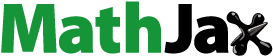
ABSTRACT
In the era of Education 4.0, the development of smart schools represents a significant step forward. Learning spaces are critical to the success of smart school construction in primary and secondary schools, due to their significant impact on school-age children. This study focuses on identifying key spatial factors that influence students’ psychological preferences and learning utility in smart learning spaces. To achieve this, a visual stated preference method was employed to design a questionnaire, which was used to survey primary and secondary school students in Harbin, China. The resulting data was analyzed using a discrete choice model, which enabled the measurement of the utility levels of different learning space factors. The results show that students’ learning space preferences are significantly influenced by a variety of spatial factors, with the combination mode of classrooms and outdoors having the highest level of influence, followed by a completely open interface, U-shape seating layout, zoned color tone, and the combination mode of classrooms and corridors; the students surveyed show a significant negative preference for heteromorphic spaces compared to rectangular spaces. The discussion recommends the design of smart learning spaces around key spatial factors to enhance the utility of student learning.
1. Introduction
The concept of smart school comes from the “Smart School Project” issued by the Ministry of Education of Malaysia in 1997, which refers to the use of technology to systematically update and design teaching methods and school management (Ong and Ruthven Citation2010). Smart learning environments merge technology and instruction, with spaces serving as the medium for this system (Gros Citation2016). Smart learning spaces not only support formal and informal learning scenarios, but also provide spaces where both physical and virtual technologies coexist. Compared with traditional learning environment, the teaching in smart learning environment has a significant positive effect on student learning outcomes (Shi et al. Citation2020). Many countries have already started to implement related government projects, such as the Future School Project in Singapore (UNESCO Citation2010), the Future Classroom Project in the European Union (UNESCO Citation2014), and the Digital School Project in Poland (Creative Commons Polska Citation2012). China has a nine-year compulsory education system. Under this special education system, China put forward the development plan of education informatization driven by education intelligence in 2012, and proposed the goal of building intelligent campuses in 2019. However, the construction of smart learning environments is still in its infancy in China, with the completion rate of smart classroom in public schools in China reached 10% in 2019. In terms of geographical distribution, the coastal regions of China, such as Southeast (26%), South China (21%) and East China (17%), are at the forefront of smart classroom construction. The Chinese primary and secondary schools follow a uniform standard for the construction of teaching and learning spaces, so guidance on the construction of space design for smart schools is important and currently lacking.
To achieve a smart transformation of education, it is important to have flexible learning environments that provide support. Studies have demonstrated that smart learning environments based on digital technology can enhance and enrich the learning experience by catering to flexibility, interactivity, engagement, and experiential learning aspect (Cheung et al. Citation2021). The mental and physical state of school-age children is more influenced by their environment, and the environmental aspects of basic education are crucial. Learning space needs reform. A large number of studies show that several factors of the learning space will affect students’ learning efficiency, such as class size, furniture layout, green space, etc (Park, Chung, and Rhee Citation2011; Perks, Orr, and Al-Omari Citation2016). However, as far as the influencing factors are concerned, the design of learning environment in the information technology era is more complicated (M K Nambiar et al. Citation2017). The impact of spatial factors of smart learning spaces on learning effectiveness has not been studied.
In transportation and other fields, Stated Preference (SP) has been utilized as a quantitative research tool to assess preference selection for perceived environmental behavior (Levinson et al. Citation2006; Yang Citation2018). However, previous learning space surveys have employed Revealed Preference (RP) and textual scale narratives with limited visual representation, thereby making it difficult to generate realistic data. This study aims to extract the spatial factors of learning spaces from the architectural perspective and connect the visual SP method with the design of learning space. Subsequently, a model of learning space utility evaluation is developed to gauge the positive or negative influence of learning spaces on students’ learning preferences in China. The central aim of this study is to investigate the connection between the psychological preferences of students and the learning space environment in smart learning spaces. Based on the findings of the evaluation model, the research aims to propose design strategies for smart learning spaces that optimize the learning experience. Three research questions are posed:
Which factors of the learning space will have an impact on students’ preference to learn?
To what extent do the spatial factors of the learning space have an impact on students’ learning preferences? Do socio-demographic characteristics, such as gender, grade influence student learning preferences?
What are the characteristics of learning spaces that generate high learning utility? How to design principles of smart learning spaces?
1.1. Practice and development of smart school
Based on the smart school project released in Malaysia in 1997, the smart school project in Iran was launched in 2004, trying to integrate ICT technology into the field of education, but it was questioned by some academics (Attaran, Alias, and Siraj Citation2012). Various initiatives have been launched globally to promote smart education. For example, the New York Smart Schools project was proposed in 2014 to improve teaching and learning through technology (Smart Schools NY Citation2014). The Devotra Smart Classrooms, launched in the Netherlands in 2015, aims to extend smart educational technologies to various countries in Africa (Devotra Smart Classroom, Citation2015). The Samsung Smart Schools programme was a collaborative effort between the Spanish Ministry of Education and Samsung aimed at improving teaching models and enhancing learning with the help of technology (Suárez-Guerrero, Lloret-Catalá, and Mengual-Andrés Citation2016). South Korea also launched its Smart Schools programme in 2018, which focuses on comprehensive reform of existing school spaces, curriculum design, educational development support systems, and educational technology (Cho et al. Citation2020).
In the information age, it is a trend that new technologies are widely used in education (Hassan and Geys Citation2016). Ghonoodi et al. suggested the need to popularize “smart schools” and improve the traditional “teaching-learning” relationship through ICT technology to increase the efficiency of teaching and learning (Ghonoodi and Salimi Citation2011). Omidinia has explained the determinants of the success of smart schools in Malaysia in terms of curriculum, teaching methods, assessment systems, teaching materials, and school environment, and has begun to focus on the impact of environmental space on smart school construction (Omidinia, Masrom, and Selamat Citation2012). In the same year, Kang Wai-Chin also came to the realization that creating smart learning environments – which promote learners’ variety and individualized development – is a crucial component of constructing smart schools (Kang Citation2016). Many new technologies and equipment, such as ICT equipment, the Internet of Things, virtual reality, etc., can be used to assist in transforming traditional classrooms into interactive smart classrooms (Wang et al. Citation2022). Based on the current state of school infrastructure in Australia, Newton et al. argue that permanent prefabricated construction of smart green schools will create new learning environments that promote student development through the integration of pedagogy, architectural space, and fabrication technology (Newton et al. Citation2018).
The importance of learning spaces in smart schools has significantly increased (Terzieva, Ilchev, and Todorova Citation2022). However, research on smart schools in China has mainly focused on pedagogical theory, and there is insufficient research focusing on learning space design for smart schools. In addition, the approach to transforming traditional learning spaces into smart learning spaces is mainly reflected in the updating of the Internet of Things, while ignoring the changes of the spaces caused by new teaching concepts and new equipment.
1.2. The influence of smart learning space on learning behavior
In 2008, Professor Radcliffe of the University of Queensland proposed a “Pedagogy-Space-Technology” design framework for the design and evaluation of learning spaces (Radcliffe Citation2008). The concept of smart education is constantly evolving, with the integration of new technologies, teaching methods, and learning spaces. The ultimate goal of smart education is to develop smart learning spaces that promote smart pedagogy, provide personalized learning services, and foster competent individuals (Zhu, Yu, and Riezebos Citation2016). Within the concept of open education, learning can occur anywhere on campus, and these areas are referred to as learning spaces (Gislason Citation2015). Smart learning spaces are personalized with technology to meet the needs of individual learners and provide support such as guidance, feedback, tips, or tools (Hwang Citation2014). A large number of studies show that flexible spaces can support and promote the use of student-centered pedagogy and increase student autonomy and well-being (Byers, Imms, and Hartnell-Young Citation2018; Kariippanon et al. Citation2018; Tapia-Fonllem et al. Citation2020). Kariippanon and other scholars studied the transformation of traditional classrooms into flexible learning spaces in eight Australian schools. They found that adapting classroom furniture and layout can create a positive relationship between teaching methods and the learning environment (Kariippanon et al. Citation2020).
In recent years, the relationships between the spatial factors of learning spaces on students’ preferences and learning behaviors has been widely concerned in the field of education and environmental studies. Barrett found that classroom environmental variables accounted for 16% of a student’s academic progress every year, and that spatial design had a significant impact on learning (Barrett et al. Citation2015). Six experts, including Davies, analyzed 210 studies on creative learning environments in schools and found that learning environments have features that is physical environmental such as visual environments, availability of resources such as ICT and use of outdoor environments, which can effectively promote the development of teenagers’ creative skills (Davies et al. Citation2013). In an IT-driven study, Sitthiworachart analyzed 119 teachers’ surveys of instructional networks and found a variety of technology-enabled learning spaces that also effectively promoted active student’ participation (Joy et al. Citation2014; Sitthiworachart et al. Citation2022). Jin and Peng collected 316 reports on student perceptions of traditional and active learning classrooms, and discussed strategies for future classroom design and optimization in four aspects: learning support, perception of space and furniture, physical environment, and instructional interaction (Jin and Peng Citation2022).
In the context of smart education, Peng et al. divided the components of learning spaces into four dimensions: physical environment, spatial layout, furniture design, and technological equipment, and explored the relationship between spatial perception factors and learning inputs (Peng, Deng, and Jin Citation2022). Many scholars explored the impact of physical and psychological teaching space environment perception on learning behavior from multiple factors such as area per student, space flexibility, seat comfort, indoor color, ICT equipment, etc (Jin and Peng Citation2022; Yaseen and Mustafa Citation2022). Despite these valuable findings, most research on the comfort factors of the physical environment without taking into account the broader context, including the shape of the space, the openness of the spatial interface, the form of the spatial composition, and other architectural spatial representations. In addition, most studies focus only on students’ psychological perceptions and ignore the impact of changes in smart education and smart schools.
1.3. Advantages and applications of the SP method
The SP method was applied to travel behavior research in the 1970s and it has been used more often to study choice behavior preferences since the 1990s (Ahern and Tapley Citation2008; Kelly et al. Citation2011; Verhetsel et al. Citation2017). The SP method is a survey method that expresses respondents’ subjective preferences for alternatives by experimentally designing multiple virtual situations for respondents to choose from with good controllability and predictability (Levinson et al. Citation2006). Ma and Zhang (Citation2016) utilized the SP method to effectively predict the leisure preferences of an automobile camp prior to its construction. Atkinson and Whitehead (Citation2015) verified the accuracy of user behaviour prediction using the SP method.
Compared to the traditional SP method, the visual SP method uses a more intuitive and understandable form of expression that is close to realistic decision-making scenarios and is sufficient to convey professionally oriented information language to non-specialists (Cheu et al. Citation2011). Kasraian used a 3D virtual reality environment to simulate a live experience, compensating for the perceptual limitations of textual descriptions (Kasraian et al. Citation2021). The use of visual aids in SP surveys, particularly for preferences that are difficult to communicate verbally, has been shown to enhance the dissemination of SP method (Perdomo et al. Citation2014).
In particular, when the object of description is architectural space, pictorial questionnaires are superior to textual descriptions, considering the variability in the understanding of spatial layout attributes by the non-professional population (Baker and Oppewal Citation2022; Bellizzi, Forciniti, and Mazzulla Citation2021). The SP method is a widely used method in the transportation field and has also been extensively researched in the context of users’ housing choices. Verhetsel utilized the SP method to investigate the characteristics of students’ housing preferences, considering variables such as house size and spatial functions (Verhetsel et al. Citation2017). The SP method is a valuable tool for analyzing users’ choice preferences for various building spaces. However, the use of the SP method for preference studies of architectural space choices of special populations, such as primary and secondary school students, is not sufficient, especially with visual graphical language.
2. Method
2.1. Study area
The site of this experiment is located in Harbin, the capital city of Heilongjiang Province, China. According to the 2021 Harbin Education Development Statistics Bulletin, there are 850 general primary and secondary schools in the city and 785,200 students enrolled in the city (). The large number and wide distribution of primary and secondary schools within Harbin, and the launch of the pilot construction of smart schools on 10 July 2020, provided the sample base for this study. The learning space in this study refers mainly to the classroom.
Table 1. Statistics of primary and secondary schools in Harbin city.
2.2. The overall methodological framework
The SP method includes three steps: factor level design, choice scheme generation, and questionnaire expression. Based on this, the technical route of this study consists of four parts: first, in the context of smart school, according to literature reviewing and pre-survey, the key factors of learning space about learning preferences are extracted, and the levels of each factor are set; second, Second, a virtual model of the learning space was constructed to generate a picture representation of the SP questionnaire, which was used to collect data on the learning preferences of the students interviewed; third, a Discrete Choice Model is established to analyze the utility of the learning space preference measurement data; finally, based on the analysis results, the design strategy of the smart school learning space is proposed, as shown in .
2.3. Factor level design
Based on the literature review in section 1.2, 7 key spatial factors of learning space and two socio-demographic factors were identified. At the same time, based on the relevant regulation standards, literature research, and survey, 2–3 value levels were set for each factor ().
Table 2. Learning space factors and level settings of smart primary and secondary schools.
2.3.1. Area per student
Average student space refers to the area per student in a classroom. Smart schools promote personalised learning, and the class sizes can significantly impact the effectiveness of the smart pedagogy. Students are most satisfied with classroom layouts with a large amount of usable space per student (Smith Citation2017). However, overly spacious classrooms also have disadvantages, such as difficulties hearing other students’ voices (Zhu Citation2021). Among them, the factor level of the area is mainly based on the “Design Code for Primary and Secondary Schools GB50099–2011”, the average usable area per student for ordinary classrooms should not be less than 1.36 m2/person in primary school, 1.39 m2/person in secondary schools. In “Beijing Standard for Primary and Secondary School Running Conditions” the area per students in ordinary classrooms is 1.7 m2/person. While in Taiwan, the teaching mode emphasizes the freedom of learning, according to “National Benchmark for Primary and Secondary School Equipment” is set at the class size of 35 students sets the average useable area per student at 2.5 m2/person − 3.2 m2/person. The UK’s BB103 announcement mentions the minimum per student area for primary and secondary schools, 2 m2/person for primary schools, 2.9 m2/person for secondary schools, and 3.2 m2/person for high schools (“GOV.UK,” Citation2018). Based on this, the area level is set at three levels: 1.36 m2/person-1.7 m2/person, 1.7 m2/person-2.5 m2/person, and 2.5 m2/person-3.2 m2/person.
2.3.2. Shape
Shape refers to the plane shape of the classroom, including rectangle and heteromorphic. The appropriate shapes of spaces can enhance the support for technical equipment arrangement and the use of smart pedagogy. Some studies have shown that students in higher grades prefer larger, simpler shapes, and students in lower grades prefer heteromorphic shapes (Jin and Peng Citation2022). The heterogeneous planes are mostly hexagonal. They have better audio-visual effects because they are designed based on the average height of students, sight distance, and the scale of teaching tools.
2.3.3. Interface
Interface refers to the surrounding enclosure structure of the classroom, including walls, windows, etc. Smart schools’ emphasis on openness can be attained by regulating building interfaces, where varying degrees of openness facilitate multi-modal, cross-space learning environment. Out of preference for natural lighting and the natural outdoor environment, most students favored large open windows facing green spaces, and the preference was more pronounced among boys than girls (Barrera-Hernández et al. Citation2020). For the interface between the corridor and the classroom, it has been suggested that expanding the window size at this interface to provide a more open view for students and teachers would have a positive psychological and physiological impact on students (Yaseen and Mustafa Citation2022). Different degrees of openness in the learning space have different effects on the willingness to learn, and the interface of the learning space is divided into completely open, semi-open, and enclosed according to the degree of openness.
2.3.4. Combination mode
The Combination mode combines formal and informal learning spaces, divided into three modes: classroom space, classroom and corridor, and classroom and outdoor. Smart schools prioritize contextual learning, and the combination of various spaces can aid in reshaping the learning environment. In the case study of the transformation of outdoor spaces in primary schools, Dillon found that working in the outdoor environment of a school promotes students’ creative development; this may be because indoors, work tends to be individual-centered. Whereas outdoors, learning activities are more likely to involve collaboration (Dillon Citation2007).
2.3.5. Seating layout
Different seating layouts are adapted to different teaching modes. This study divides classroom seating layout into three types, group style, U-shape, and determinant. The arrangement of classroom furniture plays a crucial role in supporting smart pedagogy (MacLeod et al. Citation2018). When we need to limit students’ communicative behavior and improve their ability to pay attention and think independently in the classroom, determinant is the most preferable choice. On the contrary, when brainstorming and group work is carried out, group seating arrangements can promote interaction among students as the distance between them is drawn in (Wannarka and Ruhl Citation2008). Also, the U-shape layout increases the probability of students asking questions and the desire to communicate compared to the determinant (Marx, Fuhrer, and Hartig Citation2000).
2.3.6. Color tone
Color tone refers to the color of the walls and floors around the classroom. Environmental perception factors are also important in smart classrooms, and the use of sensory devices can adjust the classroom’s color tone to meet various contextual requirements. Jin and Peng point out that more natural tones should be chosen for the classroom walls rather than pure white or gray (Jin and Peng Citation2022). Nature’s colors and cooler tones, such as green and blue can be calming. The classroom color environment can use brighter color as the main color, and add other attractive colors (Jebril and Chen Citation2021). Thus, the color tone of a learning space can be divided into monotone and zoning tone.
2.3.7. ICT equipment richness
The ICT equipment richness refers to the variety and quantity of ICT equipment in the classroom. Mobile interactive whiteboards and multi-angle displays provide ample space for students to communicate and interact, effectively leading to student engagement (Benoit Citation2018; Rezaei Citation2020). However, at the same time, some unique equipment may lead to distraction and inattention of students (Zimmermann et al. Citation2018). Projection and the Internet are the most common equipment, followed by laptops, video players, whiteboards, recording systems, etc (Sitthiworachart et al. Citation2022). In the context of smart education, electronic equipment such as projection equipment, smart screens, tablets, recording systems, media players, video conferencing equipment, and other electronic equipment support student learning in a space where the right amount of technology helps develop creative skills. In addition, the integration of technological devices facilitates a transition from teacher-centred to student-centred classroom teaching, which is a crucial aspect of the smart learning space (Looi et al. Citation2010).
2.3.8. Socio-demographic factors
Socio-demographic variables included gender, age, grade. In China, mandatory education is separated into two stages: primary school, which lasts around six years, and secondary school, which lasts around three years. Grade was used as a measure of age. Depending on the duration of the school period and the age profile of the students, the age range is 6–11 years for primary school students and 12–14 years for secondary school students.
2.4. Participants and survey design
The study used the visual SP method to design the questionnaire. Combining the seven factors and their levels resulted in 972 scenarios. To reduce the number of combinations, the study used SPSS software to conduct an orthogonal test design, which is uniformly dispersed and neatly comparable. Combined with pseudo-horizontal method, 18 groups of selected scenes were finally obtained. The virtual 3D scenes were constructed by SketchUp 2020 software according to the combination of factors. All factors comply with Chinese standards, “Design Code for Primary and Secondary Schools GB50099–2011”. Considering the limited comprehension ability of primary and secondary school students, the scenes were constructed to be simple and intuitive, and uniform components were used for the same factor level to control the influence of variables. Among them, the model components of ICT equipment factors that are not easy to observe are expressed as icons to reduce the respondents’ comprehension errors ().
Table 3. Virtual learning space selection scenario model.
The questionnaire contains two parts: one is the respondents’ personal attribute factors, including gender, age, and grade level; the second is the SP survey question, 18 groups of virtual learning space choices, where respondents compare two virtual scenarios and choose the one with their learning intention preference, or neither if they are not satisfied with both scenarios. The questionnaire survey was conducted at primary and secondary schools in Nangang District, Harbin, which is renowned for its advanced educational resources and pioneering pedagogical practices. While users of learning spaces include school staff, such as teachers, we have focused on the psychological preferences of students, as they are more attached to the learning space and directly benefit learning utility.
Prior to the main investigation, a preliminary survey was conducted to evaluate the students’ comprehension and receptiveness towards the questionnaire content and virtual scenarios. Subsequently, the questionnaire content was refined based on the feedback received from the students, which was followed by the formal questionnaire research. This study was carried out at two primary schools and two secondary schools in May 2022, with a total of 220 questionnaires, a sample size reference to previous research experience (Kelemen, Brown, and Pizza Citation2023; Peng, Deng, and Jin Citation2022). Prior to data collection, parents informed written consents were acquired, following the ethical guidelines laid down by the Institutional Review Board. The survey covered a total of 3,600 scene selections. After removing incomplete and invalid responses, 200 valid questionnaires were obtained with a validity rate of 90.9%. Of these 200, 94 were females and 106 males; 92 were primary school students and 108 secondary school students; their mean age was 10 years (6–14 years), standard deviation was 2 years.
2.5. Discrete choice model construction
Discrete Choice Models are often used to perform statistical analyses of the results of choice preference experiments, such as the SP method (Haggar et al. Citation2022; Lu et al. Citation2021; Monchambert Citation2020). Logit models have the advantage of greater robustness and are widely used (Belaïd and Massié Citation2022; Dubin and McFadden Citation1984; van den Berg et al. Citation2020). In a VR experiment simulating a fire scene, Lovreglio used a logit model to quantify the factors influencing participants’ choice of different exits (Lovreglio et al. Citation2022). Iraganaboina applied a logit model to illustrate the effect of various independent variables on energy use for different households and housing unit sizes (Iraganaboina and Eluru Citation2021).
Based on the data from learning preference survey, this study uses the Logit model in the Discrete Choice Model to calculate the weight relationship and utility function among the factors. The general principle of the Discrete Choice Model is random utility theory, which assumes that primary and secondary school students choose the space with the greatest utility for them. The learning utility of the learning space is defined as follows.
Where: is the total utility obtained by primary and secondary school students in learning space scene,
is the utility coefficient of spatial factor variables, and the mean and standard deviation of the parameters can be found under the assumption of normal distribution. Area per student and ICT equipment richness are continuous variables. Shape, interface, combination mode, seat layout, and color tone are qualitative variables, so dummy variables are treated.
3. Results
3.1. Descriptive statistics
A total of 220 questionnaires were distributed, and 200 valid questionnaires were finally recovered for analysis by excluding incomplete, duplicate, or invalid responses (). In the sample, the gender ratio of male to female was 1.13:1, and the number of primary and secondary school students was 1:0.85; The overall structure of the sample was balanced and met the requirements of the model analysis. describes the number of times the 18 classroom models were selected, and it can be seen that model 17 was selected the most, up to 189 times, and is characterized by a heteromorphic, zoning tone environment, U-shape seating layout, a combined classroom + outdoor mode, 3–4 kinds of ICT equipment, a completely open interface and the smallest area per student. While Model 18, which uses a combination mode of classrooms, enclosed interface and a group seating layout, 5–6 kinds of ICT equipment, the medium area per student and the rest of the factors are the same as Model 17, was selected the least number of times, 13 times. The descriptive statistics of the model variables and the mean and standard deviation of the data are shown in .
Table 4. Sample characteristic statistics. (n = 200).
Table 5. Statistics of the number of classroom models selected. (n = 3600).
Table 6. Descriptive statistics of classroom preference variables. (n = 200).
3.2. Spatial attribute factors
The model was fitted to the selected records using STATA MP16 (). The coefficients of the variables are the utility weights, whose absolute magnitude reflects the degree of influence of each spatial attribute factors on utility. The negative coefficient for a continuous variable indicates that the utility becomes worse as the value of that variable increases. In contrast, for the dummy variable coefficients, a negative coefficient has only a relative significance, indicating that it has the opposite effect to the reference level. The McFadden pseudo R2 is found to be 0.1202, which indicates a good model fit. provides the modeling results. Overall, six out of twelve variables are significant at the 5% level and one at the 1% level. This implies these seven variables significantly influence students’ learning preferences. The area per student, the ICT equipment richness, and the semi-open interface of the learning space were found to be not associated with learning preferences. In other words, the changes of the area per student and the number of ICT equipment, and whether it is a semi-open interface have a negligible effect on learning utility However, the change in per student area and the introduction of ICT equipment is one of the important shifts in the smart learning environment. This result is different than expected and may be related to the fact that in conducting the visual SP questionnaire, students were more concerned with spatial attribute factors that have an intuitive visual perception.
Table 7. Results of estimation of logit. (n = 200).
The classroom-outdoor combination mode is the most important to primary and secondary school students learning, followed by the classroom-corridor combination. Informal learning spaces such as outdoor -corridor combination mode are authentic and free, motivating students to explore and learn. This makes them more attractive to students than traditional classrooms. Among the spatial interface factors, completely open interface was significantly associated with students’ learning preferences. Because a more open space supports a greater variety of learning behaviors. U-shape seating layouts had higher learning utility and group layouts had the lowest, reflecting primary and secondary school students’ need for presentation spaces. This is not as expected, probably because most Chinese primary and secondary schools are still teacher-delivered. In a lecture format, the small group layout may obscure the view of some students. The zoning color tone is relatively important. As students are more sensitive to color, spaces with an overall soft tone but distinct areas are more appealing to students. The heteromorphic shape of the learning space has a significant negative impact on students’ willingness to learn. Students were more reluctant to learn in heteromorphic spaces than in rectangular learning spaces, which is consistent with the actual investigation. The existing classrooms have a large proportion of rectangular classrooms, and students are not very receptive to heteromorphic classrooms. The comparisons between the 7 key spatial factors, 19 factor levels and their resulting learning utility are further discussed. As shows, the combination mode, interface, and shape have stronger correlation with the learning utility of the learning space.
3.3. Socio-demographic factors
Various socio-demographic characteristics are found to significantly affect students’ learning space decisions. Some studies have shown that males and females have different preferences for IT-based learning environments (Yeo, Taylor, and Kulski Citation2006). The preference for learning spaces varies to varying degrees by gender (). First, the semi-completely open interface of the learning space has a negative effect on learning space preferences for males, but a positive effect for females. Second, the ranked seating layout has a significant effect on female choice preference at the 5% level, but it is non-significant for males. This indicates that males are not sensitive to the determinant seating layout.
Table 8. Adjusted associations between gender and learning space preferences.
To further explore the differences in preferences for learning spaces arising from students’ individual characteristics, two age groups, primary and secondary, were conducted (). Primary school students’ preferences for learning spaces were negatively impacted by the semi-open interface of learning spaces (-1.64), whereas they were positively impacted for secondary school students (1.26). Primary school students preferred the enclosed interface compared to the semi-open interface. However, as the interface became more open, the preference of secondary school students increased. The spatial combination of classroom and corridor had a significant effect on both primary and secondary school students, but the utility coefficient was higher for primary school students (0.594) than for secondary school students (0.322). It is obvious that primary students benefit more from learning when a classroom and corridor are combined than secondary students. The difference in the effect of other factors between secondary students and primary students was not significant.
Table 9. Adjusted associations between sociodemographic characteristics and learning space preferences.
4. Discussion
Learning utility measurement results can provide a basis for learning space design that is different from traditional design models. The key factors of learning spaces are interconnected and work together to form the unique system structure of learning spaces. Therefore, to explore the layout and level selection of learning space factors can be started from the five factors that have a significant impact on the utility results.
4.1. Students like the combination of outdoor and completely open learning spaces the most
According to the findings of this study, the combination of classroom and outdoor had the highest weighting at 1.147, followed by the completely open interface at 0.853. Since students spend most of their time indoors, the need for a visual or physical connection between the teaching space and the outdoor green space is more pressing. This is in line with previous research findings that students prefer spaces that are connected to nature, and that they prefer learning spaces that are brightly colored, airy, and naturally connected to the outside world (Barrett et al. Citation2015; van den Bogerd et al. Citation2020; Woodward and Zari Citation2018). By combining classroom and outdoor spaces, students can benefit from the advantages of both environments. Outdoor learning spaces promote creativity, imagination, and exploration, while indoor learning spaces provide structure, organization, and focus. The hands-on experiences students gain in outdoor learning make an important contribution to their emotional, cognitive, social, and physical development (Aminpour Citation2022; Shimokura, Yanagiawa, and Sasaki Citation2023).
Additionally, the combination of classroom and outdoor spaces can provide a variety of learning opportunities, allowing students to engage in different types of activities and experience different types of environments. In order to incorporate outdoor learning spaces into classroom design, it is important to consider factors such as access to natural light, ventilation, and the ability to control temperature and noise levels. In the renovation and design of learning spaces, the classroom interface can be opened with the help of movable partitions or whiteboards. In this way, the learning function of classrooms can be extended to informal spaces such as outdoors and corridors, forming a multifaceted combination of classroom and outdoors, and classroom and corridor. Smart learning spaces are characterized by innovative pedagogies, including learning by doing, collaborative learning, project-based learning, advanced technology-based learning, and game-based learning (Mogas Recalde et al. Citation2020). Open learning spaces that are interconnected with corridors and outdoors promote autonomous, diverse, and effective learning experiences.
4.2. Heteromorphic spatial shape is the factor with the lowest utility in the learning space
The results of the weight of the factors obtained in this study showed that the negative impact weight of the heteromorphic learning space was the highest at −1.114. Based on these findings, it is recommended that future designs of learning spaces should prioritize the use of rectangular shapes. This is because rectangular spaces have better sound quality and lighting effects, which can contribute to a more conducive learning environment. Additionally, rectangular spaces have a high accommodation rate, providing more flexibility in the placement of tables and chairs, and can also result in greater economic efficiency. Rectangular spaces are more suitable for configuring various types of technical facilities and equipment. Furthermore, rectangular spaces have almost no visual dead space, which can help to prevent visual discomfort that may arise in heteromorphic spaces. Overall, these recommendations can aid in designing learning spaces that promote student well-being and academic performance.
4.3. The U-shape seating layout is significantly more important than the group style and determinant
The U-shape had the highest utility weight in the seating layout at 0.527. The U-shape layout was found to be more flexible in terms of activity routes and line-of-sight changes compared to the determinant layout, thereby promoting communication among students. This finding is consistent with intergroup contact theory and the contact hypothesis (Pettigrew Citation1998), which suggest that closer proximity between students fosters positive interpersonal interactions, leading to a reduction in negative peer perceptions and an increase in positive feelings among peers. Peer interactions are known to be a powerful source of individual behavior, and peer interaction in the classroom can promote positive student behavior (Gest and Rodkin Citation2011). Moreover, the U-shape layout was found to be more convenient than the group style for adjusting excessive communication among students, thus preventing the classroom from becoming chaotic and promoting better student learning outcomes (Jin and Peng Citation2022). Flexible seating layout in classrooms have multiple smart classroom purposes, such as lectures and synchronized group learning activities. Incorporating flexible furniture design configurations, such as seating, can promote communication among students in the classroom, leading to the achievement of smart education goals. In future interior space design, flexible and convertible seating layouts should be adopted to support students’ freedom of movement and choice and to meet future individual and diverse learning patterns.
4.4. Zoning color tone is more helpful for learning behavior than monotone
In the color tone, zoning tones were weighted higher than monotone at 0.46. These findings are consistent with Barrett et al.’s (Citation2015) recommendation that a single color need not be used for all four walls in learning spaces. Rather, painting one wall in a bright color and the remaining walls in a soft color can be an effective approach. Furthermore, Liu et al.‘s (Citation2022) research revealed that cooler wall environments such as blue and green were associated with higher ratings of relaxation and enjoyment among students, whereas warmer wall environments such as yellow and red were linked to better concentration and academic performance. For smart classroom design, it is ideal that the color tone is adaptive, meaning that different tone should be able to adjust to various learning atmosphere needs. Therefore, a dynamic tone adjustment system has been proposed (Sleegers et al. Citation2013). In conjunction with the questionnaire results of this study, the usage of soft colors in the main body of the learning space can foster a sense of coherence and create a friendly and comfortable learning environment. However, it is crucial to note that excessive colors can lead to visual clutter. Therefore, limiting the main colors to three can aid in better student focus.
5. Conclusion
The study aims to enhance our understanding of the learning utility of learning spaces, with a particular focus on students’ psychological preferences for such spaces. The study finds that several spatial factors had a significant impact on students’ overall preference for learning spaces within the smart school framework, including area per student, shape, interface, combination mode, seating layout, color tone, and ICT equipment richness. The results, obtained through the use of a visual SP questionnaire and subsequent Discrete Choice Model analysis, also reveal socio-demographic factors, gender and grade level preference differences in spatial interface and seating layout factors. The findings of this study provide valuable insights into the design of learning spaces, which can be used to develop focused and forward-looking recommendations for improving learning spaces in smart primary and secondary schools.
Specifically, the study highlights the importance of considering the critical spatial factors identified in this research when designing smart learning spaces to enhance students’ learning utility and willingness. The guidelines proposed in this study can serve as a basis for the construction of personalized, contextualized, and smart learning spaces that meet the unique needs of students in primary and secondary schools. These guidelines emphasize the importance of integrating outdoor environments, providing open and rectangular spaces, using colorful partitions, and offering flexible seating layouts to enhance students’ active and ubiquitous learning. To analyze the design of a smart learning space, it is important to consider three key levels of improvement in smart education: smart pedagogy, smart technology, and smart space. This study focuses on optimizing the physical environment factors of the learning space in order to facilitate positive changes in these three dimensions. These findings can serve as a valuable basis for smart school construction, addressing the spatial facilitation of learning and promoting students’ learning satisfaction and utility, especially in the era of information-based education reform.
There are a few limitations that warrant further research. First, the learning spaces constructed in the questionnaire and the generated images visualize the spatial form of the learning spaces. However, there is still no way to provide respondents with an immersive spatial experience. Admittedly, if sufficient equipment is available, it is more attractive to use VR and other devices for environmental simulation experience. Although the findings are based on four middle and elementary school cases in Harbin, the spatial characteristics illustrated in this study were implemented based on the characteristics of the teaching mode and learning space. It is thought that the same can be applied to smart schools in other parts of China and even in other countries, although some have to be reorganized. In China primary and secondary education is compulsory, with uniform standards in building design and teaching management. Although some differences in educational goals and teaching methods between primary and secondary education were excluded from the analysis, they play an important role in complementing the differences in functional needs of learning spaces, which are distinguished in this study only in terms of the differences in psychological preferences between primary and secondary students. When designing special schools in the future, it will be necessary to comprehensively examine factors related to education, culture, and society that were not dealt with in this study.
Disclosure statement
No potential conflict of interest was reported by the authors.
Additional information
Funding
Notes on contributors
Yu Zhang
Yu Zhang Ph.D., is an Associate Professor, doctoral supervisor of the School of Architecture, and the vice dean of HIT-Shenzhen International School of Design,Harbin Institute of Technology, China. Her research focuses on child-friendly environment design, the smart school design and habitat intelligence.
Jiameng Cui
Jiameng Cui is a Phd student at Harbin Institute of Technology (School of Architecture). Her research focuses on the smart school design and healthy environment design.
Hongming Liu
Hongming Liu is present master at Harbin Institute of Technology (School of Architecture).
Ping Yu
Ping Yu is present master at Harbin Institute of Technology (School of Architecture).
Yu Ban
Yu Ban is a Phd student at Harbin Institute of Technology (School of Architecture).
Xiaotian Cheng
Xiaotian Cheng is a Phd student at Harbin Institute of Technology (School of Architecture).
References
- Ahern, A. A., and N. Tapley. 2008. “The Use of Stated Preference Techniques to Model Modal Choices on Interurban Trips in Ireland.” Transportation Research Part A: Policy and Practice 42 (1): 15–27. https://doi.org/10.1016/j.tra.2007.06.005.
- Aminpour, F. 2022. “From the Child’s Perspective: How the Layout of Outdoor School Environments Shapes Conflict Between Children’s Self-Directed Play.” Journal of Environmental Psychology 79:101727. https://doi.org/10.1016/j.jenvp.2021.101727.
- Atkinson, K., and J. C. Whitehead. 2015. “Predictive Validity of Stated Preference Data: Evidence from Mountain Bike Park Visits Before and After Trail System Expansion.” Applied Economics Letters 22 (9): 730–733. https://doi.org/10.1080/13504851.2014.972541.
- Attaran, M., N. Alias, and S. Siraj. 2012. “Learning Culture in a Smart School: A Case Study.” Procedia - Social & Behavioral Sciences 64:417–423. https://doi.org/10.1016/j.sbspro.2012.11.049.
- Baker, J., and H. Oppewal. 2022. “The Effects of Floor Plan Representations on Preferences for Apartments.” Journal of Housing and the Built Environment 38 (2): 727–752. https://doi.org/10.1007/s10901-022-09966-w.
- Barrera-Hernández, L. F., M. A. Sotelo-Castillo, S. B. Echeverría-Castro, and C. O. Tapia-Fonllem. 2020. “Connectedness to Nature: Its Impact on Sustainable Behaviors and Happiness in Children.” Frontiers in Psychology 11:276. https://doi.org/10.3389/fpsyg.2020.00276.
- Barrett, P., F. Davies, Y. Zhang, and L. Barrett. 2015. “The Impact of Classroom Design on pupils’ Learning: Final Results of a Holistic, Multi-Level Analysis.” Building & Environment 89:118–133. https://doi.org/10.1016/j.buildenv.2015.02.013.
- Belaïd, F., and C. Massié. 2022. “What are the Salient Factors Determining the Usage of Heating Energy Sources in France? Evidence from a Discrete Choice Model.” Energy and Buildings 273:112386. https://doi.org/10.1016/j.enbuild.2022.112386.
- Bellizzi, M. G., C. Forciniti, and G. Mazzulla. 2021. “A Stated Preference Survey for Evaluating Young Pedestrians’ Preferences on Walkways.” Sustainability 13 (22): 12434. https://doi.org/10.3390/su132212434.
- Benoit, A. 2018. “Investigating the Impact of Interactive Whiteboards in Higher Education: A Case Study.” Journal of Learning Spaces 7 (1): 76–90.
- Byers, T., W. Imms, and E. Hartnell-Young. 2018. “Evaluating Teacher and Student Spatial Transition from a Traditional Classroom to an Innovative Learning Environment.” Studies in Educational Evaluation 58:156–166. https://doi.org/10.1016/j.stueduc.2018.07.004.
- Cheung, S. K. S., L. F. Kwok, K. Phusavat, and H. H. Yang. 2021. “Shaping the Future Learning Environments with Smart Elements: Challenges and Opportunities.” International Journal of Educational Technology in Higher Education 18 (1): s41239-021-00254–1. https://doi.org/10.1186/s41239-021-00254-1.
- Cheu, R. L., M. Valdez, S. Kamatham, and R. Aldouri. 2011. “Public Preferences on the Use of Visualization in the Public Involvement Process in Transportation Planning.” Transportation Research Record 2245 (1): 17–26. https://doi.org/10.3141/2245-03.
- Cho, E., Y. H. Cho, M. M. Grant, D. Song, and Y. Huh. 2020. “Trends of Educational Technology in Korea and the U.S.: A Report on the AECT-Korean Society for Educational Technology (KSET) Panel Discussion.” TechTrends 64 (3): 357–360. https://doi.org/10.1007/s11528-020-00493-5.
- Creative Commons Polska [WWW Document]. 2012. “Digital School Program with Open Textbooks Approved by Polish Government!” Accessed November 24, 2022. https://creativecommons.pl/2012/04/digital-school-program-with-open-textbooks-approved-by-polish-government/.
- Davies, D., D. Jindal-Snape, C. Collier, R. Digby, P. Hay, and A. Howe. 2013. “Creative Learning Environments in Education—A Systematic Literature Review.” Thinking Skills and Creativity 8:80–91. https://doi.org/10.1016/j.tsc.2012.07.004.
- Devotra [WWW Document]. 2015. “Devotra Smart Classroom.” Accessed August 19, 2023. https://www.devotra.nl/project-references/.
- Dillon, P. 2007. Turning Peases West Inside Out: Flexible Educational Environments for Developing Possibilities and Pedagogies. England: Creative Partnerships Durham Sunderland.
- Dubin, J. A., and D. L. McFadden. 1984. “An Econometric Analysis of Residential Electric Appliance Holdings and Consumption.” Econometrica 52 (2): 345. https://doi.org/10.2307/1911493.
- Gest, S. D., and P. C. Rodkin. 2011. “Teaching Practices and Elementary Classroom Peer Ecologies.” Journal of Applied Developmental Psychology 32 (5): 288–296. https://doi.org/10.1016/j.appdev.2011.02.004.
- Ghonoodi, A., and L. Salimi. 2011. “The Study of Elements of Curriculum in Smart Schools.” Procedia - Social & Behavioral Sciences 28:68–71. https://doi.org/10.1016/j.sbspro.2011.11.014.
- Gislason, N. 2015. “The OPEN PLAN HIGH SCHOOL Educational Motivations and Challenges.” In School Design Together, edited by P. Woolner, 101–119. Abingdon: Routledge.
- GOV.UK [WWW Document]. 2018. “Area Guidelines and Net Capacity.” Accessed November 24, 2022. https://www.gov.uk/government/publications/area-guidelines-and-net-capacity.
- Gros, B. 2016. “The Design of Smart Educational Environments.” Smart Learning Environments 3 (1): 15. https://doi.org/10.1186/s40561-016-0039-x.
- Ha7ggar, P., E. Ampatzi, D. Potoglou, and M. Schweiker. 2022. “Information Sharing Preferences within Buildings: Benefits of Cognitive Interviewing for Enhancing a Discrete Choice Experiment.” Energy and Buildings 258:111786. https://doi.org/10.1016/j.enbuild.2021.111786.
- Hassan, M., and B. Geys. 2016. “Who Should Pick Up the Bill? Distributing the Financial Burden of Technological Innovations in Schools.” Computers & Education 94:193–203. https://doi.org/10.1016/j.compedu.2015.11.018.
- Hwang, G.-J. 2014. “Definition, Framework and Research Issues of Smart Learning Environments - a Context-Aware Ubiquitous Learning Perspective.” Smart Learning Environments 1 (1): 4. https://doi.org/10.1186/s40561-014-0004-5.
- Iraganaboina, N. C., and N. Eluru. 2021. “An Examination of Factors Affecting Residential Energy Consumption Using a Multiple Discrete Continuous Approach.” Energy and Buildings 240:110934. https://doi.org/10.1016/j.enbuild.2021.110934.
- Jebril, T., and Y. Chen. 2021. “The Architectural Strategies of Classrooms for Intellectually Disabled Students in Primary Schools Regarding Space and Environment.” Ain Shams Engineering Journal 12 (1): 821–835. https://doi.org/10.1016/j.asej.2020.09.005.
- Jin, S., and L. Peng. 2022. “Classroom Perception in Higher Education: The Impact of Spatial Factors on Student Satisfaction in Lecture versus Active Learning Classrooms.” Frontiers in Psychology 13:941285. https://doi.org/10.3389/fpsyg.2022.941285.
- Joy, M., J. Foss, E. King, J. Sinclair, J. Sitthiworachart, and R. Davis. 2014. “Incorporating Technologies into a Flexible Teaching Space: Incorporating Technologies ….” British Journal of Educational Technology 45 (2): 272–284. https://doi.org/10.1111/bjet.12040.
- Kang, W. C., 2016. “Smart Learning Environment Model for Secondary Schools in Malaysia: An Overview Making a Difference in Malaysian Edu [WWW Document].” http://www.slideserve.com/reuel/smart-learningenvironment-model-for-secondary-schools-in-malaysiaan-overview-making-a-difference-in-malaysian-edu.
- Kariippanon, K. E., D. P. Cliff, S. L. Lancaster, A. D. Okely, and A.-M. Parrish. 2018. “Perceived Interplay Between Flexible Learning Spaces and Teaching, Learning and Student Wellbeing.” Learning Environments Research 21 (3): 301–320. https://doi.org/10.1007/s10984-017-9254-9.
- Kariippanon, K. E., D. P. Cliff, A. D. Okely, and A.-M. Parrish. 2020. “The ‘Why’ and ‘How’ of Flexible Learning Spaces: A Complex Adaptive Systems Analysis.” Journal of Educational Change 21 (4): 569–593. https://doi.org/10.1007/s10833-019-09364-0.
- Kasraian, D., S. Adhikari, D. Kossowsky, M. Luubert, G. B. Hall, J. Hawkins, K. Nurul Habib, and M. J. Roorda. 2021. “Evaluating Pedestrian Perceptions of Street Design with a 3D Stated Preference Survey.” Environment and Planning B: Urban Analytics and City Science 48 (7): 1787–1805. https://doi.org/10.1177/2399808320946050.
- Kelemen, D., S. A. Brown, and L. Pizza. 2023. “Don’t Bug Me!: The Role of Names, Functions, and Feelings in Shaping Children’s and adults’ Conservation Attitudes About Unappealing Species.” Journal of Environmental Psychology 87:101990. https://doi.org/10.1016/j.jenvp.2023.101990.
- Kelly, C. E., M. R. Tight, F. C. Hodgson, and M. W. Page. 2011. “A Comparison of Three Methods for Assessing the Walkability of the Pedestrian Environment.” Journal of Transport Geography 19 (6): 1500–1508. https://doi.org/10.1016/j.jtrangeo.2010.08.001.
- Levinson, D., K. Harder, J. Bloomfield, and K. Carlson. 2006. “Waiting Tolerance: Ramp Delay Vs. Freeway Congestion.” Transportation Research Part F: Traffic Psychology and Behaviour 9 (1): 1–13. https://doi.org/10.1016/j.trf.2005.08.003.
- Liu, C., L. Sun, X. Jing, Y. Zhang, X. Meng, C. Jia, and W. Gao. 2022. “How Correlated Color Temperature (CCT) Affects Undergraduates: A Psychological and Physiological Evaluation.” Journal of Building Engineering 45:103573. https://doi.org/10.1016/j.jobe.2021.103573.
- Looi, C.-K., P. Seow, B. Zhang, H.-J. So, W. Chen, and L.-H. Wong. 2010. “Leveraging Mobile Technology for Sustainable Seamless Learning: A Research Agenda.” British Journal of Educational Technology 41 (2): 154–169. https://doi.org/10.1111/j.1467-8535.2008.00912.x.
- Lovreglio, R., E. Dillies, E. Kuligowski, A. Rahouti, and M. Haghani. 2022. “Exit Choice in Built Environment Evacuation Combining Immersive Virtual Reality and Discrete Choice Modelling.” Automation in Construction 141:104452. https://doi.org/10.1016/j.autcon.2022.104452.
- Lu, J., Y. Meng, H. Timmermans, and A. Zhang. 2021. “Modeling Hesitancy in Airport Choice: A Comparison of Discrete Choice and Machine Learning Methods.” Transportation Research Part A: Policy and Practice 147:230–250. https://doi.org/10.1016/j.tra.2021.03.006.
- MacLeod, J., H. H. Yang, S. Zhu, and Y. Li. 2018. “Understanding students’ Preferences Toward the Smart Classroom Learning Environment: Development and Validation of an Instrument.” Computers & Education 122:80–91. https://doi.org/10.1016/j.compedu.2018.03.015.
- Marx, A., U. Fuhrer, and T. Hartig. 2000. “Effects of Classroom Seating Arrangements on Children’s Question-Asking.” Learning Environments Research 2 (3): 249–263. https://doi.org/10.1023/A:1009901922191.
- Ma, H., and W. Zhang, 2016. “Residents’ Recreation Preference of the Vehicle Campsite in Beijing Based on the Stated Preference Method.” In: 2016 International Conference on Smart City and Systems Engineering (ICSCSE). Presented at the 2016 International Conference on Smart City and Systems Engineering (ICSCSE), 14–17. Hunan, China: IEEE. https://doi.org/10.1109/ICSCSE.2016.0014
- Mogas Recalde, J., R. Palau, N. Lorenzo Gales, and R. Gallon. 2020. “Developments for Smart Classrooms: School Perspectives and Needs.” International Journal of Mobile and Blended Learning 12 (4): 34–50. https://doi.org/10.4018/IJMBL.2020100103.
- Monchambert, G. 2020. “Why Do (Or don’t) People Carpool for Long Distance Trips? A Discrete Choice Experiment in France.” Transportation Research Part A: Policy and Practice 132:911–931. https://doi.org/10.1016/j.tra.2019.12.033.
- Nambiar, M. K. R., N. Mohd nor, K. Ismail, and S. Adam. 2017. “New Learning Spaces and Transformations in Teacher Pedagogy and Student Learning Behavior in the Language Learning Classroom.” 3L: The Southeast Asian Journal of English Language Studies 3L (4): 29–40. https://doi.org/10.17576/3L-2017-2304-03.
- Newton, C., S. Backhouse, A. Aibinu, B. Cleveland, R. Crawford, D. Holzer, P. Soccio, and T. Kvan. 2018. “Plug N Play: Future Prefab for Smart Green Schools.” Buildings 8 (7): 88. https://doi.org/10.3390/buildings8070088.
- Omidinia, S., M. Masrom, and H. Selamat. 2012. “DETERMINANTS of SMART SCHOOL SYSTEM SUCCESS: A CASE STUDY of MALAYSIA.” International Journal of Academic Research 4 (4): 107–115. https://doi.org/10.7813/2075-4124.2012/4-4/B.16.
- Ong, E. T., and K. Ruthven. 2010. “The Distinctiveness and Effectiveness of Science Teaching in the Malaysian ‘Smart school’.” Research in Science & Technological Education 28 (1): 25–41. https://doi.org/10.1080/02635140903513557.
- Park, J. C., M. H. Chung, and E. K. Rhee. 2011. “Field Survey on the Indoor Environment of Elementary Schools for Planning of Environment Friendly School Facilities.” Journal of Asian Architecture and Building Engineering 10 (2): 461–468. https://doi.org/10.3130/jaabe.10.461.
- Peng, L., Y. Deng, and S. Jin. 2022. “The Evaluation of Active Learning Classrooms: Impact of Spatial Factors on Students’ Learning Experience and Learning Engagement.” Learning Experience and Learning Engagement Sustainability 14 (8): 4839. https://doi.org/10.3390/su14084839.
- Perdomo, M., A. Rezaei, Z. Patterson, N. Saunier, and L. F. Miranda-Moreno. 2014. “Pedestrian Preferences with Respect to Roundabouts—A Video-Based Stated Preference Survey.” Accident Analysis & Prevention 70:84–91. https://doi.org/10.1016/j.aap.2014.03.010.
- Perks, T., D. Orr, and E. Al-Omari. 2016. “Classroom Re-Design to Facilitate Student Learning: A Case Study of Changes to a University Classroom.” Journal of the Scholarship of Teaching and Learning 16 (1): 53–68. https://doi.org/10.14434/josotl.v16i1.19190.
- Pettigrew, T. F. 1998. “INTERGROUP CONTACT THEORY.” Annual Review of Psychology 49 (1): 65–85. https://doi.org/10.1146/annurev.psych.49.1.65.
- Radcliffe, D. 2008. “A Pedagogy-Space-Technology (PST) Framework for Designing and Evaluating Learning Places.” Learning spaces in higher education: Positive outcomes by design: Proceedings of the next generation learning spaces, University of Queensland, Brisbane, 2008. Vol.1., pp. 11–16.
- Rezaei, A. 2020. “Groupwork in Active Learning Classrooms: Recommendations for Users.” Journal of Learning Spaces 9 (2): 1–21.
- Shimokura, R., K. Yanagiawa, and S. Sasaki. 2023. “Spatial Organisation of “Therapeutic” Spaces for Autistic Children in Special Schools: Lessons Learnt from the United Kingdom Experience.” Journal of Asian Architecture and Building Engineering 22 (2): 620–634. https://doi.org/10.1080/13467581.2022.2047982.
- Shi, Y., H. Yang, J. Zhang, Q. Pu, and H. H. Yang, 2020. “A Meta-Analysis of Students’ Cognitive Learning Outcomes in Smart Classroom-Based Instruction.” In: 2020 International Symposium on Educational Technology (ISET). Presented at the 2020 International Symposium on Educational Technology (ISET), 8–12. Bangkok, Thailand: IEEE. https://doi.org/10.1109/ISET49818.2020.00012
- Sitthiworachart, J., M. Joy, E. King, J. Sinclair, and J. Foss. 2022. “Technology-Supported Active Learning in a Flexible Teaching Space.” Education Sciences 12 (9): 634. https://doi.org/10.3390/educsci12090634.
- Sleegers, P., N. Moolenaar, M. Galetzka, A. Pruyn, B. Sarroukh, and B. van der Zande. 2013. “Lighting Affects students’ Concentration Positively: Findings from Three Dutch Studies.” Lighting Research & Technology 45 (2): 159–175. https://doi.org/10.1177/1477153512446099.
- Smart Schools NY [WWW Document]. 2014. Smart Schools NY. Accessed August 19, 2023. https://www.ny.gov/programs/smart-schools-ny.
- Smith, C. 2017. “The Influence of Hierarchy and Layout Geometry in the Design of Learning Spaces.” Journal of Learning Spaces 6 (3): 59–67.
- Suárez-Guerrero, C., C. Lloret-Catalá, and S. Mengual-Andrés. 2016. “Teachers’ Perceptions of the Digital Transformation of the Classroom Through the Use of Tablets: A Study in Spain.” Comunicar Revista Científica de Comunicación y Educación 24:81–89. https://doi.org/10.3916/C49-2016-08.
- Tapia-Fonllem, C., B. Fraijo-Sing, V. Corral-Verdugo, G. Garza-Terán, and M. Moreno-Barahona. 2020. “School Environments and Elementary School Children’s Well-Being in Northwestern Mexico.” Frontiers in Psychology 11:510. https://doi.org/10.3389/fpsyg.2020.00510.
- Terzieva, V., S. Ilchev, and K. Todorova. 2022. “The Role of Internet of Things in Smart Education.” IFAC-Papersonline 55 (11): 108–113. https://doi.org/10.1016/j.ifacol.2022.08.057.
- UNESCO [WWW Document]. 2010. “Singapore: Future Schools | ICT in Education Policy Toolkit.” Accessed November 24, 2022. https://en.unesco.org/icted/content/singapore-future-schools.
- UNESCO [WWW Document]. 2014. “iTec: Designing the Future Classroom | ICT in Education Policy Toolkit” Accessed November 24, 2022. https://en.unesco.org/icted/content/itec-designing-future-classroom.
- van den Berg, J., R. Appel-Meulenbroek, A. Kemperman, and M. Sotthewes. 2020. “Knowledge workers’ Stated Preferences for Important Characteristics of Activity-Based Workspaces.” Building Research & Information 48 (7): 703–718. https://doi.org/10.1080/09613218.2020.1726169.
- van den Bogerd, N., S. C. Dijkstra, K. Tanja-Dijkstra, M. R. de Boer, J. C. Seidell, S. L. Koole, and J. Maas. 2020. “Greening the Classroom: Three Field Experiments on the Effects of Indoor Nature on students’ Attention, Well-Being, and Perceived Environmental Quality.” Building and Environment 171:106675. https://doi.org/10.1016/j.buildenv.2020.106675.
- Verhetsel, A., R. Kessels, T. Zijlstra, and M. Van Bavel. 2017. “Housing Preferences Among Students: Collective Housing versus Individual Accommodations? A Stated Preference Study in Antwerp (Belgium).” Journal of Housing and the Built Environment 32 (3): 449–470. https://doi.org/10.1007/s10901-016-9522-5.
- Wang, J., D. E. H. Tigelaar, J. Luo, and W. Admiraal. 2022. “Teacher Beliefs, Classroom Process Quality, and Student Engagement in the Smart Classroom Learning Environment: A Multilevel Analysis.” Computers & Education 183:104501. https://doi.org/10.1016/j.compedu.2022.104501.
- Wannarka, R., and K. Ruhl. 2008. “Seating Arrangements That Promote Positive Academic and Behavioural Outcomes: A Review of Empirical Research.” Support for Learning 23 (2): 89–93. https://doi.org/10.1111/j.1467-9604.2008.00375.x.
- Woodward, E., and M. P. Zari. 2018. “Reconnecting children with nature: biophilic primary school learning environments 14.” ZEMCH 2018 International Conference, Melbourne, Australia, 2018. 581–593 .
- Yang, L. 2018. “Modeling the Mobility Choices of Older People in a Transit-Oriented City: Policy Insights.” Habitat International 76:10–18. https://doi.org/10.1016/j.habitatint.2018.05.007.
- Yaseen, F. R., and F. A. Mustafa. 2022. “Visibility of Nature-Connectedness in School Buildings: An Analytical Study Using Biophilic Parameters, Space Syntax, and Space/Nature Syntax.” Ain Shams Engineering Journal 14 (5): 101973. https://doi.org/10.1016/j.asej.2022.101973.
- Yeo, S., P. Taylor, and M. Kulski. 2006. “Internationalising a Learning Environment Instrument for Evaluating Transnational Online University Courses.” Learning Environments Research 9 (2): 179–194. https://doi.org/10.1007/s10984-006-9008-6.
- Zhu, M. 2021. “Examining Social Learning in an Active Learning Classroom Through Pedagogy-Space-Technology Frame-work.” Journal of Learning Spaces 10 (1): 15–26.
- Zhu, Z.-T., M.-H. Yu, and P. Riezebos. 2016. “A Research Framework of Smart Education.” Smart Learning Environments 3 (1): 4. https://doi.org/10.1186/s40561-016-0026-2.
- Zimmermann, P. A., R. L. Pierce, L. Stallings, and D. Largent. 2018. “Classroom Interaction Redefined: Multidisciplinary Perspectives on Moving Beyond Traditional Classroom Spaces to Promote Student Engagement.” Journal of Learning Spaces 7 (1): 45–61.