Abstract
In order to differentiate and characterize the cooked pork samples from three Chinese indigenous pig breeds (Anqingliubai, Wannanhua Pig, and Dingyuan Pig) and a typical hybrid pig breed Yorkshire×Large White×Duroc, the volatile flavor composition was determined for 32 monovarietal samples from these four pig varieties. A headspace solid-phase microextraction gas chromatography–mass spectrometry (headspace–solid-phase microextraction–gas chromatography–mass spectrometry) procedure was used to identify the volatile flavor compounds and detect the concentration of these compounds. One way analysis of variance, principal component analysis, and stepwise linear discriminant analysis were used to process data and to develop discriminant models. Principal component analysis described the correlation between samples (objects) and volatile flavor compounds (variables). The results of the principal component analysis show that the most important contributions to the differentiation of Anqingliubai cooked pork samples are undecanal and 2-undecenal. Hexadecanal and 2-decene-1-ol are the major contributions in Yorkshire×Large White×Duroc cooked pork samples. Benzaldehyde, hexanal, dodecanal, 1-octen-3-ol, and 1-octanolare strongly associated to Wannanhua pig cooked pork samples. Dingyuan pig cooked pork samples are most correlated with heptanal, octanal, 14-octadecenal, (E)-2-octenal, N-hexadcanoic acid, (E)-13-octadecenic acid 3-hydroxy-2-butanone, 2-ethylfuran and 2-butanone. Stepwise linear discriminant analysis was used for differentiation of cooked pork samples according to the varieties, resulting in a correct classification of 100%.
INTRODUCTION
In China, it is often considered, by most Chinese consumers, that pork derived from Chinese indigenous breed pigs have better eating quality than hybrid pigs. However, during the last decades, pig production, breeding the pigs based solely on performance traits, particularly on lean content and feeding efficiency, the hybrid pig has advantages in these places, so the hybrid pigs replaced the Chinese indigenous breed pigs gradually. With the development of living standards of people, the demand of higher quality of pork increased gradually. Due to high production costs and low yields, the price of the pork from the Chinese indigenous breed pigs is relatively high. Some people use the common pork to masquerade as the higher quality pork; these actions will have negative influence on the market and hinder the protection of the Chinese indigenous breed pigs. Many techniques such as pH, color, electrical conductivity, and drip loss currently used to distribute pork quality,[Citation1−Citation3] but using these techniques couldn’t discriminate between the pork from Chinese indigenous breed pigs and that from hybrid pigs accurately in a shorter time. Thus, there is a need to find new assays, well correlated with pork eating quality, to identify pork from Chinese indigenous breed pigs or hybrid pigs accurately and quickly.
Among many factors had an impact on pork eating quality, tenderness, juiciness, flavor, intramuscular fat (IMF) and color are considered the most important.[Citation4,Citation5] The breed is tremendously impacted on IMF, flavor, tenderness, juiciness, and color.[Citation2,Citation6] A high content of IMF, termed marbling fat in muscle, has been reported to positively influence juiciness, tenderness, flavor, and the separation process of fiber bundles myofibrils.[Citation2,Citation5,Citation7] In comparison with hybrid pigs, Chinese indigenous breed pigs have high IMF content and more intense marbling. The cooked pork derived from Chinese indigenous breed pigs show the higher pork flavor intensity than hybrid pigs.[Citation8] Flavor is the most important sensory attribute for the eating quality of pork.[Citation9] That is to say, Chinese indigenous breed pigs have better eating quality than hybrid pigs.
Several studies have been focused on the research of new techniques for a better prediction of meat quality. On the basis of water-holding capacity, Geesink et al.[Citation10] provided a promising way to classify pork longissimus by using near infrared reflectance spectra. Fidel et al.[Citation11] found that the measure of exoprotease activities can be used for an effective prediction of post-mortem technological pork quality. Nadia et al.[Citation12] classed the pork samples into four quality grades by using the dielectric properties of pork muscle. These evaluation methods were based on the pork quality. Some food identification methods are based on that different quality food has different volatile flavor compounds.[Citation13−Citation16] The pork from different breeds obviously has different volatile flavor compounds.[Citation8] Solid-phase micro-extraction (SPME) was proposed by Pawliszyn and coworkers[Citation17,Citation18] offering two main advantages: No extraction solvent required and combination of extraction and pre-concentration in a single step without pre-treatment of samples. This technique has been successfully used to characterize a wide range of volatile flavor compounds in cooked pork samples.[Citation19−Citation21] Therefore, a new method based on volatile flavor compounds must to be developed that will be able to distinguish the pork of Chinese indigenous breed pigs and hybrid pigs.
MATERIALS AND METHODS
Animals and Sampling
Three Chinese indigenous pig breeds (AQ (Anqingliubai), WP (Wannanhua Pig), DP (Dingyuan Pig)) in Anhui province and a typical hybrid pig breed (DLY (Yorkshire×Large White×Duroc)) were studied. Eighteen pigs of the three Chinese indigenous pig breeds (AQ = 8, WP = 8, and DP = 8) and 12 hybrid pigs were slaughtered following commercial procedures at commercial slaughterhouses closely located to our institute. The muscle longissimus dorsi, chilling at 2°C for 24 h, was removed at 2 h post-mortem. The meat was individually wrapped in plastic, coded and frozen at –40°C until completion of the slaughtering process.
Volatile Flavor Compounds Analysis
The muscle longissimus dorsi, removed of subcutaneous fat, was cut into rectangular pieces. 15 g of the meat was weighted into a 50 ml borosilicate glass reagent bottle sealed with a polytetrafluoroethylene (PTEE)-faced silicone septum, and cooked in an oil bath at 120°C for 40 min. After cooking, the reagent was left at 60°C in a water bath for 10 min to equilibrate its headspace, a SPME fiber coated with 75 μm carboxen/polydimethylsiloxane (CAR/PDMS) (Supelco, Bellefonte, PA, USA) which had been conditioned by heating in a GC injector port at 300°C for 60 min was inserted into the reagent bottle through the septum and exposed to the headspace above the sample for 30 min at 60°C. After extraction, the volatile flavor compounds were desorbed in the injector port of the GC at 250°C for 3 min. A SPME fiber coated with 100 μm PDMS (Supelco, Bellefonte, PA, USA) was used to extract the volatile flavor by the same methods.
The volatile flavor compounds were analyzed on a PE Clarus 600 GC/MS (PerkinElmer, Waltham, USA) equipped with Elite-5MS column (PerkinElmer; 30 m, ID 0.25 mm, film thickness 0.25 μm) using helium as carrier gas. The flow was maintained at 1 ml/min. The injector port was in splitless mode; the splitter was opened after 2 min. The temperature of column was kept at 40°C for 10 min, increased with 4°C/min to 240°C and kept at 240°C for 6 min. The inlet temperature was kept at 240°C, the ion source was 200°C. The mass spectrometer was operated in electron impact mode with an electronic energy of 70 eV and an emission current of 150 μA. The mass spectrometer scanned from m/z 50 to 500 at 0.25 scan/sec. Immediately before desorption, 0.1 μl of an internal standard (100 ng · μl−1 of 1,2-dichlorobenzene in methanol) was injected into the gas chromatograph. The mass spectra of the volatile flavor compounds were compared to the NIST Mass Spectral Search Program for identification. Approximate quantities of the aroma compounds were estimated by comparison of their peak areas with that of the 1,2-dichlorobenzene internal standard, obtained from the total ion chromatograms, using a response factor of one.
Statistical Analysis
Significant differences among the four pig breeds pork for each of the constituents were determined by one-way analysis of variance (ANOVA) using a SPSS Program, version 20.0 (IBM Inc., 2011). Principal component analysis (PCA) and stepwise linear discriminant analysis (SLDA) was performed using the same SPSS program. These techniques were applied to the normalized relative amounts of the volatile compounds. PCA is an unsupervised technique that reduces the dimensionality of the original data matrix retaining the maximum amount of variability,[Citation22] allowing the visualization of the different pork in a two dimensional space and identifying the directions in which most of the information is retained. It is therefore possible to explain the differences between different pork by means of factors obtained from the data sets and to determine which variables contribute the most for such differences. SLDA was used as a classification method to select variables in sequence to optimize the discrimination.[Citation23] SLDA, probably the most widely applied supervised pattern recognition method, searched for directions (discriminant functions) which achieve maximum separation among categories by maximizing the between-class variance relative to the within-class variance. SLDA minimizes the variance within categories and maximizes the variance between categories. The variables included in the analysis are determined with a SLDA using a Wilk’s Lambda as a selection criterion and an F-statistic factor to establish the significance of the changes in Lambda when a new variable is tested.
RESULTS AND DISCUSSION
Volatile Flavor Compounds
The concentration of each compound was added together for data analysis, and these compounds were adsorbed by the two SPME fibers. Thirty-three compounds were identified in the headspace of at least one breed at a concentration above 20 ng/100 g. These compounds included 13 aldehydes, 6 alcohols, 4 alkanes, 5 ketones, 2 furans, and 2 acids (). The concentration of the volatile flavor compounds was greatly different between the pork samples from these breed pigs, and the Chinese indigenous breeds shows the higher concentration than DLY. Two compounds - trans-2-undecene-1-ol and 3-hydroxy-2-butanone - are not found in the DLY. One-way analysis of variance showed that 13 compounds were not affected by breed, these compounds is Dodecanal, 14-octadecenal, trans-2-undecene-1-ol, 13-heptadecyn-1-ol,1-octanol,n-hexadcanoic acid, (E)-13-octadecenic acid, heptanone, 2-butanone, 1-hydroxy-2-propanone, 3-hydroxy-2-butanone, 2-pentylfuran, 2-ethylfuran, 2-hexylfuran. The other compounds are found affected by breed. The longissimus muscle from the Chinese indigenous breeds has the highest concentration of ketones. So the pork from the Chinese indigenous breeds is a green food.[Citation24] The compounds clearly identified were used for statistical treatments. All statistical treatments were performed on corrected peak area data (peak area/internal standard area).
Table 1 Code and mean concentrations of cooked longissimus muscle from different breeds in headspace by SPME
PCA
The 33 analytical variables used for statistical purposes were gathered into three different groups: aldehydes, alkanes, and acid and alcohols, furans and ketones. PCA from data matrix was then performed in each one of the different groups in order to find the main sources of variability and flavor compounds. Although the best procedure is to analyze all variables at one group, it was decided to subdivide the compounds into three groups of variables for the consistency of the results, maintaining the number of cases equal or higher than the number of variables.
Aldehydes
Applying PCA to the normalized relative amounts of the 13 analytical variables (aldehydes) and 32 objects (different breed pork), two principal components were extracted explaining 79.1% of the variance of initial data set. From the loading scores, it was found three variables having coefficients magnitude <0.5 — nonanal, decanal, and (E)-2-decenal — are insufficient to adequately describe the samples according to variety, and removed these variables from the matrix. The new set (data matrix 32×10) account for 93.5% of the total variance in the data. The first principal component (PC1) explains 61.4% of the variance in the initial data set and the second principal component (PC2) explain 32.1%. The eigenvalues, the cumulative percentage and percentage of variance explained by the two first principal components are showed in
Figure 1 PC1 vs. PC2 scatter plot of the main sources of variability between the pork samples: (a) distinction between the samples (scores) and (b) relation between the 13 aldehydes (loadings).
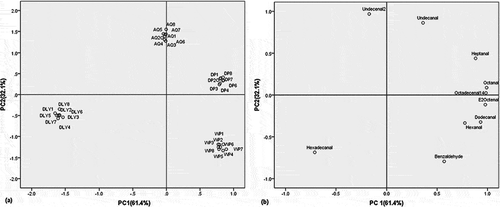
The projections of the samples along the directions identified by the first two PC’s, is reported in where the first principal component (PC1) of different pork samples is plotted against the second principal component (PC2). The separation of the different categories of the pork samples from this PC1-PC2 scatter point plot is obvious. This figure shows that pork from DP and WP varieties were separated by the second principal component, while pork from AQ variety are most influenced by the variables related with the second PC. The coefficient that defines the weight of the original variable in the PC’s can be investigated to understand which chemical compounds are responsible for the ranking of pork. Octanal (0.98), 14-octadecenal (0.97), (E)-2-octenal (0.96), dodecanal (0.94), and heptanal (0.87) were highly loaded on the first PC, while 2-undecenal (0.97) and undecanal (0.89) were loaded on the second PC explaining most of the variability. shows the corresponding loading plot that establishes the relative importance of each variable and it is therefore useful for the study of relations among the aldehydes and different pork breeds.
The samples of pork from varieties DLY are located in the third quadrant (negative PC1 and PC2). Only hexadecanal (–0.69, –0.71) is in accordance with this requisite. These samples show low values of 2-undecenal and undecanal and very low values of heptanal and all other variables influencing positively the first PC. The WP pork samples are essentially represented in the fourth quadrant (positive PC1 and negative PC2). Benzaldehyde (0.57, –0.81), hexanal (0.78, –0.34), dodecanal (0.94, –0.34) are the variables related with these samples (). They are characterized by low values of hexadecanal (–0.69, –0.71). The DP samples are located in the first quadrant (positive PC1 and PC2). Heptanal (0.87, 0.46), octanal (0.98, 0.12), 14-octadecenal (0.97, 0.05), (E)-2-octenal (0.96, –0.11) are strongly associated with this quadrant. The AQ samples are located on the positive X axis (positive PC2 and not relevant PC1). 2-Undecenal (–0.31, 0.97), Undecanal (0.32, 0.89) are the variables related with these samples.
Acids, alcohols, and alkanes
The 12 variables from the initial data matrix of acids, alcohols and alkanes explained 88.1% of the variance. From the loading scores it was found that three variables having coefficients magnitude <0.5 - limonene -are insufficient to adequately describe the samples according to variety, and removed the variables from the matrix. PCA shows a clear separation of different breed pork samples according to varieties when a data matrix (32×11) with these compounds--octane, 2,4-dimethyl-1-heptene, 4-methyloctane, 2-decene-1-ol, trans-2-undecene-1-ol, (E)-2-tetradecen-1-ol, 13-heptadecyn-1-ol, 1-octen-3-ol, 1-octanol, n-hexadcanoic acid, (E)-13-octadecenic acid — is used. The first two principal components account for 92.7% of the total variance of the initial data set. presents the eigenvalues, cumulative percentage and total variance explained by the two first principal components. The first component explains 73.1% of the variability in the data set and the second component explains 19.6%.
Table 2 Prediction capacity of pork samples discriminant model according to pork variety
The variables with the highest contribution to the first components, explaining 73.1% of total variance of data set, are octane (0.97), 2,4-dimethyl-1-heptene (0.94), 4-methyloctane (0.96), trans-2-undecene-1-ol (0.98), (E)-2-tetradecen-1-ol (0.99), 13-heptadecyn-1-ol (0.94) , n-hexadcanoic acid (-0.91) and (E)-13-octadecenic acid (-0.82). The second principal component (19.6%) is strongly correlated with 2-decene-1-ol (-0.88) and, in minor extent, 1-octen-3-ol (0.71), 1-octanol (0.65).
From the plot of the 32 breed pork samples on the plane defined by these first two principal components, the DP pork samples appear on the left side of the plane, grouped by variety, while samples of AQ, WP, DLY are found on the right side of the plane (). The first quadrant contains the WP and AQ samples. These samples are characterized by the variables associated to positive values of the two principal components mainly 1-octen-3-ol (0.66, 0.71), 1-octanol (0.71, 0.65). DLY breed pork samples are represented in fourth quadrant (positive PC1 and negative PC2). 2-Decene-1-ol (0.16, -0.88) is the only variable most related with this breed pork variety. The DP breed pork samples are located on the negative X axis (negative PC1 and not relevant PC2). N-Hexadcanoic acid (–0.91, 0.4), (E)-13-octadecenic acid (–0.82, 0.5) are the variables related with these samples.
Ketones and furans
PCA from the data matrix (32×8) built with ketones and furans, was also performed. It was observed 87.3% of the total variance of these compounds could be explained by the first two principal compounds (). The first principal component, explaining 68.8% of the variance, is mainly associated with 2-butanone (0.98), 2-pentylfuran (0.93), heptanone (0.87), 2-ethylfuran (0.89), while 1-hydroxy-2-propanone (0.78), 2-heptanone (0.59) contribute in great extent to the second principal component, explaining 18.5% of the total variance (). shows the scores scatter plot on the two first principal components, representing the differences among the 32 pork samples. represents the corresponding loading plot that establishes the relative importance of each ketones and furans, and is therefore useful for the study of relations among these compounds and the relations between these compounds and samples.
Figure 3 PC1 vs. PC2 scatter plot of the main sources of variability between the pork samples: (a) distinction between the samples (scores) and (b) relation between the eight Ketones and furans (loadings).
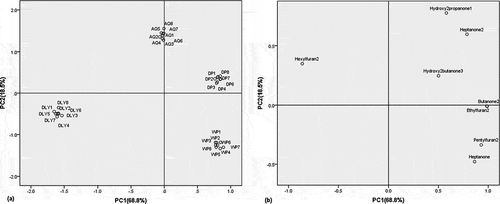
The DP breed samples appear on the first quadrant. These samples are characterized by the variables associated to positive values of the two first principal components being characterized, primarily, by 3-hydroxy-2-butanone (0.5, 0.24), 2-ethylfuran (0.98, 0) and 2-butanone (0.98, 0). DLY breed samples are represented in third quadrant (negative PC1 and negative PC2). The AQ samples are located on the positive X axis (positive PC2 and not relevant PC1). There is no these compounds associated with two places.
SLDA
After PCA, a SLDA was applied in order to obtain the most useful variables in the differentiation between pork samples and to find discrimination functions for the classification of new samples in the correct group. Forward selection method was employed using F to remove and to enter. Those variables which surpassed the predefined significance limit were excluded. The classification was performed according to pork samples: AQ, DLY, DP, and WP. Three statistically significant discriminant functions were obtained, explaining 100% of the variability; three variables had the highest F-value: 1-octanal/1-octen-3-ol and nonanal. So they were the most important variables for the differentiation of the pork samples from these four breeds. For differentiation of the pork samples according to these four breeds, three variables had the highest F-values: 1-octanal/1-octen-3-ol and nonanal. The percent of correctly classified pork samples with these variables was 100% (). Then the results can be considered satisfactory and acceptable and the selected variables are useful to classify and differentiate these pork samples by their variety.
CONCLUSIONS
In summary, this study shows that profile of volatile flavor compounds can be successfully used as discriminating parameter for identifying the breed of the pork samples. The whole analytical procedure based on SPME-GC-MS analysis is rapid and easy. The application of the stepwise LDA (based on Wilks’ Lambda criterion) method on the volatile compounds data set characterized by SPME-GC-MS resulted in predictive abilities of 100% as evaluated. All chemical groups investigated achieved a good separation according to breed, and 1-octanal/1-octen-3-ol and nonanal seem to be the most important groups for the characterization of pork samples. The variables most correlated with DLY pork samples are: hexadecanal (–0.69, –0.71) and 2-decene-1-ol (0.16, –0.88). Benzaldehyde (0.57, –0.81), hexanal (0.78, –0.34), dodecanal (0.94, –0.34) 1-octen-3-ol (0.66, 0.71) and 1-octanol(0.71, 0.65) are strongly associated to WP pork samples. DP pork samples are most associated with heptanal (0.87, 0.46), octanal (0.98, 0.12), 14-octadecenal (0.97, 0.05), (E)-2-octenal (0.96, –0.11), N-hexadcanoic acid(–0.91, 0.4), (E)-13-octadecenic acid(–0.82, 0.5) 3-hydroxy-2-butanone (0.5, 0.24), 2-ethylfuran (0.98, 0) and 2-butanone (0.98, 0). Undecanal (0.32, 0.89) and 2-undecenal (–0.31, 0.97) are the two variables most related with AQ pork samples.
ACKNOWLEDGMENT
This work was financially supported by the Eleventh Five-Year Plan Key Scientific and Technological Project of Anhui China (09020303086).
REFERENCES
- Margit, D.A.; Camilla, B.; Per, E.; Hanne, C.B.; Henrik, J.A. Cooking loss and juiciness of pork in relation to raw meat quality and cooking procedure. Food Quality and Preference 2003, 14 (4), 277–288.
- Meinert, L.; Christiansen, S.C.; Kristensen, L.; Bjergegaard, C.; Aaslyng, M.D. Eating quality of pork from pure breeds and DLY studied by focus group research and meat quality analyses. Meat Science. 2008, 80 (2), 304–314.
- Jun, Q.; Michael O.N.; Ning, W.; Claude, G.; Shiv, O.P. Pork quality and marbling level assessment using a hyperspectral imaging system. Journal of Food Engineering 2007, 83 (1), 10–16.
- Aaslyng, M.D.; Oksama, M.; Olsen, E.V.; Bejerholm, C.; Baltzer, M.; Andersen, G. Bredie, W.L.; Byrne, D.V.; Gabrielsen, G. The impact of sensory quality of pork on consumer preference. Meat Science 2007, 76 (1), 61–73.
- Wood, J.D.; Nute, G.R.; Richardson, R.I.; Whittington, F.M.; Southwood, O.; Plastow, G.; Mansbridge, R.; Da Costa, N.; Chang, K.C. Effects of breed, diet, and muscle on fat deposition and eating quality in pigs. Meat Science 2004, 67 (4), 651–667.
- Jeremiah, L.E.; Gibson, J.P.; Gibson, L.L.; Ball, R.O.; Aker d, C.; Fortin, A. The influence of breed, gender, and PSS (Halothane) genotype on meat quality, cooking loss, and palatability of pork. Food Research International 1999, 32 (1), 59–71.
- Lee, S.H.; Choe, J.H.; Choi, Y.M. Jung, K.C.; Rhee, M.S.; Hong, K.C.; Lee, S.K.; Ryu, Y.C.; Kim, B.C. The influence of pork quality traits and muscle fiber characteristics on the eating quality of pork from various breeds. Meat science 2012, 90 (2), 284–291.
- Ping, L.; Defa, L.; Jingdong, Y.; Liying, Z.; Zongyi, W. Flavour differences of cooked longissimus muscle from Chinese indigenous pig breeds and hybrid pig breed (Duroc×Landrace×Large White). Food Chemistry 2008, 107 (4), 1529–1537.
- Monald, S.; Mottram. Flavour formation in meat and meat products: A review. Food chemistry 1998, 62 (4), 415–424.
- Geesink, G.H.; Schreutelkamp, F.H.; Frankhuizen, R. Vedder, H.W.; Faber, N.M.; Kranen, R.W.; Gerritzen, M.A. Prediction of pork quality attributes from near infrared reflectance spectra. Meat Science 2003, 65 (1), 661–668.
- Toldra, F.; Flores, M. The use of muscle enzymes as predictors of pork meat quality. Food Chemistry 2000, 69 (4), 387–395.
- Ngadia, M.; Satyanarayan, R.S.D.; Vijaya, G.S. Raghavan and Samira Kazemi. Dielectric Properties of Pork Muscle. International Journal of Food Properties 2011, 6, 3.
- Pizarro, C.; Esteban-Díez, I.; Sáenz-González, C.; González-Sáiz, J.M. Vinegar classification based on feature extraction and selection from headspace solid-phase microextraction/gas chromatography volatile analyses: A feasibility study. Analytica chimica acta 2008, 608 (1), 38–47.
- Wies, C.; Dambergs, R.; Smith, P.; Cozzolino, D. Classification of Tempranillo wines according to geographic origin: Combination of mass spectrometry based electronic nose and chemometrics. Analytica Chimica Acta 2009, 660 (1–2), 227–231.
- Zhang, J.; Li, L.; Gao, N.; Wang, D.; Gao, Q.; Jiang, S. Feature extraction and selection from volatile compounds for analytical classification of Chinese red wines from different varieties. Analytica Chimica Acta 2010, 662 (2), 137–142.
- Trang, H.D.; Nguyena; Xi, C.W. Volatile, taste components, and sensory characteristics of commercial brand oyster sauces: Comparisons and relationships. International Journal of Food Properties 2012, 15, 134–144.
- Arthur, C.L.; Pawliszyn, J. SPME with thermal adsorption using fused silica optical fibers. Journal of Analytical Chemistry 1990, 62, 2145–2148.
- Lin Pan, J.; Chong, M.; Pawliszyn, J. Determination of amines in air and water using derivatization combined with solid-phase microextraction. Journal of Chromatography A 1997, 773 (1–2), 249–260.
- Xie, J.; Sun, B.; Zheng, F.; Wang, S. Volatile flavor constituents in roasted pork of Mini-pig, Food Chemistry 2008, 109 (3), 506–514.
- Kima, K.S.; Leeb, J.M.; Hong, C.H. Solid phase extraction (SPE) method for detection of irradiated meats, LWT-Food Science and Technology 2004, 37 (5), 559–563.
- Zhaoa, H.; Wanga, L.; Qiub, Y.; Zhoua, Z.; Zhongb, W.; Lib, X. Multi-walled carbon nanotubes as a solid-phase extraction adsorbent for the determination of three barbiturates in pork by ion trap gas chromatography–tandem mass spectrometry (GC/MS/MS) following microwave assisted derivatization. Analytica Chimica Acta 2007, 586 (1–2), 399–406.
- Clementi, S.; Cruciani, G.; Curti, G. Some applications of the partial least-squares method. Analytica Chimica Acta 1986, 191, 49–160.
- Jurado, J.M.; Ballesteros, O.; Alcázar, A.; Pablos, F.; Martín, M.J.; Vílchez, J.L.; Navalón, A. Characterization of aniseed-flavoured spirit drinks by headspace solid-phase microextraction gas chromatography-mass spectrometry and chemometrics. Talanta 2007, 72 (2), 506–511.
- Forss, D.A. Odour and flavour compounds from lipids. Programme for chemists in Fats and Other Lipids 1972, 13, 181–258.