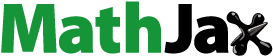
ABSTRACT
Scholars and policy makers frame the debate on labour market polarisation by emphasising the role of key drivers such as international trade and of technological change. The present paper explores these themes from a different perspective and inquires whether de-routinisation has harmed local innovation capacity. Our empirical study builds on the literature on learning-by-doing and incremental innovation and focuses on advanced manufacturing technologies (AMTs) in US Metropolitan Statistical Areas over the period 1990–2012. Results provide support to the hypothesis that de-routinisation is associated with a generalised decline of local innovation performance, particularly in AMTs.
1. Introduction
The advent of digital technologies has rejuvenated the debate on the economic and social effects of innovation. The so-called digital transformation is widely regarded as a discontinuity emanating from the Information and Communication Technology (ICT) revolution that gained momentum in the 1990s and that triggered significant changes both in employment levels and in the structure of labour markets (Organisation for Economic Co-operation and Development [OECD] Citation2016; World Bank Citation2016; Van Roy, Vértesy, and Vivarelli Citation2018).
A growing strand of research on employment and innovation analyses the labour market outcomes associated with the computer revolution. Innovation scholars argue that indirect income and price effects offset the direct effect of job destruction due to the adoption of new machinery and equipment. Whether and to what extent these compensation mechanisms work, and whether price or income effects dominate, depends on institutional factors that circumscribe the validity of empirical findings (Freeman and Soete, Citation1987; Pianta, Citation2003; Vivarelli, 1995, Vivarelli Citation2014; Piva and Vivarelli Citation2018). Studies in labour economics argue that technological change is skill-biased, and that therefore job creation and job destruction reflect a positive relation between workers’ skill levels – often proxied by years of schooling – and labour market returns (Autor, Katz, and Krueger Citation1998; Chennells and Reenen Citation1999; Acemoglu Citation2002). The predictions based on these approaches, however, do not match observed patterns of changes in labour demand. Rather, the evidence indicates that in the wake of the ICT revolution the bulk of job destruction occurred in the middle of the skill spectrum and not in the bottom part, as the skill-biased technical change (SBTC) tenet predicts (Autor, Levy, and Murnane Citation2003; Goos and Manning Citation2007; Goos, Manning, and Salomons Citation2014). Upon closer inspection, technology had a dual effect on labour demand: it substituted for routine (cognitive and manual) tasks that are more intensive among mid-skill jobs – e.g., clerks and machine operators – while increasing the productivity of, and the demand for, occupations at the opposite ends of the employment spectrum, namely high- and low- jobs that entail primarily non-routine (cognitive and manual) activities. This process is known as de-routinisation, or job polarisation. Recent studies find that technology is not only job destroying and that expanded possibilities, in the form of new products and processes, can create demand for new occupations (Bessen, Citation2018; Acemoglu and P. Restrepo Citation2019; Gregory, Salomons, and Zierahn Citation2016; Klenert, Fernández-Macías, and Antón Citation2020). Overall, the evidence reveals a more complex picture than the dominant narrative ‘robots are coming for workers’ would suggest.
This intense debate revolves around the question of whether technological change affects employment, and how much. The starting point of the present paper is that such a relationship is not only controversial for what concerns the balance between compensation and substitution effects, but also in regard to the directionality. A large body of literature, for example, suggests that labour market dynamics shape innovative performance. On the one hand, empirical studies show that flexibility and deregulation can hinder innovation due to the crowding out of firms’ core capabilities (Kleinknecht, Van Schaik, and Zhou Citation2014; Michie and Sheehan Citation2003; Wachsen and Blind Citation2016; Zhou, Dekker, and Kleinknecht Citation2011). On the other hand, for similar reasons, excess worker turnover is likely to have a negative impact on firms’ innovation dynamics (Grinza and Quatraro Citation2019).
We propose that these considerations are also relevant for the current debate on job polarisation, albeit in a way that differs from what has become the standard framing. While the literature looks mainly at how digital technologies trigger de-routinisation, the question of whether and to what extent the loss, or of the diminished availability, of routine skills may have affected innovation capacity has been disregarded. What’s more, and central to the present paper, blue-collar work tasks and the attending skills are essential for productivity and innovation. Two streams of empirical literature provide support to this intuition. The first is based on Rosenberg’s (1974) classic critique to the debate on innovation, which focused excessively on the creative leaps that enable new technologies to stem out of basic research while it disregarded incremental ‘downstream’ improvements and the importance of other knowledge sources. A second domain of applied literature shows evidence that production, mid-skill workers are crucial to achieve incremental innovation (Waeyenbergh and Pintelon, Citation2002; Alsyouf Citation2007; Kukla Citation1983; Sohal et al. Citation2001; Deivanayagam Citation1992; LewisCitation2020a, Citation2020b).
Building on the above, the present paper empirically analyses whether and to what extent the decline of mid-skill routine employment has been detrimental to innovation capacity in Metropolitan Areas (MSAs) of the United States (US). Specifically, this question is addressed in relation to innovation in manufacturing sectors over the period 1990–2012. In line with recent trends, we look at patenting dynamics in advanced manufacturing technologies (AMT), a group of integrated hardware-based and software-based solutions used in the design, manufacture or handling of products (Organisation for Economic Co-operation and Development [OECD] Citation2012). We hypothesise that routine workers are crucial to incremental experimentation and problem-solving that stand at the core of incremental innovation. Because of the specific features of this process, we further submit that the prolonged decline of demand for routine jobs observed in the United States has undermined the ability to innovate in organisational ecosystems that revolve around AMTs.
The main finding is that a 1% loss in routine-intensive occupations is negatively associated with a reduction in the local patenting capacity of around 1.8%. Interestingly, the decrease of cognitive- and manual-routine skills are negatively associated to local innovation capacity both in general and to AMTs in particular. In fact, the latter reduce more when routine jobs decrease due to losses in manual-repetitive occupations. Moreover, we also find a more pronounced negative association between routine-job losses and the local generation of incremental innovation, particularly in AMT fields. Our contribution to the literature is threefold. First, we add to the empirical work on the task-based approach by underlining that task heterogeneity captures important variance in innovation dynamics. Second, we enrich the debate on the relationship between digital technologies and labour market dynamics byinverting the traditional direction of the link between them. Third, we elaborate upon the importance of a specific set of technologies, i.e., AMTs, which are crucianot only because of their productivity-enhancing effect, but also due to their innovation-enabling role.
The rest of the paper is organised as follows. Section 2 reviews the relevant literature and develops the working hypotheses. In Section 3 we detail the main data sources, the main variables and the empirical models adopted. Section 4 presents and discuss the results. The last section concludes and summarises.
2. Theoretical background
2.1. On innovation and labour market dynamics
Academic scholars and policy makers have recently become more alert to the nature and the extent of the structural changes that are transforming the labour market, and to their relationship with the current wave of technological change in the digital domain.
The technological discontinuities that followed the ICT revolution has brought the relationship between innovation and employment back at the core of the policy and academic debates. In innovation studies, empirical analysis focuses on the existence and extent of substitution and compensation effects, the former consisting of job displacement the latter by the counterbalancing job creation due to productivity gains and new job creation (Vivarelli Citation2014; Piva and Vivarelli Citation2018; Vivarelli and Pianta Citation2000; Cirillo Citation2017). The generality of the various findings is often controversial due to the influence of institutional factors, such as regulation, that can affect the degree of market competition.
While many investigate the impact of ICTs on overall employment growth, considerations about the specific kinds of jobs that these technologies would have displaced, as well as the types of jobs that they would have promoted enrich the debate on the effects of technology. If on the one hand ICTs have accelerated the obsolescence of some occupations, on the other hand their diffusion led to an increase in demand of other occupations. According to the SBTC tenet, ICTs would affect the demand for workers depending on their skill level, thereby favouring high-skilled occupations and hindering low-skill ones (Autor, Katz, and Krueger Citation1998; Chennells and Reenen Citation1999; Acemoglu Citation2002). At the same time, recent literature on the impact of robots’ adoption on wages or employment points to mixed evidence due to a variety of reasons – i.e., differences across datasets, heterogenous time spans (Klenert, Fernández-Macías, and Antón Citation2020).
An important turning point in this line of research is the emergence of evidence that labour demand has grown for high- and low-skill jobs, and that the decline was mostly concentrated in the middle of the occupational skill spectrum. Although there still is controversy over the timing and the degree of the so-called employment polarisation, broad consensus exists on the underlying mechanics. The decline of demand for mid-skill occupations is in effect capital-labour substitution due to both falling prices of computing power and higher efficiency of automated processes in carrying out routine work tasks (Autor, Katz, and Krueger Citation1998; Autor, Levy, and Murnane Citation2003; Goos and Manning Citation2007; Goos, Manning, and Salomons Citation2014; Gregory, Salomons, and Zierahn Citation2016).Footnote1 A new approach stemming from the pioneering work of Autor, Levy, and Murnane (Citation2003) (ALM henceforth) focused directly on job skills and tasks, rather than inferring them through proxies such as a worker’s years of education. In this framework, occupational task content is understood as the ensemble of work activities that are necessary for a job to produce a unit of output. Compared to the traditional human capital theory, this approach affords a more nuanced view of how advances in technologies, changes in skill supplies, or the emergence of trade and offshoring opportunities affect the division of labour between workers and machines, the relevance of particular job tasks and, ultimately, the demand for skills (Acemoglu and Autor Citation2011).
In the ALM framework occupations are defined by the main work tasks. Accordingly, routine tasks (i.e., executing codified instructions with minimal discretion) are characteristic of middle-skilled jobs that entail repetitive cognitive (i.e., clerks) or manual (i.e., blue-collar) duties. Because routine tasks exist in the forms of rules and instructions, and since the quality of computer and communication technologies has increased while their price has declined, routine tasks are prone to be reassigned to machines or, alternatively, to be performed by low-wage workers in offshore locations. The second main category includes activities that require creativity, problem solving, intuition and social perceptiveness. These abstract tasks are characteristic of professional, managerial, technical and creative occupations that require high levels of formal education. Since analytic and interpersonal capabilities are so important, computers accrue productivity benefits to these workers by facilitating the transmission, organisation, and processing of information. This is why technology generally complements, rather than substituting, these occupations. On the other side of the skill spectrum are manual tasks, which demand visual and language recognition, personal interaction and physical dexterity. Occupations that use intensively these tasks are typically concentrated in low-skill service jobs such as food preparation, catering, driving and cleaning. Given the significant challenges entailed in automating these activities, workers in these jobs are relatively unscathed by the computer revolution.
The empirical literature in this strand focuses primarily on the contractionary impacts of international trade and of technology on employment, especially in manufacturing industries. These studies however disregard the effect of the loss, or of the diminished availability, of routine skills.
2.2. The importance of routine jobs for innovation
In spite of the profound transformations of manufacturing, blue-collar workers, and their skills, are still central to production activities (Piva, Grilli, and Rossi-Lamastra Citation2011). No doubt, the diffusion of information technology together with pressures from international competition have altered the organisation of job tasks and, a fortiori, the demand for the attendant skills. In highly automated factories and production sites of the United States workers are expected to command know-how that is considerably more specialised than it would have been a few decades ago (Dietz and Orr Citation2006). At the same time, there is evidence on factories that opt for retraining the old workforce after implementing continuous processing and control systems (Fernandez Citation2001). This is, we argue, because at the core of routine tasks embedded in blue collar jobs is a technical labour that stands at the interface between engineering and manufacturing, and consists in tacit skills heavily reliant on experience of translating the requirements of each group for the other (Barley Citation1996; Barley and Bechky Citation1994). The responsibilities of factory floor blue-collar workers entail combined use of physical and conceptual dexterity and blending technical knowledge with practical experience (Drucker Citation1999). While in the factory of the past these hands-on skills were primarily involved in the physical manipulation of specific equipment, in the modern-era factory floor employees operate ensembles of machines using routine manual skills such as assembling, maintaining and coordinating. According to the technical literature, the importance of these skills has increased together with the complexity of automated production whereby factory floor workers are now committed to high standards of performance over efficiency, quality, on-time delivery, safety, and plant cost effectiveness (Al-Najjar Citation2000; Riis, Luxhoj, and Uffe Citation1997; Mckone and Elliott Citation1998).
Further and central to the argument of the present paper, blue-collar type of routine tasks, and the attending skills, are essential for productivity and innovation. Two streams of empirical literature support this claim. The first stems from the classic Rosenberg’s (Citation1976) critique that the debate on innovation has focused excessively on the creative leaps that enable new technologies to stem out of basic research while it has neglected the role of other, less formal, knowledge sources and of incremental ‘downstream’ improvements. A few instances of the latter are the design of new products, testing and evaluating their performance through prototypes, implementing new production processes. Common to this wide range of activities is that they consist of minor modifications that better integrate design and production, establish new feedback channels between users and suppliers, and ultimately tune existing production methods. While taken individually each of these modifications may yield small improvements in performance, their cumulative effects have been observed to be massive in domains as different as agriculture, machine production and aeronautics (Rosenberg and Steinmueller Citation2013). According to a regrettably scant literature, blue collars have the potential to develop useful knowledge, ideas and competences that contribute to a firm’s innovation capacity (see i.e. Lewis Citation2020a, Citation2020bb). As Bradley, Kim, and Tian (Citation2017) point out, this class of workers can have an impact on innovation for different reasons. A first channel consists of knowledge inputs originating among production workers and flowing up to the management. Moreover, floor workers often serve as supporting staff for researchers and scientists. Indeed, according to Hayek (Citation1945), the specific knowledge developed by floor workers can be useful to firm’s innovation performance only if they are actively involved in these dynamics.
A second stream of applied literature provides relevant evidence on the extent to which incremental improvements can be ascribed to the tacit know-how of factory floor workers. These improvements include reducing downtime, limiting costs and increasing equipment productivity across a wide range of industries (Waeyenbergh and Pintelon Citation2004; Alsyouf Citation2007) as well as new product (Kukla Citation1983; Sohal et al. Citation2001) and process development (Deivanayagam Citation1992). Last but not least, there is evidence of growing importance of blue-collar workers for knowledge-bridging across functional departments, including those performing R&D, of the modern factory (Langowitz Citation1988; Hoopes and Postrel Citation1999).Footnote2
Based on these arguments, our first hypothesis is:
H1: De-routinisation is associated with decreasing innovation performance.
2.3. Routine jobs and advanced manufacturing technologies
Our empirical analysis focuses on innovation in Advanced Manufacturing Technologies (AMTs), a group of integrated hardware-based and software-based solutions for the design, manufacture or handling of products (Organisation for Economic Co-operation and Development [OECD] Citation2012). While traditional manufacturing technologies enhance process efficiency mostly through rigid and mechanised design, AMTs improve the overall effectiveness of production systems. Computer-integrated manufacturing, flexible manufacturing systems, computer-aided design and computer-aided manufacturing networks are classic instances of these technologies. Early adoption of AMTs dates back to the 1970s, spread widely in the 1980s and has since penetrated most manufacturing activities, with varying degrees of intensity and of complexity. These technologies enable higher flexibility in the design of new products, faster delivery and greater product variety at low cost (Nemetz and Fry Citation1988; Parthasarthy and Sethi Citation1992). Thereby AMTs are not only a means to improve performance in the existing remit but also a vehicle to explore new growth paths such as expanding the product range and contesting new markets (Lei and Goldhar Citation1990).
Flexible organisation designs that enable quick responses to emerging opportunities or to a changing competitive landscape are deemed most effective to reaping the benefits of AMTs (Leonard-Barton Citation1988). Lei, Hitt, and Goldhar (Citation1996) identifies key organisational features for the efficient implementation of AMTs: cultivation of new sources of tacit, organisation-embedded knowledge; cross-functional integration and coordination; flexibility in cooperating with other organisations within the value chain. Crucial to the effective implementation of AMTs, and common to all the above features, stands tacit knowledge, that is, the know-how possessed by individuals or teams that have long-standing experience of working with specific equipment over extended periods (Nonaka Citation1991; Itami Citation1987; Dougherty Citation1992). Since tacit knowledge is highly specialised and sticky, the loss of workers who master this type of know-how represents a potential hazard for productivity and innovation (Badaracco Citation1991; Nonaka Citation1991). To illustrate, workflows and routines that have been adapted to accommodate process or product modifications are likely to be firm specific, and to rely on cross-functional pathways that have consolidated over repeated iterations (Lawler, Mohrman, and Ledford Citation1992). Technical tasks like materials handling, coding and calibrating largely depend on personal insight, emerging heuristics and direct experience with equipment. Further, the cross-functional integration of design and production activities is especially important in AMT-intensive environments that rely on continuous feedback loops between management, engineering and the factory floor (Lei, Hitt, and Goldhar Citation1996).
These peculiar characteristics of AMTs, and the challenges that their deployment entail for the skill base, resonate with the previous discussion on the nature of blue-collar routine tasks. Production environments characterised by a high degree of complexity require high levels of tacit know-how, experience and repeated interaction across different functional domains. Routine workers possess these skills and are therefore crucial to incremental experimentation and problem solving that stand at the core of innovation. By the same token, we conjecture that the prolonged decline of demand for routine jobs observed in the United States has undermined the ability to innovate in organisational ecosystems that revolve around AMTs. These issues have been largely ignored by the extant literature, and our empirical analysis will tackle them to fill the gap.
Accordingly, our second hypothesis is:
H2: De-routinisation is associated with decreasing innovation performance in the domain of Advanced Manufacturing Technology.
3. Data and methods
To investigate the relationship between the de-routinisation of employment and innovation capacity in Advanced Manufacturing Technologies, we collect data on occupational tasks, employment, industrial structure and patenting at the US Metropolitan Statistical Area (MSA) level. According to the US Office of Management and Budget (OMB, Citation2010 -->), MSAs are statistical areas ‘associated with at least one -->urbanized area that has a population of at least 50,000’.Footnote3 The OMB further specifies that MSAs comprises a central county (or counties) and adjacent counties with a high degree of economic and social integration (measured through commuting flows). The OMB reviews the standard for delineating the areas every ten years, and constantly revises the delineations to reflect estimates of US Census Bureau population and commuting flows. This implies that the composition and the identification codes of MSA may vary over time. Moreover, some areas may disappear, due i.e., to loss of population below the reference threshold, while new ones may emerge. To ensure comparability and consistency of territorial units over time and across different data sources, we create a crosswalk that allows the unique identification of MSAs over changing county composition. We exclude newly identified areas when the county composition is not identifiable in prior years unambiguously. MAs divided into two or more areas by the OMB revisions have been re-aggregated. This procedure allows us to identify 290 coherent MSAs over time that are the main unit of analysis.
Data on employment, skills, patents and economic and demographic factors come from different data sources. To construct the indicators of the occupational structure, we rely on the Occupational Employment Statistics (OES) programme --> from the U.S. Bureau of Labour Statistics (BLS), which provides annual employment data by occupation profiles for each MSA. OES-BLS does not provide data for the 1990s. Occupational task data are available only for the 1990 from the decennial census programme provided by IPUMS USA. Therefore, due to data availability limitation, we restrict the construction of our occupational structure indicators at the first available years, i.e.: 1990 and 2001. The most basic geographic unit identified in IPUMS USA census data is the Public Use Microdata Area (PUMA). In order to map PUMAs to MSAs, we exploit the PUMA detailed county composition to develop a crosswalk.Footnote4 We use the US Census Bureau County Business Pattern (CBP) to collect data on the number of establishments and the level of employment by sector of activity (SIC and NAICS codes). The source for county population data is the US Census Bureau, which also provides data on counties land through the Gazetteer Files.Footnote5 We then aggregate this data at the MSA level using the crosswalk mentioned above. Patent data are from the USPTO Patents View Database.Footnote6 Our analysis covers 290 MSAs over the period 1990, 2001–2012.Footnote7
3.1. Variables
Dependent variable: Our goal is to investigate the association between de-routinisation of local labour markets and local innovation capacity, with a focus on the AMTs domain. To identify AMT-related technologies, we collect information on patents issued in new digital technologies related to manufacturing processes. In particular, we exploit the Cooperative Patent Classification (CPC) scheme that provides, for each patent, a list of technological classes encompassing specific technological domains.Footnote8 To properly select domains related to AMTs, we rely on two main sources. The technical report by Aschhoff et al. (Citation2010) provides a list of IPC classes referring to key enabling technologies and identifies the classes strictly related to AMTs.Footnote9 The report by Ménière, Rudyk, and Valdes (Citation2017) focuses instead on technologies associated to the so-called 4th Industrial Revolution. Among these, we select those strictly connected to manufacturing systems, together with their corresponding CPCs, and add them to the former list. To assign patents to MSAs we rely on information on inventors’ addresses,Footnote10 ending up with the number of patents in AMTs, yearly for each MSA. Our main dependent variable thus is given by the percentage change of local AMTs patents from 2002 to 2012.
De-routinisation index: Our main variable of interest is an index of de-routinisation of local employment, which we build following the occupational task-based framework (Autor, Levy, and Murnane Citation2003; Autor and Dorn Citation2013). We focus on changes in the Metro-Area intensity of routine job employment. The reader will recall that the prototypical mid-skill routine job entails performing repetitive cognitive (i.e., clerks) or manual (i.e., blue-collar) work tasks. To illustrate, routine cognitive tasks are bookkeeping and data entry typical of ‘Office and Administrative support’ occupations while routine manual occupations in ‘production’, ‘maintenance and repair’ entail monitoring activities on the factory floor. The construction of a de-routinisation index requires several steps. First, we merge job task requirements to their corresponding occupation classification to assign task-intensity to individual job titles. Occupations are then identified as routine intensive based on their relative task-intensity as in Acemoglu and Autor (Citation2011). Next, using the OES BLS occupational employment data and IPUMS census data, we calculate the routine employment share for each MSA as follows:
where is the routine employment share in MSA
at time
;
is total employment of occupation
in MSA
at time
and
is an indicator function equal to
if occupation
is routine intense. Our index thus consists in the difference between the share of employment in routine-intensive jobs between two periods. In our preferred specification, also due to data availability, we calculate this difference between 1990 and 2001 (i.e. the index increases the higher is the reduction in the MSA
routine-job intensity during the 1990s). Formally, we define the index
as follows:
Our expectation is that MSAs that experienced a stronger decline in routine-intensive jobs during the 1990s did suffer in terms of innovative performance in digital manufacturing technologies in the 2000s. Moreover, we split routine jobs between routine cognitive and routine manual to test for possible differential associations between changes in these two categories and local innovation in AMTs.
3.2. Empirical strategy
To investigate the relationship between employment de-routinisation and innovative efforts in AMTs at the MSA level, we first estimate the following model:
where is the percentage change in the number of AMT patents for region
between 2012 and 2002, defined as
Footnote11
;
is the change of employment share in routine jobs for region
between 1990 and 2001, defined as
Footnote12 ;
is the error term and
comprises controls for local factors, measured in 1990, that may affect the capacity of an MSA to patent in AMTs. First, we control for the employment share in high-skilled (abstract) occupations. To calculate the share of employment in high-skill (abstract) jobs at the MSA level, we rely on the ALM task-based framework, then following the same procedure adopted for the share of routine occupations described in Section 3.1. We begin with the identification of abstract-intensive occupations. Typical of professional, managerial, technical and creative occupations, abstract tasks require intuition, creativity and problem solving, and are performed by workers possessing high levels of education and analytical capabilities. Then, by applying formula (1) to abstract-intensive occupations and the employment levels in those occupations, we derive our high-skill employment share at the MSA level. By focusing on skills and tasks rather than just education attainments, the high-skill employment share offers a more precise indicator of human capital, better capturing the role of high-skilled workers for innovation. Second, we include the MSAs’ number of firms operating in the manufacturing sector, to control for the role of the industrial structure on local innovation capabilities. We also control for the local existing innovation capabilities by including the MSAs total number of patents in 1990. This last variable should control, at least partially, for decreasing returns to scale in innovation activities. Lastly, we include a control for the level of population density measured in 1990. Since MSAs show high variability in terms of population and, importantly, patenting capacity, we weight all regressions by the local per-capita level of patenting in 1990 to assign a relatively lower weight to observations with the highest patenting variance (i.e., smaller MSAs). Standard errors are clustered at the State level to account for possible spatial correlation across MSAs.Footnote13
As anticipated in Section 3.1, we split our occupational routine measure into routine cognitive () and routine manual (
) and calculate two de-routinisation indexes accordingly (
and
, respectively). Therefore, we estimate the following model:
To complement the analysis, we also estimate models in Equationequations 3(3)
(3) and Equation4
(4)
(4) on the local percentage change in all patents (ALL). Moreover, we also focus on the association between long-run changes in the local endowment of routine jobs and the generation of incremental inventions, both overall and AMT-related in particular.
4. Empirical analysis
4.1. Descriptive statistics
synthetically describes the main variables employed in the empirical analysis, while reports the descriptive statistics. provides a first glimpse of the association between the documented loss of routine-skill workers during the 1990s and AMTs innovative capability that we will explore in detail. The diagram plots the growth rate of AMT patents between 2002 and 2012 against our index of de-routinisation as per section 3.1. Each dot represents an MSA, the size being proportional to the total number of patents in 2001 in the Metro Area. shows that the raw correlation between the change in the endowment of routine workers and AMT patenting is negative. This suggests that areas characterised by higher losses in the shares of routine-skilled workers in 2001 with respect to 1990 (i.e., a positive value in the de-routinisation index) also experienced a higher decline of AMT patenting during the 2000s, especially areas with lower patenting intensity. This is an initial hint that local labour markets wherein routine-intensive jobs prevailed were more exposed to labour-for-capital substitution and job polarisation after the uptake of automation in the mid-1990s. In turn, the declining demand for routine jobs and the associated lower endowment of mid-skilled workers is negatively associated to MSAs’ patenting in AMTs.
Table 1. Variables description
Table 2. Summary Statistics
Figure 1. The relationship between the change in routine employment share between 1990 and 2001 (de-routinisation index) and the percentage growth rate in AMT patenting between 2002 and 2012, by MSA
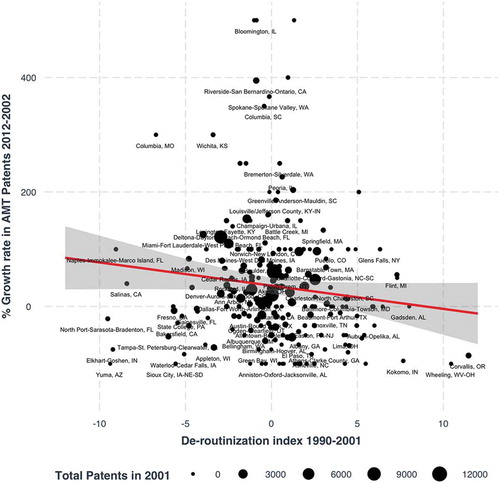
depicts the spatial distribution of changes in local AMTs patenting (our dependent variable) and the de-routinisation index (our main explanatory variable). Each MSA is coloured according to the weighted-quintile rank in the distribution of the relevant dimension. The colour scale in panel (a) indicates the MSAs with larger declines in the number of AMT patents between 2002 and 2012. The figure reveals significant geographical variation, with higher concentration of declining areas around the rust belt. The geographical distribution of changes in the routine employment share is presented in panel (b). Darker colours indicate metropolitan areas that experienced higher losses in terms of routine jobs during the 2000s.
Figure 2. Geographic distribution of the percentage change in AMTs patenting from 2002 to 2012 (panel a), and the de-routinisation index from 1990 to 2001 (panel b)
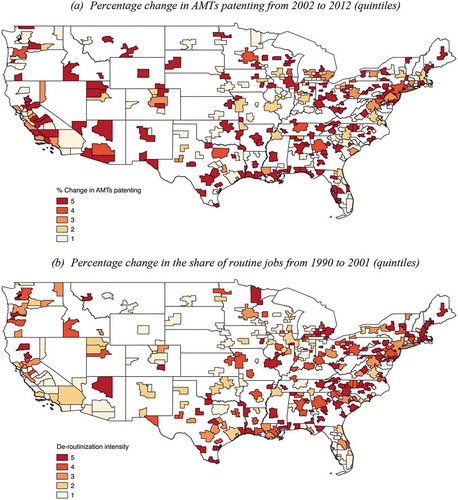
4.2. Regression analysis
4.2.1. Baseline models
This section presents the results of the econometric analysis of the long-term relationship between local changes in routine skills and local innovation capacity. reports estimates for the percentage change in local patenting over the period 2002–2012. Columns I and II refer to changes in AMT patenting, while columns III and IV refer to changes in total patenting (ALL). All the models include the full set of controls as described in Section 3.2 and are weighted by the 1990 per-capita number of local patents. Standard errors are clustered at the State level to account for possible spatial correlation across MSAs.
Table 3. De-routinisation and innovation (AMTs and Total patents)
Focusing on the results reported in , the decrease in routine-intensive employment does not reach statistical significance on local innovative capacity in AMTs (column I), while we find a negative association between the decrease in routine-intensive occupations and local innovative capacity when focusing on total patenting. In particular, our estimates indicate that a 1% decrease in routine-intensive employment (∆RSH) during the 1990s is associated with a 1.8% decrease in total patenting between 2002 and 2012 (column III). In line with expectations, we find a strong and negative association between losses in manual routine jobs on the percentage change of AMT patenting. Indeed, as reported in column II, the reduction in the share of blue-collar workers (ΔRMSH) is associated with a 4.7% decrease in local innovative capacity in AMTs. For what concerns the share of clerical workers (ΔRCSH), changes in local innovative capability in this domain seems to be not responsive to drops in the share of routine-cognitive workers. Likewise, we find that total local inventive activity is correlated more to changes in the employment of repetitive manual work (i.e., blue-collar jobs) than in routine cognitive jobs (i.e., clerks). Thereby, as reported in column IV, we estimate a negative and significant coefficient for the reduction in ΔRMSH, while the coefficient for ΔRCSH is not statistically significant. In this case, the magnitude of the ΔRMSH coefficient is at −2.8%, lower than the coefficient estimated when considering changes in local AMTs patenting. Turning to the control variables, we find positive and significant coefficients for the share of high-skill workers and for the number of manufacturing firms. Conversely, we find a negative coefficient for the total level of patenting in 1990 that likely reflects decreasing returns to scale in innovation. Lastly, the control for population density is not statistically significant.
As discussed in the theoretical section, prior studies report that de-routinisation is rooted in the so-called job polarisation effect of technology on labour demand. Accordingly, if, on the one hand, technology substituted for routine tasks, on the other hand it increases the demand for high and low skill jobs. Thus, the de-routinisation process and the consequent decrease in the share of routine-intensive occupations might have resulted in an increase in the share of high-skilled employment. In our empirical framework, this implies that controlling for the share of high-skilled employment might, in principle, bias the estimated association between the change in routine-intensive employment and the local innovation capacity. Though measuring the high-skilled employment share in 1990 should limit the potential bias, we also adopt a more conservative approach and estimate the baseline model excluding the share of high-skilled occupations from the set of control variables.Footnote14 Results are reported in and are consistent with respect to the baseline analysis summarised by . Precisely, the ∆RSH coefficient is at ~ −2.9% when considering changes in total patenting, while it does not show statistical significance in the case of AMTs. Importantly, we fully confirm the prominent association between drops in ΔRMSH and drops in patenting activity, more pronounced in the AMT case (−5.8%, column II) than for overall innovation (−3.7%, column IV). Moreover, we also estimate a negative coefficient for ΔRCSH in column III (−2.4%). As for the control variables, we find positive and significant coefficients for the number of manufacturing firms, and negative coefficients for the number of total patents at the starting point of the series. Lastly, coefficients for population density show tiny statistical significance only in column III.
Table 4. De-routinisation and innovation (AMTs and Total patents)
4.2.2. Incremental innovation
As presented in Section 4.2.1, the long-run reduction of routine workers is associated with decreasing innovative performances at the local level, especially in AMTs, confirming our hypothesis that routine workers’ tasks and their attending skills are important for productivity and innovation. Indeed, as discussed in Section 2, the necessary ‘downstream’ incremental improvements that stand at the core of innovation can be ascribed to the tacit know-how of routine workers and their increasing importance as ‘knowledge-bridges’ across modern factories functional departments. While our distinction between technology domains helps identifying the association between de-routinisation and AMT innovative efforts, the minor ‘downstream’ improvements of factory-floor workers, though their cumulative effects can be massive, are still incremental in nature, regardless of the technological domain to which they pertain. Accordingly, we complement the main analysis by looking at incremental innovations to better understanding the role of routine workers on innovative capabilities.Footnote15
To identify patents related to incremental innovations, several steps are required. Prior empirical literature exploiting patent data has primarily focused on radical and breakthrough innovation. Few studies dealt with the identification of incremental innovations using patent data (Dutta and Weiss Citation1997; Katila Citation2000), relying on ad hoc patent radicalness measures to distinguish between radical and incremental inventions (Ahuja and Morris Lampert Citation2001; Dahlin and Behrens Citation2005). For example, Wu et al. (2009) use patents received citations as a radicalness measure and consider incremental inventions those patents that belong to the top 3% of patent forward citation frequency.
For the purposes of the present subsection, we exploit the radicalness measure proposed by Squicciarini, Dernis, and Criscuolo (Citation2013) – OECD radicalness henceforth – that relies on patent backward citations. The authors compute such a measure among a set of different patent quality indicators for both EPO and (only recently) USPTO patents (the ‘OECD Patent Quality Indicators database, January 2020’). Following the measure originally proposed by Shane (Citation2001 -->), according to Squicciarini, Dernis, and Criscuolo (Citation2013): ‘ … the radicalness of a patent is measured as a time invariant count of the number of IPC technology classes in which the patents cited by the given patent are, but in which the patent itself is not classified. […] the more a patent cites previous patents in classes other than the ones it is in, the more the invention should be considered radical, as it builds upon paradigms that differ from the one to which it is applied’ [p. 53]. The radicalness index is, therefore, a continuous indicator, ranging from 0 to 1, that ‘underlines the dispersion of technology classes in the backward citations and the extent to which they differ from the focal patent’ [p. 56]. We identify patents belonging to the top 3% in the distribution of OECD radicalness by patent cohort (patent filing year) and main technology field (Schmoch Citation2008), and define them as radical patents. On the other hand, patents below this threshold (bottom 97% by field/cohort of OECD radicalness) are considered ‘incremental’.Footnote16
reports the results of the econometric analysis on the association between de-routinisation and local incremental innovative capabilities. It reports the estimates for the percentage change in incremental patenting over the period 2002–2012, where columns I and II refer to changes in AMT incremental patents and columns III and IV to total incremental patents. In all the specifications we include the full set of control variables as described in Section 3.2. Regressions are weighted by the 1990 per-capita number of local patents and standard errors are clustered at the State level.
Table 5. De-routinisation and incremental innovation (AMTs and Total patents)
We find a negative association between the decrease in routine-intensive occupations and local incremental patenting. In particular, a 1% decrease in routine-intensive employment (∆RSH) during the 1990s is associated with about 2.1% decrease in incremental patents between 2002 and 2012 (column III). While statistically insignificant, the negative magnitude of the coefficient increases to −3.1% when considering AMT incremental patenting (column I). Similar to the findings of the previous subsection, also in this case our dependent variables are more responsive to changes in the employment share of routine-manual workers than to changes in repetitive cognitive jobs (columns II and IV). In particular, we find a negative and significant coefficient for a 1% reduction in ΔRMSH at about −5% when AMT incremental patenting is under scrutiny (column II), and at about −2.9% when considering total incremental patenting changes (column IV). The coefficient for ΔRCSH is instead not statistically significant in both cases.
As for the control variables, our estimates return coefficients qualitatively in line with those already discussed in Section 4.2.1. Precisely, we document positive associations between the share of high-skill occupations and local patenting performances, the opposite for the total number of patents in 1990, and insignificant coefficients for population density.
4.3. Robustness checks
This section presents selected robustness checks. First, we test the robustness of our results to a different structure of our control variables. In accordance with our main explanatory variables, we express control variables in terms of percentage changes between 1990 and 2001, with the exception of population density. In so doing we control for the potential association between de-routinisation and de-industrialisation and to account for the contraction/expansion of the local industrial production. Results are reported in Table A1 in the Appendix and highly confirm our main findings. First, we estimate a negative and significant coefficient for ΔRSH on both AMT (column I) and total patenting (column III). Negative changes in local patenting associated with a drop in routine jobs are more pronounced for AMTs (−7.2%) than for the average patenting activity (−3.7%). Second, we find negative and statistically significant coefficients for the drop in ΔRMSH and ΔRCSH, both on total and AMTs local patenting, with changes correlated more to the reduction in the employment share of repetitive manual works (i.e. blue-collar jobs) than to the drop in routine cognitive jobs (i.e. clerks). Again, for both types of routine occupations, we estimate larger coefficients in the case of local AMT patenting.
Then, we test the robustness of our empirical analysis to different specifications of the intervals over which the percentage change in local patenting in AMTs is calculated. To this end, we compute the rate of patenting growth over three 3-year time-windows (i.e., 2002–2005, 2005–2008 and 2008–2011) to incorporate intermediate information on MSAs patenting performance during the decade 2002–2012. Here our main dependent variable is the average growth rate of AMT patents over the three periods. Results, reported in Table A2 in Appendix A, confirm the robustness of our main findings reported in , columns I and II and in , columns I and II.Footnote17 In particular, we find that a 1% decrease in the share of routine workers is associated with a significant decrease of about 1.8% of the average three-year growth in AMT patents (column I). The estimated coefficient raises in magnitude to −2.1% when we exclude the 1990 share of high-skill workers from the set of control variables (column III). The higher responsiveness of AMTs to changes in the employment of routine-manual workers (with respect to changes in routine-cognitive jobs) is also confirmed. Indeed, as reported in column II, we estimate a negative and statistically significant coefficient of ~ −4.1% for ∆RMSH, while ∆RCSH, though negative, is not statistically significant. Similar results are reported in column IV, where we estimate a coefficient for ∆RMSH at around −4.4%.
As a third robustness test, we add two additional controls to our main specification. The first concerns the impact of labour flexibility on productivity and, in turn, on innovation. According to prior studies (see i.e. Arvanitis Citation2005; Lucidi, and Kleinknecht 2009), intensive reliance on temporary contracts, a proxy of labour flexibility, can potentially hamper the accumulation of tacit and firm-specific knowledge thus reducing innovative competences (Kleinknecht, Van Schaik, and Zhou Citation2014). From an empirical point of view, Cetrulo, Cirillo, and Guarascio (Citation2019) focus on five major European economies between 1998 and 2012, finding a negative correlation between temporary contracts and product innovation. In order to account for the potential negative effect of temporary employment, we add to the set of control variables also the share of temporary workers in 1990. Unfortunately, an explicit measure of temporary workers is not available for the years covered by our analysis. Therefore, we exploit the IPUMS USA census data and collect data on the weeks worked by individuals in 1990. Precisely, we proxy temporary workers by considering those individuals that worked a number of weeks that falls below the average number of weeks nationally worked. Then, we calculate the share of those workers at the MSA level in 1990 as a control variable for the role of temporary workers. The second variable we add concerns the share of highly educated workers in 1990. While the inclusion of the share of high-skilled (abstract) employment should largely account for the fundamental role of human capital, as mentioned in Section 3.2, we substitute this variable with a more direct proxy for the level of education of the local workforce. Again, we collect data from the IPUMS USA census database and calculate the share of employed individuals with at least one year in college for each MSA. In turn, our control is given by the share of these workers in 1990. Table A3 in the Appendix reports the results when including the two further control variables described above. Here the focus is only on changes in AMT patenting.Footnote18 Results of these robustness checks are consistent with the main results. Reductions in repetitive manual occupations is in fact negatively correlated (∆RMSH), with robust statistical significance, with the local generation of AMTs. As for the share of temporary workers, we find negative and significant coefficients in all the model specifications, qualitatively in line with previous empirical evidence. On the contrary, as expected, the coefficients for the share of highly educated workers turn out to be positive and significant. Again, the presence of high-skill worker is central for innovation performances.
Lastly, we test the robustness of our measure of incremental patents. First, we calculate our dependent variables using an alternative measure of incremental patents. To this end, following Wu et al. (2009), we define ‘incremental’ the complement to the patents in the top 3% distribution of forward citations in a five-year window since filing, by patent cohort and main technology field. We express then the dependent variables as the percentage variation in the number of incremental AMT patents and incremental total patents between 2002 and 2012, respectively. Table A4 in the Appendix reports the results of the estimation using this alternative measure of incremental patents. The results are qualitatively similar to those presented in . In fact, we find a negative and significant coefficient for ∆RMSH, stable across specifications. Second, we run additional estimates to further check the robustness of our measure of incremental patents. Precisely, we test whether results hold when excluding from the radicalness measure, alternatively, the top 1%, 5% and 10% most cited patents (by cohort/technological field). The results of these tests confirm the robustness of the main analysis and are available upon request.
5. Concluding remarks and the way ahead
The relationship between technological change and labour dynamics has received much attention in the last decades. Grounded on the seminal contributions by Smith, Marx and Ricardo, a new wave of theoretical and empirical literature has been stimulated by the well-known ICT revolution that gained momentum in the late 1990s. On the one hand, these studies aimed at assessing the extent to which compensation effects could have offset substation ones, generating a net positive impact. On the other hand, it has become clear that the increasing diffusion of computers in production processes has affected workers in different ways, depending on the kind of skills. The new recent wave of technological change in the digital domain has renewed the interest in the relationship between innovation and labour markets. The peculiarities of digital technologies and their larger scope of applications has stimulated new theoretical efforts towards a framework that better accommodates their manifold nuances and pathways of impact. Starting from a dissatisfaction with the traditional model of human capital, the new framework shifts the emphasis to occupations and their skill content. This has proven effective in accounting for the intrinsic heterogeneity of both capital and labour, as well as the potential related to the expansion of the set of tasks produced by capital (Acemoglu and P. Restrepo Citation2019).
The debate on the socio-economic implications of digital innovation revolves mostly around the effect of automation and digitalisation on the demand for mid-skilled workers. The literature has widely documented increasing labour market polarisation, that is, growing labour demand at the two extremes of the skill distribution accompanied by declining demand for occupations in the middle. The debate that has ensued from the seminal findings of Autor, Levy, and Murnane (Citation2003) for the US and of Goos and Manning (Citation2007) for the UK has enlarged the geographical scope of the study of polarisationFootnote19 but has arguably neglected the impact that the loss of middle-skill routine human capital could have on innovation.
This gap is noticeable especially in consideration of the vast literature about the importance of tacit knowledge, learning-by-doing and practical know-how skills that are crucial for incremental innovation (Rosenberg Citation1976). The gap is also evident when one turns to the debate among practitioners. Due to the radical transformations that led to job polarisation, therein including technology and trade, manufacturing is changing driven by the integration of highly flexible, data-enabled, and cost-efficient processes that hold the promise of boosting competitiveness and opening new avenues for innovation. The modern factory powered by Advanced Manufacturing Technology relies on a large volume and frequency of information that can achieve higher precision, responsiveness and diversification. As usual, the higher complexity of the technology calls upon the adaptation of the skills base (Vona and Consoli Citation2015). In the case at hand, reaping the full benefits of AMTs is contingent to the availability of programming, monitoring and troubleshooting skills to handle and respond to the growing intensity and variety of feedback loops. Put otherwise, in the current stage of the life cycle, the new technology requires a new generation of blue-collar workers – or ‘new-collar’ as per the industry jargonFootnote20 – that can program, operate and maintain an ensemble of computer- and network-driven devices. This equipment has proliferated in manufacturing just as many traditional routine factory jobs have been outsourced or supplanted by the early wave of automation. The message stemming from industry experts is clear: finding, creating and retaining this kind of workers has become a critical bottleneck (Accenture and Manufacturing Institute 2014; Muro et al. Citation2015; Deloitte and The Manufacturing Institute Citation2018).
These shortcomings motivated our study on whether and to what extent the loss of routine workers, which is the trademark indicator of job polarisation, is related to the local capacity to innovate. This is clearly a complex issue that will require further research. . The present paper opens a novel avenue by focusing on the relationship between de-routinisation and innovation in AMTs in US Metropolitan Statistical Area during the period 2002–2012. The empirical analysis indicates that, on average, the loss of routine-intensive jobs is a negative predictor of local innovative capacity. In particular, we find that a 1% decrease in routine intensive occupations during the 1990s is associated to some 1.8% reduction in local total patenting during the 2002–2012 period. Further, we observe negative associations between innovation and both kinds of routine macro-tasks – repetitive cognitive and repetitive manual – with the latter showing more pronounced magnitudes. This result is larger for AMTs than the average total patenting activity. A 1% reduction in routine manual occupations is in fact significantly associated to some 4.7% reduction in local AMT patenting. Finally, we also document a negative association between de-routinisation and local incremental innovations, especially in AMTs again. Those results are robust to several tests on the construction of both dependent and independent variables, and to different econometric strategies.
Our study contributes the current debate on the relationship between technological change in the digital domain and economic performance. No doubt, compensation and substitution effects are important to grasp how technologies shape labour market dynamics. It goes without saying that organisational adaptations are a key, if understudied, ingredient in the mix. At the same time, our results shed light on a different, hitherto ignored aspect, namely that in the long-run job polarisation may jeopardise the inventive process, especially those incremental innovations based on learning-by-doing and the accumulation of on-the-job know-how. While radical innovation is important for opening up new paradigms, incremental innovation is essential to consolidate technological trajectories by ensuring continuous improvements within a paradigm. From an evolutionary viewpoint, an implication of our results is that routinisation may undermine technological variety that, coming full circle, narrows future prospects for economic development based on innovation.
These considerations call for further reflections concerning the coordination between innovation, industrial and labour policies. Currently, in many countries these three realms converge towards the creation of an environment favourable to the massive diffusion of digital technologies in production processes. However, especially labour policies should help preserve routine jobs because of their key contribution to the incremental innovation process, which is fundamental in ‘normal science’ periods. We hope that our initial exploration will lay the ground for future empirical research.
Davide Consoli acknowledges the financial support of the Ministerio de Economía, Industria y Competitividad ‘Programa Estatal de I+D+i Orientada a los Retos de la Q2 Sociedad, 2017ʹ (ECO2017-86976-R). Fabrizio Fusillo, Gianluca Orsatti and Francesco Quatraro gratefully acknowledge the funding of the Italian Ministry of University and Research, within the context of the PRIN research project n. 20177J2LS9].
No potential conflict of interest was reported by the authors. -->
Notes
1 As Cortes, Jaimovich, and Siu (Citation2017) point out, the issue is far from being settled for what concerns, first, the workings of the process by which routine occupations have declined and, second, the magnitude of aggregate decline of routine employment that can be ascribed to progress in automation technology. For critical views on polarisation, see Mishel, Shierholz, and Schmitt (Citation2013) and Hunt and Nunn (Citation2017).
2 The larger and larger implementation of specific work practices, like job rotation or cross-functions networking, highlights the importance of learning and knowledge diffusion dynamics embedded in firms’ human resources (Ortega Citation2001; Askenazy and Caroli Citation2010).
3 The OMB 2010 report is available at https://www.govinfo.gov/content/pkg/FR-2010-06-28/pdf/2010-15605.pdf.
4 Available at https://usa.ipums.org/usa/volii/puma.shtml.
5 Available at https://www.census.gov/geo/maps-data/data/gazetteer.html.
6 Available at http://www.patentsview.org/download/. It is worth stressing that the distinction between incremental and radical innovation has no impact on the discussion on the reliability of patents as a proxy of innovation. Both incremental and radical innovations can be patented provided they satisfy patent offices’ criteria. An important stream of literature relies on the statistical analysis of information contained in patent documents to derive measures to distinguish between breakthrough and incremental innovations (Silverberg and Verspagen Citation2007; Castaldi and Los Citation2012; Castaldi, Frenken, and Los Citation2015).
7 Due to data availability at the MSA level, we collect information on employment, economic and demographic characteristics in 1990, and from 2001 to 2012. Patent data are collected for the period 1990–2012.
8 The CPC has been established in 2010 to harmonise individual classification systems between the USPTO and the EPO. We exploit the PatentsView database table ‘cpc_current’ to extract information on CPC classes for US patents.
9 For each IPC contained in Aschhoff et al. (Citation2010) we identify the corresponding CPCs using the concordance table by EPO and USPTO (https://www.cooperativepatentclassification.org/cpcConcordances.html).
10 Patents filed by multiple inventors residing in different MSAs are locally assigned according to the fraction of inventors residing in each MSA.
11 ranges between −200 and 200. In the main analysis, we apply this transformation to include also MSAs that did not patent in AMTs in 2002. According to our data, 65 MSAs did not patent in AMTs in 2002.
12 It is worth noticing that for each MSA , the stronger the decline in the employment share of routine jobs during the period 1990–2001, the higher the de-routinisation index. In other words, our de-routinisation index is higher in the MSAs that experienced higher losses in routine-intensive occupations.
13 Ideally, an empirical analysis of the period 1990–2012 would have been appropriate to control for time-invariant local area characteristics and for temporal structural conditions that affect all the MSAs. Unfortunately, as mentioned in Section 3, yearly information on the local skills composition for the decade 1990–2000 are not available. As an alternative, we ran a series of panel fixed effect regressions that exploit the longitudinal dimension of our data starting from 2001. The findings are qualitatively in line with our baseline results (see Section 4.2) and confirm the negative association with the loss in routine jobs and local patenting in AMTs. Table B1 in Appendix B reports the results. We wish to thank an anonymous referee for suggesting this further test.
14 We wish to thank an anonymous referee for this suggestion.
15 We wish to thank an anonymous referee for suggesting us to more formally extend the analysis to incremental innovation by exploiting more in-depth information contained in patent documents.
16 In Section 4.3 we provide robustness checks to this analysis by considering different index thresholds used to split patents between incremental and radical, i.e.: 1%, 5% and 10%. We also test an alternative measure, following Wu et al. (Citation2019), categorising incremental innovation in terms of patent forward citation frequency.
17 In additional robustness tests, we use the patenting average growth calculated over two 5-year time windows as dependent variable. Moreover, we also test whether results are robust to changing the starting year and the end year over which the percentage change in patenting is calculated, i.e.: 2002–2010, 2002–2011, 2003–2010 and 2003–2011. These tests yield robust results and are available upon request.
18 We perform the same tests also considering variations in overall patenting as dependent variable. Results are fully consistent with the main findings and are available upon request by the authors.
19 See i.e. Autor and Dorn (Citation2013) on US commuting zones; Senftleben-König and Wielandt (Citation2014) and Dauth (Citation2014) on German local labour markets; Malgouyres (Citation2017) on French commuting units; Consoli and Sánchez-Barrioluengo (Citation2019) on Spanish provinces.
20 See National Association of Manufacturers (NAM) 2018 State of Manufacturing Address: https://www.nam.org/Newsroom/Press-Releases/2018/02/Excerpts–NAM-2018-State-of-Manufacturing-Address
References
- Acemoglu, D. 2002. Technical Change, Inequality, and the Labour Market.Journal of Economic Literature 40 (1): 772. doi:https://doi.org/10.1257/jel.40.1.7.
- Acemoglu, D., and D. H. Autor. 2011. “Skills, Tasks and Technologies: Implications for Employment and Earnings.” In Handbook of Labour Economics, edited by O. Ashenfelter and D. Card, 1043–1171. Amsterdam, The Netherlands: Elsevier. Chap. 12. -->
- Acemoglu, D., and P. P. Restrepo. 2019. “Automation and New Tasks: How Technology Displaces and Reinstates Labour.” (No. w25684). National Bureau of Economic Research.
- Ahuja, G., and C. Morris Lampert. 2001. “Entrepreneurship in the Large Corporation: A Longitudinal Study of How Established Firms Create Breakthrough Inventions.” Strategic Management Journal 22 (6–7): 521–543. doi:https://doi.org/10.1002/smj.176.
- Al-Najjar, B. 2000. “Accuracy, Effectiveness and Improvement of Vibration-based Maintenance in Paper Mills: A Case Study.” Journal of Sound and Vibration 229 (2): 389–410. doi:https://doi.org/10.1006/jsvi.1999.2492.
- Alsyouf, I. 2007. “The Role of Maintenance in Improving Companies’ Productivity and Profitability.” International Journal of Production Economics 105 (1): 70–83. doi:https://doi.org/10.1016/j.ijpe.2004.06.057.
- Arvanitis, S. 2005. “Modes of Labour Flexibility at Firm Level: Are There Any Implications for Performance and Innovation? Evidence for the Swiss Economy.” Industrial and Corporate Change 14 (6): 993–1016. doi:https://doi.org/10.1093/icc/dth087.
- Aschhoff, B., D. Crass, K. Cremers, C. Grimpe, and C. Rammer. 2010. European Competitiveness in Key Enabling Technologies. Leibniz, German:Centre for European Economic Research (ZEW). -->
- Askenazy, P., and E. Caroli. 2010. “Innovative Work Practices, Information Technologies, and Working Conditions: Evidence for France.” Industrial Relations: A Journal of Economy and Society 49 (4): 544–565.
- Autor, D. H., and D. Dorn. 2013. “The Growth of Low-Skill Service Jobs and the Polarisation of the US Labour Market.” American Economic Review 103 (5): 1553–1597. doi:https://doi.org/10.1257/aer.103.5.1553.
- Autor, D. H., F. Levy, and R. J. Murnane. 2003. “The Skill Content of Recent Techno- Logical Change: An Empirical Exploration.” Quarterly Journal of Economics 118 (4): 1279–1333. doi:https://doi.org/10.1162/003355303322552801.
- Autor, D. H., L. F. Katz, and A. B. Krueger. 1998. “Computing Inequality: Have Computers Changed the Labour Market?.” The Quarterly Journal of Economics 113 (4): 1169–1213. doi:https://doi.org/10.1162/003355398555874.
- Badaracco, J. L. 1991. The Knowledge Link. Boston, MA: Harvard Business School Press.
- Barley, S. R. 1996. “Technicians in the Workplace: Ethnographic Evidence for Bringing Work into Organization Studies.” Administrative Science Quarterly 41 (3): 404–441. doi:https://doi.org/10.2307/2393937.
- Barley, S. R., and B. A. Bechky. 1994. “In the Backrooms of Science: The Work of Technicians in Science.” Work Occupations 21 (1): 85–126. doi:https://doi.org/10.1177/0730888494021001004.
- Bessen, J. (2018). AI and Jobs: The role of demand (No. w24235). National Bureau of Economic Research.
- Bradley, D., I. Kim, and X. Tian. 2017. “Do Unions Affect Innovation?.” Management Science 63 (7): 2251–2271. doi:https://doi.org/10.1287/mnsc.2015.2414.
- Castaldi, C., and B. Los. 2012. Are New ‘Silicon Valleys’ Emerging? the Changing Distribution of Superstar Patents across US States. In Danish Research Unit for Industrial Dynamics (DRUID) Summer Conference. Copenhagen, Denmark. -->
- Castaldi, C., K. Frenken, and B. Los. 2015. “Related Variety, Unrelated Variety and Technological Breakthroughs: An Analysis of US State-level Patenting.” Regional Studies 49 (5): 767–781. doi:https://doi.org/10.1080/00343404.2014.940305.
- Cetrulo, A., V. Cirillo, and D. Guarascio. 2019. “Weaker Jobs, Weaker Innovation. Exploring the Effects of Temporary Employment on New Products.” Applied Economics 51 (59): 6350–6375. doi:https://doi.org/10.1080/00036846.2019.1619015.
- Chennells, L., and J. V. Reenen. 1999. “Has Technology Hurt Less Skilled Workers? an Econometric Survey of the Effects of Technical Change on the Structure of Pay and Jobs.” (No. W99/27). IFS Working Papers.
- Cirillo, V. 2017. “Technology, Employment and Skills.” Economics of Innovation and New Technology 26 (8): 734–754. doi:https://doi.org/10.1080/10438599.2017.1258765.
- Consoli, D., and M. Sánchez-Barrioluengo. 2019. “Polarisation and the Growth of Low-skill Service Jobs in Spanish Local Labour Markets.” Journal of Regional Science 59 (1): 145–162. doi:https://doi.org/10.1111/jors.12409. -->
- Cortes, G. M., N. Jaimovich, and H. E. Siu. 2017. “Disappearing Routine Jobs: Who, How, and Why?.” Journal of Monetary Economics 91: 69–87. doi:https://doi.org/10.1016/j.jmoneco.2017.09.006.
- Dahlin, K. B., and D. M. Behrens. 2005. “When Is an Invention Really Radical? Defining and Measuring Technological Radicalness.” Research Policy 34 (5): 717–737. doi:https://doi.org/10.1016/j.respol.2005.03.009.
- Dauth, W. 2014. “Job Polarisation on Local Labour Markets.” IAB-Discussion paper 18/2014.
- Deivanayagam, S. 1992. “Designing for Maintainability: Computerized Human Models.” Computers & Industrial Engineering 23 (1–4): 195–196. doi:https://doi.org/10.1016/0360-8352(92)90096-3.
- Deloitte and The Manufacturing Institute. 2018. “Skills Gap and Future of Work Study.” https://bit.ly/2RkBN7d
- Dietz, R., and J. Orr. 2006. “A Leaner, More Skilled U.S. Manufacturing Workforce.” Federal Reserve Bank of New York Current Issues in Economics and Finance 12 (2): 1–7.
- Dougherty, D. 1992. “A Practice-centered Model of Organizational Renewal through Product Innovation.” Strategic Management Journal 13 (S1): 77–92. doi:https://doi.org/10.1002/smj.4250131007.
- Drucker, P. 1999. “Knowledge-worker Productivity: The Biggest Challenge.” California Management Review 41 (2): 79–94. doi:https://doi.org/10.2307/41165987.
- Dutta, S., and A. M. Weiss. 1997. “The Relationship between a Firm’s Level of Technological Innovativeness and Its Pattern of Partnership Agreements.” Management Science 43 (3): 343–356. doi:https://doi.org/10.1287/mnsc.43.3.343.
- Fernandez, R. 2001. “Skill-Biased Technical Change and Wage Inequality: Evidence from a Plant Retooling.” American Journal of Sociology 107 (2): 273–320. doi:https://doi.org/10.1086/324009.
- Freeman, C., & Soete, L. (Eds.). (1987). Technical change and full employment. Wiley-Blackwell.
- Goos, M., and A. Manning. 2007. “Lousy and Lovely Jobs: The Rising Polarisation of Work in Britain.” Review of Economics and Statistics 89 (1): 118–133. doi:https://doi.org/10.1162/rest.89.1.118.
- Goos, M., A. Manning, and A. Salomons. 2014. “Explaining Job Polarisation: Routine-biased Techno- Logical Change and Offshoring.” American Economic Review 104 (8): 2509–2526. doi:https://doi.org/10.1257/aer.104.8.2509.
- Gregory, T., A. Salomons, and U. Zierahn. 2016. “Racing with or against the Machine? Evidence from Europe.” ZEW-Centre for European Economic Research Discussion Paper, (16-053).
- Grinza, E., and F. Quatraro. 2019. “Workers’ Replacements and Firms’ Innovation Dynamics: New Evidence from Italian Matched Longitudinal Data.” Research Policy 48 (9): 103804. doi:https://doi.org/10.1016/j.respol.2019.05.013.
- Hayek, F. A. 1945. “The Use of Knowledge in Society.” The American Economic Review 35 (4): 519–530.
- Hoopes, D., and S. Postrel. 1999. “Shared Knowledge, Glitches, and Product Development Performance.” Strategic Management Journal 20 (9): 837–865. doi:https://doi.org/10.1002/(SICI)1097-0266(199909)20:9<837::AID-SMJ54>3.0.CO;2-I.
- Hunt, J., and R. Nunn. 2017. Why are Fewer Workers Earning Middle Wages and Is It a Bad Thing?. University of Connectictut, Storrs (US). -->
- Itami, H. 1987. Mobilizing Invisible Assets. Cambridge, MA: Harvard University Press.
- Katila, R. 2000. “Using Patent Data to Measure Innovation Performance.” International Journal of Business Performance Management 2 (1/2/3): 180–193. doi:https://doi.org/10.1504/IJBPM.2000.000072.
- Kleinknecht, A., F. N. Van Schaik, and H. Zhou. 2014. “Is Flexible Labour Good for Innovation? Evidence from Firm-Level Data.” Cambridge Journal of Economics 38 (5): 1207–1219. doi:https://doi.org/10.1093/cje/bet077.
- Klenert, D., E. Fernández-Macías, and J. Antón. 2020. Do Robots Really Destroy Jobs? Evidence from Europe. Seville: European Commission. JRC118393.
- Kukla, R. E. 1983. “Organizing a Manufacturing Improvement Program.” Quality Progress 16 (11): 28–31.
- Langowitz, N. S. 1988. “An Exploration of Production Problems in the Initial Commercial Manufacture of Products.” Research Policy 17 (1): 43–54. doi:https://doi.org/10.1016/0048-7333(88)90020-0.
- Lawler, E. E. I. I. I., S. A. Mohrman, and G. E. Ledford. 1992. Employee Involvement and Total Quality Management. San Francisco: Jossey-Bass.
- Lei, D., and J. D. Goldhar. 1990. “Multiple Niche Competition: The Impact of CIM Technology.” Manufacturing Review 3: 195–206.
- Lei, D., M. A. Hitt, and J. D. Goldhar. 1996. “Advanced Manufacturing Technology: Organizational Design and Strategic Flexibility.” Organization Studies 17 (3): 501–523. doi:https://doi.org/10.1177/017084069601700307.
- Leonard-Barton, D. 1988. “Implementation as a Mutual Adaptation of Technology and Organization.” Research Policy 17 (5): 251–267. doi:https://doi.org/10.1016/0048-7333(88)90006-6.
- Lewis, P. 2020. “Developing Technician Skills for Innovative Industries: Theory, Evidence from the UK Life Sciences Industry, and Policy Implications.” British Journal of Industrial Relations (Forthcoming), no. 3. doi:https://doi.org/10.1111/bjir.12532.
- Lewis, P. A. 2020a. “Technicians and Innovation: A Literature Review.” Gatsby Foundation Report. 10.2139/ssrn.3405406
- Malgouyres, C. 2017. “The Impact of Chinese Import Competition on the Local Structure of Employment and Wages: Evidence from France.” Journal of Regional Science 57 (3): 411–441. doi:https://doi.org/10.1111/jors.12303.
- Mckone, K., and W. Elliott. 1998. “TPM: Planned and Autonomous Maintenance: Bridging the Gap between Practice and Research.” Production and Operations Management 7 (4): 335–351. doi:https://doi.org/10.1111/j.1937-5956.1998.tb00128.x.
- Ménière, Y., I. Rudyk, and J. Valdes. 2017. “Patents and the Fourth Industrial Revolution.” European Patent Office, Munich, Germany.
- Michie, J., and M. Sheehan. 2003. “Labour Market Deregulation, `flexibility’ and Innovation.” Cambridge Journal of Economics 27 (1): 123–143. doi:https://doi.org/10.1093/cje/27.1.123.
- Mishel, L., H. Shierholz, and J. Schmitt. 2013. “Don’t Blame the Robots: Assessing the Job Polarisation Explanation of Growing Wage Inequality.” EPI–CEPR Working Paper, https://bit.ly/2U5txNK
- Muro, M., J. Rothwell, S. Andes, K. Fikri, and S. Kulkarni. 2015. “America’s Advanced Industries: What They Are, Where They Are, and Why They Matter.” Brookings. https://brook.gs/2bQHbtT
- Nemetz, P., and L. Fry. 1988. “Flexible Manufacturing Organizations: Implications for Strategy Formulation and Organization Design.” Academy of Management Review 13 (4): 627–638. doi:https://doi.org/10.5465/amr.1988.4307510.
- Nonaka, I. 1991. “The Knowledge-creating Company.” Harvard Business Review 69/6: 96–104.
- Office of Management and Budget. (OMB). (2010). Standards for Delineating Metropolitan and Micropolitan Statistical Areas. Federal Register.
- Organisation for Economic Co-operation and Development [OECD]. 2012. The Frascati Manual. Paris: OECD.
- Organisation for Economic Co-operation and Development [OECD] 2016. “ICT and Jobs: Complements or Substitutes? the Effects of ICT Investment on Labour Market Demand by Skills and by Industry in Selected OECD Countries.” Ministerial Meeting on the Digital Economy Technical Report, OECD Digital Economy Papers n.259, Paris.
- Ortega, J. 2001. “Job Rotation as a Learning Mechanism.” Management Science 47 (10): 1361–1370. doi:https://doi.org/10.1287/mnsc.47.10.1361.10257.
- Parthasarthy, R., and S. P. Sethi. 1992. “The Impact of Flexible Automation on Business Strategy and Organizational Structure.” Academy of Management Review 17 (1): 86–111. doi:https://doi.org/10.5465/amr.1992.4279572.
- Pianta, M. (2003) “Innovation and Employment”. In: Fagerberg, J. and Mowery, D.C. (Eds) The Oxford Handbook of Innovation. Oxford University Press.
- Piva, M., L. Grilli, and C. Rossi-Lamastra. 2011. “The Creation of High-Tech Entrepreneurial Ventures at the Local Level: The Role of Local Competences and Communication Infrastructures.” Industry and Innovation 18 (6): 563–580. doi:https://doi.org/10.1080/13662716.2011.591969.
- Piva, M., and M. Vivarelli. 2018. “Technological Change and Employment: Is Europe Ready for the Challenge?.” Eurasian Business Review 8 (1): 13–32. doi:https://doi.org/10.1007/s40821-017-0100-x.
- Riis, J., J. Luxhoj, and T. Uffe. 1997. “A Situational Maintenance Model.” International Journal of Quality and Reliability Management 14 (4): 349–366. doi:https://doi.org/10.1108/02656719710170620.
- Rosenberg, N. 1976. Perspectives on Technology. Cambridge: Cambridge University Press.
- Rosenberg, N., and W. E. Steinmueller. 2013. “Engineering Knowledge.” Industrial and Corporate Change 22 (5): 1129–1158. doi:https://doi.org/10.1093/icc/dts053.
- Schmoch, U. 2008. “Concept of a Technology Classification for Country Comparisons.” Final report to the world intellectual property organisation (wipo), WIPO.
- Senftleben-König, C., and H. Wielandt. 2014. “The Polarisation of Employment in German Local Labour Markets.” Working Papers 2014007, Berlin Doctoral Program in Economics and Management Science (BDPEMS).
- Shane, S. 2001. “Technological opportunities and new firm creation.” Management science 47(2): 205–220.
- Silverberg, G., and B. Verspagen. 2007. “The Size Distribution of Innovations Revisited: An Application of Extreme Value Statistics to Citation and Value Measures of Patent Significance.” Journal of Econometrics139 (2): 318–339. doi:https://doi.org/10.1016/j.jeconom.2006.10.017.
- Sohal, A., B. D’Netto, P. Fitzpatrick, and H. Noori. 2001. “The Roles and Responsibilities of Production/Operations Managers in SMEs: Evidence from Canada.” Technovation 21 (7): 437–448. doi:https://doi.org/10.1016/S0166-4972(00)00060-2.
- Squicciarini, M., H. Dernis, and C. Criscuolo. 2013. Measuring Patent Quality: Indicators of Technological and Economic Value.OECD Science, Technology and Industry Working Papers, No. 2013/03, OECD Publishing, Paris 10.1787/5k4522wkw1r8-en.
- Van Roy, V., D. Vrtesy, and M. Vivarelli. 2018. Technology and Employment: Mass Unemployment or Job Creation? Empirical Evidence from European Patenting Firms. Research Policy 47 (9): 17621776. doi:https://doi.org/10.1016/j.respol.2018.06.008.
- Vivarelli, M. 2014. Innovation, Employment and Skills in Advanced and Developing Countries: A Survey of Economic Literature. Journal of Economic Issues 48 (1): 123–154. doi:https://doi.org/10.2753/JEI0021-3624480106.
- Vivarelli, M., and M. Pianta (Eds.). 2000. The Employment Impact of Innovation: Evidence and Policy, 1–216. London: Routledge.
- Vona, F., and D. Consoli. 2015. “Innovation and Skill Dynamics: A Life-cycle Approach.” Industrial and Corporate Change 24 (6): 1393–1415. doi:https://doi.org/10.1093/icc/dtu028.
- Wachsen, E., and K. Blind. 2016. “More Labour Market Flexibility for More Innovation? Evidence from Employer-Employee Linked Micro Data.” Research Policy 45 (5): 941–950. doi:https://doi.org/10.1016/j.respol.2016.01.020.
- Waeyenbergh, G., and L. Pintelon. 2004. “Maintenance Concept Development: A Case Study.” International Journal of Production Economics 89 (3): 395–412. doi:https://doi.org/10.1016/j.ijpe.2003.09.008.
- Waeyenbergh, G., & Pintelon, L. (2002). A framework for maintenance concept development. International Journal of Production Economics77 (3): 299–313.
- World Bank. 2016. World Development Report 2016: Digital Dividends World Bank Group. Washington. -->
- Wu, J., K. R. Harrigan, S. H. Ang, and Z. Wu. 2019. “The Impact of Imitation Strategy and R&D Resources on Incremental and Radical Innovation: Evidence from Chinese Manufacturing Firms.” The Journal of Technology Transfer 44 (1): 210–230. doi:https://doi.org/10.1007/s10961-017-9621-9.
- Zhou, H., R. Dekker, and A. Kleinknecht. 2011. “Flexible Labour and Innovation Performance: Evidence from Longitudinal Firm-Level Data.” Industrial and Corporate Change 20 (3): 941–968. doi:https://doi.org/10.1093/icc/dtr013.
APPENDIX A
Table A1. Robustness I: De-routinisation and innovation (AMTs and Total patents)
Table A2. Robustness II: De-routinisation and AMT innovation
Table A3. Robustness III: De-routinisation and AMT innovation
Table A4. Robustness IV: De-routinisation and incremental innovation (AMT and Total patents)
APPENDIX B
Table B1. Panel data regressions