit darkening. Antioxidant activity is due to some other compounds than anthocyanin, such as tannin, organic acid, etc., at early stages of ripening;[Citation51] antioxidant reached the maximum activity at stage 6 ().
CONCLUSION
In this study, as a new experiment anthocyanin content and antioxidant activity of sour cherry were determined by using MATLAB software and ANN assisted MV system under controlled conditions. The measuring technique discussed in this article consists of a CCD camera for image acquisition, fluorescent illuminants, capture card, and MATLAB software for image analysis and has been used to provide a way to determine the anthocyanin content and antioxidant activity. By using Otsu thresholding technique, cherries at different stages of ripening were segmented successfully. Evaluation of observed and predicted values of anthocyanin content and antioxidant activity showed that this system can effectively be used for determination of these attributes. Furthermore, the possibility of mistakes in the sensitive laboratory method or applying sensible material, such as DPPH, which is sensitive to light and lapse, make us find a low cost, un-destructive, and rapid way to estimate these attributes. Overall, the method presented in this study can be extended by future researches.
ACKNOWLEDGMENTS
The financial support provided by the Department of Agricultural Machinery Engineering, Faculty of Agricultural Engineering and Technology, University of Tehran, Karaj, Iran, is gratefully acknowledged.
Notes
Color versions of one or more of the figures in the article can be found online at www.tandfonline.com/ljfp.
REFERENCES
- Anonymous. Countries by commodity2010. Food and Agriculture Organization of the United Nations, FAOSTATaccessed April 9, 2012). http://www.faostat.fao.org (http://www.faostat.fao.org)
- Ames , B. , Shigenaga , M. and Oxidants , Hagen, T. 1993 . antioxidants . and the degenerative diseases of aging. Proceedings of the National Academy of Sciences of the United States of America , 90 : 7915 – 7922 .
- Boyer , J. and Hai Liu , R. 2004 . Apple phytochemicals and their health benefits . Nutrition Journal , 3 : 1 – 15 .
- Ruiz-Navajas , Y. , Viuda-Martos , M. , Fernández-López , J. , Zaldivar-Cruz , J.M. , Kuri , V. and Pérez-Álvarez , J. 2011 . Antioxidant activity of Artisanal honey from Tabasco, Mexico . International Journal of Food Properties , 14 ( 2 ) : 459 – 470 .
- Raczkowska , J. , Mielcarz , G. , Howard , A. and Raczkowski , M. 2011 . UPLC and spectrophotometric analysis of polyphenols in wines available in the Polish market . International Journal of Food Properties , 14 ( 3 ) : 514 – 522 .
- Aberoumand , A. 2011 . A review article on edible pigments properties and sources as natural biocolorants in foodstuff and food industry . World Journal of Dairy and Food Sciences , 6 ( 1 ) : 71 – 78 .
- Ghosh , D. and Konishi , T. 2007 . Anthocyanins and anthocyanin-rich extracts: Role in diabetes and eye function . Asia Pacific Journal of Clinical Nutrition , 16 ( 2 ) : 200 – 208 .
- Kalt , W. , Blumberg , J.B. , McDonald , J.E. and Vinqvist-Tymchuk , M.R. 2008 . Fillmore, Sh.A.E.; Graf, B.A.; Jennifer, M.; O'leary, J.M.; Milbury, P.E. Identification of anthocyanins in the liver, eye and brain of blueberry-fed pigs. Journal of Agricultural and Food Chemistry , 56 : 705 – 712 .
- Bellido , G.G. and Beta , T. 2009 . Anthocyanin composition and oxygen radical scavenging capacity (ORAC) of milled and pearled purple, black, and common barley . Journal of Agricultural and Food Chemistry , 57 : 1022 – 1028 .
- Astadi , I.R. , Astuti , M. , Santoso , U. and Nugraheni , P.S. 2009 . In vitro antioxidant activity of anthocyanins of black soybean seed coat in human low density lipoprotein (LDL) . Food Chemistry , 112 : 659 – 663 .
- Liu , Z. , Schwimer , J. , Liu , D. , Greenway , F.L. , Anthony , C.T. and Woltering , E.A. 2005 . Black raspberry extract and fractions contain angiogenesis inhibitors . Journal of Agricultural and Food Chemistry , 53 : 3909 – 3915 .
- DeFuria , J. , Bennett , G. , Strissel , K.J. , Perfield , J.W. , Milbury , P.E. , Greenberg , A.S. and Obin , M.S. 2009 . Dietary blueberry attenuates whole-body insulin resistance in high fat-fed mice by reducing adipocyte death and its inflammatory sequelae . Journal of Nutrition , 139 : 1 – 7 .
- Jayaprakasam , B. , Vareed , S.K. , Olson , L.K. and Nair , M.G. 2005 . Insulin secretion by bioactive anthocyanins and anthocyanidins present in fruits . Journal of Agricultural and Food Chemistry , 53 : 28 – 31 .
- Matheus , M.E. , Fernandes , S.B. , Silveira , C.S. , Rodrigues , V.P. , Menezes , F.D. and Fernandes , P.D. 2006 . Inhibitory effects of Euterpe oleracea Mart . on nitric oxide production and iNOS expression. Journal of Ethnopharmacology , 107 : 291 – 296 .
- Polder , G. , van der Heijden , G.W.A.M. and Young , I.T. 2003 . Tomato sorting using independent component analysis on spectral images . Real-Time Imaging , 9 : 253 – 259 .
- Brosnan , T. and Sun , D.W. 2003 . Improving quality inspection of food products by computer vision—A review . Journal of Food Engineering , 61 : 3 – 16 .
- Blasco , J. , Aleixos , N. and Moltó , E. 2003 . Machine vision system for automatic quality grading of fruit . Biosystems Engineering , 85 : 415 – 423 .
- Li , Y. , Dhakal , S. and Peng , Y. 2012 . A machine vision system for identification of micro-crack in egg shell . Journal of Food Engineering , 109 : 127 – 134 .
- Anami , B.S. , Savakar , D.G. and Biradar , V.S. 2009 . Identification of multiple grain image samples from tray . International Journal of Food Science and Technology , 44 : 2452 – 2458 .
- Sabliov , C.M. , Boldor , D. , Keener , K.M. and Farkas , B.E. 2002 . Image processing method to determine surface area and volume of axi-symmetric agricultural products . International Journal of Food Properties , 5 : 641 – 653 .
- Du , C.J. and Sun , D.W. 2006 . Learning techniques used in computer vision for food quality evaluation: A review . Journal of Food Engineering , 72 : 39 – 55 .
- Hatcher , D.W. , Symons , S.J. and Manivannan , U. 2004 . Developments in the use of image analysis for the assessment of oriental noodle appearance and color . Journal of Food Engineering , 61 : 109 – 117 .
- Antonelli , A. , Cocchi , M. , Fava , P. , Foca , G. , Franchini , G.C. , Manzini , D. and Ulrici , A. 2004 . Automated evaluation of food color by means of multivariate image analysis coupled to a wavelet-based classification algorithm . Analytica Chimica Acta , 515 : 3 – 13 .
- Borah , S. and Bhuyan , M. 2005 . A computer based system for matching colors during the monitoring of tea fermentation . International Journal of Food Science and Technology , 40 : 675 – 682 .
- Mery , D. and Pedreschi , F. 2005 . Segmentation of color food images using a robust algorithm . Journal of Food Engineering , 66 : 353 – 360 .
- Tanska , M. , Rotkiewicz , D. , Kozirok , W. and Konopka , I. 2005 . Measurement of the geometrical features and surface color of rapeseeds using digital image analysis . Food Research International , 38 : 741 – 750 .
- Pedreschi , F. , Leon , J. , Mery , D. and Moyano , P. 2006 . Development of a computer vision system to measure the color of potato chips . Food Research International , 39 : 1092 – 1098 .
- Yoshida , K. , Ito , D. , Shinkai , Y. and Kondo , T. 2008 . Change of color and components in sepals of chameleon hydrangea during ripeness and senescence . Phytochemistry , 69 : 3159 – 3165 .
- Kang , S.P. and Sabarez , H.T. 2009 . Simple color image segmentation of bicolor food products for quality measurement . Journal of Food Engineering , 94 : 21 – 25 .
- Unay , D. , Gosselin , B. , Kleynen , O. , Leemans , V. , Destain , M.F. and Debeir , O. 2011 . Automatic grading of bi-colored apples by multispectral machine vision . Computers and Electronics in Agriculture , 75 : 204 – 212 .
- Fernández , L. , Castillero , C. and Aguilera , J.M. 2005 . An application of image analysis to dehydration of apple discs . Journal of Food Engineering , 67 : 185 – 193 .
- Fu , L. , Okamoto , H. , Kataoka , T. and Shibata , Y. 2011 . Color based classification for berries of Japanese blue honeysuckle . International Journal of Food Engineering , 7 : 4 131–135.
- Leon , K. , Mery , D. , Pedreschi , F. and Leon , J. 2006 . Color measurement in L*a*b* units from RGB digital images . Food Research International , 39 : 1084 – 1091 .
- Kılıç , K. , Boyacı , I.H. , Koksel , H. and Ku Smenog , Lu, I. 2007 . A classification system for beans using computer vision system and artificial neural networks . Journal of Food Engineering , 78 : 897 – 904 .
- Afshari-Jouybari , H. and Farahnaky , A. 2011 . Evaluation of Photoshop software potential for food colorimetry . Journal of Food Engineering , 106 : 170 – 175 .
- Cakmak , Y.S and Boyaci , I.H. 2011 . Quality evaluation of chickpeas using an artificial neural network integrated computer vision system . International Journal of Food Science and Technology , 46 : 194 – 200 .
- Kılıç , K. , Onal-Ulusoy , B. , Yıldırım , M. and Boyacı , I.H. 2007 . Scanner-based color measurement in L*a*b* format with artificial neural networks (ANN) . European Food Research and Technology , 226 : 121 – 126 .
- Abbasgholipour , M. , Omid , M. , Keyhani , A.R. and Mohtasebi , S.S. 2011 . Color image segmentation with genetic algorithm in a raisin sorting system based on machine vision in variable conditions . Expert System with Application , 38 ( 4 ) : 3671 – 3678 .
- Makino , Y. , Ichimura , M. , Oshita , S. , Kawagoe , Y. and Yamanaka , H. 2010 . Estimation of oxygen uptake rate of tomato (Lycopersicon esculentum Mill.) . fruits by artificial neural networks modelled using near-infrared spectral absorbance and fruit mass. Food Chemistry , 121 : 533 – 539 .
- Wang , Ch. , Li , X. , Wang , W. , Feng , Y. , Zhou , Zh. and Zhan , H. 2011 . Recognition of worm-eaten chestnuts based on machine vision . Mathematical and Computer Modeling , 54 : 888 – 894 .
- Cam , M. , Hisil , Y. and Durmaz , G. 2009 . Classification of eight pomegranate juices based on antioxidant capacity measured by four methods . Food Chemistry , 112 : 721 – 726 .
- Lako , J. , Trenerry , V.C. , Wahlqvist , M. , Wattanapenpaiboon , N. , Sotheeswaran , S. and Premier , R. 2007 . Phytochemical flavonols, carotenoids and the antioxidant properties of a wide selection of Fijian fruit, vegetables and other readily available foods . Food Chemistry , 101 : 1727 – 1741 .
- Yousefi , S.H. , Emam-Djomeh , Z. , Mousavi , S.M.A. and Askari , GH.R. Comparing the effects of microwave and conventional heating methods on the evaporation rate and quality attributes of pomegranate (Punica granatum L.) juice concentrate . Food and Bioprocess Technology 2011 , doi: DOI 10.1007/s11947-011-0603-x
- Stintzing , F.C. , Trichterborn , J. and Carle , R. 2006 . Characterisation of anthocyanin–betalain mixtures for food colouring by chromatic and HPLC-DAD-MS analyses . Food Chemistry , 94 : 296 – 309 .
- Lohachoompol , V. , Srzednicki , G. and Craske , J. 2004 . The change of total anthocyanins in blueberries and their antioxidant effect after drying and freezing . Journal of Biomedicine and Biotechnology , 5 : 248 – 252 .
- Khojastehnazhand , M. , Omid , M. and Tabatabaeefar , A. 2010 . Determination of tangerine volume using image processing methods . International Journal of Food Properties , 13 ( 4 ) : 760 – 770 .
- Otsu , N. 1979 . A threshold selection method from gray-level histograms . IEEE Transactions Systems, Man, and Cybernetics , 9 ( 1 ) : 62 – 66 .
- Neurosolution for excel. NeuroDimension Inc. 2010 http://www.neurosolutions.com/ (http://www.neurosolutions.com/)
- Ramedani , Z. , Omid , M. and Keyhani , A. 2013 . Modeling solar energy potential in Tehran province using artificial neural networks . International Journal of Green Energy , 10 ( 4 ) : 427 – 441 .
- Ramedani , Z. , Omid , M. and Keyhani , A. 2012 . A method based on neural networks for generating solar radiation map. International Journal of Energy and Environment , 3 ( 5 ) : 775 – 786 .
- Serrano , M.N. , Guillean , F. , Martinez-Romero , D. , Castillo , S. and Valero , D. 2005 . Chemical constituents and antioxidant activity of sweet cherry at different ripening stages . Journal of Agricultural and Food Chemistry , 53 : 2741 – 2745 .