ABSTRACT
It has been recognized that depth of invasion (DOI) is closely associated with patient survival for most types of cancer. The purpose of this study was to determine the DOI optimal cutoff value and its prognostic value in laryngeal squamous carcinoma (LSCC). Most importantly, we evaluated the prognostic performance of five candidate modified T-classification models in patients with LSCC. LSCC patients from Harbin Medical University Cancer Hospital and Chinese Academy of Medical Sciences Cancer Hospital were divided into training group (n = 412) and validation group (n = 147). The primary outcomes were overall survival (OS) and relapse-free survival (RFS), and the effect of DOI on prognosis was analyzed using a multivariable regression model. We identified the optimal model based on its simplicity, goodness of fit and Harrell’s consistency index. Further independent testing was performed on the external validation queue. The nomograms was constructed to predict an individual’s OS rate at one, three, and five years. In multivariate analysis, we found significant associations between DOI and OS (Depth of Medium-risk invasion HR, 2.631; P < .001. Depth of high-risk invasion: HR, 5.287; P < .001) and RFS (Depth of high-risk invasion: HR, 1.937; P = .016). Model 4 outperformed the American Joint Committee on Cancer (AJCC) staging system based on a low Akaike information criterion score, improvement in the concordance index, and Kaplan-Meier curves. Inclusion of DOI in the current AJCC staging system can improve the differentiation of T classification in LSCC patients.
1. Introduction
Pathological features were included in the main T classification of head and neck squamous cell carcinoma (HNSCC) in the eighth edition of the Cancer Staging Manual of the Joint Council on Cancer (AJCC).Citation1 The creation of a new staging system for high-risk human papillomavirus-associated oropharyngeal cancer (OPC) and inclusion of depth of invasion into oral cancer T staging are two of the most important aspects of this change. Incorporating these factors into clinical practice can help doctors, patients, and researchers clearly communicate disease status and improve prognosis and treatment options for HNSCC patients. Given the importance of pathological features, the College of American Pathologists recognized the need for standardization of specialized head and neck pathology synoptic reports.Citation2,Citation3 This led to routine reporting of a defined set of pathological features, including Depth of invasion (DOI).Citation4–6
LSCC is one of the most aggressive head and neck malignancies, and in the early stages of the disease, surgical removal of the primary tumor is one of the main treatments. It should be noted that the need for postoperative adjuvant therapy depends on several recognized high risk factors, including: T3-4, N2-3, vascular invasion, peripheral nerve invasion, positive/insufficient incision margin, or lymphatic extravasation and other pathological features.Citation7–9 DOI has been widely studied as an indicator of poor prognosis of oral squamous cell carcinoma(OSCC), cholangiocarcinoma, colorectal cancer, melanoma and other malignant tumors.Citation10 In addition to OSCC, DOI is also included in AJCC cancer classifications such as cutaneous melanoma, cholangiocarcinoma, and cervical cancer.Citation11–13 The classification described in the current staging manual, T staging, is based on the extent to which we have directly observed tumors protruding from the surface and invading outwardly. DOI, however, refers to the vertical distance from the tumor basement membrane (BM) to the deepest surface of the tumor. Therefore, The combination of the current T staging with DOI may more accurately evaluate the potential risk of patients. The current T staging may underestimate the influence of the depth of primary tumor invasion on the prognosis and treatment selection of LSCC. For example, patients with laryngeal cancer with an invasion depth of 2 mm had the same T-stage as patients with laryngeal cancer with an invasion depth of 10 mm, although the latter had a worse clinical prognosis.Citation14 Previously, Masayuki et al and Ye et al demonstrated DOI as a strong predictor of cervical lymph node metastasis in LSCC.Citation15,Citation16 However, it is not clear whether DOI is an independent predictor of recurrent and survival of LSCC. In view of the above analysis, a more detailed description and inclusion of DOI may be beneficial for risk stratification of LSCC patients. This study aims to demonstrate the prognostic value of DOI for LSCC patients and, more importantly, to conceive a new staging system that can use DOI to improve the prognostic performance of LSCC. Here we compare the prognostic performance of multiple staging models, including the current AJCC and our newly constructed five-staging system.
2. Materials and methods
Patients
This is a multi-center retrospective study. We first collected 759 patients with LSCC diagnosed between January 2013 and December 2015 from the Department of Head, Neck, Nose and Throat Surgery, Harbin Medical University Tumor Hospital. Inclusion criteria: (1) pathologically proven LSCC; (2) resection of laryngeal cancer; (3) Complete clinical data such as laboratory examination and imaging examination. Exclusion criteria: (1) patients with recurrent primary tumor; (2) a history of preoperative chemotherapy and/or radiotherapy; (3) Patients with positive surgical margins. (4) patients with severe liver and kidney dysfunction; (5) Patients with severe heart disease. Clinical data (sex, age, BMI, alcohol and smoking history, tumor location, differentiation, T-stage and N-stage,TNM) and follow-up information (clinical outcome and survival) were collected through electronic medical record review. Patients were staged according to the AJCC8 staging system. Inclusion criteria require 5 or more years of postoperative follow-up and regular review, unless the patient dies during this period, otherwise it is excluded. A total of 412 patients were enrolled after applying the criteria. Patients with LSCC who met the above inclusion and exclusion criteria were also admitted from Cancer Hospital of the Chinese Academy of Medical Sciences (Beijing, China) between December 2014 and December 2015. 147 patients from the Chinese Academy of Sciences were assigned to the external validation cohort. OS was defined as the period from pathological diagnosis to date of death, and relapse-free survival (RFS) was defined as the time from initial surgery to earliest evidence of recurrence. This study was reviewed and approved by the Cancer Hospital affiliated to Harbin Medical University and the Ethics Committee of the Cancer Hospital of Chinese Academy of Medical Sciences. The study was carried out in accordance with the principles of the Declaration of Helsinki and its amendments. All participants agreed to participate in the study.
2.2 Histopathological analysis
The specimens of the primary lesion were fixed with formalin and embedded in paraffin. The specimens were made into 3–4 micron serial sections and stained with hematoxylin and eosin (H&E). The specimens were histopathologically diagnosed by two experienced pathologists, and they were not informed of the patient’s clinical data. When there are major differences in the examination results (greater than 0.5 mm), a third pathologist is required to participate. Measure the vertical distance from the deepest surface of the basement membrane (BM) to the deepest surface of the tumor using a microscope or a micrometer under an optical microscope magnification of 40 times. After multiple measurements, the average value is used as the DOI. The measured value is measured in millimeters.
2.3 Model evaluation
The proposed five models are compared with the current AJCC staging system. To develop a simple and accurate prognostic model. We use Akaike Information Criterion (AIC) to weigh the complexity of the model and the excellence of the fitting data. Next, Harrell’s Concordance Index (C-index) was used to evaluate the predictive performance of the model.Citation17 Finally, Risk stratification was confirmed by visual inspection of Kaplan Meier curves. R software 4.0.3 (Institute of Statistics and Mathematics, Vienna, Austria) was used to calculate the above evaluation index.
2.4 Construction and evaluation of nomograms
We combined the clinicopathological features with the DOI to construct a nomogram. We used the C index to evaluate the discriminative power of the nomogram and drew a calibration chart to evaluate the accuracy of the nomogram. We then compared the decision curve analysis between the AJCC eighth edition T staging and five newly constructed models.
2.5 Statistical analysis
The primary outcome was OS from cancer diagnosis to death and the second outcome was RFS from LSCC diagnosis to disease recurrence or metastasis or cancer specific death, whichever came first. Outcomes were obtained during the follow-up period through January, 2020 via an established protocol. Postoperative patients were followed up at 3–6 months intervals for the first year and then annually.
We used the X-tile method to calculate the cutoff values of DOI before survival analyses. Using these cutoff values, we categorized patients in to three groups: low-, medium-, and high-risk groups. Traditional statistical method like the ROC and youden index method can calculate only one cutoff and therefore categorize participants into two subsets (low- or high-risk groups). Statisticians have noted that the significance of many prognostic analyses using these discrepancies by only one cutoff point cannot be confirmed in subsequent clinical studies. The algorithms in the X-tile method correct for this discrepancy. In the X-tile method, two cutoff points in survival analyses can dramatically increase the likelihood of finding an aberrantly low p-value.Citation18
Further, We tested the proportional-hazards hypothesis for each covariate included in the Cox model fit using the self-checking function cox.zph provided in the survival package. For each covariate, the function cox.zph correlated the corresponding set of the normalized Schoenfeld residuals to the time, to test for the independence between the residuals and the time. In addition, we also use it to test the whole model globally. When the linear relationship between the proportional hazard assumption and time is not significant, we believe the model fits the proportional hazards assumption. And then we used univariate and multivariate Cox proportional hazards regression models to compare survival rate differences. We reported the corresponding hazard ratios (HR) and 95% confidence intervals (CIs) for associations of variables with OS or RFS of the disease. The variables with statistical significance in the univariate analysis were included in the multivariate Cox regression model. The likelihood ratios backward stepwise method was used for the multivariate Cox regression analysis. All analyses were performed using R software 4.0.3 (Institute of Statistics and Mathematics, Vienna, Austria). P < .05 was considered statistically significant.
3. Results
3.1 Baseline patient characteristics
summarizes the demographic and clinicopathological characteristics of the training and validation cohort. A total of 412 patients were included in the training set, including 336 men (81.6%) for a total of 147 patients in the validation cohort. Follow-up data showed that a total of 157 patients had relapsed, 119 patients died, and 34 patients died from non-disease relapse. Two optimal truncation values were determined by x-tile method, which were 10.5 and 19.9 respectively. In the training set, gender (P = .006), age (P = .002), BMI (P = .003), smoking (P < .001), alcohol (P = .002), and tumor location (P < .001) differences in DOI (). We divided the patients into high, medium and low risk groups according to DOI, as shown in .
Table 1. Summary data for LSCC DOI in the study patients (N = 412).
3.2 Screening for independent prognostic factors
Univariate and multivariate analyses were performed to consider the impact of clinicopathological features on 5-year overall survival and recurrence of LSCC. In univariate analysis, higher DOI predicted worse OS (Depth of Medium-risk invasion HR, 2.597; P < .001. Depth of high-risk invasion: HR, 4.478; P < .001) and RFS (Depth of high-risk invasion: HR, 1.938; P = .016). In addition, significant survival differences were found in BMI, degree of differentiation, T stage, N stage and TNM stage (). We included only the factors that were significant for univariate analysis in the multi-analysis model to further screen out the important factors. In multivariate analysis, BMI(HR, 0.926; P = .005), and DOI (Depth of Medium-risk invasion HR, 2.631; P < .001. Depth of high-risk invasion: HR, 5.287; P < .001) were significantly associated with OS (). From our results, patients at high-risk DOI were statistically more likely to die from recurrence. Regrettably, no significant difference was found among patients at intermediate risk of DOI. This result may need to be further verified in multicenter studies with larger sample sizes (Table S1).
Table 2. Overall survival analyses of 412 patients of LSCC (AJCC 8).
3.3 Construction and evaluation of prediction models
We constructed five candidate T classification models for evaluation based on AJCC 8 (). First, survival curves of LSCC patients were drawn based entirely on AJCC 8 T staging (). The distinction between stage T2 and T3 patients is its greatest weakness. Further, we combined AJCC and DOI to construct model 1 (). Although AIC and C-index were superior to AJCC 8, it still did not differentiate between stage T2 and T3 patients (Figure S1). Models 2 and 3 showed superior performance in predicting the prognosis of patients at stage T1 and T4, but unfortunately did not address the existing problems (). To solve this problem, we continue to build model 4 (). Model 4 demonstrated a good ability to stratify risk in patients of different age groups. We tried to find a new staging system that could go beyond model 4, but model 5 did not perform very well (), either in terms of risk stratification for early disease or in terms of both measures. After comprehensively considering the identification ability of patients with different stratification and referring to the two evaluation indexes AIC and C index, we believe that Model 4 is the best compared with the other four models. We continued to evaluate the layering capabilities of AJCC and model 4 on RFS. Compared with AJCC, model 4 showed better predictive ability in both AIC, C-index and Kaplan- Meier stratification ().
Figure 2. KaplaneMeier plots demonstrating the ability of different staging systems to describe the primary tumor T classifications and OS in the study patients with LSCC. (A) Current AJCC T staging. (B-F) Models 1 (B), 2 (C), 3 (D), 4 (E) and 5 (F) in our proposed system. AJCC, American Joint Committee on Cancer; LSCC, laryngeal squamous carcinoma; AIC, Akaike information criterion; C-index: Concordance Index.
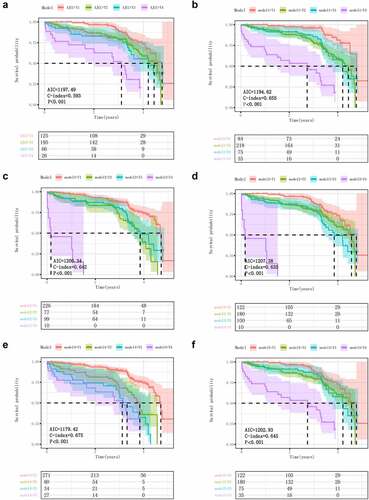
Figure 3. Kaplane-Meier plots demonstrating the ability of different staging systems to describe the primary tumor T classifications and OS/RFS in training and validation cohorts. Kaplan-Meier survival plots for RFS by AJCC T staging (A) and Models 4 (B), respectively. In the validation queue, Kaplan-Meier survival plots for OS (C) and RFS (D) by models 4, respectively. AJCC, American Joint Committee on Cancer; LSCC, laryngeal squamous carcinoma; AIC, Akaike information criterion; C-index: Concordance Index.
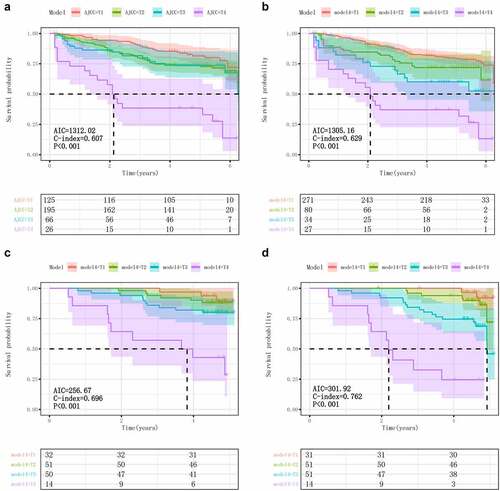
Table 3. T classifications for primary LSCC in the eighth edition of the AJCC cancer staging manual and our five candidate models.
In the external validation cohort, model 4 is also a good description of the new T-classification and OS/RFS functionality. AIC and C-index even exceeded the training cohort (). To better quantify the ability of model 4 and AJCC to distinguish patients in different stratification, we calculated the crude percentages of 5-year overall survival and median survival time of patients in both models T1-T4 (Table S2). It can be concluded that model 4 has a better ability to distinguish patients with different stratification.
3.4 Nomograms construction
In order to further improve the accuracy of the prediction, we constructed a new nomogram based on the DOI and other clinical indicators (). The nomogram C-index is 0.722 By calculating the total score, oncologists can easily obtain the probability of OS predicted by the nomogram of a single patient. We also use the calibration curve to evaluate the model’s prediction accuracy (). The results show that the prediction calibration curve of the three calibration points in 1, 3, and 5 years is close to the standard curve, which indicates that the model has good predictive performance. In addition, we also use the decision curve analysis (DCA) to evaluate the reliability of the model (). It can be seen that model 4 is significantly higher than the limit curve and other models including AJCC 8, so it has good reliability.
Figure 4. Establishment and evaluation of nomogram model. (A) Nomogram based on clinicopathological features for the prediction of 1-, 3- and 5-year OS. (B) Calibration curves of the nomogram for 1, 3 and 5 years. (C) Decision curve analysis of the nomograms based on clinicopathological features. *P<0.05, **P<0.01, ***P<0.001.
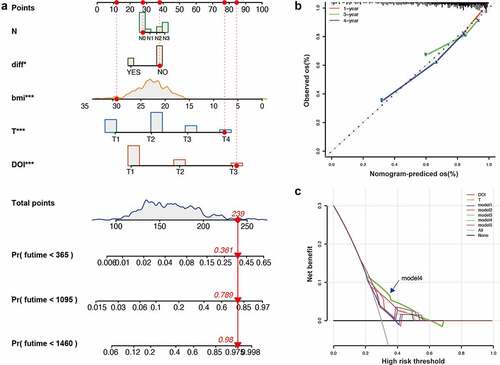
4. Discussion
The larynx is divided into special anatomical Spaces, including the anterior epiglottis space, the paraglottic space, and the subglottic space, which can affect tumor growth and spread. Due to differences in anatomical location and structure, there are differences in the biological characteristics of invasion and metastasis of supraglottic laryngeal carcinoma and glottic laryngeal carcinoma. However, in this study, patients with DOI less than 3.5 mm always showed a good prognosis of the two types of laryngeal carcinoma. With the increase of invasion depth, glottic laryngeal carcinoma could spread from the parallel space to supraglottic space, showing the biological characteristics of supraglottic laryngeal carcinoma, and invasion of supraglottic space would also show the biological characteristics of supraglottic laryngeal carcinoma. Supraglottic laryngeal carcinoma will also invade the paraglottic space or even subglottic space with the increase of invasion depth. Therefore, different types of laryngeal carcinoma may neglect or skip the anatomical classification after reaching a certain depth of invasion, resulting in a homogenous biological outcome. The traditional staging method may overlook this point. This study addresses the impact of DOI on laryngeal cancer prognosis, on the other hand, formulates two optimal DOI cutoff values for determining LSCC risk stratification. Most importantly, we construct a new staging system based on AJCC. We find that the inclusion of DOI in the current AJCC staging system provides better risk stratification in the T classification for OS.
LSCC staging with good predictive performance is the basis for personalized treatment of patients, communication with patients about prognosis and research on improving prognosis.Citation19 There are reasons to think that it is necessary to include DOI in the T class. A large number of data have shown that DOI can better reflect tumor invasion potential compared with tumor diameter.Citation20 Previously, the AJCC developed a new tumor-size staging system combined with DOI specifically for the poor prognostic performance of t-classification in many OSCC patients.Citation21,Citation22 We constructed five new LSCC models based on AJCC version 8, and evaluated the application value of the models from three aspects: the complexity of the models, the superiority of fitting data and the accuracy of prediction. Compared with existing AJCC stages, model 4 presented more comprehensive clinical implications and was relatively simple. In addition, we also show good predictive performance when applying the model to external validation queues.
Nomograms can predict tumor prognosis through simple visualization. Therefore, we developed a visual line-map based on the new model. The correlation between scores and clinical outcomes is demonstrated by an intuitive Nomu diagram, and the probability of outcome events can be easily calculated by clinicians. In our model, individual scores for each patient were calculated based on nomu’s diagram and patients were successfully stratified at risk. For patients with high score (high-risk patients), traditional surgical methods may not achieve satisfactory results, so postoperative treatment can be considered as an alternative to adjuvant therapy.
Due to the obvious prognostic value of the depth of infiltration, DOI has been included in routine pathological reports after communication between the hospital and the pathology department. The possibility of evaluating DOI in HE staining sections, along with the high reproducibility, is simple and feasible for pathologists at no additional cost, making the newly constructed TNM staging system easy to apply for routine use.
It is worth noting that our results show that patients with high BMI have better OS, which may be contrary to the traditional view. Not coincidentally, however, a total of 11,724 patients were identified from 22 trials. In sex-stratified analysis, BMI≥25 kg/m2 was associated with better 5-year overall survival in men (HR = 0.82; P = .003), except for women (HR = 1.04; P = .86).Citation23 In our study, 81.6% of patients were male, which may be one of the reasons for this conclusion. Body composition, on the other hand, varies greatly between individuals, with significant differences between races and ages. Therefore, more specific body composition indicators such as muscle tissue mass, visceral and subcutaneous fat mass should be considered.Citation24 We will continue to watch closely for further research on body composition beyond BMI.
We measured large-scale DOI data to assess its impact on patient outcomes and creatively included DOI as a pathological feature in the AJCC staging system. In addition, the study included a multicenter design, which enhanced the reliability of our results. However, some limitations must be recognized. First, it was a retrospective study and may not be applicable to subglottic laryngeal cancer. Secondly, there may be differences between the two institutions in terms of differences between pathologists used to evaluate DOI. Thirdly, socioeconomic factors, Nerve invasion, extracapsular extension, etc are not taken into account in the data analysis process, and these unmeasured variables may confuse the results. Fourth, the exclusion criteria (pre-operative chemo/RT, positive surgical margins, liver/kidney/heart disease) may limit the external validity of this study. These limitations may need to be addressed in future studies. In conclusion, our results suggest that the prognostic information provided by DOI reinforces the clinical application of the current AJCC T classification for predicting the prognosis of patients with LSCC.
In the AJCC staging system, LSCC lacks evidence for DOI classification. Our study is the first to include DOI in an LSCC staging system and assess its importance. DOI is a valuable indicator of clinicopathology that can be routinely incorporated into clinical practice and seems worthy of implementation in staging systems. It is necessary to initiate prospective studies, preferably multi-institutional studies, to further introduce TNM-DOI staging as part of routine prediction and treatment decisions for LSCC.
In LSCC, there is a lack of evidence that DOI influences patient outcomes. Our study is the first to report DOI as an independent factor influencing OS and RFS in LSCC patients. In addition, we emphasized the importance of DOI parameters as part of the new staging system for LSCC. As a valuable indicator of patient risk stratification, DOI can be routinely incorporated into clinical practice as part of routine prediction and treatment decisions for LSCC.
Ethics approval and consent to participate
All experiments involving tumor samples were conducted in accordance with the
Declaration of Helsinki and were approved by the Ethics Committee of Harbin
Medical University Cancer Institute and Hospital
Supplemental Material
Download MS Word (131.9 KB)Acknowledgments
We would like show sincere appreciation to the editors and reviewers for critical comments on this article.
Disclosure statement
The authors declare that they have no Conflict of Interest.
Data availability statement
The data used to support the findings of this study are available from the corresponding author upon request
Supplementary material
Supplemental data for this article can be accessed online at https://doi.org/10.1080/15384047.2023.2169040
Additional information
Funding
References
- Lydiatt WM, Patel SG, O’Sullivan B, Brandwein MS, Ridge JA, Migliacci JC, Loomis AM, Shah JP. Head and Neck cancers-major changes in the American joint committee on cancer eighth edition cancer staging manual. CA Cancer J Clin. 2017;67(2):122–9. 10.3322/caac.21389.
- Yunker WK, Matthews TW, Dort JC. Making the most of your pathology: standardized histopathology reporting in head and neck cancer. J Otolaryngol Head Neck Surg. 2008;37(1):48–55.none.
- King B, Corry J. Pathology reporting in head and neck cancer–snapshot of current status. Head Neck. 2009;31(2):227–231. doi:10.1002/hed.20957. discussion 32-3.
- International Consortium for Outcome Research H NC, Ebrahimi A, Gil Z, Amit M, Yen TC, Liao CT, Chaturvedi P, Agarwal JP, Kowalski LP, Cernea CR, et al. Primary tumor staging for oral cancer and a proposed modification incorporating depth of invasion: an international multicenter retrospective study. JAMA Otolaryngol Head Neck Surg. 2014;140(12):1138–1148. 10.1001/jamaoto.2014.1548
- Liao CT, Lee LY, Hsueh C, Lin CY, Fan KH, Wang HM, Hsieh CH, Ng SH, Lin CH, Tsao CK, et al. Tumor depth of invasion (Tumor > 4 cm/Depth > 10 mm and Depth > 20 mm) and through cortex/skin invasion are both valid criteria for classifying tumors as pT4a in AJCC 2018 oral cavity cancer staging system. Ann Surg Oncol. 2019;26(11):3663–3672. DOI:10.1245/s10434-019-07576-5
- Tam S, Amit M, Zafereo M, Bell D, Weber RS. Depth of invasion as a predictor of nodal disease and survival in patients with oral tongue squamous cell carcinoma. Head Neck. 2019;41(1):177–184. 10.1002/hed.25506.
- Peters LJ, Goepfert H, Ang KK, Byers RM, Maor MH, Guillamondegui O, Morrison WH, Weber RS, Garden AS, Frankenthaler RA, et al. Evaluation of the dose for postoperative radiation therapy of head and neck cancer: first report of a prospective randomized trial. Int J Radiat Oncol Biol Phys. 1993;26(1):3–11. DOI:10.1016/0360-3016(93)90167-t
- Bernier J, Domenge C, Ozsahin M, Matuszewska K, Lefebvre JL, Greiner RH, Giralt J, Maingon P, Rolland F, Bolla M, et al. Postoperative irradiation with or without concomitant chemotherapy for locally advanced head and neck cancer. N Engl J Med. 2004;350(19):1945–1952. DOI:10.1056/NEJMoa032641
- Cooper JS, Pajak TF, Forastiere AA, Jacobs J, Campbell BH, Saxman SB, Kish JA, Kim HE, Cmelak AJ, Rotman M, et al. Postoperative concurrent radiotherapy and chemotherapy for high-risk squamous-cell carcinoma of the head and neck. N Engl J Med. 2004;350(19):1937–1944. DOI:10.1056/NEJMoa032646
- Lau L, Eu D, Loh T, Ahmed Q, Lim CM. Histopathologic prognostic indices in tongue squamous cell carcinoma. Eur Arch Otorhinolaryngol. 2020;278(7):2461–2471. 10.1007/s00405-020-06329-5.
- Park JY, Kim SY, Shin DH, Choi KU, Kim JY, Sol MY, Lee HJ, Hwang C, Ryu JH, Yang KH, et al. Validation of the T category for distal cholangiocarcinoma: measuring the depth of invasion is complex but correlates with survival. Ann Diagn Pathol. 2020;46:151489. 10.1016/j.anndiagpath.2020.151489.
- Tomar AS, Finger PT, Gallie B, Mallipatna A, Kivela TT, Zhang C, Zhao J, Wilson MW, Kim J, Khetan V, et al. A multicenter, international collaborative study for American joint committee on cancer staging of retinoblastoma: part i: metastasis-associated mortality. Ophthalmology. 2020;127(12):1733–1746. DOI:10.1016/j.ophtha.2020.05.050
- Isom C, Wheless L, Hooks MA, Kauffmann RM. Early melanoma nodal positivity and biopsy rates before and after implementation of the 7th edition of the AJCC cancer staging manual. JAMA Dermatol. 2019;155(5):572–577. 10.1001/jamadermatol.2018.5902.
- Yilmaz T, Hosal S, Gedikoglu G, Turan E, Ayas K. Prognostic significance of depth of invasion in cancer of the larynx. Laryngoscope. 1998;108(5):764–768. 10.1097/00005537-199805000-00025.
- Ye LL, Rao J, Fan XW, Kong FF, Hu CS, Ying HM. The prognostic value of tumor depth for cervical lymph node metastasis in hypopharyngeal and supraglottic carcinomas. Head Neck. 2019;41(7):2116–2122. 10.1002/hed.25667.
- Tomifuji M, Imanishi Y, Araki K, Yamashita T, Yamamoto S, Kameyama K, Shiotani A. Tumor depth as a predictor of lymph node metastasis of supraglottic and hypopharyngeal cancers. Ann Surg Oncol. 2011;18(2):490–496. 10.1245/s10434-010-1219-5.
- Harrell FE Jr., Califf RM, Pryor DB, Lee KL, Rosati RA. Evaluating the yield of medical tests. JAMA. 1982;247(18):2543–6.none. doi:10.1001/jama.1982.03320430047030.
- Camp RL, Dolled-Filhart M, Rimm DL. X-tile: a new bio-informatics tool for biomarker assessment and outcome-based cut-point optimization. Clin Cancer Res. 2004;10(21):7252–7259. 10.1158/1078-0432.CCR-04-0713.
- Friedland PL, Bozic B, Dewar J, Kuan R, Meyer C, Phillips M. Impact of multidisciplinary team management in head and neck cancer patients. Br J Cancer. 2011;104(8):1246–1248. 10.1038/bjc.2011.92.
- Dirven R, Ebrahimi A, Moeckelmann N, Palme CE, Gupta R, Clark J. Tumor thickness versus depth of invasion - Analysis of the 8th edition American joint committee on cancer staging for oral cancer. Oral Oncol. 2017;74:30–33. 10.1016/j.oraloncology.2017.09.007.
- Howaldt HP, Kainz M, Euler B, Vorast H. Proposal for modification of the TNM staging classification for cancer of the oral cavity. DOSAK J Craniomaxillofac Surg. 1999;27(5):275–288. 10.1054/jcms.1999.0070.
- Yuen AP, Lam KY, Wei WI, Lam KY, Ho CM, Chow TL, Yuen WF. A comparison of the prognostic significance of tumor diameter, length, width, thickness, area, volume, and clinicopathological features of oral tongue carcinoma. Am J Surg. 2000;180(2):139–143. 10.1016/s0002-9610(00)00433-5.
- Greenlee H, Unger JM, LeBlanc M, Ramsey S, Hershman DL. Association between body mass index and cancer survival in a pooled analysis of 22 clinical trials. Cancer Epidemiol Biomarkers Prev. 2017;26(1):21–29. 10.1158/1055-9965.EPI-15-1336.
- Strulov Shachar S, Williams GR. The obesity paradox in cancer-moving beyond BMI. Cancer Epidemiol Biomarkers Prev. 2017;26(1):13–16. 10.1158/1055-9965.EPI-16-0439.