ABSTRACT
Can individuals look for multiple objects at the same time? A simple question, but answering it has proven difficult. In this review, we describe possible cognitive architectures and their predictions about the capacity of visual search. We broadly distinguish three stages at which limitations may occur: (1) preparation (establishing and maintaining a mental representation of a search target), (2) selection (using this mental representation to extract candidate targets from the visual input), and (3) post-selection processing (verifying that the selected information actually is a target). We then review the empirical evidence from various paradigms, together with their strengths and pitfalls. The emerging picture is that multiple target search comes with costs, but the magnitude of this cost differs depending on the processing stage. Selection appears strongly limited, while preparation of multiple search target representations in anticipation of a search is possible with relatively small costs. Finally, there is currently not sufficient information to determine the capacity limitations of post-selection processing. We hope that our review contributes to better targeted research into the mechanisms of multiple-target search. A better understanding of multiple-target search will also contribute to better design of real-life multiple-target search problems, reducing the risk of detrimental search failures.
It is hard to imagine an aspect of daily life in which visual search does not play a critical role. Whether it is a friend in a crowd, your destination on a map, or simply the mouse cursor on your computer monitor, individuals spend a considerable amount of their time searching for behaviourally relevant information from among an immense stream of mostly irrelevant visual input. Anyone who has tried to find a particular product in a supermarket aisle has encountered that visual search is limited by what we can attend to at any moment in time. These limitations are part and parcel of theories of visual search and of selective attention in general (Bundesen, Citation1990; Bundesen et al., Citation2005; Desimone & Duncan, Citation1995; Huang & Pashler, Citation2007; Treisman, Citation1988; Treisman & Gelade, Citation1980; Wolfe, Citation1994, Citation2007). However, the same limitations are also central to an important and currently much debated question: Can individuals look for multiple objects at the same time? As a society, we frequently ask people to look for multiple pieces of visual information simultaneously, often in serious and sometimes life-critical situations. For example, radiologists check x-ray scans for multiple abnormalities, and airport security screeners scan luggage for a wide range of potential threats. A better understanding of the limitations of multiple-target search (MTS) will likely contribute to a better design of the visual environment and reduce the risk of search failures and its detrimental consequences.
Although the question “Can we look for multiple things at the same time?” is a simple one, the answer has proven remarkably difficult. To illustrate some of the difficulties, imagine we ask a friend to go to the supermarket and bring back both tea brand A and tea brand B. If the friend indeed returns with both teas, this alone is no evidence that she looked for both brands simultaneously, in parallel, as she may of course have looked for one brand first, and then the other. She may even have been alternating back and forth between looking for brand A and looking for brand B. The critical element here at the same time will be difficult to demonstrate without precise knowledge about the underlying cognitive processes at every single moment. Also, even if we were somehow to demonstrate that the person did not look for two things simultaneously, this does not exclude the possibility that she could have done so when pressed. Conversely, even if we find evidence for some simultaneity, how simultaneous does it have to be for it to count as truly simultaneous? Is some temporal overlap at some processing stage sufficient, or do the underlying processes need to have a fully identical time course? To add to the confusion, the precise interpretation of “looking for two things simultaneously” may also vary across context. For example, in everyday language, looking for either brand A or brand B would probably also count as looking for multiple things. In fact, even the simple verb “to look for” may mean both a mental state (i.e., what is one set out to find) and a behaviour (i.e., the act of actually scanning the environment for something) – each of which may face different limitations.
Part of the reason for a lack of consensus on how many things individuals can look for simultaneously is that different studies have been focusing on different aspects of these questions. Moreover, different research groups have used different paradigms, each with their own strengths, but also each with their own idiosyncrasies and shortcomings. Thus, the answer to the question whether we can simultaneously look for multiple target objects depends on who is asked and what approach they have taken to study it. Considering all this, we believe that a comprehensive review on approaches used to study multiple-target search and the current state of the field is warranted. The aim of this review article is threefold. First, we provide a general framework of theoretical factors relevant for determining the capacity of a cognitive system, and what these factors predict for the capacity of visual search. Second, we review the literature on the different approaches to the question of multiple-target search and evaluate which architecture they support. To this end, we conceptually describe the paradigms, their usefulness and their pitfalls. Finally, we integrate the evidence and conclude which questions should be answered, and which knowledge gaps are left for future research.
Theoretical issues
Whether or not individuals can look for multiple targets simultaneously is in essence a question of capacity, which in itself has multiple components. First, we can ask whether the system is somehow limited or not. Next, if it is limited, it naturally follows to ask (a) what the capacity is – that is, how much information can be stored or used; (b) why there is a limitation – that is, is processing of serial or limited parallel nature; and (c) where is the limitation, that is, which stage or stages of processing are subject to a limitation. To start with the latter, we first need to establish which processing stages are involved in visual search in general, even when only one target is searched for.
The visual search process
The goal of visual search behaviour is to select a relevant piece of visual information (typically some form of object) from among competing stimuli. This competition is expressed as mutual suppression through inhibitory connections between neural populations that code for different information (Beck & Kastner, Citation2009; Kastner & Ungerleider, Citation2000, Citation2001). To serve adaptive behaviour, the competition for selection can be biased in favour of potentially relevant information in three different ways (e.g., Awh et al., Citation2012; Corbetta & Shulman, Citation2002). First, stimulus-driven biases cause stimuli that stand out from their background to be preferentially processed (e.g., Itti & Koch, Citation2000; Theeuwes, Citation1992). Second, implicit biases cause stimuli that have previously been selected to be selected again (e.g., Failing & Theeuwes, Citation2018; Á. Kristjánsson & Campana, Citation2010; Maljkovic & Nakayama, Citation1994; Urai et al., Citation2019). However, as the goal of visual search typically changes from situation to situation, in the current review we will focus on a third source of bias, namely top-down, goal-directed mechanisms, which establish the flexible, endogenous biases that enable the observer to adapt to dynamically changing task goals (Baluch & Itti, Citation2011; Chelazzi et al., Citation1993; Desimone & Duncan, Citation1995).
(a) presents what we regard as the canonical, core model of such goal-driven visual search (Chelazzi et al., Citation1993; Desimone & Duncan, Citation1995; Duncan & Humphreys, Citation1989; Eimer, Citation2014; Huang & Pashler, Citation2007; Wolfe, Citation1994).Footnote1 First, prior to search, during the preparation stage, the observer activates a memory representation of the target object or its most important defining feature(s) – for example red and round when looking for tomatoes. This memory representation is typically referred to as the search template, attentional set, or attentional control settings (henceforth simply template). The template has two important characteristics. First, for visual search behaviour to be able to adapt to changing task demands, the relevant memory representation must be flexible. Most theories therefore assume the template to be activated in visual working memory (VWM, Carlisle et al., Citation2011; Desimone & Duncan, Citation1995; Duncan & Humphreys, Citation1989; Olivers & Eimer, Citation2011; though see Wolfe, Citation2012) and it is thought to be controlled by a fronto-parietal control network (Bichot et al., Citation2015; Greenberg et al., Citation2010; Liu et al., Citation2003). Second, for the memory representation to actually act like a search template – that is, to guide the subsequent search process and engage in selection – it must be able to bias the incoming sensory representations to prioritize matching visual objects (Wolfe, Citation1994). For this, the fronto-parietal control network, presumably through long-range feedback connections that likely include subcortical-thalamic routes (Nakajima et al., Citation2019), is thought to increase the gain for neural populations in visual cortex that process the sought-for feature(s) throughout the visual field (Chelazzi et al., Citation1993; Maunsell & Treue, Citation2006; Saenz et al., Citation2002; Treue & Trujillo Martínez, Citation1999). It is worth noting here though, that not all VWM representations necessarily result in attentional biases, and that VWM representations may be flexibly gated to either drive attention or not (Carlisle et al., Citation2011; Carlisle & Woodman, Citation2011; de Vries et al., Citation2017; Kiyonaga et al., Citation2012; Olivers et al., Citation2011; van Loon et al., Citation2017).
Figure 1. Models of visual search. (a) The canonical sequence of visual search stages. When an individual sets out to look for an object (e.g., a tomato), first a template needs to be prepared and set up in VWM (preparation stage). Once the search display appears and the visual input enters the system, the template, through recurrent feedback mechanisms, interacts with the visual information and guides attention towards regions that match the target feature(s). Finally, the template may be used to confirm that the selected information is indeed what an observers was looking for. (b) Theoretical possibilities for the capacity of multiple-target search. Search for multiple targets can be subject to unlimited processing (multiple targets can be sought for at the same time with similar efficiency), limited parallel processing (multiple targets can be sought for at the same time, but the more targets are searched for, the less efficient search becomes), limited serial processing (only one item can be processed at a time, and observers need to alternate between searching for each target), or weighted parallel processing (multiple targets can be sought for at the same time, but search targets differ in relative priority).
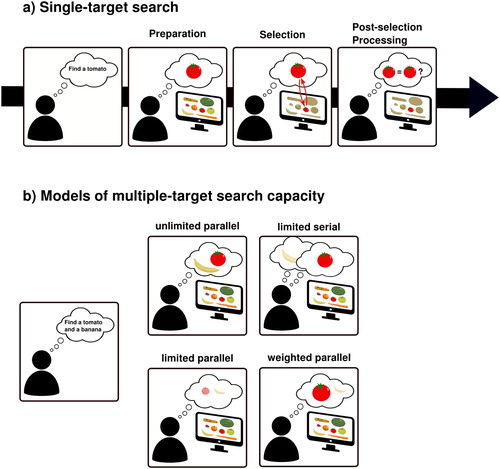
The next stage we refer to as the selection process. Selection starts when the actual search display is presented, and multiple objects begin to compete for representation. In this race for selection, template-matching objects will have a competitive advantage as the sensitivity of corresponding neuronal populations has been enhanced. Specifically, the interaction between top-down and bottom-up signals will result in strong recurrent activity, enabling the matching signal to win the competition with other signals. Thus, this way, the search process is converted from an initially visual-field-wide attentional set for a feature into a location-specific enhancement of candidate objects, thus “guiding attention” to relevant signals (Eimer, Citation2014; Moran & Desimone, Citation1985; Motter, Citation1994).Footnote2
The local enhancement of an object representation allows its location and other features to be broadcasted to other systems, for example, those driving eye movements or recognition. This is useful for the final, post-selection processing stage in which the observer verifies whether the selected object is indeed the target. If so, the object is then acted upon accordingly. If not, the object is suppressed (Klein & MacInnes, Citation1999) and the search continues with the remaining candidates, until the target is found or a termination criterion is met (Wolfe & Van Wert, Citation2010).
Critically, the question of whether one can look for multiple targets simultaneously can be evaluated at all of these stages. In particular, when talking about capacity limitations, this could refer to the number of visual memory representations that can simultaneously be active during search preparation, or to limitations in how these memories interact with the visual input during both selection and post-selection processes. In the next section, we discuss what such capacity limits might in principle look like. Note that there are other limitations that are not directly related to the problem of MTS and that we will therefore not treat, for example when stimulus discriminability is so low that it requires individuals to make serial eye movements in order to detect task-relevant features.
Serial versus parallel processing
Theoretically, the capacity of a cognitive system could be unlimited, limited by long-term memory (LTM), by VWM capacity, or by even stronger restrictions, down to one search at a time. Whatever the capacity limitation is, the underlying system that gave rise to it can be based on serial, parallel or even co-active processing. Importantly, even though the questions of capacity and seriality are related, they are not redundant, as limitations can be caused by both serial and parallel systems (Eckstein, Citation2011; Liesefeld & Müller, Citation2020; Snodgrass & Townsend, Citation1980; Townsend, Citation1990; Townsend & Ashby, Citation1983). Furthermore, it has been shown that even unlimited systems can produce data patterns that mimic those of limited systems, for example, if processing is subject to stochastic noise (e.g., Snodgrass & Townsend, Citation1980). Moreover, any of the aforementioned processing stages (preparation, selection, or post-selection) may be parallel or serial in nature. Nevertheless, as the concepts of capacity and seriality are intricately linked, in what follows, we will jointly evaluate potential capacity limitations and the underlying architecture that gives rise to them.
A variety of models is illustrated in (b) and listed in .Footnote3 First, in a system that is truly unlimited, the processing of items does not depend on the total number of items processed and processing is parallel at all stages of the search. To our knowledge there are no completely unlimited, parallel models of MTS.Footnote4 In what may come closest to an unlimited model, Wolfe and colleagues (Drew et al., Citation2017; Drew & Wolfe, Citation2014; Wolfe, Citation2012) have proposed that for certain types of search, MTS is limited by whatever set of targets observers have encoded into long-term memory within the context of a particular experiment. For example, they found that after sufficient training, observers can look for up to 100 different targets. We will discuss later how these data might be interpreted, but Wolfe and colleagues concluded that observers can successfully combine visual search with a search through LTM.
Table 1. Schematic description of possible architectures underlying MTS. Note that any of these limitations can be present at any search-related processing stage.
Limitations may be different for flexible, VWM-driven search. For example, Hollingworth and colleagues (Bahle et al., Citation2018; Beck et al., Citation2012) have argued that MTS for newly instructed targets is essentially only limited by the capacity of VWM, so that within VWM capacity, search is simultaneously guided by multiple templates (VWM-limited parallel model). In this sense, VWM of a feature and a top-down attentional bias for that feature become functionally one and the same mechanism (cf. Desimone & Duncan, Citation1995; Serences, Citation2016). Given a VWM capacity of approximately four objects (Cowan, Citation2001), search for up to four targets simultaneously should therefore be possible.
Others have argued that MTS capacity is actually smaller than VWM capacity, such that the more items an observer is looking for, the less efficient search becomes – a decrement usually referred to as a multiple-target cost. This limitation can then arise either because of a serial bottleneck (limited serial model), as has been advocated by for example the Olivers and Roelfsema groups (Houtkamp & Roelfsema, Citation2009; Olivers et al., Citation2011; Ort et al., Citation2017) and is also a key aspect of Huang and Pashler’s Boolean Map Theory (Citation2007). Or it arises because of less efficient parallel processing at one or multiple search stages (limited parallel model; Barrett & Zobay, Citation2014; Ort, Fahrenfort, ten Cate, et al., Citation2019). While the limited parallel model assumes that some limited resource in the system is distributed over multiple processes or representations, thus resulting in less efficient processing of each item, the limited serial model predicts that the only way the system can solve MTS is essentially by switching, or even repeatedly alternating, between searching separately for each one of the search targets. Assuming that such switching takes time, the serial model predicts clear switch costs in performance.
In addition to these main classes of models, there are also a number of hybrid options conceivable, some of which are very difficult to differentiate from each other. For example, a model in which the processing capacity is conceptualized as a resource that is flexibly distributed over all target representations (cf. Bays & Husain, Citation2008; Ma et al., Citation2014; Williams et al., Citation2019), but in an unequal way, such that one representation receives the bulk of the resource at the expense of the other, would make predictions very similar to those of the serial models. Another possibility would be a system that relies on both parallel and serial subsystems. For example, multiple templates might be set up to simultaneously enhance multiple visual features and thus guide attention to potentially relevant information, but then only one template can be used to select and extract information from a location at any one time. In this scenario, preparation would be parallel (limited or unlimited) while selection would be serial (limited).
Empirical evidence
We next examine these potential architectures against the empirical evidence. The question of multiple-target search has been examined with a variety of different approaches, each with its own merits and caveats. A selection of the most commonly used approaches is illustrated in .
Figure 2. Commonly used paradigms to test the capacity of multiple-target search. (a) Multiple-target cost. Individuals have to either look for one or for multiple targets in a search display. If performance is lower for the multiple-target search compared to the single-target search, one speaks of a multiple-target cost. This cost is indicative that the capacity of multiple-target search is limited. Figure adapted from Houtkamp and Roelfsema (Citation2009). (b) Redundancy gain. If individuals have to report the presence of either of two target items in a search display, responses are usually faster when both are present in the search. This alone is to be expected under serial and parallel models. However, if responses are faster than the fastest response to a single target – a so-called race model violation – this would be evidence that both target items guided attention in an integrated way, indicative of co-active parallel visual search. Figure adapted from Bahle et al. (Citation2019). (c) Switch cost. If looking for multiple targets at the same time is not possible, individuals have to alternate between two single-target searches in order to find all targets. This alternation is considered a costly process. Therefore, when observers are being instructed to look for two targets and the relevant target either repeats or changes across trials, less efficient search (slower search times, or lower accuracy) on switch trials compared to repeat trials suggests that individuals indeed alternated, and did not look for both targets simultaneously. Figure adapted from Ort et al. (Citation2017). (d) Foraging tasks – run length. individuals are instructed to select all targets from a cluttered display with many targets presented among distractors. It is assumed that under a serial model individuals would try to avoid switch costs and stick to the same target for longer runs. Conversely, short run lengths, mimicking random selections of targets, are consistent with multiple parallel biases. During feature multiple-target search (targets: red & green), run lengths are close to what would be expected by random selection of either target (consistent with parallel MTS), whereas during conjunction MTS (targets: red squares & green circles) runs are much longer (suggesting serial MTS). Figure adapted from Kristjánsson et al. (Citation2014). (e) Memory-based attentional capture. Participants are instructed to memorize an item for a later memory task. Prior to the memory test however, an unrelated search is injected, in which participants need to find a shape singleton. On different trials, zero, one, or two of the memorized colours could appear in the search display as distractors. Capture by memory-matching distractors is evidence that those colours guided attention. Figure adapted from Hollingworth and Beck (Citation2016).
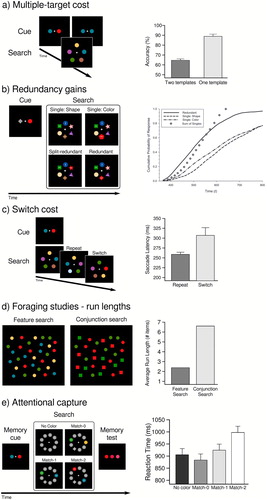
Overall multiple-target costs
Behavioural evidence
A straightforward approach to investigate multiple-target search is to let people search for multiple different target objects or features and compare their performance to single-target search (see (a)). The actual target present in the display can then be unpredictably any one of those targets. If search performance decreases with increasing target load, MTS is considered to be limited at least at one stage.
It appears that in certain situations, individuals are able to look for multiple targets without any cost. One such situation occurs when search targets are adjacent in feature space (e.g., looking for red and orange targets among green and turquoise distractors). However, it is doubtful to what extent this scenario represents true MTS, as a single template (“e.g., red-ish orange”) could be set up to guide attention to either of these features (D’Zmura, Citation1991; Hout & Goldinger, Citation2015; Menneer et al., Citation2009; Stroud et al., Citation2019), rendering search essentially a single target search for a broadly or fuzzily defined target.
Most other studies comparing multiple- to single-target search have found performance costs both in accuracy and in response times already from one additional target onward, indicating that MTS is indeed limited somewhere in the processing chain (Barrett & Zobay, Citation2014; Duncan, Citation1980; Hout & Goldinger, Citation2012, Citation2015; Kaplan & Carvellas, Citation1965; Liu et al., Citation2013; Liu & Jigo, Citation2017; Menneer et al., Citation2007; Menneer et al., Citation2009, Citation2012; Mestry et al., Citation2017; C. M. Moore & Osman, Citation1993). The important question is then what these overall costs mean for the underlying architecture – that is, whether a limited parallel or a limited serial system is responsible for the overall MTS cost, and at which stage. Two groups have tried to model the processing mode during a MTS task, using models based on signal detection theory. Houtkamp and Roelfsema (Citation2009) found that a model with a capacity limit of a single item (and therefore serial processing) was most supported by the data. In follow-up work, Barrett and Zobay (Citation2014, Citation2019) confirmed that a limited capacity model described the data well, but in contrast to Houtkamp and Roelfsema, they found that a limited parallel model best accounted for the data. They argued that the more targets need to be found, the fewer resources are available to implement a top-down bias for all target features, and the noisier the search process becomes.
With regards to the processing stage at which such limitations play out, manual responses have not been very informative. Here, oculomotor measures have proven more useful. Given that eye movements are tightly linked to the attentional system (e.g., Awh et al., Citation2006; Deubel & Schneider, Citation1996), measures like the latency of the first eye movement, or the selectivity of fixations on target items can be taken to reflect the effect of preparation and interactions of top-down biases with the visual input leading up to selection, while fixation duration on an item is taken as indicative of post-selection decision processes. Studies that used oculomotor measures indeed indicate that limitations of MTS emerge at the earlier stages of either preparation or attentional guidance prior to selection, as both latency and selectivity of saccades worsens with more targets to look for (Barrett & Zobay, Citation2019; Beck et al., Citation2012; Cave et al., Citation2018; Hout & Goldinger, Citation2015; Stroud et al., Citation2011; Stroud et al., Citation2012; Stroud et al., Citation2019). However, there is also evidence for a limitation of post-selection decision processes, as is expressed in fixation durations (Hout & Goldinger, Citation2015). In the next section, we will turn to electrophysiological evidence that further specifies which stages are affected by multiple-target costs.Footnote5
Electrophysiological evidence
Electroencephalography (EEG) is a promising technique to examine the limitations of MTS, as it has the potential to provide a more continuous measure of cognitive processing (Luck, Citation2014). One useful measure is the N2pc, a negatively deflecting event-related potential over posterior electrodes that emerges approximately 200 ms after stimulus onset contralateral to the hemifield to which individuals attend (Eimer, Citation1996; Luck & Hillyard, Citation1994). It is generally associated with selection of task-relevant objects, and thus effects on the N2pc may reflect limitations in any process leading up to it, including preparation. A number of MTS studies have revealed that the N2pc indeed deteriorates when people search for two rather than one target, manifested in a delay in N2pc onset latency, a reduced amplitude, and reduced specificity, expressed as non-target features also capturing attention (Grubert et al., Citation2016; Grubert & Eimer, Citation2013, Citation2015a). Although informative with regards to the presence of costs, the coarse spatial resolution of the N2pc has the drawback that it is difficult to actually measure the response to two targets simultaneously. Instead, conclusions have to be based on trial averages, and it is therefore unclear whether the N2pc modulations reflect serial or parallel limitations. Finally, note that the N2pc actually does not provide a continuous measure of attention across time, as it reflects a relatively brief modulation of an ERP component around 200–300 ms in response to the onset of a stimulus.
A potentially more continuous measure of top-down bias for specific features is provided by so-called steady-state visual evoked potentials (SSVEPs). An SSVEP is an oscillation in the visual cortex driven by a flickering stimulus in the visual field. It has been shown that attending to a stimulus increases the amplitude of an SSVEP, irrespective of the stimulus’ location in the visual field (Müller et al., Citation2003; Norcia et al., Citation2015). Furthermore, as the frequency of the SSVEP matches the frequency of the stimulus, it becomes possible to present multiple items carrying different features in the visual field, each flickering at its own frequency, and use SSVEP amplitude changes to monitor which feature was attended (Andersen et al., Citation2009; Andersen et al., Citation2011). Using this technique, Andersen et al. (Citation2013; see also Martinovic et al., Citation2018) found that attending to two different colours led to enhancement of the SSVEP response for both colours, relative to unattended colours. However, there was no direct comparison to a single colour baseline condition in these experiments. Andersen et al. (Citation2009) did report small costs in SSVEP amplitude when compared to a single target feature baseline. However, in the two features condition, the two target colours were the only colours present (there were no non-target colours), which in principle allowed observers to not be selective at all and might thus have resulted in an underestimation of multiple feature costs. In a different set of studies, the same research group has compared attending to conjunctions of features (i.e., attend both colour and orientation) to attending to either feature alone, and found largely additive effects (Andersen et al., Citation2008; Andersen et al., Citation2015). That is, the SSVEP response to, say, a red horizontal bar, was close to the sum of the SSVEP responses to red items and horizontal items. The authors interpreted this evidence for parallel, independent modulation of these different feature biases. As we will also return to in section on redundancy gains, additive effects per se are no evidence for a parallel model, as they can also emerge from a serial model in which observers attend to each of the features in turn. To address this concern Andersen et al. (Citation2008, Supplementary Material) performed a trial-based correlation analysis. A serial model would predict an anti-correlation in which modulation of one feature would go together with no modulation of the other feature. In support of the parallel model, no such correlation was found. However, one difference with regular MTS is that in conjunction search, the two features combine into one and the same target object, rather than representing two different targets. Remembering and looking for conjunctions may face different capacity limitations than looking for two separately defined targets. In any case, taken together, the findings using the SSVEP technique point towards a limited capacity parallel model of multiple simultaneous attentional biases (though see Thigpen et al., Citation2019 for evidence for serial switching). What is still not clear though is whether such limitations arise in the selection stage, or already in the preparation stage (i.e., maintaining multiple templates). Nor does the SSVEP technique allow for a very fine-grained time course assessment, as it requires a considerable time window to aggregate the frequency signals.
Recently, we used a multivariate decoding technique (Fahrenfort et al., Citation2017; Fahrenfort et al., Citation2018) on the raw EEG signal to continuously and simultaneously track attentional selection of multiple target objects at a millisecond resolution (Ort, Fahrenfort, ten Cate, et al., Citation2019). Moreover, the study was specifically designed to dissociate any multiple-target costs originating during the preparation of multiple biases from the process of selecting multiple matching stimuli from the search display. To do so, we independently manipulated the number of features that observers needed to memorize as templates for an upcoming search (one vs. two template features) and the number of features that actually needed to be selected from the search display to do the task (one vs. two target features). (a) illustrates the design. To test the limitations of template preparation, we then directly contrasted the spatiotemporal pattern of activity when participants looked out for one vs. two template features, but needed to select only one target feature from every search display (preparation cost). To measure the limitations of attentional selection, we compared conditions in which observers always needed to prepare two templates, but during search, either only one, or both target features needed to be extracted (selection cost). The results (see (b,c)) showed that multiple template preparation led to a relatively small time cost, in that selection started overall 21 milliseconds later when multiple templates had to be prepared relative to when only one template needed to be prepared, but then, evidence for the target accumulated equally strong over time in both conditions. In contrast, actually having to select multiple different targets was associated with a much more severe cost, with target identification proceeding at a much slower rate. Another way of describing these results is that observers could efficiently select either of the two target features, but not both. These findings indicate that the process of preparing top-down biases has a higher capacity than the process of using those biases to select matching information from the visual input (see Töllner et al., Citation2016 for corroborating findings in enumeration tasks).
Figure 3. Dissociating template preparation versus selection using EEG. (a) Design. In all conditions, observers were required to select two target characters and determine whether they were of the same (i.e., both letters or both digits) or different category (i.e., letter and digit). Depending on the condition, either one or two colours were cued to be task-relevant in the beginning of a block (thus creating one vs. two unique templates). In addition, whenever two colours were cued, targets in the search displays would be of one or of two colours (thus one vs. two unique target features had to be selected). Consequently, in the one-template-one-target-feature condition (1TMP–1TGT) one colour was cued, and both targets carried this colour in the search display, in the two-templates-one-target-feature condition (2TMP–1TGT) two colours were cued but only one of these colours was present in the search display with both targets carrying that colour, and in the two-template-two-target-feature condition (2TMP–2TGT) two colours were cued and both colours were present in the search displays. One target always appeared on the horizontal meridian (above or below fixation), and the other target on the vertical meridian (to the left or right of fixation). (b) and (c) MVPA decoding performance for target position. (b) Decoding performance comparing the 1TMP–1TGT and 2TMP–1TGT conditions, with the difference score, thus showing the effect of the number of templates – a minor preparation cost. (c) The same, now comparing the 2TMP–1TGT and 2TMP–2TGT conditions, thus showing the effect of the number of to-be-selected features within the display – a severe selection cost. For further details see Ort, Fahrenfort, ten Cate, et al. (Citation2019).
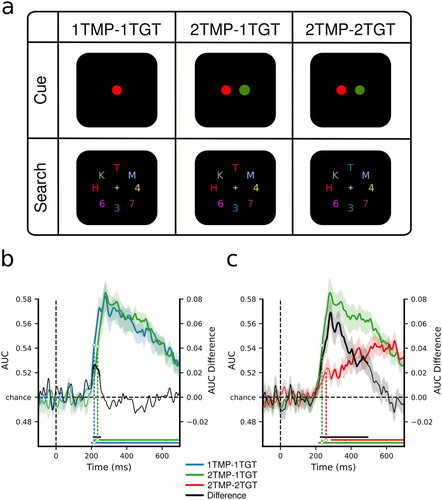
Furthermore, the design of our study allowed for additional analyses investigating whether the selection cost was due to limited parallel or limited serial processing. To do so, we correlated the decoding strength for the locations of both targets in the display across individual time points within each trial, and examined whether the decodability of one target was positively, negatively or not at all related to the other target. A negative correlation would imply that when attention was on one target, the other target automatically was not attended, and vice versa. However, we did not find any correlation, suggesting no systematic serial switching of attention between targets. Instead this finding might be taken as further evidence that the capacity limitation of MTS is caused by limited parallel processing.
Note that the relatively minor preparation cost suggests that observers were eventually prepared to search for either of the two targets, well in time for the search display onset. However, whether the process of becoming prepared is accomplished in a parallel or a serial fashion, remains an open question. That is, when observers are being given two target cues (or instructions), encoding of those cues may well be serial, as may their conversion into useful attentional templates (de Vries et al., Citation2017).
Based on these findings, we proposed a model of how multiple biases play out at the preparation and selection stages of MTS. illustrates this model. Specifically, we propose that multiple templates can simultaneously be in an activated state in memory, which then interact with the incoming signals. Importantly, these templates stand in a competitive relationship with each other (Manohar et al., Citation2019), which will have an adverse effect compared to when only one template is active. However, the severity of this adverse effect depends on what will be present in the outside world. When visual input enters the system (search display onset), the top-down biases and any matching bottom-up visual input will be combined and multiplexed into a recurrent feedback loop, enhancing regions that contain template-matching information. If only one target category needs to be selected from the display, there is essentially no competition for selection, as this target category will trigger the accompanying template, at the expense of the other template (not triggered). This template (or rather the template-based feedback loop) will therefore rapidly win the competition, albeit at a small delay relative to when only a single template is active. However, when the visual input contains multiple target categories in different locations, multiple templates are being triggered. Now the mutually suppressive relationship between templates will become detrimental with weakened recurrent activity at each of the target locations as a consequence. Evidence for target presence will therefore accumulate more slowly for both targets, resulting in less efficient selection. This entire process can run in parallel, but is severely limited.
Figure 4. Current model of multiple-target search. Preparation. When set out to look for two targets, multiple target representations can be prepared and kept in a ready-to-use state. However, these representations stand in a competitive relationship to each other (represented through round arrowheads), responsible for a minor cost that arises at this stage. Selection. During search, these top-down target biases interact with matching bottom-up signals factors and trigger recurrent feedback loops that cause both target features to be concurrently enhanced, leading to selection. However, at this stage, when both targets are present in the display, the mutual inhibitory relationship between the target representations becomes detrimental and severely impairs selection, representing the major bottleneck of multiple-target search. Post-selection processing. Once potentially task-relevant information has been selected, individuals need to verify whether it is indeed the sought-for information. Its limitations are currently unknown for VWM based search tasks.
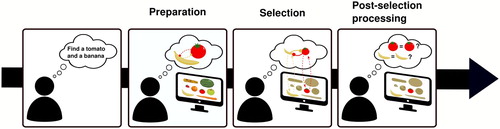
Redundancy gains from multiple target features
Further support for the idea that observers may be able to prepare search for multiple target features in parallel comes from a study by Bahle et al. (Citation2019, see also (b) here). They asked observers to look for two different target features, for example, both a colour and a shape on every trial. The search display would then contain a target that would unpredictably carry either one of those features, or both. Bahle et al. found that responses to a target that matched both features benefited from a redundancy gain, in that RTs were faster than when the target matched either feature alone. This result per se is no evidence for a parallel model, since a serial model would also predict worse performance for targets defined by a single feature. The crucial result here lies in the RT distributions, which showed that the redundancy gain for two matching target features exceeded what would be predicted on the basis of a simple summation model in which either feature independently races for selection (cf. Andersen et al., Citation2015). Such race model inequality violations provide evidence for a nonlinear co-activation of two processes (Miller, Citation1982; Mordkoff & Yantis, Citation1991; Townsend & Nozawa, Citation1995), here presumably the convergence of two top-down feature biases at a single target location. Crucially, such an interaction between two feature biases requires those biases to occur in parallel.Footnote6
Unfortunately, there is no direct evidence as to what is the source of the redundancy gain. The behavioural evidence from manual RTs does not provide direct insight as to which stage is aided. There are a couple of EEG studies that tried to disentangle pre- from post-selection stages, using the N2pc as a marker of selection (Grubert et al., Citation2011; Töllner et al., Citation2011). These studies found that the N2pc was speeded for redundant targets, suggesting a pre-selective component. However, these studies have solely used pop-out displays, in which every target stood out by a salient feature, and thus selection may have been driven by redundancy gains in saliency signals rather than top-down biases (Krummenacher et al., Citation2001; Töllner et al., Citation2011). Moreover, whereas in these studies the behavioural responses again showed evidence for race model inequality violations, a mere redundancy gain on the N2pc per se is no evidence that this violation originated at the pre-selection stage.
Finally, one more finding is relevant to mention in this context: In one condition, Bahle et al. (Citation2019, Experiment 1b) presented two separate targets in the search display, one carrying one target feature (e.g., the sought-for colour), the other carrying the other feature (e.g., the sought-for shape), and the observer was instructed to respond to either. Here too there was a redundancy gain compared to the single target conditions, as would be expected under any model, parallel or serial. Interestingly though, when looking at the RT distributions, this time search times were slower than would be expected on the basis of two independent parallel races. In other words, as in the EEG study of Ort, Fahrenfort, ten Cate, et al. (Citation2019), here too it appeared that two targets directly competed with each other for selection, supporting the limited capacity parallel model, proposed in .
Combined visual search and memory search
Another line of research has found that the costs of looking for multiple targets can be remarkably small, even when observers are asked to look for tens of different target objects. In a series of studies tapping into LTM as well as visual search (so-called hybrid search), it has been shown that with sufficient memory training observers can learn to look for up to at least 100 objects (Drew et al., Citation2017; Drew & Wolfe, Citation2014; Madrid & Hout, Citation2019; Wolfe, Citation2012; Wolfe et al., Citation2016), suggesting that the capacity of MTS might be much larger than previous research predicted. Importantly, Wolfe (Citation2012) showed that while the costs of additional distractor items presented in the display scaled linearly, the costs of having to look for more targets – that is, of having to store multiple items in LTM – grew only logarithmically. Wolfe concluded that while observers search through the display serially, search through memory occurs at least partially in parallel.
An important question is how such an LTM-based search compares to the flexible, goal-driven search that is facilitated by VWM (Drew et al., Citation2016). Hallmark of flexible, top-down templates in VWM is that they (a) result in active guidance (i.e., relevant features in the display are being enhanced and attract attention), and (b) are selective (i.e., they enhance some properties, but not others). LTM-based search may not meet these properties. To start with the latter, selectivity, it seems unlikely that observers, after memorizing tens of target objects, will be able to look for a random subset of those objects, and ignore the others (cf. Boettcher et al., Citation2018). A recent study provided some evidence for set selectivity in LTM-based search (Giammarco et al., Citation2016). However, the two sets were also studied separately and, after the memorization phase, participants were explicitly instructed to only search for members from one set and ignore the other. Thus, this study tested separation by fixed learning context rather than by flexibly changing task relevance.
But it is even questionable whether in LTM-based search attention is actively and selectively being guided towards potentially task-relevant objects on the basis of the memorized objects. Drew et al. (Citation2017) found reduced guidance (expressed not only in the “serial” display size effects but also in increased numbers of fixations on distractors) the more targets were to be remembered. Furthermore, Madrid and Hout (Citation2019) actually found LTM-based search to become worse when observers adopted an active search strategy, compared to passive scanning. Instead, LTM-based search may be better described as a recognition process rather than a search process, in the sense that observers scan the display object-by-object in a largely unguided fashion and then search their memory instead (Drew et al., Citation2017). If so, then any LTM-based limitations are likely to play out at the final, post-selection identification stage of the search process, rather than during search itself.
Switch costs
It is a well-established finding that performance suffers when tasks change from one trial to the next (e.g., Meiran, Citation2010; Monsell, Citation2003; Vandierendonck et al., Citation2010; Wylie & Allport, Citation2000). The presence of a switch cost can be considered evidence in favour of a serial component to processing because if two mental operations can be fully performed in parallel, there should not be a switch in the first place. A similar rationale can be applied to visual search (see (c)). If observers have to look for multiple targets but only one target is randomly presented per trial, the emergence of switch costs would suggest that observers were not simultaneously prepared for both targets to an equal extent, while a reliable absence of switch costs would imply parallel MTS. Many studies have indeed reported switch costs for changing targets in visual search (e.g., Brascamp et al., Citation2011; Christie et al., Citation2015; Dombrowe et al., Citation2011; Found & Müller, Citation1996; Jóhannesson et al., Citation2016; Juola et al., Citation2004; Á. Kristjánsson et al., Citation2020; Á. Kristjánsson & Campana, Citation2010; T. Kristjánsson et al., Citation2018; Kruijne & Meeter, Citation2015; Müller et al., Citation1995; Olivers & Humphreys, Citation2003; Olivers & Meeter, Citation2006; Ort et al., Citation2017; Ort et al., Citation2018; Ort, Fahrenfort, Reeder, et al., Citation2019; van Driel et al., Citation2019; Wolfe et al., Citation2003), which would be consistent with a serial component at some processing stage.
Nevertheless, even though the existence of switch costs is in itself rather uncontroversial, some authors have been hesitant to interpret such costs as evidence for limited serial processing, on the basis that the magnitude of the costs tends to be too small to represent the full switching of a template (Grubert & Eimer, Citation2015a; T. Kristjánsson & Kristjánsson, Citation2018; T. Kristjánsson et al., Citation2018). While switch costs typically are in the order of a few tens of milliseconds, the time to set up a template is commonly assumed to be an order of magnitude larger, in the range of 200–300 ms (Dombrowe et al., Citation2011; Wolfe et al., Citation2004). However, these latter estimates may be exaggerated: In Dombrowe et al. (Citation2011) participants not only needed to establish a new template, but also to inhibit the previous target as that one would occur in the next search display as a distractor. In Wolfe et al. (Citation2004) participants always had to look for specific conjunction-based targets, which is arguably a more demanding process than switching between simple features. Furthermore, in a recent fMRI study, we demonstrated that a target switch during visual search activates the regions of the same cognitive control network as is usually reported in task switching, despite the actual switch cost being “only” 66 ms (Ort, Fahrenfort, Reeder, et al., Citation2019). Similar results have been obtained with EEG (Grubert & Eimer, Citation2015b; Jenkins et al., Citation2016). It may thus be premature to downplay the significance of switch costs solely based on their small magnitude.
But even though small switch costs can be explained by a serial model, they are certainly not conclusive evidence for it. One concern about the interpretation of switch costs is that they are strongly influenced by implicit biases based on selection history. The intertrial priming literature has shown that observers have a robust bias to select the same visual features that they have selected before (Hillstrom, Citation2000; Á. Kristjánsson & Campana, Citation2010; Maljkovic & Nakayama, Citation1994). In particular, selection times become faster the more often a target feature is repeated. Therefore, what appears to be a switch cost as caused by setting up a new template might actually be a repetition benefit, caused by repeatedly selecting the same target. Importantly, if this were true, a parallel model (irrespective of capacity) might also account for the data. Specifically, two templates could be active in parallel and provide similar top-down guidance, however, selection history might selectively enhance one of the targets as it was recently selected more often, so that the total bias toward that target is stronger relative to the non-repeated target. The resulting cost/benefit in the behaviour would then merely represent the contribution of learned biases and not be related to top-down templates, and thus switch costs would not even be an indicator for the existence of a capacity limitation of MTS.
Note also that there are conditions under which little to no measurable switch costs occur. Beck et al. (Citation2012; see also Beck & Hollingworth, Citation2017) asked observers to look for either one of two target features. They constructed search displays in which always both target features were present, and thus observers could select either. Observers readily switched from one target feature to the next, either within displays (Beck et al., Citation2012) or between displays (Beck & Hollingworth, Citation2017). The researchers measured saccadic latency, and found no evidence for switch costs, which would be consistent with the parallel activation of multiple target templates. However, just like the presence of switch costs does not provide conclusive evidence for serial processing, nor does the absence of switch costs provide conclusive evidence for parallel processing. Ort et al. (Citation2017) have argued that in the scenario when both targets are always available in the display, observers can proactively switch templates before the next search display, since whatever target they switch to, it will always be there. This allows switch costs to become latent, disappearing in the time before display onset. In the more classic case, only one of the two targets is available in each display, and the observer cannot predict which. In that case, any switching has to occur reactively, after display onset, and will be expressed in behaviour. In other words, the emergence of switch costs may depend on the control that observers can exert over target selection. Indeed, Ort et al. (Citation2017, Citation2018) have demonstrated that behavioural switch costs depend on target availability, and additional neurophysiological evidence indicates that observers may approach trials where they have a choice differently from trials where they have no choice (Ort, Fahrenfort, Reeder, et al., Citation2019; van Driel et al., Citation2019).
Apart from switch costs, switch rates have also been used to make inferences about the underlying architecture of MTS (Beck et al., Citation2012; Jóhannesson et al., Citation2016; Á. Kristjánsson et al., Citation2014; T. Kristjánsson & Kristjánsson, Citation2018; T. Kristjánsson et al., Citation2018). The switch rate as a measure for parallel vs. serial processing has primarily been used in foraging paradigms in which individuals were given multiple target categories to look for on each trial, and then scanned cluttered search displays containing many instances of each of those target categories (see (d)). In doing so, participants can freely decide in which order they select the available targets, and the switch rate represents how frequently participants change from selecting one target category over the other. Frequent, randomly distributed switches are commonly interpreted as evidence for parallel strategies, whereas runs of repetitions provide evidence for serial strategies. Interestingly, several studies have found high switch rates for simple feature search (Beck et al., Citation2012; T. Kristjánsson & Kristjánsson, Citation2018; T. Kristjánsson et al., Citation2018; T. Kristjánsson et al., Citation2020). Furthermore, the switching has been found to be close to random, largely following the random structure of the search displays. This suggests that the switching is to a great extent driven by the input rather than some active controlled switching on behalf of the observer, consistent with parallel MTS (though see Ort, Fahrenfort, Reeder, et al., Citation2019; and van Driel et al., Citation2019 for evidence for active switching when multiple single-feature-defined targets are available in the display). Interestingly, when searching for a conjunction-defined target, switch rates are much reduced, as observers stick to a particular target for longer runs. This would suggest that observers can maintain multiple feature templates, but not multiple conjunction templates in parallel. However, as T. Kristjánsson et al. (Citation2018) also argued, this may be a matter of strategy rather than real limitation. Moreover, stimulus displays in conjunction search and in feature search are by definition different, which may also affect the efficiency of selection (see Footnote 6). Finally, even though observer may frequently switch in foraging, this still often comes with switch costs, even for simple features (T. Kristjánsson & Kristjánsson, Citation2018; T. Kristjánsson et al., Citation2018, Citation2020). Although these are again in the range of maximally a few tens of milliseconds, without knowing the real underlying costs of a serial switch process, it remains difficult to fully exclude a serial component.
Finally, it is not always clear at which processing stage the switch costs in MTS play out, as costs may occur at template preparation, selection, or post-selection decision stages (Becker, Citation2008b; Hillstrom, Citation2000; Huang et al., Citation2004; Á. Kristjánsson & Campana, Citation2010; Meeter & Olivers, Citation2006; Olivers & Meeter, Citation2006). Again, oculomotor measures are more revealing in this respect. Studies using oculomotor measures have shown that switch costs can already be found for the latency of the first saccade (Becker, Citation2008a, Citation2008b; Ort et al., Citation2017), suggesting a pre-selection origin. In addition, EEG measures of attention also indicate that at least some of the switch costs originated prior to selection (Christie et al., Citation2015; Grubert & Eimer, Citation2013, Citation2015a; Ort, Fahrenfort, ten Cate, et al., Citation2019; Töllner et al., Citation2008). But also here it is unclear whether this effect reflects a switch cost or a repetition benefit.
All in all then, there is little doubt that performance on target switches is worse than on target repeats during MTS. Unfortunately, it has proven far from trivial to experimentally differentiate serial from weighted-parallel models, and to our knowledge there is as yet no conclusive evidence what these costs exactly represent and what they tell us about the capacity of MTS is therefore still unclear.
Memory-based attentional capture by task-irrelevant features
The costs of MTS are usually assessed based on tasks in which multiple target representations are directly task-relevant. In another line of research, paradigms have been used in which the behavioural effects of memory representations are decoupled from their behavioural relevance (Olivers, Citation2009; Olivers et al., Citation2006; Soto et al., Citation2005; Soto et al., Citation2008). In this type of paradigm, individuals are given an item to memorize for an upcoming memory test (see (e)). Prior to the memory test, though, they first perform a search task in which they need to find an unrelated target item. At the same time, memory-matching items may also be present in the search display, typically as a distractor. This paradigm has the advantage that it allows researchers to investigate the automaticity of memory-attention interactions. Indeed, although the memorized item is entirely irrelevant for the search, observers’ attention has been found to be captured more by search items that match the memory than by search items that are unrelated to the memorized content. Thus, in a way an active visual working memory representation appears to automatically act like a top-down attentional template, which is consistent with the biased competition and sensory recruitment accounts mentioned in the section on theoretical issues (Desimone & Duncan, Citation1995; Serences, Citation2016). Furthermore, oculomotor and electrophysiological studies have shown that such capture arise at early stages of search (Hollingworth et al., Citation2013; Kumar et al., Citation2009; Liesefeld et al., Citation2017; Mannan et al., Citation2010; Olivers et al., Citation2006; Soto et al., Citation2006).
Unfortunately, with regards to the question how many VWM representations can bias attention, the results are mixed. Some studies have shown that capture disappears when more memory items are added (Beck & Vickery, Citation2019; Downing & Dodds, Citation2004; Frătescu et al., Citation2019; Houtkamp & Roelfsema, Citation2006; Soto et al., Citation2012; van Moorselaar et al., Citation2014; Williams et al., Citation2019), whereas others found capture also for two memorized items (Bahle et al., Citation2018; Chen & Du, Citation2017; Fan et al., Citation2019; Frătescu et al., Citation2019; Hollingworth & Beck, Citation2016; Soto & Humphreys, Citation2008; Zhang et al., Citation2011; Zhang et al., Citation2018). So far, only a handful of studies have tested more than two memory items and they found capture to be absent (Soto et al., Citation2012; van Moorselaar et al., Citation2014; Zhang et al., Citation2011). Also, one should keep in mind that in these studies participants were never supposed to actively look for the memorized objects in the search displays, as they would only be interfering with search. There was thus an incentive to shield the memorized representations from influencing attention, which may have contributed to the observed limitations.
One obvious factor that might determine how many items capture attention could be the VWM capacity of individuals (Cowan, Citation2001; Luck & Vogel, Citation1997). For example, if observers can only memorize one or two objects reliably, they are less likely to show capture by two memory representations. Likewise, individuals might show capture by two or more items if their VWM capacity is large. However, van Moorselaar et al. (Citation2014) presented evidence against this, as they found no systematic relationship between VWM capacity and the magnitude of attentional capture. Instead what seems to be an important factor in causing capture to be present or not when two items are memorized, is whether only one, or both of those items actually appear in the search display. Three studies have now found that capture occurs when both items return in the search display (Chen & Du, Citation2017; Frătescu et al., Citation2019; Hollingworth & Beck, Citation2016). However, it is unclear what this means for the underlying architecture of visual search. The data is consistent with a parallel model, but one could equally argue that with two items present, the chance of one of them matching an active template increases, consistent with a limited serial attentional bias (for a similar argument see Williams et al., Citation2019; though see Zhou et al., Citation2019). Further research is necessary here.
Attentional capture by task-relevant features (contingent capture)
A similar but separate line of research has focused on inadvertent capture effects when the distractors actually match the currently relevant target-defining features – called contingent capture (Folk et al., Citation1992; Wyble et al., Citation2013). In these studies, participants are instructed with the target-defining feature for an upcoming search task, but shortly before the target display appears, a cue display is presented, containing a singleton distractor. The typical finding is that the distractor captures attention more strongly when it matches the current template than when it does not, as has been demonstrated in behaviour (Ansorge et al., Citation2005; Ansorge & Horstmann, Citation2007; Folk et al., Citation1992; Folk et al., Citation2002; Folk & Anderson, Citation2010), as well as in saccadic latencies (Wu & Remington, Citation2003), and the N2pc in EEG (Brisson et al., Citation2009; Eimer & Kiss, Citation2008, Citation2010; Kiss et al., Citation2008; Leblanc et al., Citation2008; Wu & Fu, Citation2017). This indicates that its origin must at least partly be at an early stage of search, either during or prior to selection.
A number of studies have used the contingent capture paradigm to investigate if multiple templates (or attentional control settings as they are often referred to in this literature) can capture attention. The majority of studies have reported capture to still occur when observers are instructed to search for more than one target feature (Adamo, Wozny, et al., Citation2010; Becker et al., Citation2015; Giammarco et al., Citation2016; Grubert & Eimer, Citation2016; Irons et al., Citation2012; Irons & Remington, Citation2013; Ito & Kawahara, Citation2016; Kerzel & Witzel, Citation2019; Parrott et al., Citation2010; Roper & Vecera, Citation2012; Thigpen et al., Citation2019), and some research has even suggested that different templates can be applied to different spatial locations (Adamo et al., Citation2008; Adamo, Pun, et al., Citation2010; Cho & Cho, Citation2018). At the same time, contingent capture has been shown to be reduced quite systematically for multiple targets (Biderman et al., Citation2017; Folk & Anderson, Citation2010; Lagroix et al., Citation2018; Moore & Weissman, Citation2010, Citation2011), which suggests that contingent capture is subject to capacity limitations. However, as contingent capture measures too rely on trial averaging, they do not reveal the nature of these limitations, as they could be caused by serial or limited parallel processes.
Summary, conclusions and future directions
In recent years, the capacity of MTS has received considerable attention. However, much of the research has treated MTS as a unitary concept without examining its components in isolation. The fact that different processes have been lumped under the umbrella term of MTS might explain some of the contradictory findings in the literature. Here, we tried to construct an overview of the existing evidence and systematically link it to various aspects of the question “Can individuals look for multiple target at the same time?”. As is not atypical for cognition research, the question has been approached vigorously, with many different paradigms, but progress has remained largely limited to demonstrating costs (or not), and questions about exact mechanisms so far remain largely unanswered. This has resulted in a situation where there is a lot, but at the same time there is also rather little. In this section, we summarize the evidence as we presented it, identify remaining issues in the field and propose a number of open questions that we hope will be investigated in the future.
In the outset of this paper, we broke down MTS in its components: capacity (unlimited vs. VWM-limited vs. limited beyond VWM), processing architecture (serial vs. parallel), and search stage (preparation vs. selection vs. post-selection processing). After considering all the evidence it has become apparent that different limitations and processing architectures apply to different stages of search, emphasizing the multi-faceted character of MTS. Here, we list the key findings of this review:
More than one target-defining feature can simultaneously bias attention a priori, but at a cost. First, most research suggests that multiple top-down feature biases can be held in a ready-to-use state, essentially preparing the visual system to process any of multiple potential target features. This is not to mean that such preparation is unlimited. For flexible, VWM-based search, the first limitation is imposed by VWM capacity. But even within that limit, small yet reliable costs arise already when individuals try to look for two target objects at the same time, with increasing costs for each additional target to the extent that there is currently little evidence for MTS beyond two targets (Drew et al., Citation2017; Soto et al., Citation2012; van Moorselaar et al., Citation2014; Zhang et al., Citation2011). These findings are in line with a parallel processing system which is more limited than VWM itself (Olivers et al., Citation2011). Alternatively, dependent measures have so far not been sensitive enough to register additional biases. Furthermore, most research has evaluated the maintenance of a prepared state in which multiple templates can be active, but not the process of setting up those templates, a distinction that needs further research (see open questions below).
The number of features that can be selected from a display is strongly limited, presumably by impaired parallel processing. Probably the bulk of research on MTS has focused on how top-down biases interact with visual input by evaluating which features in the display are preferentially processed. However, most of these studies investigated scenarios with only one potential target per stimulus display, limiting conclusions on the limits of the selection process itself. The handful of studies that have looked into this indicate that the number of target features that can simultaneously be selected seems to be strongly limited, reflecting the true bottleneck of multiple-target search. Interestingly, what may be the limiting factor is the spatial separation of those different features: When different target features need to be selected from different locations or objects, selection efficiency decreases substantially, whereas when different target features need to be selected from the same location or object, search efficiency may actually receive a boost (Andersen et al., Citation2015; Bahle et al., Citation2019; Duncan, Citation1984). This implies that engaging in multiple, spatially separate, recurrent feedback loops may be the real multiple-target bottleneck. Finally, whether this bottleneck represents a serial or a parallel limitation has not conclusively been resolved yet, but so far the lack of any positive evidence for serial processing points towards a limited parallel model as the more likely candidate (Andersen et al., Citation2008; Bahle et al., Citation2019; Ort, Fahrenfort, ten Cate, et al., Citation2019).
Capacity and processing architecture of post-selection processes remains unknown. Finally, there has not been much research on the capacity of post-selection processing in the context of MTS. This has at least two implications. First, what researchers may believe reflects restrictions in (pre-)selection processes may actually reflect post-selection processes. Especially the use of relatively slow measures (manual RTs, fMRI) is vulnerable to this. Second, claims about post-selection capacity limitations must remain speculative at this point. On the one hand, as the number of features that can be selected at any one time is strongly limited, it stands to reason that post-selection processing is subject to the same limitations. Indeed, a few reports suggest that post-selection processing is also subject to costs (e.g., Barrett & Zobay, Citation2019; Hout & Goldinger, Citation2015). On the other hand, LTM-based recognition, as one exemplar of post-selection processing, has been shown to occur essentially in parallel, only limited by LTM capacityFootnote7 as long as people are sufficiently familiar with the objects (e.g., Wolfe, Citation2012). Therefore, this stage might in essence be unlimited. Needless to say, more research is necessary to better understand the capacity of post-selective object recognition processes in MTS.
Based on the above, we believe examining the following questions would greatly further our understanding of MTS:
Can multiple top-down biases be simultaneously set up? It has been shown that the visual system can be simultaneously biased towards multiple features. However, it has not been examined yet whether these top-down biases are initiated simultaneously or sequentially, after instruction or cues. Setting up simultaneous biases is not the same as simultaneously setting up biases. It is likely that this will require effortful cognitive operations, but that has as yet to be demonstrated.
What about more than two? Only a few studies have been conducted with more than two target representations. It would be interesting to examine whether attentional guidance parametrically diminshes with the number of templates or whether there will be a sudden drop if the number of templates exceeds two.
Do intertrial effects reflect template switching or a repetition benefit? Switch costs are used to argue for seriality of attentional guidance. However, similar effects would be caused by other task-unrelated biases (i.e., selection history). Understanding the source of switch costs is critical for evaluating the relevance of a large number of MTS studies.
Is the limitation of selection due to a serial or a parallel bottleneck? Even though we have not found strong indications of a serial bottleneck of selection of multiple targets, this essentially represents a null result. Therefore it is possible that with more power or more sensitive methods, such a serial limitation will be observed. Future research should investigate the source of the limit of MTS selection.
What are the limitations of post-selection processing? Hardly any research has been conducted on the number of templates that can concurrently be used in post-selection stages to check that the sought-for target has indeed been found. The findings in the context of hybrid search hint at a flexible system in which rather than memory-guided visual search, people may perform a vision-guided memory search, with search only being limited by LTM capacity (Drew et al., Citation2017). A major challenge in investigating this question will be to tease apart effects on selection from those on post-selection processing as they are intricately linked with each other.
Is there a fundamental difference between looking for any of multiple targets (an OR operation) and looking for all of them (an AND operation)? In many real-world search situations it is not clear how many targets are present. Therefore, it would be imprudent to limit the research on MTS to the scenario when only one target appeared in the search. Foraging studies (e.g., Á. Kristjánsson et al., Citation2014) and research on the satisfaction of search (e.g., Cain et al., Citation2013) might be important to study selection and recognition limitations for more than just two targets.
To what extent do the described findings depend on the nature of the search targets? Most research used simple features as the target-defining property, with colour being overrepresented relative to other features. However, real-world objects are usually defined by multiple features, or even integrated objects that cannot easily be decomposed into components (e.g., faces). Therefore, for the sake of external validity it is mandatory to investigate to what extent the findings generalize to more complex stimuli. So far, existing research on that topic has led to mixed findings (Andersen et al., Citation2015; Bahle et al., Citation2019; Biderman et al., Citation2017; Á. Kristjánsson et al., Citation2020; McCants et al., Citation2018; Ort et al., Citation2018; Wolfe et al., Citation1990).
What are the contributions of different memory systems to the capacity of MTS? It is commonly assumed that search targets are stored in VWM, which is therefore the source for template-based visual search. However, it has also been shown that very similar attentional effects are observed when the same target objects are used repeatedly, even when they were “outsourced” to long-term memory (Grubert et al., Citation2016). Given that long-term memory has arguably a larger capacity than VWM, MTS for many over-learned targets should, by implication, be possible. Alternatively, regardless of the memory system in which the content of a top-down bias originates, its exerting effects may be limited by mechanisms implementing, maintaining, or executing current goals.
What is the relationship of feature-based attention and spatial attention during MTS? The capacity of MTS starts as a problem of feature-based attention (number of co-existing feature-based biases), but as soon as an individual has to interact with the visual environment spatial attention also becomes relevant (number of locations that can be attended). Therefore, it is not necessarily clear whether the limitations arise due to spatial (e.g., there is a unitary attentional window), due to feature-based limitations (e.g., only one feature can be extracted at a time), or a combination of both. Furthermore, it is also still an open question whether multiple features can bias attention in a location-specific way (e.g., see Adamo et al., Citation2008; Irons & Remington, Citation2013).
What is the neurophysiological substrate of establishing and maintaining a top-down bias? As already mentioned, it is largely unknown how multiple top-down biases are established. To truly understand MTS, a mechanistic model of how top-down biases are established, maintained and applied during search will have to be formulated. One candidate mechanism by which multiple top-down biases could be established and maintained is through repeated refreshing signals from the prefrontal cortex, akin to the idea of refreshing of working memory as executive function (Lemaire et al., Citation2018; Raye et al., Citation2007) and rhythmic attentional sampling (Busch et al., Citation2009; Re et al., Citation2019; VanRullen, Citation2016). In this sense, if not repeated regularly, a top-down bias continuously fades and loses its influence on search, so that effectively the rate at which top-down biases can be refreshed would represent a limiting factor of MTS.
To conclude, can individuals look for multiple target at the same time? Without taking a more nuanced perspective to this question, a positive answer can only be given if processing at each and every stage of visual search evolves in parallel for at least two different targets. The here reviewed evidence suggests that at least one of the stages – selection – is strongly limited, while preparation of multiple top-down biases in anticipation of a search is possible with relatively small costs. At the same time, there is not sufficient information to make warranted conclusions about capacity limitations of post-selection processing. What is clear is that empirically, MTS is slower and more error-prone than single-target search. This should be taken into consideration when designing real-life search tasks (e.g., airport security scanners), for example by limiting the number of targets an individual is looking for at any moment. In any case, even though we cannot provide a conclusive model of MTS here, we hope that our taxonomy provides a useful framework which can serve as a starting point for future research.
Disclosure statement
No potential conflict of interest was reported by the author(s).
Additional information
Funding
Notes
1 This is not to say that it covers all aspects of visual search, which is one of the vastest topics of investigation within attention research. Rather, it covers what are in our view the most prominent mechanisms in light of the multiple target search debate.
2 In fact, Eimer (Citation2014) refers to this guidance as a separate stage, which triggers another process, spatial attention, which in turn further enhances the local representation (which Eimer refers to as selection). However, we prefer to see guidance as an emergent property of the competition for selection: Winning representations are by definition enhanced, including their locations or regions, which is then expressed as spatial guidance.
3 For the sake of conciseness, we will use the term “model”, even though what we mean would be better described as “classes of models”. Specifically, we do not refer to specific models or imply a specific implementations, but all potential models that have a specific features (e.g. “a parallel model with limited capacity” refers to all hypothetical models that include elements of parallel processing and are of limited capacity).
4 There are unlimited, parallel models of how information is extracted from the visual input (Eckstein et al., Citation2000; Palmer et al., Citation2000). However, these models are not directly related to the question of MTS capacity, which is about the capacity of the top-down attentional set.
5 A different form of MTS deals with so-called subsequent search misses. These occur when on rare trials more than one target is present in a search display and participants overlook these additional targets once they have found the first target, even when all targets are the same (Adamo et al., Citation2013; Cain et al., Citation2013; Cain et al., Citation2012; Cain & Mitroff, Citation2013). The misses have been primarily attributed to suboptimal search termination (i.e. observers are “satisfied” with having found one target). Nevertheless, there is also evidence of a capacity limitation at play, which may be attributed to the post-selection processing stage, dealing with target identification. For example, Adamo et al. (Citation2013) demonstrated that observers were most likely to miss the second target when they fixated it shortly after the first target, similar to an attentional blink (Raymond et al., Citation1992).
6 Note that in the classic conjunction search scenario (Treisman & Gelade, Citation1980) the target is also defined by two features. Yet there, search is typically much less efficient than the single feature search conditions it is typically compared to. However, this difference is confounded by the fact that conjunction search displays are commonly much more heterogeneous than single feature search displays (Duncan & Humphreys, Citation1989; Quinlan & Humphreys, Citation1987 for an exception). Furthermore, conjunction displays by definition also include distractors that partially match the target template, which is likely to feed into the competition for selection. Classic conjunction search is therefore not that informative with respect to MTS. The Bahle et al. (Citation2019) design avoided these issues.
7 One might make the distinction between the capacity of LTM storage (a commodity that appears largely unlimited), and the capacity of LTM access (a process that appears somewhat limited), analogous to the distinction between remembering attentional templates and applying them to select task-relevant features.
References
- Adamo, S. H., Cain, M. S., & Mitroff, S. R. (2013). Self-induced attentional blink: A cause of errors in multiple-target search. Psychological Science, 24(12), 2569–2574. https://doi.org/10.1177/0956797613497970
- Adamo, M., Pun, C., & Ferber, S. (2010). Multiple attentional control settings influence late attentional selection but do not provide an early attentional filter. Cognitive Neuroscience, 1(2), 102–110. https://doi.org/10.1080/17588921003646149
- Adamo, M., Pun, C., Pratt, J., & Ferber, S. (2008). Your divided attention, please! The maintenance of multiple attentional control sets over distinct regions in space. Cognition, 107(1), 295–303. https://doi.org/10.1016/j.cognition.2007.07.003
- Adamo, M., Wozny, S., Pratt, J., & Ferber, S. (2010). Parallel, independent attentional control settings for colors and shapes. Attention, Perception & Psychophysics, 72(7), 1730–1735. https://doi.org/10.3758/APP.72.7.1730
- Andersen, S. K., Fuchs, S., & Müller, M. M. (2011). Effects of feature-selective and spatial attention at different stages of visual processing. Journal of Cognitive Neuroscience, 23(1), 238–246. https://doi.org/10.1162/jocn.2009.21328
- Andersen, S. K., Hillyard, S. A., & Müller, M. M. (2008). Attention facilitates multiple stimulus features in parallel in human visual cortex. Current Biology, 18(13), 1006–1009. https://doi.org/10.1016/j.cub.2008.06.030
- Andersen, S. K., Hillyard, S. A., & Muller, M. M. (2013). Global facilitation of attended features is obligatory and restricts divided attention. Journal of Neuroscience, 33(46), 18200–18207. https://doi.org/10.1523/JNEUROSCI.1913-13.2013
- Andersen, S. K., Müller, M. M., & Hillyard, S. A. (2009). Color-selective attention need not be mediated by spatial attention. Journal of Vision, 9(6), 2–2. https://doi.org/10.1167/9.6.2
- Andersen, S. K., Muller, M. M., & Hillyard, S. A. (2015). Attentional selection of feature conjunctions is accomplished by parallel and independent selection of single features. Journal of Neuroscience, 35(27), 9912–9919. https://doi.org/10.1523/JNEUROSCI.5268-14.2015
- Ansorge, U., & Horstmann, G. (2007). Preemptive control of attentional capture by colour: Evidence from trial-by-trial analyses and orderings of onsets of capture effects in reaction time distributions. The Quarterly Journal of Experimental Psychology, 60(7), 952–975. https://doi.org/10.1080/17470210600822795
- Ansorge, U., Horstmann, G., & Carbone, E. (2005). Top-down contingent capture by color: Evidence from RT distribution analyses in a manual choice reaction task. Acta Psychologica, 120(3), 243–266. https://doi.org/10.1016/j.actpsy.2005.04.004
- Awh, E., Armstrong, K. M., & Moore, T. (2006). Visual and oculomotor selection: Links, causes and implications for spatial attention. Trends in Cognitive Sciences, 10(3), 124–130. https://doi.org/10.1016/j.tics.2006.01.001
- Awh, E., Belopolsky, A. V., & Theeuwes, J. (2012). Top-down versus bottom-up attentional control: A failed theoretical dichotomy. Trends in Cognitive Sciences, 16(8), 437–443. https://doi.org/10.1016/j.tics.2012.06.010
- Bahle, B., Beck, V. M., & Hollingworth, A. (2018). The architecture of interaction between visual working memory and visual attention. Journal of Experimental Psychology: Human Perception and Performance, 44(7), 992–1011. https://doi.org/10.1037/xhp0000509
- Bahle, B., Thayer, D. D., Mordkoff, J. T., & Hollingworth, A. (2019). The architecture of working memory: Features from multiple remembered objects produce parallel, coactive guidance of attention in visual search. Journal of Experimental Psychology: General, https://doi.org/10.1037/xge0000694
- Baluch, F., & Itti, L. (2011). Mechanisms of top-down attention. Trends in Neurosciences, 34(4), 210–224. https://doi.org/10.1016/j.tins.2011.02.003
- Barrett, D. J. K., & Zobay, O. (2014). Attentional control via parallel target-templates in dual-target search. PLoS ONE, 9(1), 1–9. https://doi.org/10.1371/journal.pone.0086848
- Barrett, D. J. K., & Zobay, O. (2019). Concurrent evaluation of independently cued features during perceptual decisions and saccadic targeting in visual search. Attention, Perception, & Psychophysics, https://doi.org/10.3758/s13414-019-01854-w
- Bays, P. M., & Husain, M. (2008). Dynamic shifts of limited working memory resources in human vision. Science, 321(5890), 851–854. https://doi.org/10.1126/science.1158023
- Beck, V. M., & Hollingworth, A. (2017). Competition in saccade target selection reveals attentional guidance by simultaneously active working memory representations. Journal of Experimental Psychology: Human Perception & Performance, 43(2), 225–230. https://doi.org/10.1037/xhp0000306
- Beck, V. M., Hollingworth, A., & Luck, S. J. (2012). Simultaneous control of attention by multiple working memory representations. Psychological Science, 23(8), 887–898. https://doi.org/10.1177/0956797612439068
- Beck, D. M., & Kastner, S. (2009). Top-down and bottom-up mechanisms in biasing competition in the human brain. Vision Research, 49(10), 1154–1165. https://doi.org/10.1016/j.visres.2008.07.012
- Beck, V. M., & Vickery, T. J. (2019). Multiple states in visual working memory: Evidence from oculomotor capture by memory-matching distractors. Psychonomic Bulletin & Review, 26(4), 1340–1346. https://doi.org/10.3758/s13423-019-01608-7
- Becker, S. I. (2008a). The mechanism of priming: Episodic retrieval or priming of pop-out? Acta Psychologica, 127(2), 324–339. https://doi.org/10.1016/j.actpsy.2007.07.005
- Becker, S. I. (2008b). The stage of priming: Are intertrial repetition effects attentional or decisional? Vision Research, 48(5), 664–684. https://doi.org/10.1016/j.visres.2007.10.025
- Becker, M. W., Ravizza, S. M., & Peltier, C. (2015). An inability to set independent attentional control settings by hemifield. Attention, Perception, & Psychophysics, 77(8), 2640–2652. https://doi.org/10.3758/s13414-015-0964-8
- Bichot, N. P., Heard, M. T., DeGennaro, E. M., & Desimone, R. (2015). A source for feature-based attention in the prefrontal cortex. Neuron, 88(4), 832–844. https://doi.org/10.1016/j.neuron.2015.10.001
- Biderman, D., Biderman, N., Zivony, A., & Lamy, D. (2017). Contingent capture is weakened in search for multiple features from different dimensions. Journal of Experimental Psychology: Human Perception and Performance, 43(12), 1974–1992. https://doi.org/10.1037/xhp0000422
- Boettcher, S. E. P., Drew, T., & Wolfe, J. M. (2018). Lost in the supermarket: Quantifying the cost of partitioning memory sets in hybrid search. Memory and Cognition, 46(1), 43–57. https://doi.org/10.3758/s13421-017-0744-x
- Brascamp, J. W., Blake, R., & Kristjánsson, Á. (2011). Deciding where to attend: Priming of pop-out drives target selection. Journal of Experimental Psychology: Human Perception and Performance, 37(6), 1700–1707. https://doi.org/10.1037/a0025636
- Brisson, B., Leblanc, É, & Jolicœur, P. (2009). Contingent capture of visual-spatial attention depends on capacity-limited central mechanisms: Evidence from human electrophysiology and the psychological refractory period. Biological Psychology, 80(2), 218–225. https://doi.org/10.1016/j.biopsycho.2008.10.001
- Bundesen, C. (1990). A theory of visual attention. Psychological Review, 97(4), 523–547. https://doi.org/10.1037/0033-295X.97.4.523
- Bundesen, C., Habekost, T., & Kyllingsbæk, S. (2005). A neural theory of visual attention: Bridging cognition and neurophysiology. Psychological Review, 112(2), 291–328. https://doi.org/10.1037/0033-295X.112.2.291
- Busch, N. A., Dubois, J., & VanRullen, R. (2009). The phase of ongoing EEG oscillations predicts visual perception. Journal of Neuroscience, 29(24), 7869–7876. https://doi.org/10.1523/JNEUROSCI.0113-09.2009
- Cain, M. S., Adamo, S. H., & Mitroff, S. R. (2013). A taxonomy of errors in multiple-target visual search. Visual Cognition, 21(7), 899–921. https://doi.org/10.1080/13506285.2013.843627
- Cain, M. S., & Mitroff, S. R. (2013). Memory for found targets interferes with subsequent performance in multiple-target visual search. Journal of Experimental Psychology: Human Perception and Performance, 39(5), 1398–1408. https://doi.org/10.1037/a0030726
- Cain, M. S., Vul, E., Clark, K., & Mitroff, S. R. (2012). A Bayesian optimal foraging model of human visual search. Psychological Science, 23(9), 1047–1054. https://doi.org/10.1177/0956797612440460
- Carlisle, N. B., Arita, J. T., Pardo, D., & Woodman, G. F. (2011). Attentional templates in visual working memory. The Journal of Neuroscience, 31(25), 9315–9322. https://doi.org/10.1523/JNEUROSCI.1097-11.2011
- Carlisle, N. B., & Woodman, G. F. (2011). Automatic and strategic effects in the guidance of attention by working memory representations. Acta Psychologica, 137(2), 217–225. https://doi.org/10.1016/j.actpsy.2010.06.012
- Cave, K. R., Menneer, T., Nomani, M. S., Stroud, M. J., & Donnelly, N. (2018). Dual target search is neither purely simultaneous nor purely successive. Quarterly Journal of Experimental Psychology, 71(1), 169–178. https://doi.org/10.1080/17470218.2017.1307425
- Chelazzi, L., Miller, E. K., Duncan, J., & Desimone, R. (1993). A neural basis for visual search in inferior temporal cortex. Nature, 363(6427), 345–347. https://doi.org/10.1038/363345a0
- Chen, Y., & Du, F. (2017). Two visual working memory representations simultaneously control attention. Scientific Reports, 7(1), 1–11. https://doi.org/10.1038/s41598-017-05865-1
- Cho, S. A., & Cho, Y. S. (2018). Multiple attentional control settings at distinct locations without the confounding of repetition priming. Attention, Perception, & Psychophysics, 80(7), 1718–1730. https://doi.org/10.3758/s13414-018-1549-0
- Christie, G. J., Livingstone, A. C., & McDonald, J. J. (2015). Searching for inefficiency in visual search. Journal of Cognitive Neuroscience, 27(1), 46–56. https://doi.org/10.1162/jocn_a_00716
- Corbetta, M., & Shulman, G. L. (2002). Control of goal-directed and stimulus-driven attention in the brain. Nature Reviews Neuroscience, 3(3), 201–215. https://doi.org/10.1038/nrn755
- Cowan, N. (2001). The magical number 4 in short-term memory: A reconsideration of mental storage capacity. The Behavioral and Brain Sciences, 24(1), 87–114; discussion 114–185. https://doi.org/10.1017/S0140525X01003922
- Desimone, R., & Duncan, J. (1995). Neural mechanisms of selective visual attention. Annual Review of Neuroscience, 18(1), 193–222. https://doi.org/10.1146/annurev.neuro.18.1.193
- Deubel, H., & Schneider, W. X. (1996). Saccade target selection and object recognition: Evidence for a common attentional mechanism. Vision Research, 36(12), 1827–1837. https://doi.org/10.1016/0042-6989(95)00294-4
- de Vries, I. E. J., van Driel, J., & Olivers, C. N. L. (2017). Posterior α EEG dynamics dissociate current from future goals in working memory-guided visual search. The Journal of Neuroscience, 37(6), 1591–1603. https://doi.org/10.1523/JNEUROSCI.2945-16.2016
- Dombrowe, I., Donk, M., & Olivers, C. N. L. (2011). The costs of switching attentional sets. Attention, Perception, & Psychophysics, 73(8), 2481–2488. https://doi.org/10.3758/s13414-011-0198-3
- Downing, P. E., & Dodds, C. M. (2004). Competition in visual working memory for control of search. Visual Cognition, 11(6), 689–703. https://doi.org/10.1080/13506280344000446
- Drew, T., Boettcher, S. E. P., & Wolfe, J. M. (2016). Searching while loaded: Visual working memory does not interfere with hybrid search efficiency but hybrid search uses working memory capacity. Psychonomic Bulletin & Review, 23(1), 201–212. https://doi.org/10.3758/s13423-015-0874-8
- Drew, T., Boettcher, S. E. P., & Wolfe, J. M. (2017). One visual search, many memory searches: An eye-tracking investigation of hybrid search. Journal of Vision, 17(11), 5–5. https://doi.org/10.1167/17.11.5
- Drew, T., & Wolfe, J. M. (2014). Hybrid search in the temporal domain: Evidence for rapid, serial logarithmic search through memory. Attention, Perception, & Psychophysics, 76(2), 296–303. https://doi.org/10.3758/s13414-013-0606-y
- Duncan, J. (1980). The locus of interference in the perception of simultaneous stimuli. Psychological Review, 87(3), 272–300. https://doi.org/10.1037/0033-295X.87.3.272
- Duncan, J. (1984). Selective attention and the organization of visual information. Journal of Experimental Psychology: General, 113(4), 501–517. https://doi.org/10.1037/0096-3445.113.4.501
- Duncan, J., & Humphreys, G. W. (1989). Visual search and stimulus similarity. Psychological Review, 96(3), 433–458. https://doi.org/10.1037/0033-295X.96.3.433
- D’Zmura, M. (1991). Color in visual search. Vision Research, 31(6), 951–966. https://doi.org/10.1016/0042-6989(91)90203-H
- Eckstein, M. P. (2011). Visual search: A retrospective. Journal of Vision, 11(5), 14–14. https://doi.org/10.1167/11.5.14
- Eckstein, M. P., Thomas, J. P., Palmer, J., & Shimozaki, S. S. (2000). A signal detection model predicts the effects of set size on visual search accuracy for feature, conjunction, triple conjunction, and disjunction displays. Perception & Psychophysics, 62(3), 425–451. https://doi.org/10.3758/BF03212096
- Eimer, M. (1996). The N2pc component as an indicator of attentional selectivity. Electroencephalography and Clinical Neurophysiology, 99(3), 225–234. https://doi.org/10.1016/S0921-884X(96)95711-2
- Eimer, M. (2014). The neural basis of attentional control in visual search. Trends in Cognitive Sciences, 18(10), 526–535. https://doi.org/10.1016/j.tics.2014.05.005
- Eimer, M., & Kiss, M. (2008). Involuntary attentional capture is determined by task set: Evidence from event-related brain potentials. Journal of Cognitive Neuroscience, 20(8), 1423–1433. https://doi.org/10.1162/jocn.2008.20099
- Eimer, M., & Kiss, M. (2010). The top-down control of visual selection and how it is linked to the N2pc component. Acta Psychologica, 135(2), 100–102. https://doi.org/10.1016/j.actpsy.2010.04.010
- Fahrenfort, J. J., Grubert, A., Olivers, C. N. L., & Eimer, M. (2017). Multivariate EEG analyses support high-resolution tracking of feature-based attentional selection. Scientific Reports, 7(1), 1–15. https://doi.org/10.1038/s41598-017-01911-0
- Fahrenfort, J. J., van Driel, J., van Gaal, S., & Olivers, C. N. L. (2018). From ERPs to MVPA using the Amsterdam decoding and modeling toolbox (ADAM). Frontiers in Neuroscience, 12. https://doi.org/10.3389/fnins.2018.00368
- Failing, M., & Theeuwes, J. (2018). Selection history: How reward modulates selectivity of visual attention. Psychonomic Bulletin & Review, 25(2), 514–538. https://doi.org/10.3758/s13423-017-1380-y
- Fan, L., Sun, M., Xu, M., Li, Z., Diao, L., & Zhang, X. (2019). Multiple representations in visual working memory simultaneously guide attention: The type of memory-matching representation matters. Acta Psychologica, 192, 126–137. https://doi.org/10.1016/j.actpsy.2018.11.005
- Folk, C. L., & Anderson, B. A. (2010). Target-uncertainty effects in attentional capture: Color-singleton set or multiple attentional control settings? Psychonomic Bulletin & Review, 17(3), 421–426. https://doi.org/10.3758/PBR.17.3.421
- Folk, C. L., Leber, A. B., & Egeth, H. E. (2002). Made you blink! contingent attentional capture produces a spatial blink. Perception & Psychophysics, 64(5), 741–753. https://doi.org/10.3758/BF03194741
- Folk, C. L., Remington, R. W., & Johnston, J. C. (1992). Involuntary covert orienting is contingent on attentional control settings. Journal of Experimental Psychology: Human Perception and Performance, 18(4), 1030–1044. https://doi.org/10.1037/0096-1523.18.4.1030
- Found, A., & Müller, H. J. (1996). Searching for unknown feature targets on more than one dimension: Investigating a “dimension-weighting” account. Perception & Psychophysics, 58(1), 88–101. https://doi.org/10.3758/BF03205479
- Frătescu, M., Van Moorselaar, D., & Mathôt, S. (2019). Can you have multiple attentional templates? Large-scale replications of Van Moorselaar, Theeuwes, and Olivers (2014) and Hollingworth and Beck (2016). Attention, Perception, & Psychophysics, 81(8), 2700–2709. https://doi.org/10.3758/s13414-019-01791-8
- Giammarco, M., Paoletti, A., Guild, E. B., & Al-Aidroos, N. (2016). Attentional capture by items that match episodic long-term memory representations. Visual Cognition, 24(1), 78–101. https://doi.org/10.1080/13506285.2016.1195470
- Greenberg, A. S., Esterman, M., Wilson, D., Serences, J. T., & Yantis, S. (2010). Control of spatial and feature-based attention in frontoparietal cortex. The Journal of Neuroscience : The Official Journal of the Society for Neuroscience, 30(43), 14330–14339. https://doi.org/10.1523/JNEUROSCI.4248-09.2010
- Grubert, A., Carlisle, N. B., & Eimer, M. (2016). The control of single-color and multiple-color visual search by attentional templates in working memory and in long-term memory. Journal of Cognitive Neuroscience, 28(12), 1947–1963. https://doi.org/10.1162/jocn_a_01020
- Grubert, A., & Eimer, M. (2013). Qualitative differences in the guidance of attention during single-color and multiple-color visual search: Behavioral and electrophysiological evidence. Journal of Experimental Psychology: Human Perception and Performance, 39(5), 1433–1442. https://doi.org/10.1037/a0031046
- Grubert, A., & Eimer, M. (2015a). Rapid parallel attentional target selection in single-color and multiple-color visual search. Journal of Experimental Psychology: Human Perception and Performance, 41(1), 86–101. https://doi.org/10.1037/xhp0000019
- Grubert, A., & Eimer, M. (2015b). The speed of serial attention shifts in visual search: Evidence from the N2pc component. Journal of Cognitive Neuroscience, 28(2), 319–332. https://doi.org/10.1162/jocn_a_00898
- Grubert, A., & Eimer, M. (2016). All set, indeed! N2pc components reveal simultaneous attentional control settings for multiple target colors. Journal of Experimental Psychology: Human Perception and Performance, 42(8), 1215–1230. https://doi.org/10.1037/xhp0000221
- Grubert, A., Krummenacher, J., & Eimer, M. (2011). Redundancy gains in pop-out visual search are determined by top-down task set: Behavioral and electrophysiological evidence. Journal of Vision, 11(14), 10–10. https://doi.org/10.1167/11.14.10
- Hillstrom, A. P. (2000). Repetition effects in visual search. Perception & Psychophysics, 62(4), 800–817. https://doi.org/10.3758/BF03206924
- Hollingworth, A., & Beck, V. M. (2016). Memory-based attention capture when multiple items are maintained in visual working memory. Journal of Experimental Psychology. Human Perception and Performance, 42(7), 911–917. https://doi.org/10.1037/xhp0000230
- Hollingworth, A., Matsukura, M., & Luck, S. J. (2013). Visual working memory modulates rapid eye movements to simple onset targets. Psychological Science, 24(5), 790–796. https://doi.org/10.1177/0956797612459767
- Hout, M. C., & Goldinger, S. D. (2012). Target templates: How the precision of mental representations affects attentional guidance and decision-making in visual search. Journal of Experimental Psychology: Human Perception and Performance, 38(90–112), 128–149. https://doi.org/10.1037/a0023894
- Hout, M. C., & Goldinger, S. D. (2015). Target templates: The precision of mental representations affects attentional guidance and decision-making in visual search. Attention, Perception, & Psychophysics, 77(1), 128–149. https://doi.org/10.3758/s13414-014-0764-6
- Houtkamp, R., & Roelfsema, P. R. (2006). The effect of items in working memory on the deployment of attention and the eyes during visual search. Journal of Experimental Psychology: Human Perception and Performance, 32(2), 423–442. https://doi.org/10.1037/0096-1523.32.2.423
- Houtkamp, R., & Roelfsema, P. R. (2009). Matching of visual input to only one item at any one time. Psychological Research, 73(3), 317–326. https://doi.org/10.1007/s00426-008-0157-3
- Huang, L., Holcombe, A. O., & Pashler, H. (2004). Repetition priming in visual search: Episodic retrieval, not feature priming. Memory & Cognition, 32(1), 12–20. https://doi.org/10.3758/BF03195816
- Huang, L., & Pashler, H. (2007). A Boolean map theory of visual attention. Psychological Review, 114(3), 599–631. https://doi.org/10.1037/0033-295X.114.3.599
- Irons, J. L., Folk, C. L., & Remington, R. W. (2012). All set! Evidence of simultaneous attentional control settings for multiple target colors. Journal of Experimental Psychology: Human Perception and Performance, 38(3), 758–775. https://doi.org/10.1037/a0026578
- Irons, J. L., & Remington, R. W. (2013). Can attentional control settings be maintained for two color–location conjunctions? Evidence from an RSVP task. Attention, Perception, & Psychophysics, 75(5), 862–875. https://doi.org/10.3758/s13414-013-0439-8
- Ito, M., & Kawahara, J. I. (2016). Contingent attentional capture across multiple feature dimensions in a temporal search task. Acta Psychologica, 163, 107–113. https://doi.org/10.1016/j.actpsy.2015.11.009
- Itti, L., & Koch, C. (2000). A saliency-based search mechanism for overt and covert shifts of visual attention. Vision Research, 40(10), 1489–1506. https://doi.org/10.1016/S0042-6989(99)00163-7
- Jenkins, M., Grubert, A., & Eimer, M. (2016). The speed of voluntary and priority-driven shifts of visual attention. Journal of Experimental Psychology: Human Perception and Performance, 44(1), 27–37. https://doi.org/10.1037/xhp0000438
- Jóhannesson, ÓI, Thornton, I. M., Smith, I. J., Chetverikov, A., & Kristjánsson, Á. (2016). Visual foraging with fingers and eye gaze. I-Perception, 7(2), 1–18. https://doi.org/10.1177/2041669516637279
- Juola, J. F., Botella, J., & Palacios, A. (2004). Task- and location-switching effects on visual attention. Perception & Psychophysics, 66(8), 1303–1317. https://doi.org/10.3758/BF03195000
- Kaplan, I. T., & Carvellas, T. (1965). Scanning for multiple targets. Perceptual and Motor Skills, 21(1), 239–243. https://doi.org/10.2466/pms.1965.21.1.239
- Kastner, S., & Ungerleider, L. G. (2000). Mechanisms of visual attention in the human cortex. Annual Review of Neuroscience, 23(1), 315–341. https://doi.org/10.1146/annurev.neuro.23.1.315
- Kastner, S., & Ungerleider, L. G. (2001). The neural basis of biased competition in human visual cortex. Neuropsychologia, 39(12), 1263–1276. https://doi.org/10.1016/S0028-3932(01)00116-6
- Kerzel, D., & Witzel, C. (2019). The allocation of resources in visual working memory and multiple attentional templates. Journal of Experimental Psychology: Human Perception and Performance, 45(5), 645–658. https://doi.org/10.1037/xhp0000637
- Kiss, M., Jolicœur, P., Dell’Acqua, R., & Eimer, M. (2008). Attentional capture by visual singletons is mediated by top-down task set: New evidence from the N2pc component. Psychophysiology, 45(6), 1013–1024. https://doi.org/10.1111/j.1469-8986.2008.00700.x
- Kiyonaga, A., Egner, T., & Soto, D. (2012). Cognitive control over working memory biases of selection. Psychonomic Bulletin & Review, 19(4), 639–646. https://doi.org/10.3758/s13423-012-0253-7
- Klein, R. M., & MacInnes, W. J. (1999). Inhibition of return is a foraging facilitator in visual search. Psychological Science, 10(4), 346–352. https://doi.org/10.1111/1467-9280.00166
- Kristjánsson, Á, Björnsson, A. S., & Kristjánsson, T. (2020). Foraging with Anne Treisman: Features versus conjunctions, patch leaving and memory for foraged locations. Attention, Perception, & Psychophysics, https://doi.org/10.3758/s13414-019-01941-y
- Kristjánsson, Á, & Campana, G. (2010). Where perception meets memory: A review of repetition priming in visual search tasks. Attention, Perception & Psychophysics, 72(1), 5–18. https://doi.org/10.3758/APP.72.1.5
- Kristjánsson, Á, Jóhannesson, ÓI, & Thornton, I. M. (2014). Common attentional constraints in visual foraging. PloS One, 9(6), 1–9. https://doi.org/10.1371/journal.pone.0100752
- Kristjánsson, T., & Kristjánsson, Á. (2018). Foraging through multiple target categories reveals the flexibility of visual working memory. Acta Psychologica, 183, 108–115. https://doi.org/10.1016/j.actpsy.2017.12.005
- Kristjánsson, T., Thornton, I. M., Chetverikov, A., & Kristjánsson, Á. (2020). Dynamics of visual attention revealed in foraging tasks. Cognition, 194. Article 104032. https://doi.org/10.1016/j.cognition.2019.104032
- Kristjánsson, T., Thornton, I. M., & Kristjánsson, Á. (2018). Time limits during visual foraging reveal flexible working memory templates. Journal of Experimental Psychology: Human Perception and Performance, 44(6), 827–835. https://doi.org/10.1037/xhp0000517
- Kruijne, W., & Meeter, M. (2015). The long and the short of priming in visual search. Attention, Perception, & Psychophysics, 77(5), 1558–1573. https://doi.org/10.3758/s13414-015-0860-2
- Krummenacher, J., Müller, H. J., & Heller, D. (2001). Visual search for dimensionally redundant pop-out targets: Evidence for parallel-coactive processing of dimensions. Perception & Psychophysics, 63(5), 901–917. https://doi.org/10.3758/BF03194446
- Kumar, S., Soto, D., & Humphreys, G. W. (2009). Electrophysiological evidence for attentional guidance by the contents of working memory. European Journal of Neuroscience, 30(2), 307–317. https://doi.org/10.1111/j.1460-9568.2009.06805.x
- Lagroix, H. E. P., Yanko, M. R., & Spalek, T. M. (2018). Transition from feature-search to singleton-detection strategies in visual search: The role of number of target-defining options. Journal of Experimental Psychology: Human Perception and Performance, 44(3), 387–397. https://doi.org/10.1037/xhp0000467
- Leblanc, É, Prime, D. J., & Jolicoeur, P. (2008). Tracking the location of visuospatial attention in a contingent capture paradigm. Journal of Cognitive Neuroscience, 20(4), 657–671. https://doi.org/10.1162/jocn.2008.20051
- Lemaire, B., Pageot, A., Plancher, G., & Portrat, S. (2018). What is the time course of working memory attentional refreshing? Psychonomic Bulletin & Review, 25(1), 370–385. https://doi.org/10.3758/s13423-017-1282-z
- Liesefeld, H. R., Liesefeld, A. M., Töllner, T., & Müller, H. J. (2017). Attentional capture in visual search: Capture and post-capture dynamics revealed by EEG. NeuroImage, 156, 166–173. https://doi.org/10.1016/j.neuroimage.2017.05.016
- Liesefeld, H. R., & Müller, H. J. (2020). A theoretical attempt to revive the serial/parallel-search dichotomy. Attention, Perception, & Psychophysics, 82(1), 228–245. https://doi.org/10.3758/s13414-019-01819-z
- Liu, T., Becker, M. W., & Jigo, M. (2013). Limited featured-based attention to multiple features. Vision Research, 85, 36–44. https://doi.org/10.1016/j.visres.2012.09.001
- Liu, T., & Jigo, M. (2017). Limits in feature-based attention to multiple colors. Attention, Perception, & Psychophysics, 79(8), 2327–2337. https://doi.org/10.3758/s13414-017-1390-x
- Liu, T., Slotnick, S. D., Serences, J. T., & Yantis, S. (2003). Cortical mechanisms of feature-based attentional control. Cerebral Cortex, 13(12), 1334–1343. https://doi.org/10.1093/cercor/bhg080
- Luck, S. J. (2014). An introduction to the event-related potential technique. MIT Press.
- Luck, S. J., & Hillyard, S. A. (1994). Electrophysiological correlates of feature analysis during visual search. Psychophysiology, 31(3), 291–308. https://doi.org/10.1111/j.1469-8986.1994.tb02218.x
- Luck, S. J., & Vogel, E. K. (1997). The capacity of visual working memory for features and conjunctions. Nature, 390(6657), 279–281. https://doi.org/10.1038/36846
- Ma, W. J., Husain, M., & Bays, P. M. (2014). Changing concepts of working memory. Nature Neuroscience, 17(3), 347–356. https://doi.org/10.1038/nn.3655
- Madrid, J., & Hout, M. C. (2019). Examining the effects of passive and active strategies on behavior during hybrid visual memory search: Evidence from eye tracking. Cognitive Research: Principles and Implications, 4(1), 39. https://doi.org/10.1186/s41235-019-0191-2
- Maljkovic, V., & Nakayama, K. (1994). Priming of pop-out: I. Role of features. Memory & Cognition, 22(6), 657–672. https://doi.org/10.3758/BF03209251
- Mannan, S. K., Kennard, C., Potter, D., Pan, Y., & Soto, D. (2010). Early oculomotor capture by new onsets driven by the contents of working memory. Vision Research, 50(16), 1590–1597. https://doi.org/10.1016/j.visres.2010.05.015
- Manohar, S. G., Zokaei, N., Fallon, S. J., Vogels, T. P., & Husain, M. (2019). Neural mechanisms of attending to items in working memory. Neuroscience & Biobehavioral Reviews, 101, 1–12. https://doi.org/10.1016/j.neubiorev.2019.03.017
- Martinovic, J., Wuerger, S. M., Hillyard, S. A., Müller, M. M., & Andersen, S. K. (2018). Neural mechanisms of divided feature-selective attention to colour. NeuroImage, 181, 670–682. https://doi.org/10.1016/j.neuroimage.2018.07.033
- Maunsell, J. H. R., & Treue, S. (2006). Feature-based attention in visual cortex. Trends in Neurosciences, 29(6), 317–322. https://doi.org/10.1016/j.tins.2006.04.001
- McCants, C. W., Berggren, N., & Eimer, M. (2018). The guidance of visual search by shape features and shape configurations. Journal of Experimental Psychology: Human Perception and Performance, 44(7), 1072–1085. https://doi.org/10.1037/xhp0000514
- Meeter, M., & Olivers, C. N. L. (2006). Intertrial priming stemming from ambiguity: A new account of priming in visual search. Visual Cognition, 13(2), 202–222. https://doi.org/10.1080/13506280500277488
- Meiran, N. (2010). Task switching and cognitive self control. In Self control in society, mind and brain (pp. 202–220). https://doi.org/10.1093/acprof:oso/9780195391381.001.0001
- Menneer, T., Barrett, D. J. K., Phillips, L., Donnelly, N., & Cave, K. R. (2007). Costs in searching for two targets: Dividing search across target types could improve airport security screening. Applied Cognitive Psychology, 21(7), 915–932. https://doi.org/10.1002/acp.1305
- Menneer, T., Cave, K. R., & Donnelly, N. (2009). The cost of search for multiple targets: Effects of practice and target similarity. Journal of Experimental Psychology: Applied, 15(2), 125–139. https://doi.org/10.1037/a0015331
- Menneer, T., Stroud, M. J., Cave, K. R., Li, X., Godwin, H. J., Liversedge, S. P., & Donnelly, N. (2012). Search for two categories of target produces fewer fixations to target-color items. Journal of Experimental Psychology: Applied, 18(4), 404–418. https://doi.org/10.1037/a0031032
- Mestry, N., Menneer, T., Cave, K. R., Godwin, H. J., & Donnelly, N. (2017). Dual-target cost in visual search for multiple unfamiliar faces. Journal of Experimental Psychology: Human Perception and Performance, 43(8), 1504–1519. https://doi.org/10.1037/xhp0000388
- Miller, J. (1982). Divided attention: Evidence for coactivation with redundant signals. Cognitive Psychology, 14(2), 247–279. https://doi.org/10.1016/0010-0285(82)90010-X
- Monsell, S. (2003). Task switching. Trends in Cognitive Sciences, 7(3), 134–140. https://doi.org/10.1016/S1364-6613(03)00028-7
- Moore, C. M., & Osman, A. M. (1993). Looking for two targets at the same time: One search or two? Perception & Psychophysics, 53(4), 381–390. https://doi.org/10.3758/BF03206781
- Moore, K. S., & Weissman, D. H. (2010). Involuntary transfer of a top-down attentional set into the focus of attention: Evidence from a contingent attentional capture paradigm. Attention, Perception & Psychophysics, 72(6), 1495–1509. https://doi.org/10.3758/APP
- Moore, K. S., & Weissman, D. H. (2011). Set-specific capture can be reduced by preemptively occupying a limited-capacity focus of attention. Visual Cognition, 19(4), 417–444. https://doi.org/10.1080/13506285.2011.558862
- Moran, J., & Desimone, R. (1985). Selective attention gates visual processing in the extrastriate cortex. Science, 229(4715), 782–784. https://doi.org/10.1126/science.4023713
- Mordkoff, J. T., & Yantis, S. (1991). An interactive race model of divided attention. Journal of Experimental Psychology: Human Perception and Performance, 17(2), 520–538. https://doi.org/10.1037/0096-1523.17.2.520
- Motter, B. C. (1994). Neural correlates of attentive selection for color or luminance in extrastriate area V4. Journal of Neuroscience, 14(4), 2178–2189. https://doi.org/10.1523/JNEUROSCI.14-04-02178.1994
- Müller, H. J., Heller, D., & Ziegler, J. (1995). Visual search for singleton feature targets within and across feature dimensions. Perception & Psychophysics, 57(I), 1–17. https://doi.org/10.3758/BF03211845
- Müller, M. M., Malinowski, P., Gruber, T., & Hillyard, S. A. (2003). Sustained division of the attentional spotlight. Nature, 424(6946), 309–312. https://doi.org/10.1038/nature01812
- Nakajima, M., Schmitt, L. I., & Halassa, M. M. (2019). Prefrontal cortex regulates sensory filtering through a Basal Ganglia-to-Thalamus pathway. Neuron, 103(3), 445–458.e10. https://doi.org/10.1016/j.neuron.2019.05.026
- Norcia, A. M., Appelbaum, L. G., Ales, J. M., Cottereau, B. R., & Rossion, B. (2015). The steady-state visual evoked potential in vision research: A review. Journal of Vision, 15(6), 1–46. https://doi.org/10.1167/15.6.4
- Olivers, C. N. L. (2009). What drives memory-driven attentional capture? The effects of memory type, display type, and search type. Journal of Experimental Psychology: Human Perception and Performance, 35(5), 1275–1291. https://doi.org/10.1037/a0013896
- Olivers, C. N. L., & Eimer, M. (2011). On the difference between working memory and attentional set. Neuropsychologia, 49(6), 1553–1558. https://doi.org/10.1016/j.neuropsychologia.2010.11.033
- Olivers, C. N. L., & Humphreys, G. W. (2003). Attentional guidance by salient feature singletons depends on intertrial contingencies. Journal of Experimental Psychology. Human Perception and Performance, 29(3), 650–657. https://doi.org/10.1037/0096-1523.29.3.650
- Olivers, C. N. L., & Meeter, M. (2006). On the dissociation between compound and present/absent tasks in visual search: Intertrial priming is ambiguity driven. Visual Cognition, 13(1), 1–28. https://doi.org/10.1080/13506280500308101
- Olivers, C. N. L., Meijer, F., & Theeuwes, J. (2006). Feature-based memory-driven attentional capture: Visual working memory content affects visual attention. Journal of Experimental Psychology: Human Perception and Performance, 32(5), 1243–1265. https://doi.org/10.1037/0096-1523.32.5.1243
- Olivers, C. N. L., Peters, J., Houtkamp, R., & Roelfsema, P. R. (2011). Different states in visual working memory: When it guides attention and when it does not. Trends in Cognitive Sciences, 15(7), 327–334. https://doi.org/10.1016/j.tics.2011.05.004
- Ort, E., Fahrenfort, J. J., & Olivers, C. N. L. (2017). Lack of free choice Reveals the cost of having to look for more than one object. Psychological Science, 28(8), 1137–1147. https://doi.org/10.1177/0956797617705667
- Ort, E., Fahrenfort, J. J., & Olivers, C. N. L. (2018). Lack of free choice reveals the cost of multiple-target search within and across feature dimensions. Attention, Perception, and Psychophysics, 80(8), 1904–1917. https://doi.org/10.3758/s13414-018-1579-7
- Ort, E., Fahrenfort, J. J., Reeder, R., Pollmann, S., & Olivers, C. N. L. (2019). Frontal cortex differentiates between free and imposed target selection in multiple-target search. NeuroImage, 202, 116133. https://doi.org/10.1016/j.neuroimage.2019.116133
- Ort, E., Fahrenfort, J. J., ten Cate, T., Eimer, M., & Olivers, C. N. (2019). Humans can efficiently look for but not select multiple visual objects. ELife, 8. Article e49130. https://doi.org/10.7554/eLife.49130
- Palmer, J., Verghese, P., & Pavel, M. (2000). The psychophysics of visual search. Vision Research, 40(10), 1227–1268. https://doi.org/10.1016/S0042-6989(99)00244-8
- Parrott, S. E., Levinthal, B. R., & Franconeri, S. L. (2010). Complex attentional control settings. Quarterly Journal of Experimental Psychology, 63(12), 2297–2304. https://doi.org/10.1080/17470218.2010.520085
- Quinlan, P. T., & Humphreys, G. W. (1987). Visual search for targets defined by combinations of color, shape, and size: An examination of the task constraints on feature and conjunction searches. Perception & Psychophysics, 41(5), 455–472. https://doi.org/10.3758/BF03203039
- Raye, C. L., Johnson, M. K., Mitchell, K. J., Greene, E. J., & Johnson, M. R. (2007). Refreshing: A minimal executive function. Cortex, 43(1), 135–145. https://doi.org/10.1016/S0010-9452(08)70451-9
- Raymond, J. E., Shapiro, K. L., & Arnell, K. M. (1992). Temporary suppression of visual processing in an RSVP task: An attentional blink? Journal of Experimental Psychology: Human Perception and Performance, 18(3), 849–860. https://doi.org/10.1037/0096-1523.18.3.849
- Re, D., Inbar, M., Richter, C. G., & Landau, A. N. (2019). Feature-based attention samples stimuli rhythmically. Current Biology, 29(4), 693–699.e4. https://doi.org/10.1016/j.cub.2019.01.010
- Roper, Z. J. J., & Vecera, S. P. (2012). Searching for two things at once: Establishment of multiple attentional control settings on a trial-by-trial basis. Psychonomic Bulletin & Review, 19(6), 1114–1121. https://doi.org/10.3758/s13423-012-0297-8
- Saenz, M., Buracas, G. T., & Boynton, G. M. (2002). Global effects of feature-based attention in human visual cortex. Nature Neuroscience, 5(7), 631–632. https://doi.org/10.1038/nn876
- Serences, J. T. (2016). Neural mechanisms of information storage in visual short-term memory. Vision Research, 128, 53–67. https://doi.org/10.1016/j.visres.2016.09.010
- Snodgrass, J. G., & Townsend, J. T. (1980). Comparing parallel and serial models: Theory and implementation. Journal of Experimental Psychology: Human Perception and Performance, 6(2), 330–354. https://doi.org/10.1037/0096-1523.6.2.330
- Soto, D., Greene, C. M., Chaudhary, A., & Rotshtein, P. (2012). Competition in working memory reduces frontal guidance of visual selection. Cerebral Cortex, 22(5), 1159–1169. https://doi.org/10.1093/cercor/bhr190
- Soto, D., Heinke, D., Humphreys, G. W., & Blanco, M. J. (2005). Early, involuntary top-down guidance of attention from working memory. Journal of Experimental Psychology: Human Perception and Performance, 31(2), 248–261. https://doi.org/10.1037/0096-1523.31.2.248
- Soto, D., Hodsoll, J., Rothstein, P., & Humphreys, G. W. (2008). Automatic guidance of attention from working memory. Trends in Cognitive Sciences, 12(9), 342–348. https://doi.org/10.1016/j.tics.2008.05.007
- Soto, D., & Humphreys, G. W. (2008). Stressing the mind: The effect of cognitive load and articulatory suppression on attentional guidance from working memory. Perception & Psychophysics, 70(5), 924–934. https://doi.org/10.3758/PP.70.5.924
- Soto, D., Humphreys, G. W., & Heinke, D. (2006). Working memory can guide pop-out search. Vision Research, 46(6), 1010–1018. https://doi.org/10.1016/j.visres.2005.09.008
- Stroud, M. J., Menneer, T., Cave, K. R., & Donnelly, N. (2012). Using the dual-target cost to explore the nature of search target representations. Journal of Experimental Psychology: Human Perception and Performance, 38(1), 113–122. https://doi.org/10.1037/a0025887
- Stroud, M. J., Menneer, T., Cave, K. R., Donnelly, N., & Rayner, K. (2011). Search for multiple targets of different colours: Misguided eye movements reveal a reduction of colour selectivity. Applied Cognitive Psychology, 25(6), 971–982. https://doi.org/10.1002/acp.1790
- Stroud, M. J., Menneer, T., Kaplan, E., Cave, K. R., & Donnelly, N. (2019). We can guide search by a set of colors, but are reluctant to do it. Attention, Perception, & Psychophysics, 81(2), 377–406. https://doi.org/10.3758/s13414-018-1617-5
- Theeuwes, J. (1992). Perceptual selectivity for color and form. Perception & Psychophysics, 51(6), 599–606. https://doi.org/10.3758/BF03211656
- Thigpen, N., Petro, N. M., Oschwald, J., Oberauer, K., & Keil, A. (2019). Selection of visual objects in perception and working memory one at a time. Psychological Science, 30(9), 1259–1272. https://doi.org/10.1177/0956797619854067
- Töllner, T., Conci, M., Müller, H. J., & Mazza, V. (2016). Attending to multiple objects relies on both feature- and dimension-based control mechanisms: Evidence from human electrophysiology. Attention, Perception, & Psychophysics, 78(7), 2079–2089. https://doi.org/10.3758/s13414-016-1152-1
- Töllner, T., Gramann, K., Müller, H. J., Kiss, M., & Eimer, M. (2008). Electrophysiological markers of visual dimension changes and response changes. Journal of Experimental Psychology. Human Perception and Performance, 34(3), 531–542. https://doi.org/10.1037/0096-1523.34.3.531
- Töllner, T., Zehetleitner, M., Krummenacher, J., & Müller, H. J. (2011). Perceptual basis of redundnacy gains in visual pop out search. Journal of Cognitive Neuroscience, 23(1), 137–150. https://doi.org/10.1162/jocn.2010.21422
- Townsend, J. T. (1990). Serial vs. parallel processing: Sometimes they look like tweedledum and tweedledee but they can (and should) be distinguished. Psychological Science, 1(1), 46–54. https://doi.org/10.1111/j.1467-9280.1990.tb00067.x
- Townsend, J. T., & Ashby, F. G. (1983). Stochastic modeling of elementary psychological processes. CUP Archive.
- Townsend, J. T., & Nozawa, G. (1995). Spatio-temporal properties of elementary perception: An investigation of parallel, serial, and coactive theories. Journal of Mathematical Psychology, 39(4), 321–359. https://doi.org/10.1006/jmps.1995.1033
- Treisman, A. M. (1988). Features and objects: The fourteenth Bartlett memorial lecture. The Quarterly Journal of Experimental Psychology, 40(2), 201–237. https://doi.org/10.1080/02724988843000104
- Treisman, A. M., & Gelade, G. (1980). A feature-integration theory of attention. Cognitive Psychology, 12(1), 97–136. https://doi.org/10.1016/0010-0285(80)90005-5
- Treue, S., & Trujillo Martínez, J. C. (1999). Feature-based attention influences motion processing gain in macaque visual cortex. Nature, 399(6736), 575–579. https://doi.org/10.1038/21176
- Urai, A. E., de Gee, J. W., Tsetsos, K., & Donner, T. H. (2019). Choice history biases subsequent evidence accumulation. ELife, 8. Article e46331. https://doi.org/10.7554/eLife.46331
- Vandierendonck, A., Liefooghe, B., & Verbruggen, F. (2010). Task switching: Interplay of reconfiguration and interference control. Psychological Bulletin, 136(4), 601–626. https://doi.org/10.1037/a0019791
- van Driel, J., Ort, E., Fahrenfort, J. J., & Olivers, C. N. L. (2019). Beta and theta oscillations differentially support free versus forced control over multiple-target search. Journal of Neuroscience, 39(9), 1733–1743. https://doi.org/10.1523/JNEUROSCI.2547-18.2018
- van Loon, A. M., Olmos Solis, K., & Olivers, C. N. L. (2017). Subtle eye movement metrics reveal task-relevant representations prior to visual search. Journal of Vision, 17(6), 13–13. https://doi.org/10.1167/17.6.13
- van Moorselaar, D., Theeuwes, J., & Olivers, C. N. L. (2014). In competition for the attentional template: Can multiple items within visual working memory guide attention? Journal of Experimental Psychology. Human Perception and Performance, 40(4), 1450–1464. https://doi.org/10.1037/a0036229
- VanRullen, R. (2016). Perceptual cycles. Trends in Cognitive Sciences, 20(10), 723–735. https://doi.org/10.1016/j.tics.2016.07.006
- Williams, J. R., Brady, T. F., & Störmer, V. S. (2019). Natural variation in the fidelity of working memory representations cause only one item to guide attention [Preprint]. https://doi.org/10.31234/osf.io/c4t92
- Wolfe, J. M. (1994). Guided search 2.0 A revised model of visual search. Psychonomic Bulletin & Review, 1(2), 202–238. https://doi.org/10.3758/BF03200774
- Wolfe, J. M. (2007). Guided search 4.0: Current progress with a model of visual search. In Integrated models of cognitive systems (pp. 99–119). https://doi.org/10.1093/acprof
- Wolfe, J. M. (2012). Saved by a log: How do humans perform hybrid visual and memory search? Psychological Science, 23(7), 698–703. https://doi.org/10.1177/0956797612443968
- Wolfe, J. M., Aizenman, A. M., Boettcher, S. E. P., & Cain, M. S. (2016). Hybrid foraging search: Searching for multiple instances of multiple types of target. Vision Research, 119, 50–59. https://doi.org/10.1016/j.visres.2015.12.006
- Wolfe, J. M., Butcher, S. J., Lee, C., & Hyle, M. (2003). Changing your mind: On the contributions of top-down and bottom-up guidance in visual search for feature singletons. Journal of Experimental Psychology: Human Perception and Performance, 29(2), 483–502. https://doi.org/10.1037/0096-1523.29.2.483
- Wolfe, J. M., Horowitz, T. S., Kenner, N., Hyle, M., & Vasan, N. (2004). How fast can you change your mind? The speed of top-down guidance in visual search. Vision Research, 44(12), 1411–1426. https://doi.org/10.1016/j.visres.2003.11.024
- Wolfe, J. M., & Van Wert, M. J. (2010). Varying target prevalence reveals two dissociable decision criteria in visual search. Current Biology, 20(2), 121–124. https://doi.org/10.1016/j.cub.2009.11.066
- Wolfe, J. M., Yu, K. P., Stewart, M. I., Shorter, a. D., Friedman-Hill, S. R., & Cave, K. R. (1990). Limitations on the parallel guidance of visual search: Color x color and orientation x orientation conjunctions. Journal of Experimental Psychology: Human Perception and Performance, 16(4), 879–892. https://doi.org/10.1037/0096-1523.16.4.879
- Wu, X., & Fu, S. (2017). The different roles of category- and feature-specific attentional control settings on attentional enhancement and inhibition. Attention, Perception, & Psychophysics, 79(7), 1968–1978. https://doi.org/10.3758/s13414-017-1363-0
- Wu, S.-C., & Remington, R. W. (2003). Characteristics of covert and overt visual orienting: Evidence from attentional and oculomotor capture. Journal of Experimental Psychology: Human Perception and Performance, 29(5), 1050–1067. https://doi.org/10.1037/0096-1523.29.5.1050
- Wyble, B., Folk, C., & Potter, M. C. (2013). Contingent attentional capture by conceptually relevant images. Journal of Experimental Psychology: Human Perception and Performance, 39(3), 861–871. https://doi.org/10.1037/a0030517
- Wylie, G., & Allport, A. (2000). Task switching and the measurement of “switch costs”. Psychological Research, 63(3–4), 212–233. https://doi.org/10.1007/s004269900003
- Zhang, B., Liu, S., Doro, M., & Galfano, G. (2018). Attentional guidance from multiple working memory representations: Evidence from eye movements. Scientific Reports, 8(1), 1–9. https://doi.org/10.1038/s41598-018-32144-4
- Zhang, B., Zhang, J. X., Huang, S., Kong, L., & Wang, S. (2011). Effects of load on the guidance of visual attention from working memory. Vision Research, 51(23), 2356–2361. https://doi.org/10.1016/j.visres.2011.09.008
- Zhou, C., Lorist, M. M., & Mathôt, S. (2019). Behavioral and Computational Evidence for Simultaneous Guidance of Attention by Multiple Working Memory Items [Preprint]. https://doi.org/10.1101/629378