ABSTRACT
Part 1 of this study summarizes data for a field investigation of contaminant concentration variability within individual, discrete soil samples (intra-sample variability) and between closely spaced, “co-located” samples (inter-sample variability). Hundreds of discrete samples were collected from three sites known respectively to be contaminated with arsenic, lead, and polychlorinated biphenyls. Intra-sample variability was assessed by testing soil from ten points within a minimally disturbed sample collected at each of 24 grid points. Inter-sample variability was assessed by testing five co-located samples collected within a 0.5-m diameter of each grid point. Multi Increment soil samples (triplicates) were collected at each study site for comparison. The study data demonstrate that the concentration of a contaminant reported for a given discrete soil sample is largely random within a relatively narrow (max:min <2X) to a very wide (max:min >100X) range of possibilities at any given sample collection point. The magnitude of variability depends in part on the contaminant type and the nature of the release. The study highlights the unavoidable randomness of contaminant concentrations reported in discrete soil samples and the unavoidable error and inefficiency associated with the use of discrete soil sample data for decision making in environmental investigations.
Introduction
This paper summarizes the results of a field study that investigated the scientific underpinnings and reliability of discrete soil sample data for decision making in environmental investigations. A more detailed review of the study is presented in reports prepared by the Hawaii Department of Health (HDOH, rCitation2015a,Citationb). The term “variability” is used in a very general sense to describe largely random differences in contaminant concentration over very short distances at the scale of a typical discrete soil sample. The study was designed to answer three basic questions: 1) How variable is the concentration of a contaminant within randomly selected subsamples of an unprocessed discrete soil? (intra-sample variability), 2) How variable is the concentration of a contaminant between co-located, discrete samples collected within a short distance of each other? (inter-sample variability), and 3) What are the implications for reliance on discrete soil sample data to guide site characterization and remedial actions? The first two questions are addressed in Part 1 of this paper. The third question is explored in Part 2 (Brewer et al., Citation2016).
The use of discrete samples, or samples collected from individual points, to characterize suspected contaminated soil is based on two simple but important underlying assumptions (Gilbert, Citation1987; USEPA, Citation1987, Citation1989a): 1) the concentration of a contaminant in soil around a given sample collection point is relatively uniform at the scale of the mass of soil collected (e.g., 100–200 g), and more importantly, the mass of soil tested (e.g., 1–30 g) and 2) the concentration trend between individual discrete sample points is linear and predictable. These assumptions allow identification and mapping of large-scale contaminant concentration trends and even point-by-point decision making, such as comparison of data to published screening levels. Such uniformity also negates consideration of the mass of soil collected, as well as the precise location of the sample collection point. If true, these assumptions greatly simplify environmental investigations.
Surprisingly, the reliability of this assumption of uniformity has never been fully tested in the field, especially considering the hundreds of thousands of sites investigated based on discrete soil sample methodologies. The few studies available suggest quite the opposite—that the concentration of contaminants in soil at the scale of a discrete soil sample at a given point is, in fact, random. This issue is well studied for explosive-related contamination (e.g., Ramsey and Hewitt, Citation2005). The United States Environmental Protection Agency (USEPA) lab method for explosives (Method 8330B) includes a detailed discussion of representative procedures for both field sample collection and lab sub-sampling (USEPA, Citation2006). Research on contaminant variability and representative sampling or sub-sampling issues is less common for other types of soil contaminants. Clausen et al. (Citation2013) demonstrated improved data quality for metals in surface soil samples from three small-arm ranges when using Multi Increment sampling methods compared to conventional discrete or grab sampling. (The term “Multi Increment®” is trademarked by Charles Ramsey and EnviroStat, Inc.; see Ramsey and Hewitt, Citation2005.) Hadley and Bruce (Citation2013) provide a good overview of this issue and the risk of decision error when relying on discrete sample data to characterize, assess, and ultimately remediate an area of contaminated soil. Such problems are exemplified in the field by the need for repeated yet often inconclusive sampling efforts, confusion over seemingly isolated “hot spots” and “cold spots,” failed confirmation samples following remedial actions, questionable elimination of high-concentration “outlier” data for estimation of contaminant means, and the discovery of contamination at sites previously thought to be clean.
Discrete sampling methods were developed for testing relatively uniform industrial waste and similar media, rather than particulate matter like soil, where distributional heterogeneity of contaminants can be very high. This has led to a re-examination of sampling theory and the recent movement to “Decision Unit” (DU) and Multi Increment (MI) sample collection methods in order to obtain more representative and reliable sample data. The State of Hawaii began making the transition to DU-MI investigation methods in 2006 and first published comprehensive field and laboratory guidance in 2008 (later updated in 2016; HDOH, Citation2016). An introduction to Sampling Theory and “Incremental Sampling Methodology” was also recently published by the Interstate Technology and Regulatory Council (ITRC, Citation2012).
Anecdotal evidence and small studies of random, small-scale distributional heterogeneity of contaminant concentrations in soil is presented in several early USEPA guidance documents (e.g., USEPA, Citation1989b, Citation1990, Citation2003). Detailed published field studies of discrete sample variability and reliability for non-explosives-related contaminants are limited, however. This paper provides data to help fill this gap. Three sites with different types and releases of contaminants were selected for intensive discrete sample collection. Hundreds of samples were collected and analyzed at each site. The variability of contaminant concentrations both within individual samples and between co-located samples was then quantitatively evaluated and summarized, and the resulting implications for the reliance on discrete sample data to guide environmental investigations are outlined in Part 1 of this paper. Part 2 (Brewer et al., Citation2016) expands on the implications of reliance on discrete soil sampling methodology for environmental investigations.
Methods
Selection of study sites
Three sites, one on the island of Hawaii and two on the island of Oahu, were selected for the study (; refer to study reports for more detailed site locations; HDOH, Citation2015a,Citationb). Each site was known from previous investigations to be contaminated with arsenic, lead, and polychlorinated biphenyls (PCBs), respectively. An intentional effort was made to select sites that spanned an anticipated wide range of contaminant variability at the scale of a typical, discrete soil sample. Although the magnitude of variability was unknown, existing data suggested that variability was relatively low at the arsenic-contaminated site (Study Site A) and very high at the PCB-contaminated site (Study Site C). It was hypothesized, ultimately correctly, that variability at the lead-contaminated site (Study Site B) would fall somewhere in between. Reported levels of arsenic and lead in soil are well above the anticipated background, estimated to be up to 24 mg/kg for the former and 73 mg/kg for the latter (HDOH, Citation2012). Although not specifically evaluated as part of this study, it is expected that relative variability within contaminated areas would be significantly greater than within uncontaminated areas due to nugget and related effects.
Figure 1. Study site locations in Hawaii. Study Site A is on the Island of Hawaii. Study Sites B and C are on the Island of Oahu.
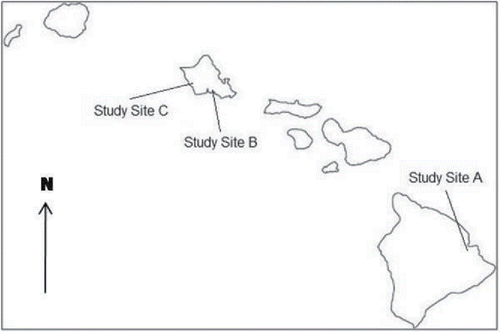
As described below, a 24-point grid was established at each study site and used to evaluate discrete sample variability (). Soil within an individual sample collected at each grid was tested multiple times in order to assess intra-sample variability. Closely spaced, “co-located” samples collected around each grid point were then individually processed and tested in order to assess inter-sample variability. The combined data were then used to estimate the total variability of contaminant concentrations in discrete sample-size masses of soil around each individual grid point.
Figure 2. Design of discrete soil sample collection for individual grid points. Left: Twenty-four point grid established for sample collection (Study Site B grid depicted). Upper right: Grid point sample collection design for Study Site A (arsenic) and Study Site B (lead); multiple XRF tests of Sample A used to assess intra-sample variability and individual testing of Samples A–E used to assess inter-sample variability. Lower right: Grid point sample collection design for Study Site C (PCBs); intra-sample variability tested by placing subsamples of the sixth sample in ten separate jars for individual testing and individual testing of Samples A–E (bags) used to assess inter-sample variability.
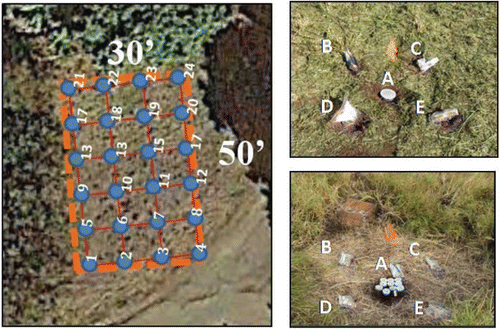
Study site A
Study Site A is an area of known arsenic-contaminated soil within a public park in Hilo on the Island of Hawaii. The park is adjacent to a stream- and spring-fed body of fresh to brackish water known as Waiakea Pond, which serves as an important estuary and ecological habitat. Past investigations had shown both the sediment in the pond and the immediately adjacent soil to be contaminated with arsenic (e.g., Hallacher et al., Citation1985; HDOH, Citation2013; Silvius et al., Citation2005).
Historical operations suspected to be tied to the arsenic contamination include a factory that converted sugarcane fiber to a ceiling and wallboard product, referred to as “Canec,” and a sugar mill, both formerly located on the upper, southern side of the pond (Bernard and Orcutt, Citation1983). The Hawaiian Cane Products plant in Hilo produced arsenic-treated building material from the 1930s through the 1960s. The arsenic served as a termiticide and preservative. Wastewater from the plant is believed to have been discharged directly into Waiakea Pond. Contamination of soil adjacent to the pond could be due to past disposal of dredge spoils, flooding, and/or direct discharges of wastewater from the plant. Arsenic-contaminated sediment in the pond could also be associated with runoff from former sugarcane fields in the watershed that drains to the pond (Cutler et al., Citation2013). Contribution of arsenic contamination from the former sugar mill located on the edge of the pond is also likely (e.g., mud from cane-washing activities), although this has not been investigated in detail.
An open, grass-covered area adjacent to the pond in the northeastern area of the park was selected for sample collection. Soils at the site are characterized by dark-brown, clayey, fine sands with silt and minimal coarse material. Prescreening of surface soil with a portable x-ray fluorescence detector (XRF) confirmed arsenic concentrations over 100 mg/kg. A grid of 24 points at a 30-foot spacing was designated within a 150′ × 90′ area.
Elevated levels of arsenic in former agricultural soils have been identified in several areas of Hawaii. Studies have shown the arsenic to be tightly bound to iron oxides in young, iron-rich volcanic soils (Cutler, Citation2011; Cutler et al., Citation2006, Citation2013). The bioavailability of the arsenic is exceptionally low (generally <10–20%) and in most cases does not pose a significant health risk to humans in spite of the relatively high total arsenic concentrations present in the soil (HDOH, Citation2011; Juhasz et al., Citation2014; Roberts et al., 2007).
Study site B
Study Site B is an area of lead-contaminated soil at a former municipal incinerator site on the Island of Oahu. The incinerator operated from the early 1970s through the mid-1990s and generated 60–120 tons of ash per day (AMEC, Citation2009). The soils include fill material placed across the property during construction and operation of the incinerator. Previous investigations identified lead-contaminated soil throughout the property, extending to a depth of ten feet or more in some areas (AMEC, Citation2013).
A 50′× 30′ area was ultimately selected for sample collection. Soils surrounding the facility are characterized by grayish-yellow to yellowish-orange sand to silty sand with an average of 25% coarse sand and gravel. Prescreening of surface soil in the area with a field XRF indicated concentrations of lead in excess of 200 mg/kg. A grid of 24 points at a ten-foot spacing was designated for sample collection.
Study site C
Study Site C is an area of PCB-contaminated soil at a former radio broadcasting station on the Island of Oahu. The 93-acre site operated as an antenna relay station from the 1940s through the 1970s. Equipment and buildings were progressively removed from the site in the 1980s and 1990s.
Site investigations in 2009 and 2011 identified PCB impacts to soil in Multi Increment samples collected from a four-acre area adjacent to the former transmitter station (Element Environmental, Citation2011). Follow-up discrete samples were collected around the former transmitter station in an attempt to identify areas of higher contamination and assist in future more focused MI sample collection. Samples were tested using field immunoassay kits, with splits of some samples submitted to a laboratory for analysis by GC/MS Method 8082. The resulting data suggested very high small-scale distributional heterogeneity of PCBs in the soil, with the concentration of PCBs in closely spaced samples varying by an order of magnitude or more in an apparently random manner. The exact cause of the variability was unknown.
A 100′× 60′ area that overlapped different soil types and suspected areas of higher and lower PCB concentrations was selected for inclusion in the study. Three distinct soil types were observed at the site. Soils in the western third of the study area are characterized by native, black to brownish-black, clayey, silty sand to sandy silt with minimal coarse material (mollisol). Soils in the eastern third of the study area are characterized by dark, reddish-brown to grayish-yellow, gravely, silty sand that represents imported, mixed volcanic soil and cinder fill. This area was formerly used for the storage of electrical equipment and was known to be more heavily contaminated with PCBs than the surrounding area. Soils in the middle portion of the study site are characterized by a mixture of native soil and fill. A 24-point grid at a spacing of 20 feet was designated for sample collection.
Sample collection
Sampling design and collection method
Discrete soil samples were collected from the three study sites in 2013 and 2014. The top two inches of soil below any grass and organic debris layer were targeted. Stainless steel trowels or similar tools were used to collect samples. Study areas were cleared of high vegetation as needed prior to fieldwork.
At Study Site A (arsenic) and Study Site B (lead), a discrete sample of 400–500 g was collected from the center of each grid point and placed in a rigid, eight-ounce plastic container, with care taken to minimize disturbance of the soil during collection (see , grid point Sample A). These samples were used to evaluate intra-sample variability of contaminant concentrations using a portable XRF. A 200–300 g discrete soil sample was then collected from each corner of a one-meter square centered on each grid point (see ). These samples were placed in separate one-quart zip-lock freezer bags (grid point Samples B, C, D, and E) and, in conjunction with the sample collected from the grid center point, used to evaluate small-scale, inter-sample contaminant concentration variability.
All discrete samples from Study Site A and Study Site B were submitted to a commercial laboratory for processing and analysis following XRF analysis of the center-point samples (total of five samples per grid point). Samples were processed and subsampled at the laboratory using representative methods identical to methods for MI samples (HDOH, Citation2016; ITRC, Citation2012). Each sample was air-dried, sieved to <2 mm, and then spread into a thin layer. A 10-g mass of soil was then collected in a systematic, random manner from 30 or more points within the sample to prepare a subsample for analysis.
A slightly modified approach was used to test intra- and inter-sample variability at Study Site C (PCBs; see ). A 200–300 g sample was collected from the center of each grid point as well as the corners of a one-meter square centered on each grid point in the same manner as carried out for each corner. These samples were processed and representatively subsampled and tested in the same manner as described above to assess inter-sample variability around each grid point. A sixth 400–500 g sample was then collected from the center area of each grid point. This sample was placed into ten separate four-ounce jars, with each jar representing a subsample for the primary sample (see ). A 10-g mass of soil was then removed from each jar by the laboratory after mechanical mixing (“homogenization”) and independently tested for PCBs. The resulting data were used to evaluate intra-sample variability at Study Site C.
Triplicate (i.e., primary plus two replicates) MI samples were also collected from each study site area for comparison to the discrete sample data (refer to HDOH, Citation2016). Fifty-four increment samples were collected from Study Site A and Study Site B. Sixty increment MI samples were collected from Study Site C. Increments were collected in a systematic (grid), random fashion using a stainless-steel sampling tube. Increments for each individual sample were combined and placed in a heavy zip-lock freezer bag and submitted to a laboratory for processing, subsampling, and testing in accordance with MI sample protocols (HDOH, Citation2016). The total bulk mass of each MI sample was approximately 1–2 kg.
Sample processing and analysis
Samples collected from Study Site A and Study Site B to assess intra-sample variability (Sample A suite) were analyzed using a field portable XRF in a manner similar to EPA Method 6200 (USEPA, Citation2007a). An Olympus Delta 2000 standard XRF with a four-watt x-ray tube and silicon drift detector was utilized. Field calibration standards, blanks, and spikes were used for QA/QC measures (refer to HDOH, Citation2015a). The instrument beam width is approximately 1 cm in diameter. The effective penetration depth of the beam for soil was estimated to be 1 cm for each 30-s reading, with total soil mass tested per reading of approximately 1 g. This is similar to the mass of soil traditionally tested for metals at commercial labs based on USEPA subsampling and extraction methods, including Method 6010C (USEPA, Citation2000, see also USEPA, Citation1996, Citation2007b).
The cover of each center grid-point sample container was removed, and five XRF readings were made from evenly spaced points on the top of each exposed sample. The sample was then turned over and pressed out onto a clean plastic sheet with minimal disturbance. Five additional readings were then made from evenly spaced locations on the exposed bottom side of the sample. Samples were not air-dried prior to testing in order to retain the cohesiveness of the soil. Soil at Study Site A was visibly moist. Samples were subsequently weighed, allowed to air-dry, and then reweighed to estimate the original percentage of moisture. The XRF data were subsequently adjusted to account for soil moisture and reported as dry weight (refer to HDOH, Citation2015a). Samples from Study Site B were not significantly moist upon collection, and the XRF data were assumed to reasonably approximate dry weight (moisture estimated to be <10%).
Following XRF testing of the “A” suite of samples, all samples (Samples A through E) collected at Study Sites A and B were submitted to a commercial laboratory for processing and representative subsampling using laboratory protocols for MI samples (HDOH, Citation2016). All samples collected from Study Site C were submitted to the laboratory immediately after collection. Samples to be used to assess inter-sample variability (Samples A through E) were likewise dried, sieved to the <2 mm particle size, and subsampled in accordance with MI protocols (HDOH, Citation2016).
Arsenic (Study Site A) and lead (Study Site B) analyses were carried out using Method 6010B. A 10-g mass of soil was digested, extracted, and analyzed in order to minimize laboratory Fundamental Error (HDOH, Citation2016). This contrasts with the recommendation to test only 1 g of soil in the USEPA lab method (USEPA, Citation1996). Samples from Study Site C were tested for PCBs using Method 8082. A 10-g mass of soil was tested in accordance with standard method recommendations. Grain-size analysis was carried out on the center sample from each grid point (“A” samples”) using Method D422 (ASTM, Citation1998). In the case of Study Site C, grain-size analysis was carried out on the combined subsamples 1–5 of the discrete sample that was used to evaluate intra-sample variability.
Results
Data generated from the field study are summarized in , , and , respectively. Individual sample results are provided in the supplement to this paper. A more detailed presentation and evaluation of the data is provided in the field reports prepared for the study (HDOH, Citation2015a,Citationb).
Table 1. Study Site A summary of combined arsenic intra- and inter-sample variability (see supplement).
Table 2. Study Site B summary of combined lead intra- and inter-sample variability (see supplement).
Table 3. Study Site C summary of combined PCBs intra- and inter-sample variability (see supplement).
Intra-sample variability
The variability between the maximum and minimum concentrations of contaminants reported for test of individual discrete samples (e.g., max:min) clearly increases from Study Site A to Study Sites B and C (; refer also to supplement). Data are typically right-skewed, with the mean max:min ratio significantly higher than the median max:min ratio. This is especially true for the lead and PCB study sites. The median is used for discussion purposes in this paper since it is more representative of the data in general.
Table 4. Summary of intra- and inter-sample variability at study sites (see supplement).
The variability of intra-sample XRF data for arsenic at Study Site A is low in comparison to Study Sites B and C, with a median max:min ratio of just 1.4 (ratio of median maximum-to-minimum reported concentration within an individual sample; ). The average Relative Standard Deviation (RSD) of the intra-sample data is just 12%, with a range of 5–30% (refer to supplement). The range of max:min ratios reported for samples is likewise very tight, with a maximum ratio of 2.5 calculated for a grid point intra-sample data set. An average Relative Percent Difference (RPD) of 44% was calculated for maximum- and minimum-reported concentrations relative to the mean, with a range of 3–117% for individual grid point samples (see supplement). The greatest degree of variability was measured in the discrete sample tested from Grid Point WLP-4, with the concentration of arsenic in individual subsamples ranging from 554 to 1,412 mg/kg, with a mean of 801 mg/kg (XRF data; refer to supplement).
The overall low variability of arsenic concentrations within an individual discrete sample suggests a relatively even distribution of arsenic in the soil at the scale of a 1-g mass. Detailed studies of the distribution and geochemistry of arsenic in soils indicate that arsenic is concentrated in micrometer-sized nuggets of iron hydroxide particles disseminated throughout the fines fraction of the soil (; Cutler, Citation2011; Cutler et al., Citation2006, Citation2013). Given the iron-rich nature of the volcanic soils and the fact that the arsenic at this site is associated with discharges of contaminated wastewater, a more uniform distribution of arsenic in the soil could be expected.
Figure 3. Arsenic-concentrated nuggets of iron hydroxide in volcanic soils typical of Study Site A (Cutler Citation2011).
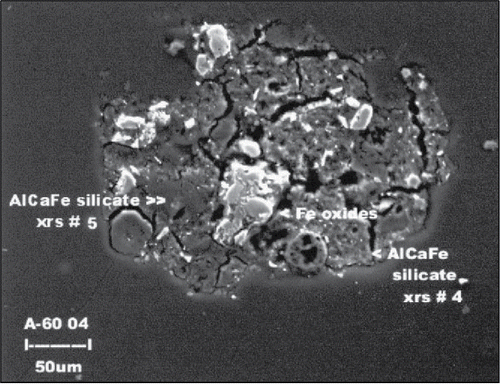
The intra-sample variability of lead concentrations within individual soil samples at Study Site B is distinctly higher than that observed at Study Site A, with a median max:min ratio of 3.5 and a maximum of 15 (). An average RSD of 40% was calculated for the data sets, with a range of 20–96%. Both indicate considerably more distributional heterogeneity within individual samples. An average RPD of 126% was calculated, with a range of 29–567% (see supplement). The highest intra-sample variability was measured for the sample collected at Grid Point WI-2, with a subsample range of 19–276 mg/kg reported and a mean of 104 mg/kg (XRF data; refer to supplement). The heterogeneous nature of lead at the scale of a 1-g subsample most likely reflects random small-scale variations in the amount of ash in any given mass of fill material. Pockets of light-colored material within the soil a few millimeters to centimeters across and presumed to be ash were evident in the field. Intentionally targeting these spots with a portable XRF (in a field screening experiment) yielded a notably higher concentration of lead than the surrounding soil.
Intra-sample variability of contaminant concentrations is greatest for PCBs at Study Site C, with a median max:min ratio of 7 but a range of 2.1–116 (). The average RSD of the intra-sample data sets is 72% but reflects a broad range of 17–277%. A similarly elevated average RPD of 999% was calculated for the data set, with a range of 38–4,067% for individual samples (see supplement). Reported concentrations of PCBs in subsamples tested from the discrete sample collected from Grid Point VOA-8, for example, ranged from 0.19 to 22 mg/kg (max:min ratio 116), with a mean concentration of 2.5 mg/kg (refer to supplement). The high intra-sample variability reported is not concentration dependent. Intra-sample variability of PCB concentrations measured for the sample collected at Grid Point VOA-12 ranged from 270 to 19,000 mg/kg, reflecting a max:min ratio of 70 (mean concentration 7,337 mg/kg).
Dramatic differences of PCB concentrations are thought to reflect the presence of millimeter-sized PCB-infused nuggets of soil within the samples. This interpretation is supported by a corresponding increase in the concentration of Total Petroleum Hydrocarbons in samples with elevated PCBs (HDOH, Citation2015a; refer to supplement). The formation of oil-infused nuggets in soil can be demonstrated by pouring oil onto dry flour (). Infiltration of oils and other liquids into dry particulate matter is governed by two forces, gravity and capillary action (Goodman, Citation2001; Murray and Sivakumar, Citation2010; Santamarina, Citation2001). The molecules of the liquid are initially drawn to each other by cohesive forces, forming a rounded droplet (). The liquid inside the droplet is under positive pressure in comparison to the surrounding air. The surface of the droplet and particles it comes in contact with are attracted by adhesive (van der Waals) capillary forces. Particles initially become bound to the surface of the liquid, coating and forming a rim around the droplet. Gravity and capillary forces gradually overwhelm cohesive forces within the droplet and begin to draw the droplet into the particulate mass. Eventually, a state is reached where the droplet is drawn entirely into the particulates, creating a saturated aggregate. Remaining cohesive forces within the liquid cause the aggregate to separate from the surrounding particles and form a rounded “nugget,” with a final coat of fine particles adhered to the outer surface.
Figure 4. (A) Droplets of olive oil formed on dry flour; note tracks left by retreating oil as it quickly formed more compact, higher standing droplets. (B) Migration of oil droplet into flour over a period of several minutes; note the distinct, thin rim that forms around the outer perimeter of the resulting nugget of oil-infused flour. (C) Olive oil-infused nuggets sieved from dry flour.
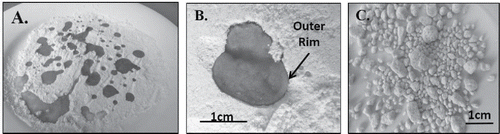
depicts a photomicrograph of apparent PCB-infused nuggets identified in a soil sample from Study Site C (Sample VOA-12-8; HDOH, Citation2015a). Note the distinct thin, light-colored rim around the perimeter of the nugget in the photomicrograph, with a coating of darker granular material adhered to the outside. Some larger grains of rock particles within the soil appeared to be covered with a dark granular material that could similarly represent a relict coating of PCB oil. This was one of ten subsamples tested from a discrete sample collected from the grid point. A concentration of 11,000 mg/kg was reported for the subsample. The concentration of PCBs reported for the full set of subsamples ranged from 270 to 19,000 mg/kg (see supplement). The high variability of PCB concentrations between the subsamples can reasonably be attributed to the presence or absence of one or more PCB-infused nuggets in the 10-g mass randomly removed and tested by the laboratory.
Figure 5. Photomicrograph of suspect PCB-infused nugget of silty soil at Study Site C, representing a remnant drop of waste oil released to the surface (Sample VOA-12 (8)). Note granular nature of interior material and distinct, thin, light-colored rim around the outer perimeter of the clump with darker particles of soil adhered to the outside.
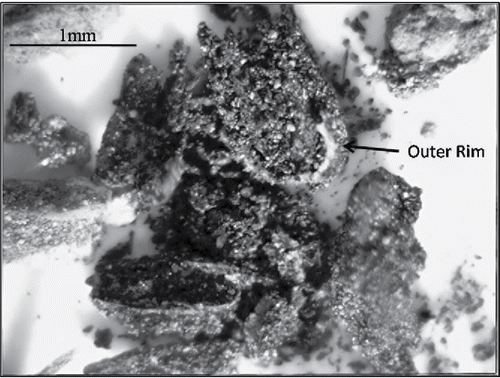
Inter-sample variability
The variability of contaminant concentrations in sets of processed co-located samples collected around individual grid points again increased from Study Site A to Study Site B to Study Site C (). Although unpredictable for any given grid points, the average variability between sets of co-located samples was similar to the average variability within individual samples.
The median ratio of maximum- to minimum-reported arsenic in co-located, processed discrete samples collected around grid points at Study Site A is 1.3, essentially identical to the median intra-sample variability of 1.4 (). The variability of max:min ratios for the Study Site A data sets is likewise almost identical to that observed for intra-sample data, ranging from 1.0 to 2.2, versus 1.2 to 2.5 for the latter. The most dramatic variability between co-located samples was observed at Grid Point WLP-2, where the concentration of arsenic in co-located samples ranged from a low of 120 mg/kg to a high of 260 mg/kg (refer to supplement). The RSD for individual grid point data sets is similarly low, ranging from 1.5% to 38% with an average value of 14% (refer to supplement). The variability of arsenic concentrations between random 1-g masses of soil within an individual 200–300-g sample is thus for all practical purposes identical to the variability observed between random co-located, processed discrete samples.
Similar observations were made at Study Sites B and C. The median ratio of maximum- to minimum-reported lead in co-located, processed discrete samples collected around grid points at Study Site B is 2.3, somewhat lower than the variability identified within individual samples (median max:min ratio 4.3) but still noticeably higher than Study Site A (). The difference in variability between individual grid points is also higher, ranging from a low of 1.3 to a high of 6.7. The greatest variability between co-located samples was observed at Grid Point WI-7, with concentrations of lead ranging from 120 to 800 mg/kg reported (refer to supplement). No correlation in inter-sample variability is apparent with respect to the mean concentration of lead identified for each grid point. The increased distributional heterogeneity around individual grid points is further reflected in the RSDs calculated for each data set, ranging from 11% to 81% with an average of 30% (refer to supplement). This is again interpreted to reflect the random distribution of small pockets of lead-contaminated ash within the soil.
Variability between co-located discrete samples is again greatest at Study Site C, with a median maximum- to minimum-reported ratio of PCBs of 4.7 (). The range of max:min ratios is roughly half that observed for individual samples but is broad, from a low of 1.4 to a high of 42. The concentration of PCBs in co-located samples collected around Grid Point VOA-11, for example, ranged from 4.8 to 200 mg/kg, for a max:min ratio of 42 (refer to supplement). The high inter-sample variability measured is again not concentration dependent. Concentrations of PCBs in co-located samples collected from Grid Point VOA-20 ranged from 0.33 to 8.1 mg/kg, reflecting a max:min ratio of 25 (mean concentration 2.2 mg/kg). An average RSD of 72% was calculated for inter-sample data sets at Study Site C, coincidental with the average RSD calculated for the intra-sample data sets and reflecting a broad range of 15–151% (refer to supplement).
Variability trends between co-located samples at each study site are random and cannot be assumed to be reflective of larger-scale trends across the site. This is interpreted to be primarily controlled by small-scale, random distributional heterogeneity of contaminant concentrations (Minnitt et al., Citation2007; Pitard, Citation1993, Citation2005, Citation2009; see also ITRC, Citation2012). This will be discussed in more detail in Part 2 of this study (Brewer et al., Citation2016). Consistent problems with quality control were not reported by the laboratory, and analytical error is assumed to be minimal relative to subsample collection error. Replicate subsamples from processed discrete samples were not tested by the laboratory. It is possible that the mass of the subsamples (10 g) was simply inadequate to be representative of average contaminant distribution within the samples. Laboratory replicate data for Multi Increment samples collected at Study Site C were very consistent, however (HDOH, Citation2015a). Ten-gram subsamples were tested for arsenic and lead at Study Sites A and B, rather than one-gram subsamples formally recommended by the laboratory method. This mass is predicted to address significant, Fundamental Error in subsample collection (for <2 mm-sized particles) and improve the precision of the test results (Pitard, Citation1993; see also HDOH, Citation2016; ITRC, Citation2012).
The total concentration of arsenic reported using the portable XRF for samples tested for intra-sample variability at Study Site A was consistently higher than that reported for co-located samples tested by extraction-based Method 6010B (average of 31%; see supplement). This is not unexpected, since the extraction of arsenic from iron-rich soils is known to be inefficient (HDOH, Citation2016). The XRF data represent a more accurate measurement of total arsenic in the samples. In contrast to arsenic at Study Site A, the total concentration of lead reported using the portable XRF was consistently lower than reported by extraction-based Method 6010B (average −6.8%; see supplement). This is assumed to be due to two factors: 1) an increased efficiency in laboratory extraction of lead compared to other metals, and 2) a reduction in XRF readings due to moisture in the samples.
Note that the intra- and inter-sample variability measured for an individual grid point cannot be assumed to be representative of that specific location. Testing alternative samples from the same general location might yield significantly different results, although the overall range of variability for the study site as a whole would likely be similar to the results of this study.
Total intra- and inter-sample variability
The total variability of contaminant concentrations reported for discrete sample-sized masses of soil collected around an individual grid is a factor of both intra- and inter-sample variability. A statistically significant estimate of the full range of variability could only be made by testing a much larger number of samples than that tested in this study. A crude estimation can, however, be made by applying the RPD measured for intra-sample data at a specific grid point to the concentrations reported for co-located processed samples collected at the grid point (refer to supplement).
show estimates of the total range of contaminant concentrations in discrete sample-sized masses of soil around individual grid points at each study site, taking into account both intra- and inter-sample variability. These estimates are provided for example only and are based on the limited number of samples available for evaluation. Testing of additional samples around grid points would no doubt reveal greater variability than identified in the study.
For example, the RPD for intra-sample data at Grid Point #1 in Study Site A is ±16% (refer to supplement). The lowest concentration of arsenic reported for the set of co-located processed discrete samples collected around the same grid point was 130 mg/kg (Sample WLP-1C). A high of 200 mg/kg was reported for the sample set (Sample WLP-1B). Adjusting the former downward by 16% yields a hypothetical lower-bound arsenic concentration of 109 mg/kg for discrete sample-sized masses of soil around the grid point. Adjusting the latter upward by the same percentage yields a hypothetical upper-bound concentration of 231 mg/kg. In total, this predicts an adjusted hypothetical range of arsenic concentrations of 109–231 mg/kg in discrete samples around the grid point. Note that this prediction applies to Method 6010B analysis as carried out for the processed samples. A range similar in magnitude but higher in concentration would be predicted for XRF data since, as described above, this method is better able to capture the true concentration of total arsenic in the soil.
Estimated in this manner, the hypothetical average total variability of contaminant concentrations in discrete samples collected around individual grid points progressively increases from a median max:min ratio of 2.0 for Study Site A, 7.5 for Study Site B, and 39 for Study Site C (). This corresponds to a median estimated RPD for grid point samples at the study sites of 96%, 650%, and 3,802%, respectively. The greatest adjusted range in arsenic concentrations predicted for individual grid points at Study Site A is 114–463 mg/kg, for Grid Point WLP-16 (refer to supplement).
Significantly broader potential ranges of total variability are predicted for Study Sites B and C. Concentrations of lead in random discrete samples collected around Grid Point WI-2 at Study Site B are predicted to range from 14 to 581 mg/kg (see supplement). The corresponding RPD estimated for the grid point is 4,050% (). Concentrations of PCBs in random discrete samples collected around Grid Point VOA-12 at Study Site C are predicted to range from an astounding 14 to 15,797 mg/kg (see supplement), with a corresponding RPD estimated for the grid point of 115,916% ().
The degree of variability measured within an individual sample or between co-located samples is by itself not necessarily a predictor of the total magnitude of variability for the grid point as a whole. The corresponding RPD estimated for Grid Point WLP-16 at Study Site A is 308% (). Measured intra-sample variability was, in contrast, greatest at Grid Point WLP-4. Inter-sample variability was greatest at Grid Point WLP-2. In practice, intra-sample variability may or may not be smaller than inter-sample variability for any given location, and it is difficult if not impossible to predict for any given site or sample location point. The representativeness of an individual discrete soil sample for soil within the immediate vicinity of a sample collection location is thus unknowable.
The relative overall increase in small-scale variability between the study sites is not unexpected, based on differences in the chemicals of concern and the presumed mechanism of contaminant release. Contamination at Study Site A is associated with the release of arsenic-contaminated wastewater and/or water-based pesticides into fine-grained soils. This scenario is likely to lead to a relatively low small-scale distributional heterogeneity of the contaminant in soil. Concentration is, however, a function of the mass of soil tested (Pitard, Citation1993). Variability in reported concentrations of arsenic would be anticipated to progressively increase as smaller and smaller masses of soil were tested. Use of an electron microprobe to test microgram-sized masses of soil, for example, would be able to distinguish between arsenic-rich nuggets of iron hydroxide and the surrounding iron-depleted matrix and even coatings of pure 100% arsenic on the nuggets (; Cutler, Citation2011).
The common question of the “maximum” concentration of a contaminant present in soil at a contaminated site is thus moot. If present, then the maximum concentration will always be 100% at a small enough scale. In this sense, the concept of “uniformity” is entirely mass dependent. As to be discussed in Part 2 of this paper, the question for any site investigation is to determine the appropriate mass, volume, and area of soil for which a concentration is desired. The mass of soil tested by a laboratory is entirely arbitrary and may, or more likely may not, have any direct relation to the objectives of the site investigation.
Contamination at Study Site B is believed to be related to incomplete mixing of lead-contaminated incinerator ash with native fill soil. This mixture is clearly evident in the sample data, with low concentrations of reported lead similar to anticipated natural background levels in soil (default 73 mg/kg; HDOH, Citation2012) and higher concentrations of lead indicating the presence of incinerator ash in the subsample extracted and tested (typically >1,000 mg/kg). Total variability is greatest at Study Site C. This is interpreted to reflect both variability in larger-scale release patterns at the site as well as significant variability within and between co-located samples due to the random presence or absence of PCB-infused nuggets of soil in a given sample.
Particle size distribution in soil (e.g., clay, silt, sand, and gravel) can also affect distributional heterogeneity and contaminant concentration variability within a soil sample (Minnitt et al., Citation2007; Pitard, Citation1993, Citation2009). Sampling theory predicts that variability in contaminant concentrations within a given mass of soil will increase with increasing nominal particle size. As shown in , both the intra- and inter-sample variabilities of contaminant concentrations are significantly higher in the coarser-grained, gravelly sands of Study Site B (median total variability = 6.8) and the fill area of Study Site C (median total variability = 33) in comparison to the clayey, fine sands of Study Site A (median total variability = 1.8). However, it is unclear if the difference in variability between the sites is controlled primarily by grain size. The fill material at Study Site B contained a smaller amount of silt and clay than the fill material at Study Site C but exhibited a distinctly lower (though still high) variability in contaminant concentrations.
The variability of PCB concentrations is likewise not clearly attributable to differences in particle-size distribution between fine-grained native soil and coarse-grained fill material at Study Site C (). Total variability in PCB concentrations is similar between the native clayey silts in the western area of the site (median total variability 94; range 7.1–895) and the gravelly sands of the eastern part of the site (median total variability 121; range 22–1,160). Variability was noticeably lower in the area of mixed native soil and fill material (median total variability 12; range 6.2–69), even though the normalized <2 mm grain-size distribution is similar to the fill soil. The cause of this difference is uncertain.
Sampling theory likewise predicts that small-scale variability in contaminant concentrations will increase with increasing mean concentration of the chemical (Minnitt et al., Citation2007; Pitard, Citation1993, Citation2009). The mean concentration of PCBs in a sample does not appear to significantly control relative intra-sample variability, however (). The ratio of maximum- to minimum-reported concentrations of PCBs in the samples as well as the average RSD for samples is similar for the native soil and the fill material, even though the average concentrations vary dramatically (; average 0.61 and 1,135 mg/kg, respectively).
Multi increment sample data sets
Data for triplicate sets of Multi Increment soil samples collected from each of the study sites are summarized in . The progressive increase in the variability of replicate samples from Study Site A to Study Site C reflects the increase observed for the discrete sample data, although within a considerably smaller range of uncertainty.
Table 5. Summary of MI replicate data for study sites (see supplement).
Arsenic concentrations of 220, 250, and 230 mg/kg were reported for replicate samples collected at Study Site A. This reflects an RSD of just 6.5% and implies very good precision of the data. Replicate samples collected at Study Site B were slightly more variable in spite of having a similar bulk sample mass and number of increments, with concentrations of 240, 270, and 350 mg/kg reported. This most likely reflects the random inclusion of more ash-concentrated increments in the latter samples. An RSD of 20% was calculated for the data, still below the target of 35% considered to reflect reasonably reliable precision (HDOH, Citation2016; ITRC, Citation2012). Variability of replicate data was considerably greater at Study Site C, with concentrations of 19, 24, and 270 mg/kg reported, reflecting a relative standard deviation of 138%.
Aside from better conforming to basic requirements of sampling theory (Pitard, Citation1993), an important advantage of MI replicate data in comparison to a single set of discrete sample data is that representativeness in terms of field precision can be tested and evaluated. The RSD values for MI samples collected from Study Sites A and B indicate good precision and a high confidence in the mean estimated for any one sample. The MI replicate RSD of 138% for Study Site C quickly calls into question the representativeness of any individual Multi Increment sample collected at the site. A 95% Upper Confidence Level (UCL) value of 346 mg/kg is calculated for the data set using the Student's t-test method, with a UCL of 467 mg/kg calculated using the Chebyshev test method (). These values are well above the arithmetic mean of 104 mg/kg and suggest that the data are unreliable for decision making. Re-subsampling and testing of the samples by the laboratory yielded identical results, suggesting that the data are representative of the samples collected and that the variability between replicates is due to field error rather than laboratory error.
Two sources of field error are likely (Pitard, Citation1993). The bulk mass of the samples (1–2 kg) might have been inadequate to address Fundamental Error and extreme distributional heterogeneity of PCBs in the soil. The collection of soil increments from too few locations within the targeted area is also likely to have played an important role. The history of the site and the results of previous investigations also suggested that the eastern half of the site was likely to be more heavily contaminated with PCBs than the western portion (Element Environmental, Citation2011). Data precision would likely be significantly improved by characterization of the suspected primary spill (source) area and anticipated cleaner areas as separate Decision Units (HDOH, Citation2016; see also ITRC, Citation2012). The precision of the data could be further improved by increasing the total mass of samples collected as well as increasing the number of increments included in each sample.
It would be erroneous to consider the high replicate an “outlier” and omit the data from further consideration. The error lies in how the samples were collected, not in the data itself. Such errors might go unnoticed in a single set of discrete samples, especially if the sample set was not representative of the full variability of PCB concentrations in soil (at the scale of a discrete sample) within the targeted area.
Conclusions
The results of this study demonstrate the uncertainty in the use of contaminant concentration data for small, random masses of soil (traditionally referred to as “discrete” or “grab” samples) as a primary part of environmental investigations. Discrete soil sample data cannot be assumed to be representative of the sample submitted. The sample submitted cannot be assumed to be representative of the immediate area from which it was collected. This has significant implications for the continued reliance on discrete soil sample data as a primary sampling strategy for environmental investigations. Discrete sample data can only be assumed to represent the actual mass of soil tested by the laboratory, typically 1–30 g. How the concentration of the discrete sample(s) relates to the objectives of the site investigation—for example, an assessment of potential direct-exposure risks—cannot be reliably determined for common site scenarios. Data reported for a given sample is random within an unknown range of potential concentrations specific to the areas where the sample was collected, the sample itself, and the mass of soil removed from the sample and tested by the laboratory. A different concentration can be expected to be reported if a co-located sample were collected from a nearby location. A different contaminant concentration can also be anticipated if a different mass of soil is removed from a sample for testing. Mechanical mixing of a sample, sometimes referred to as “homogenization,” can in theory reduce but not eliminate intra-sample variability. Mixing can also exacerbate heterogeneity problems by causing finer particles, where contaminants might be concentrated, to separate from coarser particles and settle to the bottom of the container.
This inherent and unavoidable randomness in discrete sample data can introduce significant and unseen error into attempts to identify and assess large-scale patterns of contamination that are the normal primary targets of environmental investigations. While variability within individual discrete samples might be relatively low (e.g., <100%), as observed for the study site impacted by arsenic-contaminated wastewater, a similar relative variability in co-located samples cannot be assumed to apply at other sites or for other contaminants. Among other factors, this will depend on the chemical of concern and its properties, how the waste was released into the soil, and subsequent disturbance of the area since that time. Variability and potential error increases where contaminants are concentrated in small nuggets within the soil, either as initial particles and fragments or following concentration of the contaminant in isolated nuggets by some other mechanism, as demonstrated at the PCB site in the study. In these cases, the variability of contaminant concentrations in discrete samples around individual collection points can exceed several orders of magnitude.
These factors cause the common practice of comparing individual discrete sample data points to risk-based screening levels for the identification of large-scale contamination patterns highly prone to error, even when random variability around an individual sample collection point is relatively low (e.g., ±100%). Underestimation of the extent of contamination is unavoidable, as variability at the scale of an individual discrete sample begins to range both above and below the screening levels employed. This same variability can be expected to produce purely artificial and seemingly isolated “hot spots” and “cold spots” in isoconcentration maps that would reappear but shift locations if a second independent set of discrete samples were collected. Failure to recognize artificial “cold spots” within an otherwise contaminated area can lead to premature termination of a site investigation, the primary cause of “failed” confirmation samples. The failure lies not in the sample itself, but in the inadequate consideration of sample representativeness. Removal of small artificial “hot spots” can lead to mistaken assumptions that the overall mean concentration of a contaminant with a targeted exposure area has been significantly reduced. Random variability of contaminant concentrations in soil at the scale of a laboratory subsample also leads to one of the most striking types of decision errors in the use of discrete sample data: the exclusion of “outlier” data in risk assessments in order to force a data set to fit geostatistical models that were not designed to deal with variability within an infinitely large population of particulate matter.
These problems cannot be addressed by the collection of even more discrete sample data or the development of better statistical tools. A new paradigm and better training of environmental professionals is required. Field workers in the mining and agriculture industries long ago recognized the unreliability and inefficiency of discrete sample data, as crops failed and estimates of ore reserves proved inadequate. Decision Unit and Multi Increment sampling and investigation methods were initially developed in the 1950s to specifically address these shortcomings (HDOH, Citation2016; ITRC, Citation2012; Minnitt et al., Citation2007; Pitard, Citation1993, Citation2009; Ramsey and Hewitt, Citation2005). The effects of poor sampling approaches are less discernable in the environmental industry, where data quality tends to be governed more by outdated regulatory requirements than by economics and reliability. Moving forward requires a better understanding of the nature and magnitude of potential error associated with the use of discrete sample data in environmental investigations. These issues, and the need to transition to more effective and efficient “DU-MI” investigation methods, are explored in more detail in Part 2 of this paper (Brewer et al., Citation2016).
As a final note, data for total arsenic in soil collected by using a portable XRF were consistently higher than data reported by extraction-based testing at a fixed laboratory. It is important to clarify that this does not reflect a bias or error in the XRF data. The XRF data are, in fact, interpreted to more accurately reflect the total mass of arsenic in the samples due to incomplete extraction of arsenic from soil particles under the fixed-laboratory method. The combined use of DU-MI investigation approaches and a portable XRF operated by a well-trained person could well replace the need for fixed-laboratory analysis in the near future. Care in XRF data interpretation is still warranted, however. A slightly low bias for the concentration of lead reported using the XRF is interpreted to be associated with interference from moisture in the soil.
Disclaimer
The conclusions expressed in the paper are, however, our own and may not necessarily reflect those of the reviewers. Guidance published by the Hawaii Department of Health and referenced in this paper was funded partly through the use of U.S. EPA State Response Program Grant funds. Its contents do not necessarily reflect the policies, actions, or positions of the U.S. Environmental Protection Agency. The Hawaii Department of Health does not speak for or represent the U.S. Environmental Protection Agency.
Conflict of interest
The authors declare no conflict of interest.
1244171_supplement.xlsx
Download MS Excel (10.6 MB)Acknowledgments
The authors wish to acknowledge the numerous environmental consultants and regulators who provided input and often lively debate during preparation of this paper.
Funding
The field study carried out by the Hawaii Department of Health was conducted under a grant from the U. S. Environmental Protection Agency.
References
- AMEC. 2009. Facility closure plan, Waipahu Municipal Solid Waste Incinerator. Prepared for City and County of Honolulu, Department of Environmental Services, Honolulu, HI.
- AMEC. 2013. Remedial Investigation Report, Environmental Hazard Evaluation—Former Waipahu Incinerator Facility, Waipahu, Hawaii. Prepared for City and County of Honolulu, Department of Environmental Services, Honolulu, HI.
- American Society for Testing and Materials (ASTM). 1998. Standard Test Method for Particle-Size Analysis of Soils. West Conshohocken, PA ( Designation: D 422-63).
- Bernard, N. D., and Orcutt, A. M. 1983. Dynamic Processes of Hilo Bay. University of Hawaii, Marine Option Program.
- Revise to: Brewer, R., Peard, J., and Heskett, M. 2016. A critical review of discrete soil sample reliability: Part 2—Implications. Soil Sediment Cont. Available at: http://dx.doi.org/10.1080/15320383.2017.1244172
- Clausen, J. L., Georgian, T., Bednar, A., Perron, N., Bray, A., Tuminello, P., Gooch, G., Mulherin, N., Gelvin, A., Beede, M., Saari, S., Jones, W., and Tazik, S. 2013. Demonstration of Incremental Sampling Methodology for Soil Containing Metallic Residues. United States Army Corps of Engineers, Engineer Research and Development Center, Vicksburg, MS ( EDRC TR-13-9).
- Cutler, W. C. 2011. Bioaccessible arsenic in soils of the Island of Hawaii. Ph.D. dissertation, University of Hawaii-Manoa, Department of Geology and Geophysics, Honolulu, HI.
- Cutler, W. C., Brewer, R. C., El-Kadi, A., Hue, N. V., Niemeyer, P. G., Peard, J., and Ray, C. 2013. Bioaccessible arsenic in soils of former sugar cane plantations, Island of Hawaii. Sci. Total Environ. 442, 177–188.
- Cutler, W. C., Hue, N., Ortiz-Escobar, M. E., and Martin, T. 2006. Approaches to reduce bioaccessible arsenic in Hawaii soils. Proceedings of the 5th International Conference on Remediation of Chlorinated and Recalcitrant Compounds, Monterey, CA, May 2006.
- Element Environmental. 2011. Final Project Report Phase II Confirmatory Sampling 84-acre Former Voice of America site, Maili, Oahu, Hawaii. Prepared for U.S. Coast Guard, Honolulu, HI.
- Gilbert, R. O. 1987. Statistical Methods for Environmental Pollution Monitoring. New York: Van Nostrand Reinhold Company, Inc.
- Goodman, J. 2001. Water Drops: Cohesion and Adhesion. Appalachian State University. Available at: http://www.appstate.edu/∼goodmanjm/rcoe/asuscienceed/background/waterdrops/waterdrops.html (accessed 10/1/2014).
- Hadley, P. W., and Bruce, M. L. 2013. On representativeness. Environ. Forensics 14, 109–120.
- Hallacher, L. E., Kho, E. B., Benard, N. D., Orcutt, A. M., Dudley, W. C., and Hammond, T. M. 1985. Distribution of arsenic in the sediments and biota of Hilo Bay, Hawaii. Pacific Sci. 39 (3), 266–273.
- Hawaii Department of Health (HDOH), Hazard Evaluation and Emergency Response. 2011. Update to Soil Action Levels for Inorganic Arsenic and Recommended Soil Management Practices. Honolulu, HI, November 2011.
- Hawaii Department of Health (HDOH), Hazard Evaluation and Emergency Response. 2012. Hawaiian Islands Soil Metal Background Evaluation. Honolulu, HI.
- Hawaii Department of Health (HDOH), Hazard Evaluation and Emergency Response. 2013. DU-MI Investigation of Arsenic Contamination in Waiakea Pond Sediments. Honolulu, HI.
- Hawaii Department of Health (HDOH), Hazard Evaluation and Emergency Response. 2015a. Small-Scale Variability of Discrete Soil Sample Data, Part 1: Field Investigation of Discrete Sample Variability. Honolulu, HI.
- Hawaii Department of Health (HDOH), Hazard Evaluation and Emergency Response. 2015b. Small-Scale Variability of Discrete Soil Sample Data, Part 2: Causes and Implications for Use in Environmental Investigations. Honolulu, HI.
- Hawaii Department of Health (HDOH), Office of Hazard Evaluation and Emergency Response. 2016. Technical Guidance Manual. Honolulu, HI.
- Interstate Technology Regulatory Council (ITRC). 2012. Incremental Sampling Methodology. Washington, DC.
- Juhasz, A. L., Herde, P., Herde, C., Boland, J., and Smith, E. 2014. Validation of the predictive capabilities of the Sbrc-G in vitro assay for estimating arsenic relative bioavailability in contaminated soils. Environ. Sci. Technol. 48, 12962–12969.
- Minnitt, R. C. A., Rice, P. M., and Spangenberg, C. 2007. Part 1: Understanding the components of the fundamental sampling error: A key to good sampling practice. J. South. Afr. Inst. Min. Metall. 107, 505–511.
- Murray, E. J., and Sivakumar, V. 2010. Unsaturated Soils: A Fundamental Interpretation of Soil Behavior. London: Wiley-Blackwell.
- Pitard, F. F. 1993. Pierre Gy's Sampling Theory and Sampling Practice. New York: CRC Press.
- Pitard, F. F. 2005. Sampling correctness—A comprehensive guideline. Proceedings of the Sampling and Blending Conference, Sunshine Coast, Queensland, Australia, May 9–12, 2005.
- Pitard, F. F. 2009. Theoretical, practical and economic difficulties in sampling for trace constituents. Proceedings of the 4th World Conference on Sampling and Blending, Southern African Institute of Mining and Metallurgy, Cape Town, October 19–23, 2009.
- Ramsey, C. A., and Hewitt, A. D. 2005. A methodology for assessing sample representativeness. Environ. Forensics 6, 71–75.
- Santamarina, J. C. 2001. Soil behavior at the microscale: Particle forces. Proceedings of the Symposium on Soil Behavior and Soft Ground Construction, Massachusetts Institute of Technology, Cambridge, MA, October 5–6, 2001.
- Silvius, K., Moravcik, P., and James, M. 2005. Hilo watershed based restoration plan. Prepared for Hawaii Department of Health, Polluted Runoff Control.
- U.S. Environmental Protection Agency (USEPA), Office of Emergency and Remedial Response. 1987. Data quality objectives for remedial response activities. Washington, DC. ( EPA/540/G-87/003).
- U.S. Environmental Protection Agency (USEPA), Office of Policy, Planning, and Evaluation. 1989a. Methods for evaluating the attainment of cleanup standards, volume 1: Soils and solid media. Washington, DC. ( EPA/230/U2-89/042).
- U.S. Environmental Protection Agency (USEPA), Environmental Monitoring Systems Laboratory. 1989b. Soil Sampling Quality Assurance User's Guide. Washington, DC. ( EPA/600/8-69/046).
- U.S. Environmental Protection Agency (USEPA), Environmental Monitoring Systems Laboratory. 1990. A Rationale for the Assessment of Errors in the Sampling of Soils. Washington, DC. ( EPA/800/4-90/013)
- U.S. Environmental Protection Agency (USEPA). 1996. SW846 Methods: Method 3050B, acid Digestion of Sediments, Sludges and Soils. Washington, DC.
- U.S. Environmental Protection Agency (USEPA). 2000. SW846 Methods: Method 6010C, Inductively Coupled Plasma-Atomic Emission Spectrometry. Washington, DC.
- U.S. Environmental Protection Agency (USEPA), Office of Research and Development. 2003. Guidance for Obtaining Representative Laboratory Analytical Subsamples from Particulate Laboratory Samples. Washington, DC. ( EPA/600/R-03/027).
- U.S. Environmental Protection Agency (USEPA). 2006. SW846 methods: Method 8330B, nitroaromatics, Nitramines, and Nitrate Esters by High Performance Liquid Chromatography (HPLC). Washington, DC.
- U.S. Environmental Protection Agency (USEPA). 2007a. SW846 methods: Method 6200—Field Portable x-ray Fluorescence Spectrometry for the Determination of Elemental Concentrations in Soil and Sediment. Washington, DC.
- U.S. Environmental Protection Agency (USEPA). 2007b. SW846 methods: Method 3051A, Microwave Assisted Acid Digestion of sediments, Soils and Oils. Washington, DC.