ABSTRACT
The objective of this study was to develop a molecular predictive model from quantitative real-time polymerase chain reaction methods to describe the growth of S. aureus strains in artificially contaminated pork in storage dependent of a constant temperature (7–30°C). This model acquired by quantitative real-time polymerase chain reaction methods was compared to a conventional predictive model using data. This study used three of the main growth models to fit the growth equation. The results proved that Modified Gompertz, Logistic, and Richards models were adequate for describing the growth curves. These models had the very low rate of the growth of S. aureus in pork during a lag phase. The growth rate increased with temperature, and the lag time decreased. Lag phases were apparent in all models, and those samples stored at low temperatures had longer lag phases. There was no significant difference in the molecular and conventional predictive models for any of the growth curves. However, the use of a molecular predictive model could save more time and labor to construct more precise models of certain pathogens. In conclusion, the molecular predictive model could provide an effective method to lessen the risk of S. aureus of pork.
Introduction
Staphylococcus aureus, an opportunistic pathogenic gram-positive bacterium, is a causative agent of a wide group of infections ranging from superficial lesions to life threatening septicaemia.[Citation1] S. aureus is commonly found in the nostrils and on the skin and hair of warm blooded animals. It has been shown that approximately 30–50% of the human population are carriers. S. aureus can grow over a wide temperature range (7–48.5°C) with an optimum temperature of 30–37°C.[Citation2] This specialty enables S. aureus to grow on many types of foods.[Citation3] Thus, early recognition of S. aureus is a key issue for public health policy.
Predictive models have been utilized to analyze the population dynamics of pathogenic and spoiling bacteria at several temperatures for different lengths of time during food storage. Predictive models use methods such as experimental design, data collection, data analysis, and summary (e.g., growth rate, lag time, etc.) to enable the establishment of databases. With the development of predictive models and the advancement of computation,[Citation4] highly complicated specific models have been implemented. For instance, sigmoid functions have represented modified Gompertz, Logistic, and Richards models.[Citation5]
A characteristic microbial growth curve is composed of three stages: lag, exponential, and stationary.[Citation6] To confirm whether prediction supply a good description of growth in foods, models claim to be verified to assess their predictive capacity. As a consequence, in food processing operations, predictive mathematical modeling can be helpful in everyday decision making for hazard analysis critical control point performance and risk assessment in situations where process differences, improper handling, or temperature fluctuations have occurred.
Much research has been published utilizing many types of models revealing that different models have different performances. Juneja et al.[Citation7] estimated the goodness-of-fit of three primary models on the growth of Salmonella in chicken. Ding et al.[Citation8] used mathematical modeling (Gompertz, Logistic, and Baranyi model) on the growth of S. aureus in sandwiches. Ye et al.[Citation9] developed and validated a molecular predictive model (Gompertz, Baranyi, Logistic, and Huang model) to describe the growth of Listeria monocytogenes in vacuum-packaged chilled pork by real-time polymerase chain reaction (PCR). However, few published articles have been based on a comparative study of different predictive models of S. aureus in pork.
Although most of the raw data for predictive modeling originated from traditional microbiological methods, the approach is time consuming and labor intensive. Because meat can easily be bacteriologically contaminated and can be an ideal substrate for many types of spoilage microorganisms,[Citation10] it is difficult to correctly count the targeted bacteria in the absence of background bacterial flora.
Previous studies showed that antibiotic-resistant bacteria had been used to study growth features by utilizing a selective medium while adding varieties of antibiotics.[Citation11] Nevertheless, the method has not always been found to be suitable. The research also showed that the growth rates had significant differences between rifampicin-resistant and wild-type strains of E. coli O157:H7.[Citation12]
In the last few years, many varieties of rapid detection techniques for microbes have been determined for the definitive quantitation of the microorganisms of food products. With real-time PCR, the assay has proven to have enormous potential as a result of its high specificity and sensitivity.[Citation13] By setting the standard working curve, real-time PCR assays allow access to quantitative terms and S. aureus counting.[Citation14] Currently, as a traditional method, real-time PCR has the potential to be used.
All of the raw data originated from traditional microbiological counting assays for predictive modeling. Little research has reported on advancing molecular predictive modeling from quantitative real-time PCR detection assays, which is the aim of this work. As a consequence, the main purpose of this research was to advance molecular predictive modeling for describing the growth of S. aureus in pork in storage under constant temperature conditions. Additionally, a comparison between the molecular predictive modeling acquired by real-time PCR and conventional predictive modeling acquired by traditional microbiological methods, will be achieved. This study will assess the feasibility of molecular predictive modeling for the evaluation of the microbial safety of pork.
Materials and Methods
Bacterial Strains and Inoculum Preparation
In this study, three bacterial strains of S. aureus were used. S. aureus ATCC 25923 (food isolate) was acquired from the China Center of Industrial Culture Collection (Beijing, China). S. aureus (food isolate) was obtained from this laboratory. S. aureus (food isolate) was obtained from Jiangsu Entry–Exit Inspection and Quarantine Bureau.
The three strains of S. aureus were separately stored at –80°C in nutrient broth (NB) with 30% glycerol. As for the preparation of inoculum, frozen suspensions from each strain were dissolved and streaked onto selective agar (Baird Parker agar with egg yolk [BP-EY] agar with selective supplements; Land Bridge, Beijing, China) after the plates were incubated at 37°C for 48 h.
Single colonies from the BP-EY plate transferred to 6 mL tubes of tryptic soy broth (TSB) were incubated at 37°C for 4 h, and then, they were transferred to another fresh TSB tube and incubated at 37°C for 18 h. Three milliliters of all three strains of S. aureus (approximately 108 cfu·mL–1) were mixed, and then, the inoculum was prepared from a suspension diluted to 106 cfu·mL–1.
Samples Preparation
Samples (90% lean pork) were purchased from a local retail store. The outer pork layers removed by sterile surgical scissors were discarded. The inner pork samples were distributed into 20 g portions (approximately 6 cm × 6 cm × 1 cm) and packaged into sterile plastic bags. Then, each specimen was packaged by a vacuum-packaging machine (DC800-FBi-E, Promax packaging solution, USA) and then underwent high pressure (300 MPa, 10 min at 20°C) to inactivate the background flora.[Citation9] The effectiveness of this process was confirmed for eliminating background bacteria. Before being tray-packaged, after pressure treatment, each sample was inoculated with 100 μL of a solution with approximately 106 cfu·mL–1 of S. aureus. For the tray-packaged pork, the initial inoculum of S. aureus was approximately 103–104 cfu·cm–2.
Storage Conditions and Growth Measurements
Inoculated samples were incubated with different incubation temperatures (7, 10, 15, 20, 25, and 30°C) under isothermal conditions for different lengths of time. At each appropriate growth temperature, the samples were analyzed for the enumeration of S. aureus at frequent intervals. After each temperature was studied, six of the relative growth curves were accomplished.
S. aureus Counting
Each tray-packaged pork sample (20 g) was homogenized in 80 mL of sterile saline (0.9%) following shaking at 230 rpm for 10 min with a stomacher. Further measures were taken as detailed in the following text.
Traditional Microbiological Method
Viable counts were acquired by plating a dilution in sterile saline onto selective agar (BP-EY agar with selective supplements; Land Bridge, Beijing, China) after being incubated at 37°C for 72 h. We recorded an average cfu·mL–1 from two platings of each growth curve point to obtain better estimates of the growth kinetics.
SYBR Green Real-Time PCR Assay
DNA extraction
A 2 mL aliquot was shifted to a 2 mL sterile tube after being centrifuged (Avanti J-E, Beckman Coulter, USA) at 200 g (4°C) for 1 min. The supernatant (1 mL) was shifted to a sterile centrifuge tube (1.5 mL) under aseptic conditions, and then, another centrifugation was performed at 12,000 × g for 2 min (4°C). The pellet was stored at –20°C and used to further test the DNA extraction. Using the manufacturer’s instructions, with some revisions, bacterial DNA was extracted with a TIANamp Bacteria DNA Kit (Tiangen Biotech Beijing Co., Ltd., China). The revisions extended the incubation time of lysozyme (1 h) and proteinase K (2 h).
DNA standard curves
The oligonucleotide primers used in this study were synthesized by Invitrogen Life Technologies (Shanghai Yingjun Biotechnology Co. Ltd. China). The primers nuc-f (GCG ATT GAT GGT GAT ACG GTT) and nuc-r (AGC CAA GCC TTG ACG AAC TAA AGC) used in this study were based on previous work using the S. aureus-specific nuc (thermonuclease) gene.[Citation15] In real-time PCR, a 270 bp fragment of the nuc gene of S. aureus was used for amplification.
Optimization of real-time PCR condition: Bacterial DNA was extracted using the TIANamp Bacteria DNA Kit (Tiangen Biotech Beijing Co., Ltd., China) according to the manufacturers’ instruction. Reactions were carried out in MicroAmp optical eight-tube strips using an ABI Prism 7500 (Applied Biosystems, USA) sequence detection system. SYBR® Green I and ROX were used as reporter and passive reference dyes, respectively. The optimized real-time PCR (SYBR® Green) reaction mixture contained: 10 μL of 2× SYBR® Premix Ex TaqTM (Tli RNaseH Plus; TaKaRa Biotechnology Dalian Co., Ltd., China), 200 nM each primer, 0.4 μL of ROX Reference Dye II (TaKaRa), 2 μL of DNA and deionized sterile water to a final volume of 20 μL.
Specificity
After real-time PCR (SYBR® Green) amplification, melting curve analyses were carried out using ABI PRISM 7500 SDS software version 1.4 (Applied Biosystems). The PCR products were heated to 95°C for a period of 15 s, cooled at 60°C for 1 min, and then slowly heated back to 95°C for 15 s and finally 60°C for 15 s.
Standard curve
For the DNA standard curve, S. aureus ATCC 25923 DNA was serially diluted by a factor of 10 in deionized water, producing dilutions ranging from 90 ng to 9 pg of DNA for consistency with an nuc copy number of 107–103·μL–1. For each dilution, nine cycle threshold-values were generated (DNA extraction per sample in triplicate and real-time PCR per DNA extraction in triplicate) and included when calculating the standard curve.
Real-time PCR of artificially contaminated pork
Pork samples (20 g) were aseptically weighed into sterile plastic styrofoam trays. The tray-packaged pieces of pork were placed under ultraviolet light for 20 min, and then inoculated with 200 μL of different concentrations of S. aureus, and placed at room temperature. Controls consisted of uninoculated samples were treated identically to those inoculated.
The sensitivity of artificially contaminated samples was tested on the influence of constituents of pork. The concentrations of S. aureus inoculated on pork (20 g) ranged from approximately from 107 to 10° cfu·mL–1. DNA was extracted from artificially contaminated pork samples, and then subjected to real-time PCR. Viable counts were obtained simultaneously by plating a dilution made in deionized water onto selective culture media and then incubating at 37ºC for 48 h. Quantitative results for each were obtained from DNA standard curves. Simultaneously, traditional plating methods were performed for bacterial enumeration. By comparing the above two assays, the results were able to evaluate the detection accuracy of this method.
Growth Curve Models of S. aureus
Growth curves were produced by the natural logarithm of cfu·mL–1 versus time by the mean microbial counts at each time point from the five tests at constant temperatures. According to the non-linear equations (modified Gompertz, Logistic, and Richards), a variety of modeling methods were chosen as models for the growth dynamics and re-parameterized to describe the microbial growth parameters in .
Table 1. Mathematical growth models used to predict growth of S. aureus.
Modified Gompertz and Logistic models are derived from experiments and, on certain occasions, can overvalue the maximum growth rates. However, these models have been widely used.[Citation7,Citation12] To directly compare these models in this study, two additional biologically based growth models were used. Richards models had difficulty with fittings because parameter estimates went into an area where the function forecasted a large value so that an overflow error resulted. However, the Richards model with four parameters visibly gave reasonably good fits of the data and had been used previously.[Citation16]
Assessment of Model Performance
Correlation coefficients (R2), bias factors (Bf), accuracy factors (Af), the mean square error (MSE) and standard error of prediction (SEP) were calculated to assess the goodness-of-fit of the overall models. The goodness-of-fit of the original model was assessed by the corrected R2. The MSE was used to assess the difference between the growth data calculated by the model and that tested by the experimental method. The smaller the MSE, the better the model fit.
A validation test was performed to express the influence of the storage temperature (12, 16, 23, and 28°C) on the growth of S. aureus in pork. In the meantime, validation experiments were performed for assessing the models using Af and Bf. The Af shows the spread of the results from the predicted values. The Bf indicates the mean relative error of the predicted and observed bacterial growth. The equations provide a definition for R2, MSE, Af, Bf, and SEP.[Citation17,Citation18] The residual sum of squares (RSS; was defined by the difference between observed and predicted values) was counted for each growth curve.
Statistical Analysis
In the isothermal condition (7–30°C), the achieved S. aureus growth data were fitted to the models (modified Gompertz, Logistic, and Richards) by Marquardt iterative assay according to a non-linear regression procedure using Origin software (version 8.6; Microcal Software Inc., Northampton, MA). In the Origin software program, defining the starting values of the parameters was extremely important because if unsuitable values were used, the model could not converge and could result in parameter over-valuing or under-valuing. After several iterations according to the non-linear program, the given initial values converged and yielded estimated values of the parameters.[Citation16]
Results and Discussion
Real-Time PCR
Specificity of real-time PCR
Optimal primer concentrations, defined as the lowest Ct with the highest ΔRn (delta value of the normalized reporter signal minus the baseline signal) for the given target concentration of 0.9 ng, were 200 nM for each primer in real-time PCR (SYBR® Green). The nuc gene of the S. aureus strains used for the selection of bacterial DNA templates from non-target bacteria (from meat or meat products) had the anticipated enhancement of fluorescence, leading to 100% specificity. The mean peak Tm of the amplicons acquired with curves specific for the nuc gene of S. aureus tested (in triplicate) was 80.6°C ± 0.35 (ranging from 80.2 to 80.9°C). No amplification was seen, including either water or DNA from all of the other species, and no peak was acquired for them in the melting curve, confirming their use as negative controls (data not shown).
Standard curve
DNA standard curves were created using serial dilutions of S. aureus ATCC 25923 DNA in sterile water. DNA was extracted and subjected to real-time PCR to construct the standard curves. The linearity range of the DNA standard curve was from 90 ng to 9 pg (107–103 cfu·mL–1), covering five orders of magnitude. At the DNA level, the equation of the Log cfu·mL–1 versus the threshold cycle (CT) values obtained was y = –3.274x + 38.6, with an R2 value of 0.9998, and the reaction efficiency (E = 10–1/slope – 1)[Citation19] was 102.04%. Based on the information as described above, the R2 and E-values were acceptable for the method. The relevant coefficients of variation (CV), ranging from 0.05–0.28%, demonstrated the high precision of our real-time PCR (data not shown).
Quantitative detection of S. aureus in artificially contaminated pork samples
Pork having complex matrix influenced the efficiency of DNA extraction and PCR amplification.[Citation9] Artificially contaminated pork samples were investigated in order to assess the applicability of real-time PCR for direct detection and quantification of S. aureus. In the first case, the probability of S. aureus detection by real-time PCR was determined over the range from 107 to 10° cfu·mL–1. The actual detection limit of artificially contaminated pork was determined to be 10 cfu·mL–1 for real-time PCR (SYBR® Green, Tm: 80.4ºC ± 0.3). However, in previously published studies associated with real-time PCR, most of the methods had required one- or two-step enrichment procedures. The study confirmed real-time PCR (SYBR® Green) with pre-enrichment to detect Salmonella enterica in Serrano, and it was found that the detection limits were 102 cfu·g–1.[Citation20] Furthermore, quantification by real-time PCR relies on the efficiency and accuracy of the quantitative detection.
For detection of artificially contaminated pork, each cfu was obtained by real-time PCR using DNA calibration curves of S. aureus real-time PCR standards (data not shown). The results of real-time PCR (SYBR® Green) proved that each cfu was significantly different between the plate counts and real-time PCR (SYBR® Green) detection (data not shown).
Because of its high specificity and accuracy, real-time PCR has been widely used to detect a number of kinds of bacteria in meat and meat products.[Citation21] The disadvantages of the real-time PCR model had been the absence of distinction between live/dead cells; however, the study showed that the DNA-based quantitative real-time PCR assay was more accurate than ribonucleic acid-based assay when tested samples untreated.[Citation22] In other words, as a traditional method, real-time PCR has the potential to be used.
Primary Modeling of S. aureus Using Conventional Microbiological Methods and Real-Time PCR
In this study, the growth curves of a tube of three S. aureus strains on tray-packaged pork at different constant temperatures (7–30°C) were obtained. This research proved that the growth curves of S. aureus in tray-packaged pork (7–30°C) were acquired by traditional microbiological methods and real-time PCR (, , and ).
Figure 1. Growth curves of S. aureus on pork. a: Growth curves of S. aureus on pork at constant temperature (20°C) obtained using a conventional microbiological method and real-time PCR; b: Growth curves of S. aureus on pork at constant temperature (25°C) obtained using a conventional microbiological method and real-time PCR; c: Growth curves of S. aureus on pork at constant temperature (30°C) obtained using a conventional microbiological method and real-time PCR. The empty red circles represent the mean values of quintuplicate trials of raw data points using a conventional microbiological method; the solid red circles represent the mean values of quintuplicate trials of raw data points using real-time PCR. The red lines represent the modified Gompertz model; the black lines represent the Logistic model; the blue lines represent the Richards model.
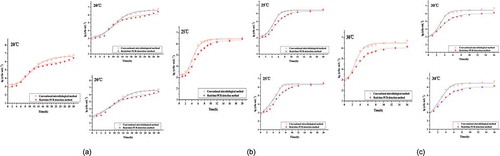
Three primary growth models (modified Gompertz, Logistic, and Richards) were used for fitting (, , and ). Although modified Gompertz and Logistic models invariably overvalue the maximum growth rate, they can both take the place of most of the empirical models and have been used previously.[Citation12] Therefore, modified Gompertz and logistic models were used as reference models. Richards models represented other more biologically based growth models were used in this study to directly compare these models.[Citation23] , , and showed that all of the isothermal growth curves were sigmoidal, following the exhibited lag, exponential, and stationary phases. Specifically, when incubated at 7–30°C, all three models (the modified Gompertz, Logistic, and Richards models) were applicable to describing the growth of S. aureus inoculated into tray-packaged pork samples. The three models fit molecular and traditional predictive growth curves.
The fitting figures of the three different models made clear that although there was a small deviation of the colony forming units from the original bacterial value, there were numbers of colony forming units of S. aureus similar to the stationary phase. In the lag phase (LT), the logistic and Richards models were similar to each other. However, in the exponential phase period transitioning into the stationary phase in comparison to the other models, the values of the modified Gompertz model were lower. The largest concentration of bacteria was not significant. The growth rate (log cfu·mL–1/h) increased with temperature and varied between 0.04 and 0.95 log cfu·mL–1/h, and the lag time decreased from 33 h to 1 h ().
Table 2. The growth parameters of S. aureus on pork calculated from modified Gompertz equation, modified Logistic equation, and Richards equation using a conventional microbiological method and real-time PCR.
S. aureus can grow over a wide temperature range (7.0–48.5°C).[Citation2] A large number of predictive mathematical models have been developed to predict the growth of pathogenic organisms during the storage of meat and meat products.[Citation17,Citation24] This study showed that the growth rate increased with temperature, and the lag time decreased. LTs were apparent in all models, and those samples stored at low temperatures had longer LTs than those at high temperatures. The growth rate and lag time were similar with the published ones which were listed by Lee et al.[Citation25] In this study, the rates derived from the molecular and conventional detection methods were compared for growth of S. aureus. In conclusion, in order to avoid that S. aureus reaching higher levels before consumption, meat and meat products can be stored at lower temperatures.
Secondary Modeling of S. aureus Using Conventional Microbiological Methods and Real-Time PCR
Specific growth rate (SGR) and LT calculated from three different model (the modified Gompertz, Logistic, and Richards model) formulas analyzed by non-linear regression were used to establish secondary models to express the influence of storage temperature on the growth of S. aureus in pork. The square root equation and natural logarithm equation constructed for SGR and LT, respectively, are shown in . Three secondary growth models (modified Gompertz, Logistic, and Richards) were used for fitting the LT model () and GR model (). The results showed that when tray-packaged pork inoculated with S. aureus and incubated at constant temperatures from 7–30°C, S. aureus grew as expected. The growth rate increased with temperature and the lag time decreased.
Table 3. The secondary models (specific growth rate and lag phase) of S. aureus on pork at constant temperature (7 ~ 30°) obtained using real-time PCR and conventional microbiological method.
Figure 2. Lag time of S. aureus in pork at constant temperature (7 ~ 30°C) obtained using a conventional microbiological method and real-time PCR. The solid red triangles represent the mean values of quintuplicate trials of raw data points using a conventional microbiological method; the solid red squares represent the mean values of quintuplicate trials of raw data points using real-time PCR. The red lines represent the modified Gompertz model; the black lines represent the Logistic model; the blue lines represent the Richards model.
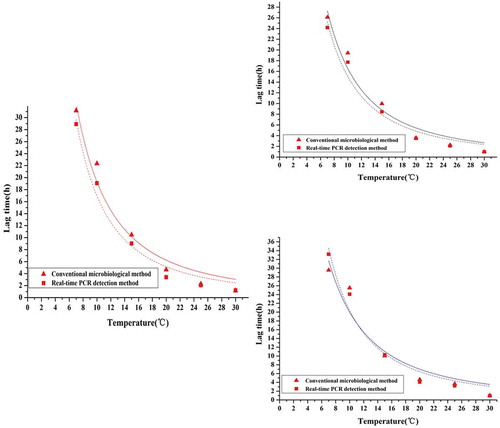
Figure 3. Specific growth rate of S. aureus in pork at constant temperature (7 ~ 30°C) obtained using a conventional microbiological method and real-time PCR. The solid red triangles represent the mean values of quintuplicate trials of raw data points using a conventional microbiological method; the solid red squares represent the mean values of quintuplicate trials of raw data points using real-time PCR. The red lines represent the modified Gompertz model; the black lines represent the Logistic model; the blue lines represent the Richards model.
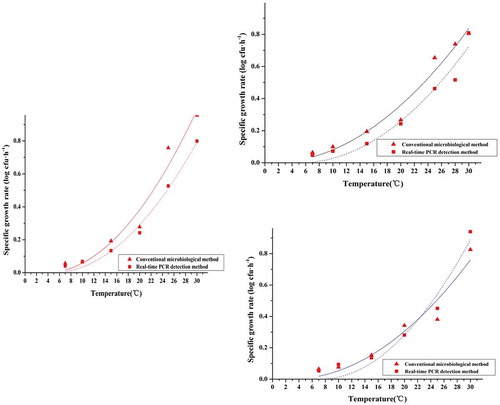
Primary Model Evaluation of S. aureus
Parameter values and performance statistics of three fitted equations at each measured temperature are shown in . The resulting R2 values were ≥0.9609 and the MSE values were ≤0.2799 log cfu·mL–1 in each of the three models, and thus, all met the defined requirements.[Citation26] The results proved that the modified Gompertz model had a good statistical fit with the observed data and that its R2 values were close to 1 among all three models. However, the differences of the three models were not significant.
Table 4. Fitting evaluation of growth models (modified Gompertz, Logistic equation, and Richards model) by real-time PCR and conventional microbiological method.
The approximated MSE values were within the precision of microbial enumeration, showing that three different models fit the isothermal growth data well. The small MSE value indicated that the model was accurate in depicting the effect of time on the colony forming units of S. aureus at a steady temperature.[Citation27] Therefore, the fitting results of all of the models were available.
An additional six data sets at each temperature were obtained to compare the observed values with the model predictive values, and the Bf and Af values were calculated by equations[Citation17,Citation18] to assess the performance of the developed models. Ross[Citation26] suggested that for models representing pathogen growth rate, Bf within a range of 0.9–1.05 could be considered to be good, within the range of 0.7–0.9 or 1.06–1.15 could be considered to be acceptable, and less than 0.7 or 1.15 could be considered to be unacceptable. From , all of the Bf and Af values were close to 1.0 and thus were in an acceptable range.[Citation28]
The objective indication of model performance was based on the indexes of bias and accuracy. A Bf less than 1 indicates that a model is, in short, fail-safe.[Citation18] Therefore, the Af should be calculated. As shown in , the average Af of the models obtained by the conventional microbiological method was 1.0452, and the average Af of the models obtained by real-time PCR was 1.0507. The results showed that the predictions were not different in observations in all of the models and that the predicted curves could accurately describe the growth of S. aureus on tray-packaged pork at a constant temperature.
Secondary Model Evaluation of S. aureus
Model evaluation is a necessary method to assess the interpolation capability of a newly developed model, and it is a key step in their development.[Citation29] The first stage is internal evaluation, which verifies the model against the data that is used for constructing the model.[Citation30] This assures that the model accurately indicates the data from which it was generated and describes any biological trend in those data.[Citation31] In this study, to evaluate the prediction accuracy of the secondary model (SGR and LT), a validation test was performed to express the influence of the storage temperature (12, 16, 23, and 28°C) on the growth of S. aureus in pork. All of the secondary models (SGR and LT) containing molecular and traditional predictive models were evaluated by correlation coefficient (R2), Bf, and Af values. The secondary model (SGR), containing molecular and traditional predictive models, was evaluated by the MSE, RSS, and %SEP.
First, the resulting R2 values were ≥0.9418 and the MSE (SGR) values were ≤0.0078 log cfu·mL–1 in each of the three models, and thus, all met the defined requirements.[Citation26] It can be seen that the modified Gompertz model provided a good statistical fit to the observed data, and its R2 values were close to 1 in all three models. However, the differences were often small. The estimated MSE (SGR) values were within the precision of microbial enumeration, indicating that the three different models fitted the isothermal growth data well.
Second, the Bf and Af are employed to evaluate the performance of the presented models, which are considered to be objective indications of the model performance.[Citation32] Bf is a measure of the extent of under- or over-prediction by the model of the SGR or LT observed.[Citation33] Usually, a Bf value of less than 1 indicates that the predicted value is higher than the observed value, which results in a fail-safe model. Similarly, a Bf with a value of more than 1 showing that the prediction leads to a lower level of confidence and is lower than that of the observed value, which is also dangerous and needs to be avoided. Bf is an important parameter for the model under consideration.[Citation32]
The average Bf values for the models (SGR and LT) obtained by the conventional microbiological method were 0.9696 and 0.9627, and those by the real-time PCR method were 0.9868 and 0.9625, respectively. The Bf values for the models denoted that the results were unreliable. Other published research also showed unsafe results. Jin et al.[Citation32] indicated that fail-safe predictions of Bf for a model developed for L. monocytogenes. Baert et al.[Citation34] showed that Bf values of 0.64–0.81 for SGR models depicted the effect of storage temperature on the growth rate and LT of 6 Penicillium expansum strains. However, Armas et al.[Citation35] suggested that Bf values within the range of 0.6 to 3.99 were acceptable for the SGR of pathogens and spoilage organisms. Dalgaard and Jorgensen[Citation29] showed that Bf values in the range of 0.75 to 1.25 should be regarded as successfully validated for modeling seafood spoilage microorganisms.
Generally, the model was considered to have high performance and accuracy if the value of Af was 1, whereas a larger value of Af results in a lower accuracy of the average estimate. In this study, the results showed that the average Af values for the models (SGR and LT) obtained by the conventional microbiological method were 1.068 and 1.0403 and that by the real-time PCR method were 1.022 and 1.0392, respectively. To date, there is no acknowledged standard for the Af. Ross et al.[Citation36] suggested that an acceptable Af could be based on the number of environmental parameters in a kinetic model. Jin et al.[Citation35] showed that the Af values calculated from SGR and LT models for L. monocytogenes in broth were 1.67 and 1.72, respectively. Ding, Dong, Jin, and Oh[Citation8] suggested that the Af value of 1.11 for an SGR model of L. monocytogenes in lettuce was treated with electrolysed oxidizing water. However, in this research, the Af of the models were good for validation compared to the mentioned references of other publications.[Citation26,Citation37]
Finally, the results showed that average SEP values of 3.8668 and 4.4434% for the models (SGR) were obtained by the conventional microbiological method and the real-time PCR method, respectively. The results also proved that average RSS values of 0.0249 and 0.0227 for the models (SGR) obtained by the conventional microbiological method and the real-time PCR method, respectively. In short, the smaller the SEP and RSS values, the better the fit.[Citation26]
Conclusion
Under the conditions of constant temperature (7–30°), this study evaluated and compared molecular predictive modeling with conventional predictive modeling for the growth of S. aureus in artificially contaminated pork. The models, based on the Modified Gompertz, Logistic, and Richards models, had the very low rate of the growth of S. aureus in pork during a LT. The growth rate increased with temperature, and the lag time decreased. LTs were apparent in all models, and those samples stored at low temperatures had longer LTs than those at high temperatures. The results of all of the experiments proved that there was no significant difference between the molecular predictive model and the traditional predictive model, and that the molecular predictive model could be used to predict the growth of S. aureus in artificially pork.
Acknowledgment
We are grateful to Dr. Ron Tume of CSIRO, Animal, Food, and Health Sciences, Australia for his valuable advice and assistance with English language.
Funding
This research was funded by National Natural Science Foundation of China (Grant No: 31071614, No: 31200422), the National Science & Technology Pillar Program during the Twelfth Five-year Plan Period of China (2012BAD28B03), Three Agricultural Projects of Jiangsu province of China (SX(2011)146).
Additional information
Funding
References
- Kluytmans, J.A.J.W.; Wertheim, H.F.L. Nasal Carriage of Staphylococcus Aureus and Prevention of Nosocomial Infections. Infection 2005, 33, 3–8.
- Schmitt, M.; Schuler-Schmid, U.; Scmidt-Lorenz, W. Temperature Limits of Growth, TNase, and Enterotoxin Production of Staphylococcus Aureus Strains Isolated from Foods. International Journal of Food Microbiology 1990, 11, 1–19.
- Le Loir, Y.; Baron, F.; Gautier, M. Staphylococcus Aureus and Food Poisoning: Review. Genetics and Molecular Research 2003, 2(1), 63–76.
- McMeekin, T.A.; Olley, J.; Ross, T.; Ratkowsky, D.A. Predictive Microbiology: Theory and Application; Research Studies Press: Tauton, UK, 1993.
- Zwietering, M.H.; Jongenburger, I.; Rombouts, F.M; Van’t, R.K. Modeling of the Bacterial Growth Curve. Applied and Environmental Microbiology 1990, 56(6), 1875–1881.
- Monod, J. The Growth of Bacterial Cultures. Annual Review of Microbiology 1949, 3(1), 371–394.
- Juneja, V.K.; Melendres, M.V.; Huang, L.; Gumudavelli, V.; Subbiah, J.; Thippareddi, H. Modeling the Effect of Temperature on Growth of Salmonella in Chicken. Food Microbiology 2007, 24, 328–335.
- Ding, T.; Dong, Q.L.; Jin, Y.G.; Oh, D.H. Study on Predictive Model of the Growth of Listeria Monocytogenes in Ready-to-Eat Lettuce Treated with Electrolyzed Oxidizing Water. International Journal of Food Science and Technology 2010, 31, 87–90.
- Ye, K.; Wang, H.; Zhang, X.; Jiang, Y.; Xu, X.; Zhou, G. Development and Validation of a Molecular Predictive Model to Describe the Growth of Listeria Monocytogenes in Vacuum-Packaged Chilled Pork. Food Control 2013, 32, 246–254.
- Jackson, T.C.; Acuff, G.R; Dickson, J.S. Meat, Poultry, and Seafood. In Doyle, M.P.; Beuchat, T.J.; Eds.; Food Microbiology: Fundamentals and Frontiers (pp. 83–100), ASM Press: Washington, DC, 1997.
- Oscar, T.P. Predictive Models for Growth of Salmonella Typhimurium DT104 from Low and High Initial Density on Ground Chicken with a Natural Microflora. Food Microbiology 2007, 24(6), 640–651.
- Huang, L.H. Growth Kinetics of Escherichia Coli O157: H7in Mechanically Tenderized Beef. International Journal of Food Microbiology 2010, 140(1), 40–48.
- Rodríguez-Lázaro, D.; Jofré, A.; Aymerich, T.; Garriga, M.; Pla, M. Rapid Quantitative Detection of Listeria Monocytogenes in Salmon Products: Evaluation of Pre-Real-Time PCR Strategies. Journal of Food Protection 2004, 68(7), 1467–1471.
- Fusco, V.; Quero, G.M.; Morea, M.; Blaiotta, G.; Visconti, A. Rapid and Reliable Identification of Staphylococcus Aureus Harbouring the Enterotoxin Gene Cluster (egc) and Quantitative Detection in Raw Milk by Real-Time PCR. International Journal of Food Microbiology 2011, 144, 528–537.
- Brakstad, O.G.; Aasbakk, K.; Maeland, J.A. Detection of Staphylococcus Aureus by Polymerase Chain Reaction of the nuc Gene. Journal of Clinical Microbiology 1992, 30, 1654–1660.
- Velugoti, P.R.; Bohra, L.K.; Juneja, V.K.; Huang, L.H.; Wesseling, A.L.; Subbiah, J. Dynamic Model for Predicting Growth of Salmonella spp. in Ground Sterile Pork. Food Microbiology 2011, 28(4), 796–803.
- Gunvig, A.; Hansen, F.; Borggaard, C. A Mathematical Model for Predicting Growth/No-Growth of Psychrotrophic C. Botulinum in Meat Products with Five Variables. Food Control 2013, 29(2), 309–317.
- Pal, A.; Labuza, T.P.; Diez-Gonzalez, F. Evaluating the Growth of Listeria Monocytogenes in Refrigerated Ready-to-Eat Frankfurters: Influence of Strain, Temperature, Packaging, Lactate and Diacetate, and Background Microflora. Journal of Food Protection 2008, 71(5), 1806–1816.
- Pfaffl, M.W. A New Mathematical Model for Relative Quantification in Real-Time RT-PCR. Nucleic Acids Research 2001, 29(9), e45.
- Miller, N.D.; Draughon, F.A.; D’Souza, D.H. Real–Time Reverse Transcriptase Polymerase Chain Reaction for Salmonella Enterica Detection from Jalapeno and Serrano Peppers. Foodborne Pathogens and Disease 2010, 7(4), 367–373.
- Brightwell, G.; Clemens, R. Development and Validation of a Real-Time PCR Assay Specific for Clostridium Estertheticum and C. Estertheticum-Like Psychrotolerant Bacteria. Meat Science 2012, 92(4), 697–703.
- Ye, K.P.; Zhang Q.Q.; Jiang, Y.; Xu, X.L.; Cao J.X.; Zhou G.H. Rapid Detection of Viable Listeria Monocytogenes in Chilled Pork by Real-Time Reverse-Transcriptase PCR. Food Control 2012, 25(1), 117–124.
- Darukaradhya, J.; Phillips, M.; Kailasapathy, K. Selective Enumeration of Lactobacillus Acidophilus, Bifidobacterium spp., Starter Lactic Acid Bacteria, and Non–Starter Lactic Acid Bacteria from Cheddar Cheese. International Dairy Journal 2006, 16(5), 439–445.
- Juneja, V.K.; Marks, H.; Huang, L.; Thippareddi, H. Predictive Model for Growth of Clostridium Perfringens During Cooling of Cooked Uncured Meat and Poultry. Food Microbiology 2011, 28(4), 791–795.
- Lee, Y.J.; Jung, B.S.; Kim, K.T.; Paik, H.D. Predictive Model for the Growth Kinetics of Staphylococcus Aureus in Raw Pork Developed Using Integrated Pathogen Modeling Program(IPMP) 2013. Meat Science 2015, 107, 20–25.
- Ross, T. Indices for Performance Evaluation of Predictive Models in Food Microbiology. Journal Applied Bacteriology 1996, 81(5), 501–508.
- Giffel, M.C.; Zwietering, M.H. Validation of Predictive Models Describing the Growth of Listeria Monocytogenes. International Journal of Food Microbiology 1999, 46(2), 135–149.
- Yang, Z.Q.; Jiao, X.A.; Li, P.; Pan, Z.M.; Huang, J.L.; Gu, R.X.; Fang, W.M.; Chao, G.X. Predictive Model of Vibrio Parahaemolyticus Growth and Survival on Salmon Meat As a Function of Temperature. Food Microbiology 2009, 26(6), 606–614.
- Dalgaard, P.; Jorgensen, L.V. Predicted and Observed Growth of Listeria Monocytogenes in Seafood Challenge Tests and in Naturally Contaminated Cold-Smoked Salmon. International Journal of Food Microbiology 1998, 40, 105–115.
- Baranyi, J.; Roberts, T. A Dynamic Approach to Predicting Bacterial Growth in Food. International Journal of Food Microbiology 1994, 23(3–4), 277–294.
- Bang, W.S.; Chung, H.J.; Jin, S.S.; Ding, T.H.; Wang, I.G.; Woo, G.J.; Ha, S.D.; Bahk, G.J.; Oh, D.H. Prediction of Listeria Monocytogenes Growth Kinetics in Sausages Formulated with Antimicrobials As a Function of Temperature and Concentrations. Food Science and Biotechnology 2008, 17, 1316–1321.
- Jin, S.S.; Jin, Y.G.; Yoon, K.S.; Woo, G.J.H.; Wang, I.G.; Bahk, G.J.; Oh, D.H. Predictive Modeling of the Growth and Survival of Listeria Monocytogenes Using a Response Surface Model. Food Science and Biotechnology 2006, 15, 715–720.
- Dong, Q.L.; Tu, K.; Guo, L.Y.; Li, H.W.; Zhao, Y. Response Surface Model for Prediction of Growth Parameters from Spores of Clostridium Sporogenes Under Different Experimental Conditions. Food Microbiology 2007, 24, 624–632.
- Baert, K.; Valero, A.; Meulenaer, B.D.; Samapundo, S.; Ahmed, M.M.; Bo, L.; Debevere, J.; Devlieghere, F. Modeling the Effect of Temperature on the Growth Rate and Lag Phase Of Penicillium Expansum in Apples. International Journal of Food Microbiology 2007, 118, 139–150.
- Armas, A.D.; Wynne, A.; Sutherland, J.P. Validation of Predictive Models Using Independently Published Data. In Abstracts: 2nd International Conference on Predictive Microbiology, Hobart, Tasmania, Australia, February 18–22, 1996.
- Ross, T.; Dalgaard, P.; Tienungoon, S. Predictive Modelling of the Growth and Survival of Listeria in Fishery Products. International Journal of Food Microbiology 2000, 62, 231–245.
- Neumeyer, K.; Ross, T.; Thomson, G.; McMeekin, T.A. Validation of a Model Describing the Effects of Temperature and Water Activity on the Growth of Psychrotrophic Pseudomonads. International Journal of Food Microbiology 1997, 38, 55–63.