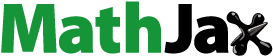
ABSTRACT
Particulate matter (PM) is a major primary pollutant emitted during wildland fires that has the potential to pose significant health risks to individuals/communities who live and work in areas impacted by smoke events. Limiting exposure is the principle measure available to mitigate health impacts of smoke and therefore the accurate determination of ambient PM concentrations during wildland fire events is critical to protecting public health. However, monitoring air pollutants in smoke impacted environments has proven challenging in that measurement interferences or sampling conditions can result in both positive and negative artifacts. The EPA has performed research on methods for the measurement of PM2.5 in a series of laboratory-based studies including evaluation in smoke. This manuscript will summarize the results of the laboratory-based evaluation of federal equivalent method (FEM) monitors for PM2.5 with particular attention being given to the Teledyne-API Model T640 PM Mass monitor, as compared to the filter-based federal reference method (FRM). The T640 is an optical-based PM monitor and has been gaining wide use by state and local agencies in monitoring for PM2.5 U.S. National Ambient Air Quality Standards (NAAQS) attainment. At present, the T640 (includes both T640 and T640×) comprises ~44% of the PM2.5 FEM monitors in U.S. regulatory monitoring networks. In addition, the T640 has increasingly been employed for the higher time resolution comparison/evaluation of low-cost PM sensors including during smoke impacted events. Results from controlled non-smoke laboratory studies using generated ammonium sulfate aerosols demonstrated a generally negative T640 measurement artifact that was significantly related to the PM2.5 concentration and particle size distribution. Results from biomass burning chamber studies demonstrated positive and negative artifacts significantly associated with PM2.5 concentration and optical wavelength-dependent absorption properties of the smoke aerosol.
Implications: The results detailed in this paper will provide state and local air monitoring agencies with the tools and knowledge to address PM2.5 measurement challenges in areas frequently impacted by wildland fire smoke. The observed large positive and negative artifacts in the T640 PM mass determination have the potential to result in false exceedances of the PM2.5 NAAQS or in the disqualification of monitoring data through an exceptional event designation. In addition, the observed artifacts in smoke impacted air will have a detrimental effect on providing reliable public information when wildfires occur and also in identifying reference measurements for small sensor evaluation studies. Other PM2.5 FEMs such as the BAM-1022 perform better in smoke and are comparable to the filter-based FRM. Care must be taken in choosing high time resolution FEM monitors that will be operated at smoke impacted sites. Accurate methods, such as the FRM and BAM-1022 will reduce the burden of developing and reviewing exceptional event request packages, data loss/disqualification, and provide states with tools to adequately evaluate public exposure risks and provide accurate public health messaging during wildfire/smoke events.
Introduction
Due to environmental regulations including setting new and revising existing National Ambient Air Quality Standards (NAAQS, EPA Citation2013), PM2.5 concentrations have declined over the past decades (Aguilera et al. Citation2021; Lurmann, Avol, and Gilliland Citation2015; McClure and Jaffe Citation2018) except in wildfire prone areas (Aguilera et al. Citation2021; McClure and Jaffe Citation2018). Western U.S. wildfires have been increasing in size and intensity in recent decades due to factors including climate change and land management practices (Landis et al. Citation2018; U.S. Department of Agriculture [USDA] Citation2016; USDA Citation2014; Westerling Citation2016; Westerling et al. Citation2014; Yue et al. Citation2013). Wildland fire smoke is a major source of fine particulate matter with a mass median aerodynamic diameter <2.5 μm (PM2.5) that is released into the ambient air (Reid et al. Citation2005; Urbanski et al. Citation2018; Xu et al. Citation2022) resulting in adverse effects on air quality and posing a significant risk to human health (Aguilera et al. Citation2021; Gan et al. Citation2017; Gupta et al. Citation2018; Liu et al. Citation2015; McClure and Jaffe Citation2018). Limiting exposure is the principal measure available to mitigate negative public health outcomes and air quality impacts of smoke. Therefore, the accurate determination of PM2.5 concentrations during wildland fire events provides information necessary for time critcial mitigation strategies to reduce health impacts and protect public health. Additionally, new non-regulatory monitoring methodologies based upon small sensor technology are also gaining traction resulting in a critical need for accurate monitoring in both smoke and non-smoke impacted ambient air (Holder et al. Citation2020; Kelleher et al. Citation2018; Landis et al. Citation2021; Mehadi et al. Citation2020; EPA Citation2019).
The U.S. Federal Reference Method (FRM) for the determination of PM2.5 in the Atmosphere (Noble et al. Citation2001; EPA Citation1997) is technically sound (Peters et al. Citation2001), has served for decades in monitoring attainment to NAAQS for PM2.5, and has been used as a reference for comparison of other monitoring methods (Le et al. Citation2020). The integrated nature of the FRM, where a sample is collected on Teflon® filter media over a 24-h period with subsequent gravimetric determination of the collected mass, results in data not being available for weeks or even months after the sampling period has ended. Higher time resolution (1–60 min), continuous PM2.5 monitoring methods have been developed and, in some cases, when designated as Federal Equivalent Methods (FEM) by the EPA, can be used in lieu of the FRM for monitoring attainment to the PM2.5 NAAQS. The transient and rapidly changing nature of wildfire smoke events makes higher time resolution FEM monitoring methods desirable to track those changing conditions, communicate to the public (AirNow Citation2022), and assess the performance of non-regulatory sensors, which also operate on higher time resolution (i.e., minutes). In addition, the capability of automated high-time resolution PM2.5 monitors can be incorporated into online platforms and provide real-time information visualization of data to monitoring agencies, air quality researchers, and to the public. Prior to being designated as an FEM, candidate methods must perform to prescribed standards relative to the FRM, per requirements detailed in 40 CFR Part 53. Generally, FEM designated PM2.5 monitors correlate well and agree with FRM results over 24-h sampling periods. However, under some sampling conditions, including at higher PM2.5 concentrations (>80 μg m−3) and during smoke impacts, significant divergence is observed (Hagler et al. Citation2022; Landis et al. Citation2021; Le et al. Citation2020).
This study investigates the measurement artifacts associated with the Teledyne-API Model T640 PM Mass Monitor, an EPA designated FEM, in smoke-free laboratory chamber-based studies and during controlled burn experiments in a large-scale combustion chamber. The T640 is an optical-based PM monitor and has been gaining wide use by state and local agencies in monitoring for PM2.5 NAAQS attainment. At present (2022), the T640 (includes both T640 and T640×) comprises ~44% of the PM2.5 FEM monitors in U.S. regulatory monitoring networks. In addition, the T640 has increasingly been employed for the higher time resolution comparison/evaluation of low-cost PM sensors including during smoke impacted events. As such, understanding the performance capabilities of the T640 both in and out of smoke impacted environments is desirable. Hourly averaged T640 PM2.5 mass concentration results were directly compared to collocated filter-based FRM results collected over identical time periods. Additional measurements of PM2.5 mass (using an alternate designated FEM), particle size distributions, aerosol optical properties, and individual burn modified combustion efficiencies (MCE; Urbanski Citation2013) were also calculated to assist in identifying significant factors contributing to the observed artifacts. Based on the results from the measurements, the magnitude of the observed artifact was quantified under various controlled smoke and non-smoke conditions, provides statistically significant covariates to the T640 measurement artifacts, and discusses potential underlying causes of the artifacts.
Methods and study locations
Overview of methods evaluated
In this study, the continuous PM2.5 mass measurement results from the Teledyne T640 were compared to collocated filter-based FRM samples collected over 1-hr sampling periods in a series of chamber experiments. These experiments included the generation of polydisperse ammonium sulfate ((NH4)2SO4) particles into a laminar flow clean air stream and biomass burning smoke using different fuels and burning conditions under static conditions. All instruments/samplers were operated according to their FRM and FEM designation. The measurement principle, designation (FRM versus FEM), and deployment information for the instruments/samplers under investigation and additional measurements used in this manuscript are summarized in , Table S1, Table S2 and are described in Sections 2.1.1-2.1.6. Initial studies in the absence of smoke were carried out at the EPA Office of Research and Development Human Studies Research facility in Chapel Hill, North Carolina in March 2018. Laboratory-based chamber burns at the U.S. Forest Service (USFS) Fire Sciences Laboratory (FSL) in Missoula, Montana were conducted during April 2018, April 2019, and April 2021 campaigns. Fuels for the laboratory-based chamber burns consisted of ponderosa pine, larch, and Douglas fir needles, cones, and fine woody debris. Details of the Chapel Hill and Missoula chambers are provided in Sections 2.2.1 and 2.2.2, respectively.
Table 1. PM measurement methods.
Teledyne API T640
The Teledyne API (San Diego, CA, USA) Model T640 is a real-time, continuous PM mass monitor that uses scattered light spectrometry for measurement. The T640 is an EPA designated FEM for PM2.5 mass (EPA Citation2016). The T640 continuously samples ambient air at a volumetric flow rate of 5 l min−1 through a non-size selective inlet followed by passage through a sample conditioning downtube where a heater is used to ensure that the sampled aerosol’s relative humidity (RH) does not exceed 35%. The T640 estimates PM2.5 mass concentration based on an undisclosed proprietary algorithm which converts measured aerosol light scattering (σsp) in the white light wavelengths range, to PM2.5 mass concentration. Aerosol light scattering is generated using a polychromatic LED light source. Concentrations are measured by the T640 on a 1-min average time scale and the 10-min rolling average of PM2.5 mass concentration is reported at a resolution of 0.1 μg m−3. During the chamber studies (), the T640s conducted 60 separate 1-min PM2.5 concentration measurements per hour which were then averaged for comparison to 1-hr integrated samples obtained from the reference FRMs (section 2.1.3). T640 data were collected and stored through internal data storage and via an Envidas Ultimate (DR DAS, Granville, OH, USA) data acquisition system (DAS). During all chamber study periods, T640s were collocated near (~1 m) FRM reference samplers. Four individual T640 units (serial numbers 294, 295, 296, and 661) were deployed at various times during this study and are detailed in Table S1.
Met One BAM 1022
1-h average PM2.5 mass concentrations during the 2021 chamber study were obtained using the Met One Model BAM-1022 (Grants Pass, OR, USA) as given in . The BAM-1022 is an EPA designated FEM for PM2.5 (EPA Citation2015). The BAM-1022 continuously samples ambient air through an EPA-approved louvered PM10 inlet at a volumetric flow rate of 16.7 l min−1. The sampled PM10 aerosol is further fractionated into a PM2.5 aerosol downstream of the inlet via an EPA-approved very sharp cut cyclone (VSCC-Mesa Labs, Butler, NJ, USA). Downstream of the VSCC, a heater ensures that the RH of the PM2.5 aerosol does not exceed 35%. The resulting size fractionated, RH conditioned aerosol is then collected on a glass-fiber filter tape and its mass is measured by the aerosol attenuation of a 14C beta radiation source and mass concentration determined by dividing the sampled mass by the volume of air sampled. The BAM-1022 employs an in-line sampling geometry in which the attenuation of beta rays across filter media is measured and particulate matter is sampled simultaneously, resulting in higher time resolutions (15 min; ConcS) and samples for a longer proportion of the time (59 versus 42 min) than the previous generation BAM-1020 (Met One Citation2021, Citation2022). However, for the purposes of this study, PM2.5 mass concentrations are reported by the BAM-1022 on an hourly basis (ConcHR). BAM-1022 data were collected and stored through internal data storage and via an Envidas Ultimate DAS. The BAM-1022 was collocated near (~1 m) a T640/FRM pair.
Filter-based FRM
PM2.5 reference measurements were performed according to EPA FRM criteria as described in 40 CFR Part 50 Appendix L. Samples were collected using Tisch Environmental (Cleves, OH, USA) Model TE-WILBUR filter-based FRM samplers as given in . Per 40 CFR Part 50 Appendix L, FRM sampling protocol dictates that samples be collected over a 24-h time period at a flow rate of 16.7 l min−1. However, for the purposes of this study, FRM samples were collected over 1-h sampling periods to maximize the number of samples collected over short study periods and due to the high PM concentrations generated in the chambers during this study. Each PM2.5 Tisch FRM used a standard EPA PM10 louvered inlet coupled with a BGI (Model VSCCA) VSCC for PM2.5 fractionation. FRM samplers were collocated near (~1 m) T640 and BAM-1022 monitors. Four individual FRM samplers (serial numbers 16, 20, 21, and 22) were deployed during the studies and are detailed in Table S1.
FRM sample filter media consisted of Measurement Technology Laboratories (MTL, Minneapolis, MN, USA) Model PT47P polytetrafluoroethylene (PTFE) 2 µm pore size pressure drop equivalent membrane filters with a hydro-inert support ring. Filters were weighed in accordance with EPA Guidance Document 2.12 (EPA Citation2016) for monitoring PM2.5 in ambient air in the EPA National monitoring program reference weighing laboratory (Research Triangle Park, NC, USA). Filters were weighed both before and after sample collection by an MTL Model AH1 automated robotic weighing instrument. Prior to weighing, filters were equilibrated for a minimum of 24-h in a temperature and humidity controlled clean room. The AH1 used a Mettler-Toledo (Columbus, OH, USA) Model XP2U micro balance with a readability of up to 0.1 μg. The response of the micro balance was checked every ten filters by verification of Class 0 weight standards from Rice Lake Weighing Systems (Rice Lake, WI, USA). Filter weights were performed in triplicate and were accepted as valid if the response to the weight standards was ± 5 µg. FRM sampler operation and pre-/post-sample filter weighing during this study period have been described previously by Krug et al. (Citation2021).
PM size distribution measurements
Ambient aerosol size distributions were monitored using a TSI Incorporated (Shoreview, MN, USA) Model 3321 Aerodynamic Particle Sizer (APS) as detailed in Table S2. During Chapel Hill chamber experiments, the instrument was placed on the chamber floor and in the FSL chamber burn sampling the APS was placed on a table within the designated smoke chamber sampling area and located within 2 m of the T640s and FRMs. The instruments were configured with the Stokes correction off, the lower channel bound 0.487 μm, and the upper channel bound 20.535 μm. During the Chapel Hill study, the sampling time was set to 5 sec and the density was set to 1.7 g cm−3 (ammonium sulfate), and during the FSL chamber studies the sampling time was set to 10 sec and the density was set to the default value of 1.0 g cm−3 (unknown smoke aerosol density). The APS calculated mass median aerodynamic diameter (MMAD) values were extracted from the mass (dM) exported files to investigate the effect of aerosol size distribution on T640 response. The APS was factory cleaned and calibrated by TSI in 2018, 2019, and 2021 prior to each year’s sampling campaign.
Magee AE33 Aethalometer
Continuous optical black carbon (BC) aerosol measurements were made using a Magee Scientific (Berkeley, CA, USA) Model AE33 seven wavelength spectrum Aethalometer equipped with a Magee Scientific Model 5610 sample stream dryer and BGI (Waltham, MA, USA) Model SCC-1.829 sharp cut PM2.5 cyclone as given in Table S3. Reported BC concentrations represent the self-adsorption compensated BC6 (880 nm) infrared channel. Other reported channels include self-adsorption compensated BC1 (370 nm); BC2 (470 nm); BC3 (520 nm); BC4 (590 nm) BC5 (660 nm); and BC7 (950 nm). Performance of the AE33 light emitting diode (LED) optical benches were verified prior to and after each chamber testing campaign using the Magee Scientific Model 7662 neutral density optical filter validation kit. The Magee AE33 measurement principle and real-time loading effect compensation algorithm based on two parallel spot measurements has been described previously by Drinovec et al. (Citation2015). Results obtained from the AE33 are indicative of the optical properties of the smoke aerosols and for the purposes of this paper were averaged to the 1-Hr filter-based FRM sample periods. Delta-C or ΔC (Allen, Babich, and Poirot Citation2004) is an objective measure of the wavelength (λ) dependence of aerosol optical absorption between the AE33 ultraviolet (UV; 370 nm) and infrared (IR; 880 nm) channels and was calculated using EquationEquation 1(1)
(1) .
CO/CO2 measurements
Carbon monoxide (CO) and carbon dioxide (CO2) were determined at 1 hz resolution with a Picarro (Santa Clara, CA, USA) Model G2401 Cavity Ringdown Spectroscopy (CRDS) instrument. Data was recorded via internal data storage. A three-point calibration using gas mixtures of CO and CO2 in scientific grade zero air was run daily to maintain accuracy of the CRDS measurements. CRDS CO/CO2 data was used in determining MCE of individual burns as described in Landis et al. (Citation2021).
Temperature, pressure, and flow calibration of measurement instruments
Leak checks and calibrations of sampler temperature, pressure, and flow rates were performed at the beginning and midway through each study period followed by post-study flow/temperature/pressure verifications. All calibrations and verifications/audits were performed using the Mesa Labs BGI (Butler, NJ, USA) TetraCal®. To ensure that the same periods were sampled by all instruments, internal analyzer/sampler clocks were synchronized at the beginning of each sampling day.
Chamber-based study site locations
2018 Chapel Hill ammonium sulfate aerosol chamber study
Initial testing of the T640 versus the PM2.5 FRM in the absence of smoke was carried out at the EPA Office of Research and Development human studies research facility in Chapel Hill, North Carolina (Ghio et al. Citation2012) as part of the EPA Wildland Fire Sensor Challenge (Landis et al. Citation2021). Tests were conducted over a predetermined range of target PM concentrations at varying temperature and relative humidity conditions as described in Landis et al. (Citation2021). Single component PM2.5 test aerosols consisting of ammonium sulfate ((NH4)2SO4) were generated using a TSI Model 9306 Six-Jet Atomizer. The T640 and FRM sampled from a 4.8 × 5.8 × 3.2 m stainless-steel exposure chamber with a single-pass laminar flow (ceiling to floor) air system with approximately 40 air changes per hour (113 m3 min−1). The outside air was purified by passing through a bed of Purafil® (KMnO4 on an alumina substrate) and a bed of Purafil Corporation (Doraville, GA) Puracol® activated charcoal, dehumidified, passed through a bed of Hopcalite® (copper and magnesium oxide), and sent to the Clean Air Plenum. The chamber air was taken from the Clean Air Plenum, brought to the proper test protocol conditions by being heated/cooled and humidified with deionized water. Target pollutants were injected into the air stream before passing through a mixing baffle and entering the top of the chamber. The filter-based FRM and the T640 continuous monitor sampled from a shared port, which penetrated the side of the chamber.
USFS Missoula FSL burn chamber studies
The laboratory-based burn chamber studies were performed at the U.S. Forest Service’s combustion testing facility at the FSL in Missoula, Montana by EPA and USFS personnel. These static chamber burns occurred in the spring of 2018 (April 16–24, 2018; 33 burns; Landis et al. Citation2021; Whitehill et al. Citation2022), the spring of 2019 (April 15–26, 2019; 31 burns; Krug et al. Citation2021), and again in the Spring of 2021 (April 12–24, 2021; 40 burns; Long et al. Citation2021). For the purposes of this manuscript, the three chamber burn study periods were combined into a single data set since the studies were procedurally identical with only minor differences in fuels and burning conditions. The main combustion chamber is a square room with internal dimensions 12.4 × 12.4 × 19.6 m high and a total volume of 3000 m3 and has been described previously (Christian et al. Citation2004; Landis et al. Citation2021). Each T640 instrument was collocated with a PM2.5 FRM sampler at a distance of approximately 1 m. Fuel beds consisting of ponderosa pine, larch, and Douglas fir needles and mixed woody debris were prepared and placed in the middle of chamber. The amount and moisture content of the fuels were varied to generate different flaming/smoldering conditions during the burns. During the chamber burns, the combustion room was sealed and the fuel bed was ignited. Two large circulation fans on the chamber walls and one on the ceiling facilitated mixing and assured spatially homogeneous conditions during the burn periods (Landis et al. Citation2021). The chamber is not hermetically sealed and some air exchange with the outdoor ambient air does occur resulting in a slow dilution with ambient air during containment of the smoke. A slow decrease in the particle concentration due to dilution with ambient air over the 1-hr sampling period will not be dissimilar to the dynamic conditions of ambient air monitoring during smoke events. In general, chamber RH values were below 50% facilitating dry burning condition.
Statistical analysis
Data processing and all statistical analyses were performed using SAS v.9.4 (SAS Institute, Cary, NC, USA). FEM instrument PM2.5 measurement accuracy versus the FRM was calculated using EquationEquation (2)(2)
(2) , and the precision of collocated FRM and FEM samplers was calculated using the coefficient of variation or relative standard deviation using EquationEquation (3)
(3)
(3) .
Where X is the reported FEM concentration and R is the FRM concentration.
Where = mean of collocated PM2.5 concentrations,
= the sum of square of differences between individual collocated PM2.5 concentrations and the mean, and n = the number of collocated PM2.5 observations.
Parametric statistics used in this analysis include polynomial (e.g., linear, quadratic, cubic) least squares regression and multilinear regression (MLR) analysis. The assumptions of the parametric procedures were examined using residual plots, skewness, and kurtosis coefficients, Shapiro–Wilk test, and the Brown–Forsythe test. Nonparametric statistics used in this analysis include the Wilcoxon sign rank test. A level of significance of α = 0.05 was used for all statistical procedures unless otherwise stated. The SAS UNIVARIATE, CORR, REG, and GLM procedures were used for testing data distributions for normality and hypothesis testing Mu0 = 0 (population mean is not significantly different from 0), developing scatter plot matrices and calculating Spearman rank correlation coefficients, least squares general linear model regressions, and MLR analysis, respectively.
The MLR analysis models were evaluated to ensure the residuals from the statistical model were approximately Normally distributed with mean zero and constant variance. The dependent and independent variables in the Chapel Hill (NH4)2SO4 chamber study MLR analysis were log transformed to ensure the model residual distribution was not significantly different from Normal (Shapiro–Wilk test), and a constant value of 1 was added to the MMAD independent variable to avoid values <1 prior to transformation. No data transformations were necessary for the Missoula FSL smoke chamber MLR analysis as the model residuals met the parametric procedure assumptions. Some of the independent variables from the Missoula FSL smoke chamber studies were found to be correlated so independent variable interaction terms were included in the MLR analysis. Initially, all independent variables (chamber measurements) and all possible interactions were included in the MLR analysis. A stepwise MLR model optimization was conducted where non-significant independent variables and interactions were sequentially removed until all remaining terms were significant.
Results and discussion
Controlled chamber ammonium sulfate aerosol studies
The 1-Hr average PM2.5 mass concentration results from the Chapel Hill chamber-based studies using ammonium sulfate aerosols are given in . The Chapel Hill chamber studies were conducted over a 4-day period with the first runs of each day being a chamber zero (runs 1, 8, 15, and 22 in ) where no aerosols were introduced into the chamber as described by Landis et al. (Citation2021). Significant differences (p < 0.0001) in the results obtained from the T640 as compared to the FRM during the non-smoke controlled chamber studies were observed with the T640 under estimating PM2.5 mass. Linear regression results of the FRM versus T640 PM2.5 mass results during the Chapel Hill chamber runs are given in and indicates that the T640 on average measured~15% lower than the FRM. The FRM and T640 PM2.5 results are highly correlated with a coefficient of determination (r2) value of 0.992. Regression statistics of the comparison of PM2.5 mass concentration results during the Chapel Hill controlled chamber studies are given in Table S4. The results given in Table S4 indicate that only slight improvements in r2 are obtained when higher order polynomial models (e.g., quadratic, cubic) are fit. The accuracy of the T640 as compared to the FRM calculated by EquationEquation 1(1)
(1) during the Chapel Hill chamber study was 78.2 ± 10.2% (mean ± standard deviation).
Controlled chamber burns
The 1-Hr average PM2.5 mass concentration results from the 2018, 2019, and 2021 Missoula FSL burn chamber studies are given in , respectively. As previously stated (Table S1), multiple T640s and FRMs were operated in the burn chamber during each year’s study period. The T640 results given in represent the mean of the results obtained from the two T640s that were collocated in the chamber during each burn. Similarly, the FRM results given in represent the mean of the results obtained from the two (2018) or three (2019 and 2021) FRMs that were collocated in the chamber during each burn. Collocated precision and absolute percent differences (APD) values for both the T640s and FRMs operated during each study year are presented in Table S5 and in all cases were ≤5% showing individual sampler agreement across the chamber and no observed monitor to monitor biases. Unlike the Chapel Hill (NH4)2SO4 aerosol study results, during the FSL chamber burns the T640 regularly (87 out of 104 burns) measured higher PM2.5 mass concentrations compared to the FRM. Since similar fuels were used and similar environmental and burn conditions were employed across all three burn study periods (2018, 2019, and 2021), the data sets were combined into a single data set for statistical analysis. Significant differences in the results obtained from the T640 as compared to the FRM during the smoke chamber studies were observed in each study period (p <0 .0001 to p = .019) as well as the aggregate combined data (p < 0.0001).
Figure 2. T640 and FRM PM2.5 mass concentration averages (1-Hr) from the (a) 2018 (b) 2019 and (c) 2021 Missoula FSL burn chamber studies.
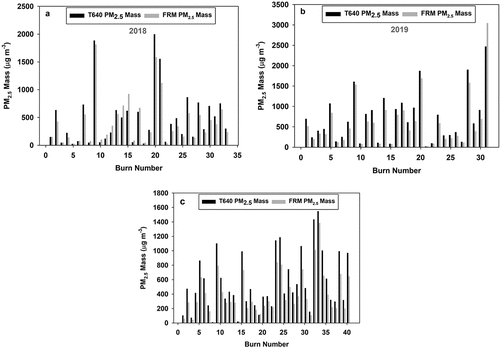
Linear regression results of the FRM versus T640 PM2.5 mass concentrations the Missoula FSL burn chamber studies along with uncertainties in both the FRM and T640 measurements are depicted in and summarized in Table S4. Although the linear regression model shows significant correlation (r2 = 0.916) it does appear that higher order polynomial models better fit the data. shows regression results using quadratic and cubic models. Both the quadratic and cubic models show a better correlation with the best fit being generated by the cubic model (r2 = 0.965 and intercept y0 = −2.69). Several of the higher PM2.5 concentration points (>1500 μg m−3) appear to be driving the quadratic/cubic relationship between the FRM and T640 results (). Uncertainty in the T640 measurement
results is also greater at higher concentrations, as indicated in . The accuracy of the T640 as compared to the FRM calculated by EquationEquation (1)(1)
(1) during the 2018, 2019, and 2021 Missoula FSL chamber burns and for the combined data set is summarized in Table S6. The study integrated overall T640 accuracy was 65.4 ± 17.4%. The highest accuracy was achieved during the 2019 study (71.3 ± 13.0%) and the lowest during the 2021 study (58.0 ± 17.6%).
Figure 3. Scatter plot with linear regression statistics of 1-Hr FRM versus T640 PM2.5 mass concentration results from the Missoula chamber studies.
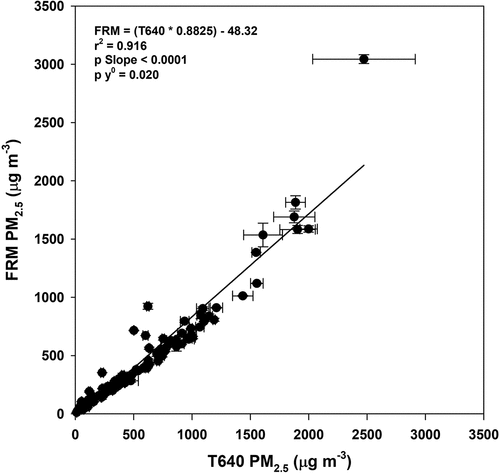
Figure 4. Scatter plot with (a) quadratic and (b) cubic regression statistics of 1-Hr FRM versus T640 PM2.5 mass concentration results from the Missoula Chamber Studies.
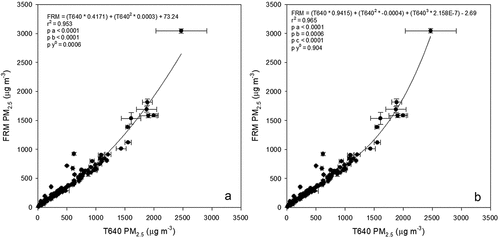
Past studies at ambient air monitoring sites that were impacted by smoke from nearby fires have resulted in the measurement of short-term 1-Hr PM2.5 mass concentrations in the 1,000 to 3,000+ μg m−3 range (Guyot et al. Citation2021; Landis et al. Citation2021). Laing and Jaffe (Citation2019) reported several wildfire smoke impacted sites measuring daily PM2.5 concentrations >250 μg m−3 over multiple day periods, including the highest PM2.5 concentrations ever recorded by a U.S. regulatory monitor (642 μg m−3 at Seeley Lake, MT during 2017 Rice Ridge fire). It is reasonable to assume with 24-Hr PM2.5 averages exceeding 250 μg m−3 and approaching 650 μg m−3, that short-term PM2.5 averages could approach and exceed 1000+ μg m−3 similar to those reported during the Fort McMurray Horse River Wildfire in Alberta, Canada by Landis et al. (Citation2021). The burn chamber generated hourly PM2.5 concentrations reported in this study ranging from 21.3 to 3044.6 μg m−3 (as measured by the FRM) are within levels observed during ambient air studies at wildfire smoke impacted sites. However, the highest point observed (3044.6 μg m−3) would be an exception at an ambient site and result only in circumstances where the fire line was extremely close to the ambient monitoring site.
As previously described, during the 2021 chamber studies a Met One BAM-1022 was operated in the chamber collocated with a T640 and FRM. gives the 1-Hr average PM2.5 mass concentration obtained from the FRM and BAM-1022 during the 2021 Missoula smoke chamber study and the results of the linear regression of FRM versus BAM-1022 results during the period, respectively. As indicated, the FRM versus the BAM-1022 comparison is linear and highly correlated (r2 = 0.999). The BAM-1022 is significantly different from the FRM (p < 0.0001) and does on average underestimate PM2.5 mass concentrations compared to the FRM by ~15% (46.2 ± 41.6 μg m−3) over all concentration ranges. Regression statistics of the comparison of PM2.5 mass concentration results for all study periods and the combined data are summarized in Table S4. The accuracy of the BAM-1022 as compared to the FRM was 88.6 ± 4.7% (Table S6). These results indicate that even under high concentration smoke events, the BAM-1022 predictably responds with the FRM.
Methodological influence on the observed T640 PM2.5 artifact
For the purposes of this work, the analytical artifact (both positive and negative) in the T640 PM2.5 mass determination, termed ΔFRM, is estimated using EquationEquation 3(3)
(3) as the difference between the mean FRM and the mean T640 PM2.5 mass concentration results for the same chamber run/burn period:
During the Chapel Hill chamber studies ΔFRM values were always positive as the T640 underestimated PM2.5 mass as compared to the FRM. Alternatively, the Missoula chamber burns resulted in both positive and negative ΔFRM values with the majority (87 out of 104 or ~84%) being negative. The magnitude and direction of ΔFRM for both the Chapel Hill runs and Missoula chamber burns are given in , respectively. depicts the scatter plots and presents the linear regression statistics for the FRM versus the magnitude of the artifact from the Chapel Hill and Missoula chamber studies. For the Chapel Hill (NH4)2SO4 study, the magnitude of the artifact is simply ΔFRM since all the values were positive. For the Missoula smoke chamber burns, the magnitude of the artifact is represented by the absolute value of ΔFRM (| ΔFRM|) since some values of ΔFRM were positive, and others were negative. As indicated, during both the Chapel Hill and Missoula chamber studies, the magnitude of ΔFRM is positively correlated with increases in PM2.5 concentration as measured by the FRM. As mentioned previously, the T640 estimates PM2.5 mass concentration via an algorithm which converts measured light scattering signal (Bsca) to PM2.5 mass. As such, any factor that might affect the ability of the T640 to measure Bsca reliably and predictably across its designed wavelengths or significant changes in aerosol physical properties (e.g., optical cross section, density) will result in a sampling or Bsca to PM2.5 mass calibration model artifact. We hypothesize that multiple factors may contribute to the observed sampling artifact associated with the T640 both in smoke and during the (NH4)2SO4 aerosol studies. These factors include particle size distribution effects (inability of T640 to accurately quantify ultra-fine particles); attenuation of incident and scattered light at high aerosol concentrations; non-uniform wavelength (λ) dependent optical properties or density changes of the sampled aerosol; and effect of environmental conditions (chamber temperature and RH) on the T640 mass determination. MLR analysis was used to investigate possible factors including those listed above that may contribute to the observed T640 instrument measurement artifact (ΔFRM). Tables S7 and S8 list all of the variables included in the MLR analysis of both the Chapel Hill (NH4)2SO4 and Missoula smoke chamber study results, respectively.
Figure 6. Time series showing the magnitude and direction of the T640 measurement artifact, ΔFRM from a) the 2018 Chapel Hill ammonium sulfate aerosol studies and b) the 2018, 2019, and 2021 Missoula FSL chamber burns. The chamber zeros performed at the beginning of each day were removed from the Chapel Hill data set.
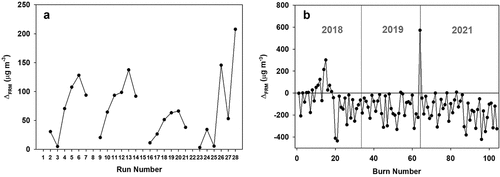
Figure 7. Scatter plot with linear regression statistics of 1-Hr ΔFRM versus FRM PM2.5 mass concentration results from a) the 2018 Chapel Hill ammonium sulfate aerosol studies and b) the 2018, 2019, and 2021 Missoula FSL chamber burns.
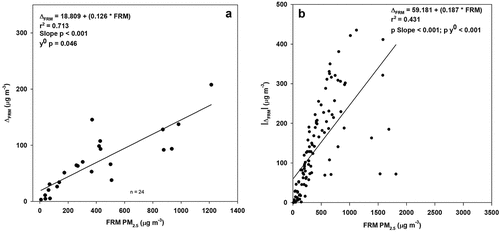
Chapel Hill chamber study MLR analysis
During the Chapel Hill chamber study, a single component aerosol ((NH4)2SO4) was used as the test media. As a result, the optical properties of the aerosol were constant over all runs and negating the need to make optical property measurements during this portion of the study. As described above and displayed in , the magnitude in the T640 artifact increases with increases in PM2.5 mass concentration. As such PM2.5 mass concentration was included as a variable in the MLR analysis. The effects of particle size distribution were investigated using the TSI model 3321 APS reported mean MMAD for each run. MMADs for the Chapel Hill runs ranged from 0.867 to 1.05 μm (Table S7). It should be noted that the Model 3321 APS begins to lose counting efficiency at particle sizes below 0.8 microns aerodynamic diameter (Peters, Ott, and O’Shaughnessy Citation2006; Volckens and Peters Citation2005). As a result, the MMADs provided by the APS is likely biased high for the Chapel Hill study due to a large concentration of particles being generated with aerodynamic diameters less than 0.8 microns. Two runs (23 and 26) were excluded from the MLR analysis due to APS 3321 instrumental issues. Ambient (chamber) temperature and RH were also included as independent variables due to their potential to affect the deliquescence and efflorescence properties of the aerosol which in turn may influence light scattering (Lyamani, Olmo, and Alados-Arboledas Citation2008; Tang Citation1996) and mass determination by the T640. Table S7 gives the variables used and gives the MLR analysis results from the 2018 Chapel Hill chamber runs.
Table 2. T640 ΔFRM MLR analysis results from the 2018 Chapel Hill chamber runs.
Overall, the model could explain ~88.3% (R2 = 0.883) of the variability in ΔFRM. The PM2.5 mass concentration (FRM) and MMAD were found to be significant variables with p values of <0.0001 and 0.005, respectively. Both temperature and RH were not significant, which was not surprising as the T640 is designed to compensate for those parameters through the heated sample conditioning downtube described in section 2.1.1. PM2.5 mass concentration as measured by the FRM is most significant in explaining the variability in ΔFRM per the results given in . We hypothesize that high PM2.5 concentrations result in attenuation of incident and/or scattered light in the detection cell of the T640 ultimately resulting in a measurement artifact. Light attenuation by aerosols, smoke included, is generally caused by two factors: 1) light scattering and 2) light absorption (Foster Citation1959). For the Chapel Hill chamber runs, the generated (NH4)2SO4 aerosols are non-light absorbing (Peñaloza-Murillo Citation1998) meaning light attenuation is the result of scattering. In the T640 detector cell, the light source and detector are configured at a 90° angle from each other (Teledyne-API Citation2019), and light scattering generated by the presence of aerosols in the cell is detected at that angle. Under normal operation light is scattered randomly in all directions, but the T640 algorithm takes that into account in estimating mass concentrations. As the number density of aerosols increases in the detection cell at high PM concentration both the incident light and scattered light may scatter (re-scatter) in directions other than 90° to a point where the PM2.5 mass calibration algorithm is no longer accurately estimating PM mass, resulting in an artifact. The positive sign associated with the estimated value for the FRM (PM2.5 mass concentration) variable (0.9036, ) further validate the observations displayed in , that increases in PM2.5 mass concentration corresponding to increases in the T640 artifact (ΔFRM). MMAD was also significant in explaining the variability in ΔFRM however to a less extent than PM2.5 concentration. It is well documented that particle size distribution influences aerosol scattering efficiencies (Curtis et al. Citation2007; Grimm and Eatough Citation2009; Lyamani, Olmo, and Alados-Arboledas Citation2008; Weinert et al. Citation2003). However, the effect is opposite, with a negative estimate value (−4.3163, ) than that observed with the FRM variable in that decreases in MMAD result in increases in the ΔFRM.
Missoula chamber burn MLR analysis
The MLR analysis results for the Missoula chamber burns are given in . The variables used in the Missoula chamber MLR analysis are given in Table S8. Similar to the Chapel Hill (NH4)2SO4 aerosol runs, PM2.5 mass (FRM), temperature, and RH were included as variables. Model 3321 APS MMADs were obtained on a limited number of runs (APS was not operated during 2018 chamber burns and burns 1–9 of the 2021 study period). In contrast to the Chapel Hill runs, both positive and negative values for the T640 artifact (ΔFRM) were obtained (). As previously stated, no transformations were performed on the Missoula chamber data prior to MLR analysis. Also, in contrast to the Chapel Hill study, smoke is a complex aerosol with many components resulting in significant changes to aerosol optical properties from burn to burn. To investigate the effect of aerosol optical properties on the T640 artifact, two additional variables were included in the MLR analysis. First, black carbon (BC) concentrations obtained from the self-adsorption compensated BC6 (880 nm) IR channel (Drinovec et al. Citation2015) of the Magee AE33 Aethalometer. As previously described, light attenuation by aerosols, smoke included, is generally caused by light scattering and light absorption (Foster Citation1959). BC is a highly effective at attenuating light through absorption (Cappa et al. Citation2019; Healy et al. Citation2015) and biomass burning is a major source of BC in the atmosphere (Cheng et al. Citation2022; Li et al. Citation2019). As such, it is reasonable to hypothesize that significant concentrations of BC in smoke may result in an artifact in the T640 response. For similar reasons hypothesized in the previous section during the non-smoke Chapel Hill studies, light attenuation by scattering at high biomass burning aerosol loading may also result in an artifact. shows the time series of BC concentrations during the Missoula chamber burns. The BC average over the 104 chamber burns was 58.9 μg m−3 with maximum values reaching as high as 552.8 μg m−3 ( and Table S8).
Figure 8. Time series showing 1-Hr average a) BC concentrations and b) ΔC during the 2018, 2019, and 2021 Missoula FSL chamber burns.
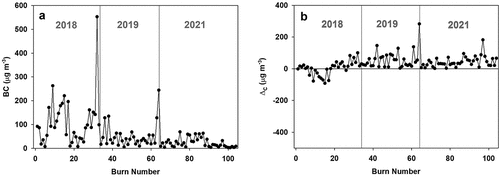
Table 3. T640 ΔFRM MLR analysis results from the Missoula chamber burns.
Biomass burning aerosols are predominantly organic aerosol (OA; 60–95%, Urbanski et al. Citation2022), some of which are light absorbing and are labeled as brown carbon (BrC). BrC are known for an absorption spectrum that decreases from near-UV to visible wavelengths (Laskin, Laskin, and Nizkorodov Citation2015). The composition of BrC is poorly characterized and its optical properties are highly variable (Kirchstetter, Novakov, and Hobbs Citation2004; Laskin, Laskin, and Nizkorodov Citation2015; Saleh et al. Citation2013). Additionally, OA coatings on BC can increase the absorption by BC through a “lensing” effect (Lack et al. Citation2012). Therefore, the second variable related to the optical properties of the smoke aerosol included in the MLR analysis was ΔC which is the differential absorption between the Aethalometer UV (370 nm) and IR (880 nm) channels and is thus a measure of BrC. ΔC has been used in past source apportionment studies to differentiate between fossil fuel and biomass burning BC emitting combustion sources (Allen, Babich, and Poirot Citation2004; Wang et al. Citation2010; Wang et al. Citation2011a, Citation2011b).
gives a time series of ΔC values from the Missoula chamber burns. For the most part ΔC values trend positive, which is consistent with past studies. However, during certain periods, mainly during the 2018 chamber burns, ΔC values were negative. This period is dominated by negative ΔC values, also corresponded to the period where ΔFRM values were positive or where the T640 was underestimating PM2.5 mass compared to the FRM (). During the early part of the 2018 burn period, oven dried fuels were employed with moisture content below 5%. In addition, on many of the burns where ΔFRM was positive and ΔC negative, fine woody debris comprised >90% of the biomass consumed. However, the biomass smoke that resulted in the T640 results underestimating as compared to FRM results was produced by fuel conditions (oven dried with moisture content below 5%) that will be rare in the natural environment. Finally, modified combustion efficiencies (MCE) of each burn were included as a variable in the MLR analysis to determine the contribution and significance of combustion conditions on the T640 response. Past studies have indicated that combustion conditions may affect optical properties of the produced smoke aerosol (Garg et al. Citation2016; Martinsson et al. Citation2015, Citation2017) which in turn may be significant in explaining the artifact in the T640 mass determination.
As given in , the optimized MLR generated model for the Missoula FSL chamber burns could explain ~85% (R2 = 0.8499) of the variability in ΔFRM when using ΔC, FRM PM2.5, and MCE as independent variables. PM2.5 mass concentration (FRM), ΔC, and MCE were found to be significant variables all with p <0.0001. In addition, the interactions between ΔC and MCE (ΔC*MCE) and PM2.5 mass concentration and BC (FRM*BC) were also found to be significant with p of <0.0001. As in the Chapel Hill studies, temperature, and RH were not significant and as such were removed from the model as part of the optimization process. In addition, BC concentration (BC), was also not significant in explaining the variability in ΔFRM even though the interaction between the FRM and BC (FRM*BC) variables was significant. During the Missoula burn chamber studies, ΔC was the most significant variable interaction in explaining the variability in ΔFRM per the results given in . Similar to the Chapel Hill studies in the absence of smoke, we anticipate that light scattering at high PM2.5 and BC concentrations are contributing to attenuation of incident and scattered light resulting in an artifact. However, the complex composition of smoke aerosols, also introduces wavelength-dependent light absorbing species operationally defined as BrC that also appear to be significantly contributing to the observed T640 measurement artifact along with other markers of aerosol optical properties. The physical properties of fuel beds such as moisture content, particle size, packing density, geometry, and total mass affect combustion conditions (e.g., the relative mix of flaming/smoldering combustion) and the emitted species (Urbanski et al. Citation2022). Previous studies have found the light-absorption and the wavelength dependence of absorption of smoke aerosol to vary considerably with fuels and MCE (McMeeking et al. Citation2014) thus offering possible explanations of the ΔC*MCE and FRM*BC interactions. Our data suggest that unlike the generated (NH4)2SO4 aerosol study, in fresh burn chamber generated biomass smoke, the particle size distribution was not significant in explaining variability in ΔFRM (using the 57 burns for which we have data) and was thus removed from the model during the optimization process. As stated previously, the MMAD calculated using the APS is most likely biased high due to inefficiencies of the analyzer at aerodynamic diameters below 0.8 microns. Particle size distributions of fresh biomass burning smoke generally lie in the ultrafine and accumulation mode (Hosseini et al. Citation2010; Reid et al. Citation2005) with significant mass from particles with aerodynamic diameters below 0.5 microns. As such, it is reasonable to assume that MMAD may be significant if data accounting for these smaller particles were included in the MLR analysis, or may be one of the reasons MCE (a potential surrogate for MMAD) was significant.
After reviewing the Chapel Hill and Missoula Chamber MLR results on the impact of PM2.5 mass on ΔFRM, the data from two other unrelated ambient studies that EPA participated and included T640 and collocated FRM or beta attenuation-based FEM measurements were reviewed. ΔFRM was calculated for 4 hr integrated data collected from Logan, UT, USA during the Utah Winter Fine Particle Study (UWFPS; U.S. NOAA Citation2017; Franchin et al. Citation2018) and ΔBAM was calculated from 4 hr integrated data collected at the U.S. Embassy in Sarajevo, Bosnia and Herzegovina (Hagler et al. Citation2022) to evaluate their relationship to PM2.5 mass. Data summaries are presented in Supplemental Information Tables S9 and S10 and scatter plots with linear regression statistics of FRM/BAM versus T640 PM2.5 mass concentration and ΔFRM/ΔBAM FRM versus FRM/BAM PM2.5 mass concentration results for each study are presented in Supplemental Information Figures S1 and S2. In both ambient studies evaluated there is a strong relationship between PM2.5 measured by the FRM/BAM versus the T640 (p < 0.0001; r2>0.97) and the ΔFRM/BAM are positively correlated with increases PM2.5 concentration (p < 0.0004) as measured by the FRM/BAM. The amount of ΔFRM/BAM variance explained by the PM2.5 mass concentration ranged from 36% (Logan) to 61% (Sarajevo), which is consistent with our chamber study findings. The UWFPS results included biomass smoke impacts from wood burning for home heating which may have imparted additional T640 artifact covariates similar to our Missoula chamber studies reducing the explanitary power of the ΔFRM versus FRM PM2.5 mass concentration simple linear regression model.
Implications
Wildland fires (wildfires and prescribed fires) emit significant amounts of primary PM2.5 into ambient air posing significant risk to human health and having adverse effects on air quality in downwind population centers. The transient nature and rapidly changing conditions along with the complex make up of emitted pollutants in smoke, can result in significant measurement challenges. High time resolution, rapidly available, and accurate PM2.5 monitoring data are sought after for use in smoke mitigation strategies to reduce exposure and communicate health risks to the public. Accurate, hourly measurements of PM2.5 are essential to a variety of retrospective activities – human health studies, evaluation of smoke and air quality models, and the identification/demonstration of wildfire produced “exceptional events” that affect air quality with respect to the NAAQS (EPA Citation2013). PM2.5 monitoring data have also seen wide use to investigate the performance of small sensors under smoke impacted conditions. Small sensors generally operate on higher time resolutions (1–60 min) and often utilize optical (light scattering) measurement principles similar to the T640. The findings from this research effort raise concerns that routine regulatory monitoring and wildland fire research study PM2.5 measurements utilizing optical measurement monitors such as the T640 may be reporting measurement artifacts (both positive and negative) during smoke impacted events for which data end users need to be cognizant. Studies have employed T640 or similar optical based PM mass monitors for evaluating/calibrating the performance of sensors (Bi et al. Citation2021; Holder et al. Citation2020; Kelly et al. Citation2017; Wallace et al. Citation2021; Wallace et al. Citation2022a; Zheng et al. Citation2018), characterizing population exposures for epidemiological modeling (Gan et al. Citation2017; Heaney et al. Citation2021; Liu et al. Citation2015; O’Neill et al. Citation2021), the large-scale use of thousands of monitors in estimating either geographic variability (Bi et al. Citation2020) or exposure to indoor-generated particles (Liang et al. Citation2021; Wallace et al. Citation2022b), and in the development and/or evaluation of deterministic smoke generation and dispersion models for wildland fire events (Chow et al. Citation2022; Mallia et al. Citation2020; Ye et al. Citation2021) assuming these measurements are accurate based upon their EPA FEM designations.
Author contributions
Russell W. Long served as principal investigator and prepared the manuscript with contributions from all coauthors. Russell W. Long, Maribel Colón, Shawn P. Urbanski, Emily Lincoln, Jonathan Krug, Robert Vanderpool, and Matthew S. Landis performed the Chapel Hill and Missoula FSL chamber-based data collection and/or analysis. Surender Kaushik performed supervisory review of this research effort and corresponding manuscript.
Disclaimer
The views expressed in this paper are those of the authors and do not necessarily reflect the views or policies of EPA. It has been subjected to Agency review and approved for publication. Mention of trade names or commercial products do not constitute an endorsement or recommendation for use.
UAWM-2022-0127_Supplement_Rev-1_final.docx
Download MS Word (34.9 KB)Acknowledgment
The EPA through its Office of Research and Development (ORD) funded and conducted this research. We thank Greg Byrd (TRC), David Diaz-Sanchez (EPA), and Al Little (EPA) for supporting the Chapel Hill, NC chamber study; Andrew Habel (JTI, AECOM) and Ali Kamal (EPA) for supporting the Missoula FSL Chamber studies; James Szykman (EPA) and Cortina Johnson (EPA) for editorial support; Libby Nessley (EPA) for QA/QC support; and Kristen Foley (EPA) for statistical technical review.
Disclosure statement
No potential conflict of interest was reported by the author(s).
Supplementary data
Supplemental data for this article can be accessed online at https://doi.org/10.1080/10962247.2023.2171156.
Data availability statement
Datasets related to this manuscript can be found at https://catalog.data.gov/dataset/epa-sciencehub.
Additional information
Notes on contributors
Russell W. Long
Russell W. Long, Ph.D., corresponding author, is a senior research chemist with the U.S. EPA, Office of Research and Development, based in Research Triangle Park, NC.
Shawn P. Urbanski
Shawn P. Urbanski, Ph.D., is a research physical scientist with the USDA Forest Service, Rocky Mountain Research Station, based in Missoula, MT.
Emily Lincoln
Emily Lincoln is a physical scientist with the USDA Forest Service, Rocky Mountain Research Station, based in Missoula, MT.
Maribel Colón
Maribel Colón is a research chemist with the U.S. EPA, Office of Research and Development, based in Research Triangle Park, NC.
Surender Kaushik
Surender M. Kaushik, Ph.D. is a Supervisory Physical Scientist at the U.S. EPA, Office of Research and Development, based in Research Triangle Park, NC.
Jonathan D. Krug
Jonathan D.Krug is an engineer with the U.S. EPA, Office of Research and Development, based in Research Triangle Park, NC.
Robert W. Vanderpool
Robert W. Vanderpool is a senior aerosol physicist with the U.S. EPA, Office of Research and Development, based in Research Triangle Park, NC.
Matthew S. Landis
Matthew S. Landis is a senior research environmental health scientist with the U.S. EPA, Office of Research and Development, based in Research Triangle Park, NC.
References
- Aguilera, R., T. Corringham, A. Gershunov, and T. Benmarhnia. 2021. Wildfire smoke impacts respiratory health more than fine particles from other sources: Observational evidence from Southern California. Nat. Commun. 12 (1):1493. doi:10.1038/s41467-021-21708-0.
- AIRNow. Accessed September, 2022. https://www.airnow.gov.
- Allen, G., P. Babich, and R. Poirot. 2004. Evaluation of a new approach for real time assessment of Wood Smoke PM. Proceedings of Air & Waste Management Association Visibility Specialty Conference on Regional and Global Perspectives on Haze: Causes, Consequences and Controversies, Asheville, NC, October.
- Bi, J., L. Wallace, J. A. Sarnat, and Y. Liu. 2021. Characterizing outdoor infiltration and indoor contribution of PM2.5 with citizen-based low-cost monitoring data. Environ. Pollut. 276:116793. doi:10.1016/j.envpol.2021.116763.
- Bi, J., A. Wildani, H. H. Chang, and Y. Liu. 2020. Incorporating low-cost sensor measurements into high-resolution PM2.5 modeling at a large spatial scale. Environ. Sci. Technol. 54 (4):2152–62. doi:10.1021/acs.est.9b06046.
- Cappa, C. D., X. Zhang, L. M. Russell, S. Collier, A. K. Y. Lee, C. -L. Chen, R. Betha, S. Chen, J. Liu, D. J. Price, et al. 2019. Light absorption by ambient black and brown carbon and its dependence on black carbon coating state for two California, USA, cities in winter and summer. J. Geophys. Res.: Atmos. 124 (3):1550–77. doi:10.1029/2018JD029501.
- Cheng, Y.-H., Y.-C. Huang, A. S. Pipal, M.-Y. Jian, and Z.-S. Liu. 2022. Source apportionment of black carbon using light absorption measurement and impact of biomass burning smoke on air quality over rural central Taiwan: A yearlong study. Atmos. Pollut. Res. 13 (1):13. doi:10.1016/j.apr.2021.101264.
- Chow, F. K., K. A. Yu, A. Young, E. James, G. A. Grell, I. Csiszar, M. Tsidulko, S. Freitas, G. Pereira, L. Giglio, et al. 2022. High-resolution smoke forecasting for the 2018 camp fire in California. Bull. Am. Meteorol. 103 (6):E1531–52. Accessed September 13, 2022. https://journals.ametsoc.org/view/journals/bams/103/6/BAMS-D-20-0329.1.xml.
- Christian, T. J., B. Kleiss, R. J. Yokelson, R. Holzinger, P. J. Crutzen, W. M. Hao, B. H. Saharjo, and D. E. Ward. 2004. Comprehensive laboratory measurements of biomass-burning emissions: 2. First intercomparison of open-path FTIR, PTR-MS, and GC- MS/FID/ECD. J. Geophys. Res.; Atmos. 109 (D2). doi:10.1029/2003JD003874.
- Curtis, D. B., M. Aycibin, M. A. Young, V. H. Grassian, and P. D. Kleiber. 2007. Simultaneous measurement of light-scattering properties and particle size distribution for aerosols: Application to ammonium sulfate and quartz aerosol particles. Atmos. Environ. 41 (22):4748–58. doi:10.1016/j.atmosenv.2007.03.020.
- Drinovec, L., G. Moˇcnik, P. Zotter, A. S. H. Prévôt, C. Ruckstuhl, E. Coz, M. Rupakheti, J. Sciare, T. Müller, A. Wiedensohler, et al. 2015. The “dual-spot” Aethalometer: An improved measurement of aerosol black carbon with real-time loading compensation. Atmos. Meas. Tech. 8 (5):1965–79. doi:10.5194/amt-8-1965-2015.
- Foster, W. W. 1959. Attenuation of light by wood smoke. Br. J. Appl. Phys. 10 (9):416. doi:10.1088/0508-3443/10/9/309.
- Franchin, A., D. L. Fibiger, L. Goldberger, E. E. McDuffie, A. Moravek, C. C. Womack, E. T. Crosman, K. S. Docherty, W. P. Dube, S. W. Hoch, et al. 2018. Airborne and ground-based observations of ammonium-nitrate dominated aerosols in a shallow boundary layer during intense winter pollution episodes in northern Utah. Atmos. Chem. Phys. 18 (23):17259–76. doi:10.5194/acp-18-17259-2018.
- Gan, R. W., B. Ford, W. Lassman, G. Pfister, A. Vaidyanathan, E. Fisher, J. Volckens, J. R. Pierce, and S. Magzamen. 2017. Comparison of wildfire smoke estimation methods and associations with cardiopulmonary-related hospital admissions. GeoHealth 1 (3):122–36. doi:10.1002/2017GH000073.
- Garg, S., B. P. Chandra, V. Sinha, R. Sarda-Esteve, V. Gros, and B. Sinha. 2016. Limitation of the use of the absorption angstrom exponent for source apportionment of equivalent black carbon: A case study from the North West Indo-Gangetic Plain. Environ. Sci. Technol. 50 (2):814–24. doi:10.1021/acs.est.5b03868.
- Ghio, A. J., J. M. Soukup, M. Case, L. A. Dailey, J. Richards, J. Berntsen, R. B. Devlin, S. Stone, and A. Rappold. 2012. Exposure to wood smoke particles produces inflammation in healthy volunteers. Occup Environ Med 69 (3):170–75. doi:10.1136/oem.2011.065276.
- Grimm, H., and D. J. Eatough. 2009. Aerosol measurement: The use of optical light scattering for the determination of particulate size distribution, and particulate mass, including the semi-volatile fraction. J. Air Waste Manag. Assoc. 59 (1):101–07. doi:10.3155/1047-3289.59.1.101.
- Gupta, P., P. Doraiswamy, R. Levy, O. Pikelnaya, J. Maibach, B. Feenstra, A. Polidori, F. Kiros, and K. C. Mills. 2018. Impact of California fires on local and regional air quality: The role of a low-cost sensor network and satellite observations. GeoHealth 2 (6):172–81. doi:10.1029/2018GH000136.
- Guyot, A., J. Pudashine, R. Uijlenhoet, A. Protat, V. R. N. Pauwels, V. Louf, and J. P. Walker. 2021. Wildfire smoke particulate matter concentration measurements using radio links from cellular communication networks. AGU Adv. 2 (1). doi:10.1029/2020AV000258.
- Hagler, G., T. Hanley, B. Hassett-Sipple, R. Vanderpool, M. Smith, J. Wilbur, T. Wilbur, T. Oliver, D. Shand, V. Vidacek, et al. 2022. Evaluation of two collocated federal equivalent method PM2.5 instruments over a wide range of concentrations in Sarajevo, Bosnia and Herzegovina. Atmos. Pollut. Res. 13 (4):101374. doi:10.1016/j.apr.2022.101374.
- Healy, R. M., J. M. Wang, C. -H. Jeong, A. K. Y. Lee, M. D. Willis, E. Jaroudi, N. Zimmerman, N. Hilker, M. Murphy, S. Eckhardt, et al. 2015. Light-absorbing properties of ambient black carbon and brown carbon from fossil fuel and biomass burning sources. J. Geophys. Res.: Atmos. 120 (13):6619–33. doi:10.1002/2015JD023382.
- Heaney, A., J. D. Stowell, J. C. Liu, R. Basu, M. Marlier, and P. Kinney. 2021. Impacts of fine particulate matter for wildland fire smoke on respiratory and cardiovascular health in California. GeoHealth 6 (6). doi:10.1029/2021GH000578.
- Holder, A. L., A. K. Mebust, L. A. Maghran, M. R. McGown, K. E. Stewart, D. M. Vallano, R. A. Elleman, and K. R. Baker. 2020. Field evaluation of low-cost particulate matter sensors for measuring wildfire smoke. Sensors 20 (17):4796. doi:10.3390/s20174796.
- Hosseini, S., L. Qi, D. Cocker, D. Weise, A. Miller, M. Shrivastava, J. Miller, S. Mahalingam, M. Princevac, and H. Jung. 2010. Particle size distributions from laboratory-scale biomass fires using fast response instruments. Atmos. Chem. Phys. Discuss. 10:8065–76. doi:10.5194/acpd-10-8595.
- Kelleher, S., C. Quinn, D. Miller-Lionberg, and J. Volckens. 2018. A low-cost particulate matter (PM2.5) monitor for wildland fire smoke. Atmos. Meas. Tech. 11 (2):1087–97. doi:10.5194/amt-11-1087-2018.
- Kelly, K. E., J. Whitaker, A. Petty, C. Widmer, A. Dybwad, D. Sleeth, R. Martin, and A. Butterfield. 2017. Ambient and laboratory evaluation of a low-cost particulate matter sensor. Environ. Pollut. 221:491–500. doi:10.1016/j.envpol.2016.12.039.
- Kirchstetter, T. W., T. Novakov, and P. V. Hobbs. 2004. Evidence that the spectral dependence of light absorption by aerosols is affected by organic carbon. J. Geophys. Res. 109 (D21):D21208. doi:10.1029/2004JD004999.
- Krug, J., R. Long, M. Colón, A. Habel, S. Urbanski, and M. S. Landis. 2021. Evaluation of small form factor, filter-based PM2.5 samplers for temporary non-regulatory monitoring during wildland fire smoke events. Atmos. Environ. 265:118718. doi:10.1016/j.atmosenv.2021.118718.
- Lack, D. A., M. S. Richardson, D. Law, J. M. Langridge, C. D. Cappa, R. J. McLaughlin, and D. M. Murphy. 2012. Aircraft instrument for comprehensive characterization of aerosol optical properties, Part 2: Black and brown carbon absorption and absorption enhancement measured with photo acoustic spectroscopy. Aerosol. Sci. Technol. 46 (5):555–68. doi:10.1080/02786826.2011.645955.
- Laing, J. R., and D. A. Jaffe. 2019. Wildfires and PM concentrations in the Western United. EM, June.
- Landis, M. S., E. S. Edgerton, E. M. White, G. R. Wentworth, A. P. Sullivan, and A. M. Dillner. 2018. The impact of the 2016 Fort McMurray horse river wildfire on ambient air pollution levels in the Athabasca oil sands region, Alberta, Canada. Sci. Total Environ. 618:1665–76. doi:10.1016/j.scitotenv.2017.10.008.
- Landis, M. S., R. W. Long, J. Krug, M. Colon, R. Vanderpool, A. Habel, and S. Urbanski. 2021. The U.S. EPA wildland fire sensor challenge: Performance and evalution of solver submitted multi-pollutant sensor systems. Atmos. Environ. 247:1016. doi:10.1016/j.atmosenv.2020.118165.
- Laskin, A., J. Laskin, and S. A. Nizkorodov. 2015. Chemistry of atmospheric brown carbon. Chem. Rev. 115 (10):4335–82. doi:10.1021/cr5006167.
- Le, T. C., K. K. Shukla, Y. T. Chen, S. C. Chang, T. Y. Lin, Z. Li, D. Y. H. Pui, and C. J. Tsai. 2020. On the concentration differences between PM2.5 FEM monitors and FRM samplers. Atmosph. Environ. 222:117138. doi:10.1016/j.atmosenv.2019.117138.
- Liang, Y., D. Sengupta, M. J. Campmier, D. M. Lunderberg, J. S. Apte, and A. Goldstein. 2021. Wildfire smoke impacts on indoor air quality assessed using crowdsourced data in California. Proc. Natl. Acad. Sci. 118 (36), November 18. doi:10.1073/pnas.2106478118.
- Li, H., K. D. Lamb, J. P. Schwarz, V. Selimovic, R. J. Yokelson, G. R. McMeeking, and A. A. May. 2019. Inter-comparison of black carbon measurement methods for simulated open biomass burning emissions. Atmos. Environ. 206:156–69. doi:10.1016/j.atmosenv.2019.03.010.
- Liu, J. C., G. Pereira, S. A. Uhl, M. A. Bravo, and M. L. Bell. 2015. A systematic review of the physical health impacts from non-occupational exposure to wildfire smoke. Environ. Res. 136:120–32. doi:10.1016/j.envres.2014.10.015.
- Long, R. W., A. Whitehill, A. Habel, S. Urbanski, H. Halliday, M. Colón, S. Kaushik, and M. S. Landis. 2021. Comparison of ozone measurement methods in biomass burning smoke: An evaluation under field and laboratory conditions. Atmos. Meas. Tech. 14 (3):1783–800. doi:10.5194/amt-14-1783-2021.
- Lurmann, F., E. Avol, and F. Gilliland. 2015. Emissions reduction policies and recent trends in Southern California’s ambient air quality. J. Air Waste Manag. Assoc. 65 (3):324–35. doi:10.1080/10962247.2014.991856.
- Lyamani, H., F. J. Olmo, and L. Alados-Arboledas. 2008. Light scattering and absorption properties of aerosol particles in the urban environment of Granada, Spain. Atmos. Environ. 42 (11):2630–42. doi:10.1016/j.atmosenv.2007.10.070.
- Mallia, D. V., A. K. Kochanski, K. E. Kelly, R. Whitaker, W. Xing, L. E. Mitchell, A. Jacques, A. Farguell, J. Mandel, P. -E. Gaillardon, et al. 2020. Evaluating wildfire smoke transport within a coupled fire-atmosphere model using a high-density observation network for an episodic smoke event along Utah’s Wasatch Front. J. Geophys. Res.: Atmos. 125 (20). doi: 10.1029/2020JD032712.
- Martinsson, J., H. A. Azeem, M. K. Sporre, R. Bergström, E. Ahlberg, E. Öström, A. Kristensson, E. Swietlicki, and K. E. Stenström. 2017. Carbonaceous aerosol source apportionment using the Aethalometer model – Evaluation by radiocarbon and levoglucosan analysis at a rural background site in southern Sweden. Atmos. Chem. Phys. 17 (6):4265–81. doi:10.5194/acp-17-4265-2017.
- Martinsson, J., A. C. Eriksson, I. E. Nielsen, V. B. Malmborg, E. Ahlberg, C. Andersen, R. Lindgren, R. Nyström, E. Z. Nordin, W. H. Brune, et al. 2015. Impacts of Combustion conditions and photochemical processing on the light absorption of biomass combustion aerosol. Environ. Sci. Technol. 49 (24):14663–71. doi:10.1021/acs.est.5b03205.
- McClure, C. D., and D. A. Jaffe. 2018. US particulate matter air quality improves except in wildfire-prone areas. Proc. Natl. Acad. Sci. U.S.A. 115 (31):7901–06. doi:10.1073/pnas.1804353115.
- McMeeking, G. R., E. Fortner, T. B. Onasch, J. W. Taylor, M. Flynn, H. Coe, and S. M. Kreidenweis. 2014. Impacts of nonrefractory material on light absorption by aerosols emitted from biomass burning. J. Geophys. Res.: Atmos. 119 (12): 272– 12,286. doi:10.1002/2014JD021750.
- Mehadi, A., H. Moosmüller, D. E. Campbell, W. Ham, D. Schweizer, L. Tarnay, and J. Hunter. 2020. Laboratory and field evaluation of real-time and near real-time PM2.5 smoke monitors. J. Air Waste Manag. Assoc. 70 (2):158–79. doi:10.1080/10962247.2019.1654036.
- Met One Instruments, Inc. 2021. BAM-1022 particulate monitor operation manual. BAM 1020-9805 REV F.
- Met One Instruments, Inc. 2022. BAM-1020 particulate monitor operation manual. BAM 1022-9805 REV F.
- Noble, C. A., R. W. Vanderpool, T. M. Peters, F. F. McElroy, D. B. Gemmill, and R. W. Wiener. 2001. Federal reference and equivalent methods for measuring fine particulate matter. Aerosol Sci. Technol. 34 (5):457–64. doi:10.1080/02786820121582.
- O’neill, S. M., M. Diao, S. Raffuse, M. Al-Hamdan, M. Barik, Y. Jia, S. Reid, Y. Zou, D. Tong, J. J. West, et al. 2021. A multi-analysis approach for estimating regional health impacts from the 2017 Northern California wildfires. J. Air Waste Manag. Assoc. 71 (7):791–814. doi:10.1080/10962247.2021.1891994.
- Peñaloza-Murillo, M. 1998. An alternative method for obtaining the optical properties of monodisperse spherical non-absorbing aerosol using a cell-transmissometer. J. Aerosol Sci. 29:560–66. doi:10.1016/S0021-8502(98)00560-6.
- Peters, T. M., G. A. Norris, R. W. Vanderpool, D. B. Gemmill, R. W. Wiener, R. W. Murdoch, F. F. Mcelroy, and M. Pitchford. 2001. Field performance of PM2.5 federal reference method samplers. Aerosol Sci. & Technol 34 (5):433–43. doi:10.1080/02786820116873.
- Peters, T. M., D. Ott, and P. T. O’Shaughnessy. 2006. “Comparison of the GRIMM 1.108 and 1.109 portable aerosol spectrometer to the TSI 3321 Aerodynamic particle sizer for dry particles. Ann. Occup. Hyg. 50 (8):843–50. doi:10.1093/annhyg/mel067.
- Reid, J. S., R. Koppmann, T. F. Eck, and D. P. Eleuterio. 2005. A review of biomass burning emissions Part II: Intensive physical properties of biomass burning particles. Atmos. Chem. Phys. 5 (3):799–825. doi:10.5194/acp-5-799-2005.
- Saleh, R., C. J. Hennigan, G. R. McMeeking, W. K. Chuang, E. S. Robinson, H. Coe, N. M. Donahue, and A. L. Robinson. 2013. Absorptivity of brown carbon in fresh and photo-chemically aged biomass-burning emissions. Atmos. Chem. Phys. 13 (15):7683–93. doi:10.5194/acp-13-7683-2013.
- Tang, I. N. 1996. Chemical and size effects of hygroscopic aerosols on light scattering coefficients. J. Geophys. Res. 101 (D14):19245–50. doi:10.1029/96JD03003.
- Teledyne-API. 2019. The model T640 PM mass monitor specifications sheet. https://www.teledyne-api.com/prod/Downloads/SAL000090E%20-%20T640.pdf.
- U.S. Department of Agriculture. 2016. Forest health monitoring: National status, trends, and analysis 2015. Asheville, NC: Forest Service Research & Development, Southern Research Station, SRS-213.
- U.S. Department of Agriculture (USDA). 2014. United States forest resource facts and historical trends, FS-1035. Washington, DC. https://www.fia.fs.fed.us/library/brochures/docs/2012/ForestFacts_1952-2012_English.pdf.
- U.S. Department of Commerce National Oceanic and Atmospheric Administration (NOAA). 2017 Utah winter fine particulate study final report, March 2018. Accessed September, 2022. https://csl.noaa.gov/groups/csl7/measurements/2017uwfps/finalreport.pdf.
- U.S. Environmental Protection Agency (EPA). 1997. Reference method for the determination of fine particulate matter as PM2.5 in the atmosphere, 40 CFR Part 50 Appendix L.
- U.S. Environmental Protection Agency (EPA). 2013. National Ambient air quality standards for particulate matter, final rule. Federal Register Vol. 78, No. 10, January 15.
- U.S. Environmental Protection Agency (EPA). 2016. Teledyne advanced pollution instrumentation model T640 PM mass monitor. Automatic equivalent method: EQPM-0516-236. Federal Register: Vol. 81, page 45285, August 28.
- U.S. Environmental Protection Agency (EPA). 2019. Studies advance air monitoring during wildfires and improve forecasting of smoke. https://www.epa.gov/sciencematters/studies-advance-air-monitoring-during-wildfires-and-improve-forecasting-smoke, July 30.
- U.S. Environmental Protection Agency (EPA). Met One Instruments, Inc. 2015. BAM-1022 real time beta attenuation mass monitor-outdoor PM2.5 FEM configuration. Automated equivalent method: EQPM-1013-209. Federal Register: Vol. 80, page 51802, August 26.
- Urbanski, S. P. 2013. Combustion efficiency and emission factors for wildfire-season fires in mixed conifer forests of the northern Rocky Mountains, US. Atmos. Chem. Phys. 13 (14):7241–62. doi:10.5194/acp-13-7241-2013.
- Urbanski, S. P., S. M. O Neill, A. L. Holder, S. A. Green, and R. L. Graw. 2022. Emissions. In Wildland fire smoke in the United States: A scientific assessment, D. L. Peterson, S. M. McCaffrey, and T. Patel-Weynand. ed., 121–65. Cham, Switzerland: Springer Nature Switzerland AG. Chapter 5. doi:10.1007/978-3-030-87045-4_5.
- Urbanski, S. P., M. C. Reeves, R. E. Corley, R. P. Silverstein, and W. M. Hao. 2018. Contiguous United States wildland fire emission estimates during 2003–2015. Earth Syst. Sci. Data 10 (4):2241–74. doi:10.5194/essd-10-2241-2018.
- Volckens, J., and T. M. Peters. 2005. Counting and particle transmission efficiency of the aerodynamic particle sizer. J Aerosol Sci 36 (12):1400–08. doi:10.1016/j.jaerosci.2005.03.009.
- Wallace, L., J. Bi, W. R. Ott, J. A. Sarnat, and Y. Liu. 2021. Calibration of low-cost PurpleAir outdoor monitors using an improved method of calculating PM2.5. Atmos. Environ. 256:118432. doi:10.1016/j.atmosenv.2021.118432.
- Wallace, L. A., T. Zhao, and N. E. Klepeis. 2022a. Indoor contribution to PM2.5 exposure using all PurpleAir sites in Washington, Oregon, and California. Indoor Air 32 (9):13105. doi:10.1111/ina.13105.
- Wallace, L., T. Zhao, and N. E. Klepeis. 2022b. Calibration of PurpleAir PA-I and PA-II monitors using daily mean PM2.5 concentrations measured in California, Washington, and Oregon from 2017 to 2021. Sensors 22 (13):4741. doi:https://doi.org/10.3390/s22134741.
- Wang, Y., P. K. Hopke, O. V. Rattigan, X. Xia, D. C. Chalupa, and M. J. Utell. 2011a. Characterization of residential wood combustion particles using the two-wavelength Aethalometer. Environ. Sci. Technol. 45 (17):7387–93. dx. doi:10.1021/es2013984.
- Wang, Y., P. K. Hopke, O. V. Rattigan, and Y. Zhu. 2011b. Characterization of ambient black carbon and wood burning particles in two urban areas. J. Environ. Monit. 13 (7):1919–26. doi:10.1039/c1em10117j.
- Wang, Y., J. Huang, T. J. Zananski, P. K. Hopke, and T. M. Holsen. 2010. Impacts of the Canadian forest fires on atmospheric mercury and carbonaceous particles in northern New York. Environ. Sci. Technol. 44 (22):8435–40. doi:10.1021/es1024806.
- Weinert, D. W., T. G. Cleary, G. W. Mulholland, and P. F. Beever. 2003. Light scattering characteristics and size distribution of smoke and nuisance aerosols. In Fire Safety Science. Proceedings of the 7th International Symposium on Fire Safety Science, 209–20, Gaithersburg, Maryland, USA.
- Westerling, A. L. 2016. Increasing western U.S. forest wildfire activity: Sensitivity to changes in the timing of spring. Philos. Trans. R. Soc., B 371 (1696):20150178. doi:10.1098/rstb.2015.0178.
- Westerling, A., T. Brown, T. Schoennagel, T. Swetnam, M. Turner, and T. Veblen. 2014. Briefing: Climate and wildfire in western U.S. forests. In Forest conservation and management in the Anthropocene: Conference proceedings. Proceedings. RMRS-P-71, ed. V. A. Sample and R. P. Bixler, 81–102. Fort Collins, CO: US Department of Agriculture, Forest Service. Rocky Mountain Research Station.
- Whitehill, A., R. Long, A. Habel, and M. S. Landis. 2022. Evaluation of Cairpol and Aeroqual sensors in wildland fire plumes. Atmosphere 13 (6):877. doi:10.3390/atmos13060877.
- Xu, Q., A. L. Westerling, A. Notohamiprodjo, C. Wiedinmyer, J. J. Picotte, S. A. Parks, M. D. Hurteau, M. E. Marlier, C. A. Kolden, J. A. Sam, et al. 2022. Wildfire burn severity and emissions inventory: An example implementation over California. Environ. Res. Lett. 17 (8):085008. doi:10.1088/1748-9326/ac80d0.
- Ye, X. X., P. Arab, R. Ahmadov, E. James, G. A. Grell, B. Pierce, A. Kumar, P. Makar, J. Chen, D. Davignon, et al. 2021. Evaluation and intercomparison of wildfire smoke forecasts from multiple modeling systems for the 2019 Williams Flats fire. Atmos. Chem. Phys. 21 (18):14427–69. doi:10.5194/acp-21-14427-2021.
- Yue, X., L. J. Mickley, J. A. Logan, and J. O. Kaplan. 2013. Ensemble projections of wildfire activity and carbonaceous aerosol concentrations over the western United States in the mid-21st century. Atmos. Environ. 77:767–80. doi:10.1016/j.atmosenv.2013.06.003.
- Zheng, T., M. H. Bergin, K. K. Johnson, S. N. Tripathi, S. Shirodkar, M. S. Landis, R. Sutaria, and D. E. Carlson. 2018. Field evaluation of low-cost particulate matter sensors in high- and low-concentration environments. Atmos. Meas. Tech. 11 (8):4823–46. doi:10.5194/amt-11-4823-2018.