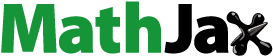
Abstract
The Perceived Macroeconomic Uncertainty (PMU) is seen as unpredictable volatility about the future economic development at aggregate level. While prior research explains how uncertainty (in general) influences international trade flows, research on the role of PMU in international trade flows is scarce. This article attempts to address this lack of understanding. Utilizing the gravity model and multicountry level data, our results show that: (1) the level of PMU in both importing countries and exporting countries has a significant negative impact on exports, but the effect of PMU of importing countries is larger than that of PMU of exporting countries; (2) PMU in importing countries has a trade diversion effect, suggesting that exporters are more willing to export to countries with relatively lower level of PMU; (3) the negative effects of PMU on trade have declined after the 2008 Great Financial Crisis, which may be related to the relative stability of the PMU index since the Great Financial Crisis and the increased concern of traders about other factors, such as trade policy uncertainty and Sino-US economic conflicts. Our research enriches prior findings that examine the effects of uncertainty on trade flows and carries important policy implications.
JEL CODE:
1. Introduction
The impact of uncertainty on trade has long been a topic of scholarly debate. It is widely accepted that uncertainty influences firms’ investment decision and economic activities (Bernanke, Citation1983), as well as their decision to export (Dixit, Citation1989; Grossman & Razin, Citation1985; Helpman & Razin, Citation1978). While there are many types of trade-related uncertainty, recent scholarly advances focus mainly on trade policy uncertainty (TPU), economic policy uncertainty (EPU) and demand uncertainty. For example, Handley and Limão (Citation2015) examine the role of TPU and finds that the decline in TPU prompted Portuguese enterprises to enter export markets. Similarly, Handley and Limão (Citation2017) demonstrates that the decline in TPU in Sino-US trade has increased the willingness of Chinese firms to enter the export market. Similar findings are also shown by Feng et al. (Citation2017) and Imbruno (Citation2019), among a few others.
As TPU is just one of the key components of policy uncertainty, scholars have also begun to pay attention to the overall impact of EPU on trade recently. For example, using quarterly data from 31 countries in 1998–2016, Tam (Citation2018), show that United States and Chinese EPU has a negative impact on global trade flows. Utilizing country-pair data, Jia et al. (Citation2020) find that a higher level of EPU in importing countries has an inhibitory effect on trade volume in exporting countries, but the impact of EPU in exporting countries is not significant.
With regards to the role of demand uncertainty, Novy and Taylor (Citation2020) argue that in response to uncertainty shocks, firms optimally adjust their inventory policy by cutting their orders of foreign intermediates disproportionately, which leads to a bigger contraction in international trade flows than in domestic economic activity. Using French firm-level data, De Sousa et al. (Citation2020) demonstrate that the industry-wide measures of expenditure uncertainty, such as the variance (or volatility) and the skewness of expenditure, in the destination countries not only affect firms’ entry/exit decisions but also export sales, and this is particularly true for the most productive firms.
Although most researchers agree that increased uncertainty leads to a decline in trade flows, a smaller number of studies also argue that uncertainty may stimulate trade activity. The classical ‘Qi-Hartman-Abel’ theory suggests that risk-averse firms will take more risk and increase investment in order to compensate for the loss caused by increased uncertainty (Abel, Citation1983; Hartman, Citation1972; Oi, Citation1961), which in turn may produce a trade-enhancing effect. In a general equilibrium trade model with information frictions, Baley et al.(Citation2020) shows that increases in uncertainty increase both the mean and variance in returns to exporting, and facilitates cross-country risk sharing, hence, trade can increase or decrease with uncertainty, depending on preferences. These conflicting views and findings suggest that further research is required to clarify the role of uncertainty.
Building on the insights from previous work on the subject, our article focuses on the impact of perceived macroeconomic uncertainty (PMU) on international trade. We adopt the perceived macroeconomic uncertainty index developed by Ozturk and Sheng (Citation2018) as the proxy for macroeconomic uncertainty. The index is constructed based on subjective forecasts of market participants and reflects perceived economic uncertainty. Compared with other measures on economic uncertainty such as volatility in stock market (Bloom, Citation2009), statistical forecasts (Jurado et al., Citation2015) and newspaper-based policy-related uncertainty (Baker et al., Citation2016), this index has the following advantages: First, the index utilizes surveys of professional forecasters directly rather than making objective statistical forecasts, which helps to avoid the omission of relevant information and leads to spurious estimates of uncertainty. Second, this index contains richer information than other alternative measures. As it captures the perceived uncertainty of market participants, the PMU index is not only related to the factors such as GDP and consumption that has direct effects on trade, but also considers other types of uncertainty related to other macroeconomic variables indirectly, such as investment, interest rates and unemployment rate. Third, the index has closer connection to trade. Besides the ‘sunk cost’ channel, expectation is also a key factor that affects the behavior of exporters (Handley, Citation2014; Handley & Limão, Citation2015; Nguyen, Citation2012). Therefore, the PMU index is a better proxy for macroeconomic uncertainty than other measures.
The contributions of this article are twofold. First, although there is a large body of studies which examine the role of uncertainty on trade, the study on the impact of the macroeconomic uncertainty on trade is rare. This is surprising as uncertainty about macroeconomic fundamentals (Jurado et al., Citation2015) is one of the most important kinds of uncertainty faced by importers or exporters. Theoretically, both the expectation of firms and the value of real option of ‘sunk cost’ in trade are highly correlated with the macroeconomic uncertainty. For example, the perceived uncertainty and unpredictable volatility about the future market development can be considered a key reason of trade collapse after the Great Financial Crisis (GFC) in 2008 and Covid-19 pandemic undoubtedly, even after considering the other factors, such as the downturn of real economic activity, the vulnerability of financial system and the malfunction of global production chain. Employing a multicountry dataset, this article considers whether the PMU of exporter and corresponding importer countries influence the trade flows between them. Our study, therefore, enriches prior research that has examined the effects of uncertainty on trade flows (Baley et al., Citation2020; De Sousa et al., Citation2020; Handley, Citation2014; Handley & Limão, Citation2015; Imbruno, Citation2019; Novy & Taylor, Citation2020).
Second, we not only focus on the overall effect of PMU on export building on the gravity models of previous studies (Anderson, Citation2011; Anderson & Van Wincoop, Citation2003), but also investigate whether there is a trade diversion effect and whether such effect changed before and after the 2008 Global Finance Crisis. In this way we advance the literature by taking into consideration the role of the third partner’s uncertainty and time-varying characteristic which has been largely ignored in the prior research on the effects of uncertainty on exports.
The rest of this article is structured as follows. Section 2 presents theoretical model and develops hypotheses. Section 3 describes data and econometric specifications. Section 4 shows empirical results, and the final section concludes.
2. Theoretical model and hypothesis
We follow Dixit and Pindyck (Citation1994) and establish a classic real option model in order to explore the sunk cost mechanism of PMU on trade and propose theoretical hypothesis. It is assumed that there is only one export enterprise in a country’s market. In order to export, the enterprise has to pay the irreversible entry cost I, and the export value of the enterprise is:
(1)
(1)
among them,
measures the market demand faced by enterprises after excluding market volatility and
represents the perceived (not the realized) fluctuation of domestic and foreign markets; that is, it measures the PMU faced by enterprises in export.
Referring to the classic real option model of Dixit and Pindyck (Citation1994), we assume that obeys the variation of the standard geometric Brownian motion, and set up the following equation:
(2)
(2)
where,
is the drift rate of market prospects;
represents the variance of market volatility, consisting of two parts: market fluctuation in importing country
and that of domestic country
> 0 measures the sensitivity of exporting firms to market volatility.
is the increment of the Wiener process, with mean and variance being 0 and
respectively. EquationEquation (2)
(2)
(2) indicates that the current status of the domestic and foreign markets is known, but the future situation is uncertain and fluctuates with time t.
As enterprises are more familiar with the domestic market and have stronger control over domestic economic uncertainty, the degree of uncertainty caused by fluctuation of domestic market of the same size is smaller than that of the foreign market; therefore, the variance of the market fluctuation is as shown in EquationEquation (3)(3)
(3) :
(3)
(3)
among them,
and
are the variances of the fluctuations of the importing country and the domestic market,
measures the control of the domestic market fluctuations, and
is the correlation coefficient between the target market and the domestic fluctuations. Based on the linkage of the world economy, this article assumes
The export choice of an enterprise in a macroeconomic uncertain environment can be considered holding a delayed option, implying that the firm will export at a certain time in the future. If the market at home and abroad is expected to function better in the future, the enterprise will execute the option and start exporting. However, if market is expected to be worse, the enterprise will give up the option – it will reduce export or give up export at this stage. Therefore, the value of the enterprises’ export choice can be regarded as a function of the market volatility U. The objective of the enterprise is to maximize the value of the export choice; that is:
(4)
(4)
where
is the expectation operator,
is the net export earnings of the enterprise during the t period, and r is the discount rate. We assume that
otherwise the larger the T, the larger the
the firm will delay the export indefinitely, and the EquationEquation (4)
(4)
(4) does not have an optimal solution.
According to the real option theory, in order to accurately measure the export choice of the enterprise, it is necessary to find out the critical condition of the enterprise for export. When the market condition becomes
the export value of the enterprise is not less than the value of the holding option. Then, the enterprise executes the option and begins to export. Accordingly, adopting the method of Dixit and Pindyck (Citation1994), we use contingent claims analysis to hedge the value of the option held by the enterprise with a certain asset portfolio. This will help to ensure that the company can obtain market risk-free interest rates.
This article constructs a combination of one unit delay option long and
units export asset (V) shorts. At time t + dt, the combination holder can obtain the added value of the combination on the one hand, including the added value of the delayed option (
) and that of the export asset (
), namely,
on the other hand, the enterprise holds
each unit export asset shorts must also pay a certain amount of interest to the bulls, i.e.,
where
is the opportunity cost of the company holding the delayed option. Therefore, at time
the total return for holding the combination is
According to Ito’s lemma, we have:
(5)
(5)
Substituting EquationEquations (1)(1)
(1) and Equation(2)
(2)
(2) , the total revenue of the constructed portfolio is:
(6)
(6)
Assuming that the portfolio return is a risk-free return, the function must satisfy the following conditions in order to solve the delay option mechanism F and the critical value of the optimal export return V*.
Initial condition: optimal condition:
In order to meet the initial conditions, should take the following form:
(7)
(7)
where
and
are the roots of the equations
and
to be solved. The critical value of market conditions and export value of enterprises are obtained as follows:
(8)
(8)
According to EquationEquation (8)(8)
(8) ,
is a decreasing function of
and the derivatives of
with respect to
and
can be obtained.
(9)
(9)
(10)
(10)
Based on the EquationEquations (8)–(10), when the other variables are constant, the increase of causes the
value to decrease and the
to increase, which leads to delayed execution of option or reduced exports.
In addition, importers will also be affected by the uncertainty of demand (De Sousa et al., Citation2020) and worry about the market prospects besides exporters, thus, reducing the volume of purchases (Nguyen, Citation2012). Therefore, we get the following hypothesis.
Hypothesis 1: The increase in PMU will lead enterprises to abandon or reduce exports, resulting in a decline in a country’s exports.
According to the EquationEquations (9)(9)
(9) and Equation(10)
(10)
(10) , where,
we know that
thereby obtaining the hypothesis 2 herein.
Hypothesis 2: PMU in the importing country has a greater impact on a country’s exports than PMU of exporting country.
3. Variables and data
3.1. PMU index
The PMU indicator selected in this article is from Ozturk and Sheng (Citation2018), which is measured based on eight fundamental-related indicators, namely GDP, consumption, investment, industrial output, inflation, long- and short-term interest rates, and unemployment rate. Ozturk and Sheng (Citation2018) breaks down the PMU into Common Uncertainty, measuring perceived variability of future aggregate shocks of professional analysts, and Idiosyncratic Uncertainty, representing the disagreement among professional forecasters, and then weighted the two to get the Total Uncertainty.
(11)
(11)
where,
states the volatility of a typical forecaster,
is the weight of individual forecast error in consensus forecast error. The first component of EquationEquation (11)
(11)
(11) is the common uncertainty perceived by all forecasters, and the second component is the forecast disagreement and captures idiosyncratic uncertainty.
As both the common uncertainty and idiosyncratic uncertainty have impact on trade and the two kinds of uncertainty in some economies are highly correlated, it is more suitable to use the Total Uncertainty as the proxy for PMU index of an economy.
3.2. Econometric model
The gravity model has a strong explanatory power for factors affecting trade flows between two countries, and has achieved great success in many applications. Based on standard gravity equation, referring to existing literature, the empirical model is set as follows:
(12)
(12)
where,
represent control variables affecting the trade between the two countries. Referring to Anderson and Van Wincoop (Citation2003), we introduce multilateral-resistance factors (MRT) to control the endogeneity, and set the following baseline econometric model:
(13)
(13)
where
denotes real export from country
to country j,
and
are perceived macroeconomic uncertainty index of import and export country, while
and
are the real GDP of country
and j, respectively.Footnote1
stands for real exchange rate, while CPI represents consumer price index.
is the geographic distance between country
and j,
and
are dummy variables and are equal to 1 if a pair of exporting-importing countries have a common border, free trade agreement, same language and same currency, respectively. In order to alleviate the endogeneity issue, the PMU indexes are lagged.
captures multilateral-resistance factors. Following Head and Mayer (Citation2014) and Heid and Larch (Citation2016), we introduce fixed effects of import country and export country, as well as time fixed effects as approximate substitutes of MRT, and retains distance, language and other items that do not change with time.
3.3. Data
This article selects the 44 countries (or regions) provided by the PMU data source as the sample (see the Appendix for the list of countries or regions), which spans from the first quarter of 2002 to the third quarter of 2017. The countries and regions in our sample are important players in global trade, and are also heterogeneous in terms of the size of the economy and the level of economic development. The data of nominal export denominating in US dollars are sourced from the IMF DOTS database, and are deflated by CPI index of United States (Caballero et al., Citation2018; Santos Silva & Tenreyro, Citation2006). As the trade value between some economies is zero, the logarithm is meaningless; therefore, the sample with zero trade value is eliminated.Footnote2 PMU data is from Sheng’s personal website.Footnote3 As PMU indexes are monthly data and we convert them into quarterly data by aggregate average. GDP is the real GDP denominated in US dollars. We obtained the data on GDP measured in national currency from IMF IFS and converted it into US dollars with the currency’s real exchange rate against US dollars. The nominal exchange rate data are derived from the Pacific Exchange Rate Service website.Footnote4 We calculated the real exchange rate based on the national CPI level and the nominal exchange rate, with the CPI data from the IMF IFS. Data on distance, border, language and currency wereobtained from the CEPII, while FTA data were provided bypersonalwebsite of Jose De Sousa.Footnote5
shows the statistical descriptions of the variables. As can be seen, the range of PMU among countries ranges from 0.02 to 1.43, indicating a higher level of heterogeneity among countries with different levels of economic development.
Table 1. Descriptive statistics of variables.
4. Empirical results
4.1. Basic results
displays the empirical results obtained by estimating a panel data regression. In so doing, we controlled for the fixed effects of both importing and exporting countries, as well as time fixed effects. Column (4) shows the benchmark specification with PMU from both import and export countries as well as all control variables, while the other columns (Columns 1–3) display alternative specifications. The results show that importing countries’ PMU significantly impedes export and a 1% increase in importing country’s PMU will drive down exports by around 0.1141%. By contrast, a 1% increase in exporting country’s PMU will drive down exports by around 0.0687%. These results indicate that the PMU of both importing and exporting countries indeed are important factors influencing the decision of exporters to enter the market of a country.
Table 2. Basic regression results.
We find that the coefficient of PMU in the exporting country is also negative and significant, indicating that a PMU rise in exporting countries would also lead to a decline in trade. This may be due to the fact that the rising PMU of exporting countries will not only lead to the deterioration of exporters’ expectations for the future economy, increase the financing difficulties and financing costs of export enterprises, but also affect the product supply capacity and supply chain stability of exporters, thus, reducing export willingness and export ability. Indeed, Ozturk and Sheng (Citation2018) show that the period of PMU rise generally coincides with a period of economic recession or financial crisis in an economy. During the period of economic recession or financial crisis, the market sentiment is low, export investment behavior is cautious, and the real option value of sunk cost is increased. This is particularly true for the period of financial crisis. During such a period, the phenomenon of bank and enterprise bankruptcy occurs more frequently, and the ability of enterprises to obtain financing support decreases, which also disturbs the normal production chain. However, compared with the importing country, the PMU of the exporting country has a relatively weaker effect, which is consistent with Hypothesis 2. We offer three tentative explanations for these results. First, in terms of the sunk cost channels, as exporter’s investment in sunk costs is used in the importing country’s market, not in the exporting country’s market, only macroeconomic changes in the importing country will affect the exporter’s adjustment to the sunk cost. Second, in terms of the expectation channels, exporters have more accurate and stable expectations of macroeconomic changes in their own countries and are, therefore, less sensitive to the changes in macroeconomic uncertainties. Finally, as international trade plays an important role in the development of national economy, countries all over the world often attach great importance to trade growth. Once export is affected by domestic PMU, countries will adopt export promotion strategy to offset the impact.
The results regarding control variables are well in line with the predictions in standard trade models. Among them, the coefficients of GDP of both exporting country and importing country are positive and significant, indicating that higher GDP of trading partners will contribute to the trade growth between them. Moreover, the coefficient of GDP of the importing country is larger than that of the exporting country. This result suggests that the greater the market demand of the importing country, the more trade it will create. The coefficient of the real exchange rate is negative and significant. This is a ‘standard’ result which is well expected and is in line with the theory of balance of payments elasticity analysis. The coefficient of geographical distance between two countries is negative and significant. This result indicates that the farther the two countries are, the smaller the trade scale between them. Among the dummy variables, the coefficients of the common boundary, FTA agreement, common language and common currency are all positively significant at 1% confidence level, which are consistent with the results of the gravity model.
4.2. Further discussion
4.2.1. Whether the trade diversion effects exist?
Theoretically, country ’s export to country
may be affected by the level of PMU in country
or PMU in other countries outside country
When country
’s PMU level is higher than that of other countries, exporters tend to reduce their exports to country
and turn to export to competing countries, resulting in a trade-diversion effect, amplifying the inhibition degree of trade by importing country’s PMU. Following Nguyen et al. (Citation2018), we regress the specification below:
(14)
(14)
is the indicator measuring the relative PMU of country
against other country’s PMU. To alleviate endogeneity, we use GDP of the importing country as the weight, and define
as the PMU indicator of all trading countries except country
The calculation formula is:
(15)
(15)
shows the regression results of trade diversion effects of relative PMU in importing countries. Column (1) is the regression result of only adding the trade diversion effect index and column (2) is the regression result after adding control variables, the value of adjusted R2 improves from 0.6604 to 0.8496. As is shown in , the coefficient of relative PMU of country is significantly negative, indicating that there is a significant trade diversion effect to third country. This conclusion is consistent with findings of Nguyen et al. (Citation2018) on FDI.
Table 3. Trade diversion effects of PMU of importing country.
4.2.2. Whether the impact of PMU changed before and after the Great Financial Crisis?
The outbreak of Great Financial Crisis in 2008 has had a huge impact on global trade, which declined by more than 10 percent during the financial crisis, leading to a ‘trade collapse’. Even after the financial crisis, the global economy has gradually recovered; the global trade has still fallen into a state of low growth. After 2012, the average growth rate of global trade has been lower than that of global GDP, which is in sharp contrast to the pre-crisis period (IMF, 2016). Meanwhile, the overall slow recovery of the global economy and the rise of trade protectionism are all likely to have an impact on trade behavior through sunk cost channels and expectation channels, thus, changing the impact of PMU.
In order to empirically judge whether the impact of PMU before and after the financial crisis has changed, this article introduces a dummy variable of the 2008 GFC. We use Lehman Brothers’ bankruptcy filing in September 2008 as a sign of the financial crisis. It is 0 before September of the year and 1 afterwards.
The regression equation is set to:
(16)
(16)
At this time, the influence coefficients of PMU of exporting countries and importing countries on trade after the financial crisis are and
respectively. If
and
are not significant, it means that there is no change in the effect of PMU before and after the GFC. If
and
are both greater than zero and significant, it means that after GFC in 2008, the effect of PMU has decreased, otherwise, it means that after GFC in 2008, the effect of PMU has increased.
shows the regression results of EquationEquation (16)(16)
(16) . Whether it only includes the product term of the exporting country PMU and
or the product term of the importing country (Columns 1 and 2) and
or both (Column 3), the results all show that after the outbreak of the financial crisis in 2008, the negative impact of PMU on trade has declined.
Table 4. Impact of PMU on export before and after the Great Financial Crisis.
This article argues that this may have two explanations: (1) as PMU mainly measures the perception of analysts and market participants on the overall downside risk of the future macro economy, this index reaches its peaks during most of the recessionary episodes and remains low during expansions. However, after the 2008 GFC, although some economies (such as Italy and Greece) have experienced a certain degree of recession due to the impact of the European debt crisis, the world and most countries are recovering, causing the PMU index to remain at a relatively low level, thus, weakening the impact of PMU through sunk costs and demand expectations channels. (2) After the GFC, the growth rate of global trade has dropped significantly, ‘anti-globalization’ and trade protection measures prevailed, which made traders more pessimistic about trade expectations. Even if the PMU of importing and exporting countries stabilized at relatively low levels, it was difficult to boost trade confidence. Conversely, traders paid more attention to more direct factors affecting trade, such as trade policy uncertainty and exchange rate fluctuations.
4.3. Robustness test
First, some scholars believe that trade policy uncertainty (Handley & Limão, Citation2015, Citation2017) and newspaper-based policy-related uncertainty (Jia et al., Citation2020; Tam, Citation2018) will also generate some effect on trade. If the impact of these uncertainties is not controlled, the estimated results of may be biased. Because the relevant literature of TPU mainly uses specific event in individual countries for research, it is not suitable for the sample characteristics of this article. Therefore, we mainly consider the impact of EPU. Specifically, the EPU variables of importing country and exporting country are included in EquationEquation (12)(12)
(12) , i.e., the regression equation is set as:
(17)
(17)
and
is economic policy uncertainty index of country
and
the index is developed by Baker et al. (Citation2016) and the data comes from the policy uncertainty website.Footnote6 We select the countries which are overlapped by two indexes (EPU and PMU), and finally get 20 economies,Footnote7 and the sample also ranges from 2002Q1 to 2017Q3. Ozturk and Sheng (Citation2018) believe that the correlation between EPU and PMU is very low. For the United States, the correlation between the two is only 0.18 and can be used as two independent variables.
shows the empirical results after adding EPU variables. The EPU coefficient of importing countries is significantly negative, but the EPU coefficient of exporting countries is not significant. This result is similar to Jia et al. (Citation2020). Compared with , although the PMU coefficients of importing and exporting countries have declined to a certain extent after adding the EPU variable, their impact on trade is still significantly negative, and the PMU of importing countries has a greater impact. The results are the same as in .
Table 5. Impact of PMU on export after adding the EPU variable.
Second, Santos Silva and Tenreyro (Citation2006) pointed out that according to Jensen’s inequality (E(ln y) ≠ ln E(y)), when using log-linear gravity model for empirical analysis of trade, even if the export country, import country and time effects are controlled, heteroscedasticity will lead to biased estimation. In addition, the traditional log-linear gravity model cannot deal with zero trade volume. Therefore, many studies suggest using the Poisson Pseudo-Maximum Likelihood Estimation (PPML) method to overcome these problems. We adopt PPML as a robustness test for the impact of PMU on trade and the result did not change in any significant way. In fact, in recent years, more and more papers have used PPML to estimate the gravity model (Anderson et al., Citation2016; Fally, Citation2015).
The specific setting of the PPML is:
(18)
(18)
The regression results are shown in . Column (1) is the basic result of PPML estimation, which is basically consistent with the results in . Besides, the estimated results in Column (3) and Columns (4)–(6) are also similar with and . This means that after considering the Heteroscedasticity and the estimation bias problem that Jensen’s inequality may bring, the previous empirical conclusions have not changed, indicating that the empirical results in this article are robust.
Table 6. Robustness test using PPML method.
We also used another way to treat MRT as a robustness test. The academia generally uses two ways to deal with MRT. One is to introduce importing country, exporting country and time fixed effects as approximate substitutions of multilateral trade resistance factors, but retaining distance, language and other variables that do not change with time, as what we have done previously. The other way is to introduce a set of dummy variables, including importing country, exporting country, time and pairing fixed effects, according to Anderson and Van Wincoop (Citation2003), Baltagi et al. (Citation2003), but this method can hinder the estimation of variables such as real exchange rate. Therefore, a more compromised solution is to add the pairing fixed effect, but not to keep the distance, language and other variables that do not change with time.
We followed Baltagi et al. (Citation2003) to remove the variables that do not change with time, and add a pairing fixed effect to regress the equation; the results are basically consistent with the methods of Heid and Larch (Citation2016), as is shown in Model (2) of . It can be seen that the coefficients of variables are in line with expectations, showing that our results are robust.
5. Conclusions
This article develops the premise that PMU will affect trade behavior through sunk cost, trade financing and expectation channels. Our results suggest that the levels of PMU in both importing and exporting countries have significant impacts on export. Furthermore, the export is not only affected by the PMU of importing countries, but also by that of the third countries, indicating that there is a trade diversion effect of PMU on exports. When the PMU of the importing country is higher than that of other countries, the exporting country tends to reduce its exports to the importing country and turn to exporting to third countries.
The results suggest that reducing the level of PMU is an important way to achieve stable growth of world trade and overcome existing difficulties after 2008 GFC, especially after the outbreak of COVID-19 pandemic. The results carry several policy implications. First, ‘anti-globalization’ sentiment and policies should be resolutely resisted and opposed. Anti-globalization initiatives from countries such as the United States will drive up global macroeconomic uncertainty. This in turn will not only undermine domestic output growth, but also have detrimental effect on global trade recovery. Governments and business society across the world should be highly alert to the harm that ‘anti-globalization’ policies and movement may create, and stabilize and reduce the level of PMU on a global scale. Second, it is necessary to strengthen international macroeconomic policy coordination and jointly deal with the threat of COVID-19 pandemic. Since the outbreak of COVID-19, the global economy has fallen into a deep recession with global and national macroeconomic uncertainties rising sharply, causing a slump in global trade. Countries should abandon the mutual accusations and political frictions surrounding the pandemic and control jointly the spread of the pandemic through the coordination of international monetary, fiscal and capital flow policies. This will help to stabilize market expectations and reduce macroeconomic uncertainty, thus promoting the recovery and development of international trade.
The study has several limitations. First, although our model suggests that PMU will affect trade through sunk cost channels and expectation channels, data constraints do not allow us to examine empirically the existence of the two channels and quantify their effects. On the one hand, it is difficult to measure sunk cost and, on the other, it is difficult to obtain data on expectations.
Second, PMU is the perceived uncertainty reflected in the surveys of professional forecasters and is constructed as a comprehensive index. The index covers eight variables but gives equal weight for each. However, trade theory tells us that some variables such as the growth rates of GDP, consumption and investment, may have much more impact on trade flows than the levels of inflation, short- and long-term interest rates. This means that the estimated results would be different if different weights are allowed for each of the eight variables. Hence, care should be taken when interpreting the results of this study.
Disclosure statement
No potential conflict of interest was reported by the authors.
Additional information
Funding
Notes
1 Ozturk and Sheng (Citation2018) find that PMU shocks have large and persistent impacts on industrial production and unemployment rate, but this impact has obvious time lag, indicating that and
have limited influence on control variables in gravity model, such as
and
which will not cause serious endogenous problems between variables.
2 As the number of zero trade flows in the sample is very small, to delete the zero trade flows will have little impact for the empirical results.
7 The economies includes Australia, Brazil, Canada, Chile, China, France, Germany, Hong Kong, India, Italy, Japan, Mexico, Netherlands, Russia, Singapore, South Korea, Spain, Sweden, UK and United States.
References
- Abel, A. B. (1983). Optimal investment under uncertainty. American Economic Review, 73(1), 228–233.
- Anderson, J. E. (2011). The gravity model. Annual Review of Economics, 3(1), 133–160. https://doi.org/10.1146/annurev-economics-111809-125114
- Anderson, J. E., & Van Wincoop, E. (2003). Gravity with gravitas: A solution to the border puzzle. American Economic Review, 93(1), 170–192. https://doi.org/10.1257/000282803321455214
- Anderson, J. E., Vesselovsky, M., & Yotov, Y. V. (2016). Gravity with scale effects. Journal of International Economics, 100, 174–193. https://doi.org/10.1016/j.jinteco.2016.03.003
- Baker, S. R., Bloom, N., & Davis, S. J. (2016). Measuring economic policy uncertainty. The Quarterly Journal of Economics, 131(4), 1593–1636. https://doi.org/10.1093/qje/qjw024
- Baley, I., Veldkamp, L., & Waugh, M. (2020). Can global uncertainty promote international trade? Journal of International Economics, 126, 103347. https://doi.org/10.1016/j.jinteco.2020.103347
- Baltagi, B. H., Egger, P., & Pfaffermayr, M. (2003). A generalized design for bilateral trade flow models. Economics Letters, 80(3), 391–397. https://doi.org/10.1016/S0165-1765(03)00115-0
- Bernanke, B. S. (1983). Irreversibility, uncertainty, and cyclical investment. The Quarterly Journal of Economics, 98(1), 85–106. https://doi.org/10.2307/1885568
- Bloom, N. (2009). The impact of uncertainty shocks. Econometrica, 77(3), 623–685. https://doi.org/10.3982/ECTA6248
- Caballero, J., Hale, G., & Candelaria, C. (2018). Bank linkages and international trade. Journal of International Economics, 115, 30–47. https://doi.org/10.1016/j.jinteco.2018.08.006
- De Sousa, J., Disdier, A. C., & Gaigné, C. (2020). Export decision under risk. European Economic Review, 121, 103342. https://doi.org/10.1016/j.euroecorev.2019.103342
- Dixit, A. (1989). Entry and exit decisions under uncertainty. Journal of Political Economy, 97(3), 620–638. https://doi.org/10.1086/261619
- Dixit, A. K., & Pindyck, R. S. (1994). Investment under uncertainty. Princeton University Press.
- Fally, T. (2015). Structural gravity and fixed effects. Journal of International Economics, 97(1), 76–85. https://doi.org/10.1016/j.jinteco.2015.05.005
- Feng, L., Li, Z., & Swenson, D. L. (2017). Trade policy uncertainty and exports: Evidence from China’s WTO accession. Journal of International Economics, 106, 20–36. https://doi.org/10.1016/j.jinteco.2016.12.009
- Grossman, G., & Razin, A. (1985). The pattern of trade in a Ricardian model with country-specific uncertainty. International Economic Review, 26(1), 193–202. https://doi.org/10.2307/2526534
- Handley, K. (2014). Exporting under trade policy uncertainty: Theory and evidence. Journal of International Economics, 94(1), 50–66. https://doi.org/10.1016/j.jinteco.2014.05.005
- Handley, K., & Limão, N. (2015). Trade and investment under policy uncertainty: Theory and firm evidence. American Economic Journal: Economic Policy, 7(4), 189–222. https://doi.org/10.1257/pol.20140068[Mismatch]
- Handley, K., & Limão, N. (2017). Policy uncertainty, trade, and welfare: Theory and evidence for china and the United States. American Economic Review, 107(9), 2731–2783.https://doi.org/10.1257/aer.20141419
- Hartman, R. (1972). The effects of price and cost uncertainty on investment. Journal of Economic Theory, 5(2), 258–266. https://doi.org/10.1016/0022-0531(72)90105-6
- Head, K., & Mayer, T. (2014). Gravity equations: Workhorse, toolkit, and cookbook. Handbook of International Economics, 4, 131–195. https://doi.org/10.1016/B978-0-444-54314-1.00003-3
- Heid, B., & Larch, M. (2016). Gravity with unemployment. Journal of International Economics, 101, 70–85. https://doi.org/10.1016/j.jinteco.2016.03.008
- Helpman, E., & Razin, A. (1978). Uncertainty and International Trade in the Presence of Stock Markets. The Review of Economic Studies, 45(2), 239–250. https://doi.org/10.2307/2297338 http://www.jstor.org/stable/2297338
- Imbruno, M. (2019). Importing under trade policy uncertainty: Evidence from China. Journal of Comparative Economics, 47(4), 806–826. https://doi.org/10.1016/j.jce.2019.06.004
- IMF. (2016 October) Subdued demand: Symptoms and remedies. World Economic Outlook.
- Jia, F., Huang, X. Y., Xu, X. Y., & Sun, H. Y. (2020). The effects of economic policy uncertainty on export: A gravity model approach. Prague Economic Papers, 29(5), 600–622. https://doi.org/10.18267/j.pep.754
- Jurado, K., Ludvigson, S. C., & Ng, S. (2015). Measuring uncertainty. American Economic Review, 105(3), 1177–1216. https://doi.org/10.1257/aer.20131193
- Nguyen, D. X. (2012). Demand uncertainty: Exporting delays and exporting failures. Journal of International Economics, 86(2), 336–344. https://doi.org/10.1016/j.jinteco.2011.10.007
- Nguyen, Q., Kim, T., & Papanastassiou, M. (2018). Policy uncertainty, derivatives use, and firm-level FDI. Journal of International Business Studies, 49(1), 96–126. https://doi.org/10.1057/s41267-017-0090-z
- Novy, D., & Taylor, A. M. (2020). Trade and uncertainty. The Review of Economics and Statistics, 102(4), 749–765. https://doi.org/10.1162/rest_a_00885
- Oi, W. (1961). The desirability of price instability under perfect competition. Econometrica, 29(1), 58–64. https://doi.org/10.2307/1907687
- Ozturk, E. O., & Sheng, X. S. (2018). Measuring global and country-specific uncertainty. Journal of International Money and Finance, 88, 276–295. https://doi.org/10.1016/j.jimonfin.2017.07.014
- Santos Silva, J. M., & Tenreyro, S. (2006). The log of gravity. Review of Economics and Statistics, 88(4), 641–658. https://doi.org/10.1162/rest.88.4.641
- Tam, P. S. (2018). Global trade flows and economic policy uncertainty. Applied Economics, 50(34–35), 3718–3734. https://doi.org/10.1080/00036846.2018.1436151
Appendix
Table A1. The list of countries/regions in this article.