Abstract
This paper is a post-hoc analysis of a previous study performed to investigate the relationship between computerized tomography (CT) and lung function in 51 outpatients with mild-to-moderate COPD. We studied whether changes in lung function and radiographic patterns may help to explain dyspnea, the most disturbing symptom in patients with COPD. The Medical Research Council (MRC) dyspnea scale shows, by univariate analysis, a similar strength of association to CT expiratory lung density and to DLCO, a functional index of lung parenchymal loss. The MRC dyspnea scale shows a somewhat less strength of association with a small vertical heart on plain chest films. In multivariate analysis, the model with the strongest association to the MRC dyspnea scale (r = 0.76, p < 0.0001) contains 4 explanatory variables (DLCO, FRC, PaCO2, and radiographic pattern of pulmonary hypertension). We suggest that diffusing capacity reflects the emphysematous component of hyperinflation, associated by definition with destruction of terminal airspace walls, as distinct from the air trapping component, which is ascribed to airway obstruction and associated with FRC. PaCO2 mainly reflects the ventilatory components, i.e., ventilatory drive and ventilatory constraints, of pulmonary gas exchange in COPD, while radiographic pattern of pulmonary hypertension likely reflects hypoxic vascular changes, which depend mainly on ventilation/perfusion mismatch and give rise to pulmonary arterial hypertension that may contribute per se to dyspnea. In conclusion, our analysis points out that chronic effort dyspnea variance may account for up to 58% (r2 = 0.58) by lung function tests and radiographic patterns. Thus, about 42% of the MRC dyspnea variance remains unexplained by this model. On the other hand, dyspnea ascertainment is dependent on subjective behavior and evaluation and in tests is influenced by individual performance and perception. For example in the 6-minute walk test, a similar or higher proportion (60%) of the overall variance is unexplained.
INTRODUCTION
The role of high resolution computed tomography (HRCT) in the diagnosis and assessment of COPD is established and still expanding while effort dyspnea represents the most disturbing symptom in patients with COPD thus markedly deteriorating the quality of their life. Hence, it appears of great interest to investigate whether HRCT findings and, possibly, other radiologic features may help to explain, either taken alone or in combination with lung function tests, the symptom of dyspnea in patients with COPD. In a previous analysis (Citation[1]), univariate logistic regression has shown that significant lung functional changes and dyspnea perception in patients with COPD are predicted by 10% or greater changes in four different measures of HRCT lung attenuation: percentage area with lung attenuation values below –950 Hounsfield Units (HU) at 90% of vital capacity (VC) [RAI950 (% lung area)], percentage area with lung attenuation values below –910 HU at 10% of VC [RAE910 [% lung area]), mean lung attenuation at 90% of VC (Mean CT insp), and mean lung attenuation at 10% of VC (Mean CT exp).
However, multivariate logistic regression of the 4 HRCT parameters on lung function impairment and dyspnea perception has clearly shown that, whereas inspiratory measures better assess the extent of emphysematous tissue loss and expiratory ones better reflect airflow limitation and lung hyperinflation with attendant dyspnea perception, only mean CT exp significantly predicts the MRC dyspnea scale (Citation[1]). This analysis was done to investigate whether there are additional explanatory variables among lung function tests and plain chest X-rays findings that correlate with and explain the magnitude of dyspnea.
Patients and methods
Fifty-one COPD outpatients in stable clinical conditions (5 females, age 64 ± 8 years, smoking history 43 ± 5 pack-years) with mild to severe nonreversible airflow obstruction (Citation[2], Citation[3]) were the subjects of this study. Patients had been enrolled in the outpatients clinic if willing to participate and able to undergo spirometrically gated HRCT (Citation[1]). The institutional review board approved the study protocol; individual informed written consent was obtained from all patients.
Presence and severity grades of dyspnea were assessed from the answers to the Medical Research Council (MRC) questionnaire (Citation[4]). For the evaluation of lung function impairment, dynamic and static lung volumes, single breath diffusing capacity for carbon monoxide, and arterial blood gases were measured (Citation[2], Citation[3], Citation[5]). Chest X-ray films (CXR) were obtained in the erect position for postero-anterior and lateral views (Citation[6]).
On the same day of the clinical and functional evaluation, patients underwent spirometrically gated HRCT scanning according to the technique developed by Kalender and colleagues (Citation[7], Citation[8]). Images have been acquired at the level of the carina, 5 cm above, and 5 cm below the carina. Scanning parameters were 1 mm collimation, 140 Kpv, 146 mA, 1 second scanning time; a high-resolution algorithm (window width 1500 HU, window level –500 HU) was used for images reconstruction. A semiautomated density discriminating software generated Hounsfield Units (HU) frequency histograms for both lungs at each of three selected lung levels ( and ) to derive RAI950 (% lung area), RAE910 (% lung area), Mean CT insp, and Mean CT exp.
Figure 1 High-resolution computed tomography (HRCT) scans at the carina level, out of the 3 selected lung levels, spirometrically gated: (A) at 10% of vital capacity, (B) at 90% of vital capacity.
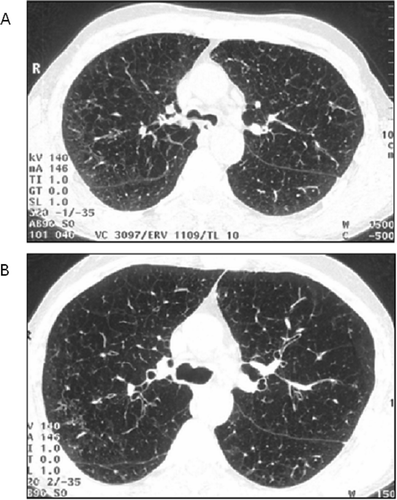
Figure 2 HRCT scan at the carina level, at 10% of vital capacity. Lung parenchyma contours are outlined; the 3 HU frequency functions represent distribution of lung density for both lungs (A), right (B), and left ones (C), respectively, and are used to compute Mean CT exp (HU) (ME in the picture = − 787.9 HU for both lungs); the vertical line represents − 910 HU threshold, and it is used to compute RAE910 (% lung area) (corresponding to % AR in the picture = 16,8 for both lungs). Other figures in the picture are not used in the analyses.
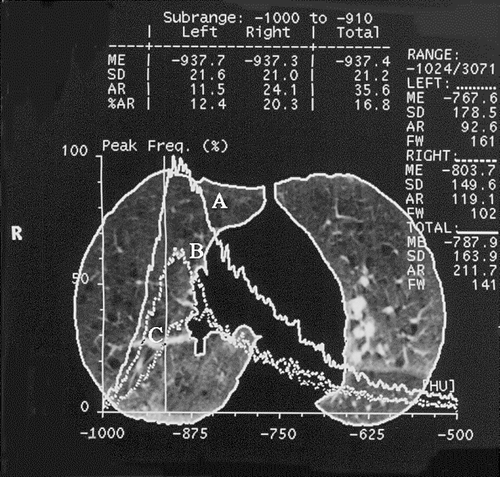
Statistical analysis
Univariate linear regression analysis was applied considering MRC dyspnea scale as the dependent variable. Multivariate direct regression analysis was performed considering lung function and HRCT data in turn as independent variables. To minimize the number of variables in the 2 models a forward stepwise analysis was then applied. The same multivariate direct procedure was applied to the whole set of functional and HRCT density data while the number of variables in the model was minimized by both a forward and a backward stepwise analysis. The role of plain CXR findings as explanatory variables of the MRC scale of dyspnea was investigated by means of univariate and multivariate direct and forward stepwise analysis. The same multivariate direct procedure was applied to the whole set of functional parameters, HRCT density data and CXR findings while the number of variables in the model was minimized by both a forward and a backward stepwise analysis. For the above-mentioned statistical procedures significance was set at p ≤ 0.05. Statistical analysis was performed using a statistical package (PSS/PC WIN 11.5.1; SPSS; Chicago, IL).
RESULTS AND DISCUSSION
Pulmonary function data, MRC dyspnea score, and spirometrically-gated HRCT densitometric measurements obtained in the 51 patients with COPD showed a great range of variation, likely reflecting the wide spectrum of clinical and functional COPD characteristics in this series of patients (Citation[1]).
Lung function and HRCT density vs. dyspnea by univariate analysis
From the univariate regression of the variables (), the MRC score of dyspnea showed a similar strength of association to a functional index, under these clinical conditions, of lung parenchymal loss [DLCO (%pred)] and to HRCT indexes of expiratory lung density [RAE910 (% lung area), Mean CT exp (HU)]. Historical papers on assessment of dyspnea in patients with COPD usually do not mention DLCO (% pred) as a possible explanatory variable (Citation[9], Citation[10], Citation[11], Citation[12]). However, the association reported between single breath carbon monoxide lung diffusion and CT (Citation[13], Citation[14], Citation[15], Citation[16], Citation[17], Citation[18]) or HRCT lung density (Citation[1], Citation[19], Citation[20], Citation[21], Citation[22], Citation[23], Citation[24]) suggests that lung diffusion may provide a functional counterpart of the imaging (morphologic) assessment by CT or HRCT and thus may concur to explain dyspnea in patients with COPD.
Table 1 Univariate analysis of Medical Research Council (MRC) dyspnea score association with lung function, Chest X-Ray (CXR) findings and High- Resolution Computed Tomography (HRCT) density in 51 patients with COPD
This association has been observed under CT or HRCT inspiratory and expiratory conditions with different statistical significance (Citation[1], Citation[18], Citation[20], Citation[25]), depending mainly on the respiratory functional characteristics of the studied patients. The better association of HRCT expiratory rather than inspiratory lung density to dyspnea, as suggested by the univariate analysis, is not unexpected since it has been reported that HRCT inspiratory lung density is a poor predictor of the elastic properties of the lung tissue (Citation[18], Citation[24]). Loss of lung tissue is associated with both airway obstruction and consequent air trapping. The results of univariate regression indicate that the MRC score of dyspnea is also significantly associated with functional indexes of obstruction/limitation of expiratory flow and lung hyperinflation (). Finally, there was a significant association, though to a lesser degree, of the MRC dyspnea scale to the variables of pulmonary gas exchange ().
Lung function and HRCT density vs. dyspnea by separate multivariate analysis
On the basis of the results reported in , it is expected that multivariate regression analysis may shed light on these associations. From the multivariate analysis of the association of the MRC dyspnea scale to lung function and HRCT density, respectively (), it appears, with respect to the direct regression of the 9 lung function variables, that the MRC score of dyspnea may be associated with all of them, with a correlation coefficient of 0.73 (p < 0.001). Whereas minimizing the number of variables in the model by stepwise regression, the MRC scale of dyspnea is associated to a 3-variable model [DLCO (% pred), PaCO2 (mmHg), and FRC (% pred)] with a correlation coefficient only slightly lower (r = 0.71, p < 0.0001).
Table 2 Multivariate analysis of separate MRC dyspnea score association with lung function and HRCT density in 51 patients with COPD, respectively
This suggests that lung function tests reflecting lung parenchymal loss, pulmonary gas exchange, and lung hyperinflation, respectively, may account (r2 = 0.50) for 50% of MRC dyspnea variance in patients with COPD. As to the 4 variables of lung HRCT density, it appears that the MRC score of dyspnea is associated, with a correlation coefficient of 0.63 (p < 0.001), to all of them by direct regression, with the 2 expiratory variables showing a higher correlation coefficient compared to the 2 inspiratory ones. By stepwise regression, just 1 expiratory variable [RAE910 (% lung area)] almost accounts (r = 0.61, p < 0.0001) for the overall direct correlation, thus confirming the results of the multivariate logistic regression, reported previously (Citation[1]), that expiratory HRCT lung density explains the MRC dyspnea score significantly better than inspiratory HRCT lung density. This, from the pathophysiological point of view, is compatible with the conclusions of several authors (Citation[1], Citation[23], Citation[24], Citation[25]). Gevenois et al. (Citation[25]) concluded that expiratory HRCT lung density probably reflects expiratory airway obstruction and the subsequent air trapping in addition to emphysema and the attendant increase in terminal airspace volume.
On the other hand, Mishima et al. (Citation[23]) found that an accurate index of parenchymal loss, derived from fractal analysis of terminal airspace geometry and more sensitive than the overall exten t of low attenuation areas on the inspiratory CT [RAI960 (% lung area)], was not correlated with functional indexes of airway obstruction and hyperinflation. Likewise, Baldi et al. (Citation[24]), as already mentioned for the univariate analysis, observed that RAI950 (% lung area) cannot accurately predict the elastic properties of the lung tissue and, hence, airway obstruction and consequent air trapping. From a methodological point of view, it was found that results of multivariate analysis derived by either logistic regression (Citation[1]) or ordinary regression agree.
Lung function and HRCT density vs. dyspnea by combined multivariate analysis
In the multivariate analysis of joint association of the MRC dyspnea scale to lung function and HRCT density (), the MRC score of dyspnea was associated to all 9 lung function (plus 4 HRCT lung density) variables with a correlation coefficient of 0.75 (p < 0.001). However, minimizing the number of variables in the model by forward stepwise regression, the MRC dyspnea scale was associated to a 3-variable model [RAE910 (% lung area), FRC (% pred), and PaCO2 (mmHg)] with a correlation coefficient somewhat lower (r = 0.69, p < 0.0001). Pooling together all 13 variables of lung function and HRCT lung density, separately analyzed in , the 3-variable models obtained in by forward stepwise regression was expected. Indeed, RAE910 (% lung area) is the variable with the highest correlation coefficient (r = 0.61, p < 0.0001) in the univariate analysis () and is strongly associated to DLCO (% pred) (r = −0.72) just slightly less than RAI950 (% lung area) (r = −0.73) (Citation[1]). Thus, RAE910 (% lung area) has the characteristics to replace DLCO (% pred) in the model.
Table 3 Multivariate analysis of combined MRC dyspnea score association with lung function and HRCT density in 51 patients with COPD
On the other hand, after minimizing the number of variables in the model by backward stepwise regression (), the MRC scale of dyspnea was associated to the 3-variable model [PaCO2 (mmHg), DLCO (% pred), and FRC (% pred)] with a correlation coefficient slightly lower (r = 0.71, p < 0.0001). This pooled model has the same variables and the same statistical significance as the separate model for lung function variables in . According to the observations from the literature (Citation[20], Citation[23], Citation[24]) and analysis of our data, it appears that DLCO (% pred) likely reflects the emphysematous component of hyperinflation associated to lung tissue loss as distinct from the air-trapping component present in FRC (% pred), whereas PaCO2 (mmHg) mainly reflects the ventilatory components, i.e., ventilatory drive and ventilatory constraints, of pulmonary gas exchange. The weak association of PaCO2 (mmHg) to the MRC score of dyspnea (r = 0.29, p < 0.04) in the univariate regression () suggests that the role of this variable, whose level depends on ventilatory drive which tends to lower it and on tidal volume constraints that tend to raise it, may be better appreciated in the multivariate regression when the role of other explanatory variables of dyspnea, i.e., DLCO (% pred) and FRC (% pred), are accounted for.
Adding chest X-ray to explain dyspnea
The role of plain CXR findings as explanatory variables of the MRC dyspnea scale is examined in and . It appears from the direct regression of 6 selected CXR findings (Citation[6], Citation[26]), that the MRC dyspnea scale may be associated with 5 of them with a correlation coefficient of 0.57 (p < 0.003), whereas, minimizing the number of variables in the model by stepwise regression, the MRC dyspnea scale is associated with a 2 variable model (small vertical heart, increased lung volumes) with a correlation coefficient only slightly lower (r = 0.54, p < 0.0001) (). Overall, as explanatory variables for MRC scale of dyspnea, CXR findings (r2 = 0.29) appear somewhat weaker than HRCT lung density (r2 = 0.37) and both radiographic patterns are weaker than lung function tests (r2 = 0.50).
Table 4 Multivariate analysis of separate MRC dyspnea score association with 6 CXR findings in 51 patients with COPD
Table 5 Multivariate analysis of combined MRC dyspnea score association with lung function, HRCT density, and CXR findings in 51 patients with COPD
Nonetheless, co-correlation among variables and close values among correlation coefficients also suggest including plain CXR findings in the multivariate analysis. It turns out, from the direct regression of the 9 lung function, 4 HRCT lung density, and 6 CXR variables, that the MRC dyspnea scale is associated with 18 variables (excluded: Enlarged right heart sections) with a correlation coefficient of 0.81 (p < 0.001). When the number of variables in the model is minimized by forward stepwise regression, the MRC dyspnea scale is associated with a 3-variable model [RAE910 (% lung area), Increased lung volumes, and PaCO2 (mmHg)] with a correlation coefficient somewhat lower (r = 0.70, p < 0.0001) (). From the point of view of the pathophysiologic compatibility of this model with that obtained by the same type of analysis in , it appears that a CXR finding, i.e., increased lung volumes, reflecting pulmonary changes of emphysema, can replace a lung function test, i.e., FRC (% pred), reflecting lung hyperinflation. It is known that these 2 variables both reflect the increase of distal airspace volume and, therefore, the 2 models are compatible with each other.
Furthermore, minimizing the number of variables in the model by backward stepwise regression (), the MRC score of dyspnea is associated with a 4-variable model [Pulmonary hypertension, FRC (% pred), PaCO2 (mmHg), and DLCO (% pred)] with a correlation coefficient slightly lower (r = 0.76, p < 0.0001). Thus, it appears that HRCT lung density variables behave similar to the multivariate analysis, which does not contain plain CXR findings, confirming the stability of the model based on variables reflecting the various components of lung hyperinflation (emphysematous component and air trapping component) and pulmonary gas exchange (ventilatory drive and tidal volume constraints). As to plain CXR findings, interestingly, increased lung volumes replace FRC (% pred) in the forward model, as already discussed, and pulmonary hypertension enters the backward model suggesting that pulmonary artery hypertension (PAH), reflecting mainly the hypoxic vascular changes (Citation[27]) of chronic bronchitis (Citation[6]), adds to the explanation of dyspnea in patients with COPD. This was expected, since PAH per se is characterized by the presence of dyspnea (Citation[28]) and the severity of PAH, independently from etiology, is clinically assessed by New York Heart Association (NYHA) functional classes that are chiefly based on dyspnea (Citation[29], Citation[30]).
Methodological considerations
Before reaching any conclusion it is pertinent to address the characteristics of the methods and in particular the multivariate analysis. The outcome variable, i.e., exertional dyspnea, as assessed by the MRC dyspnea scale (Citation[4], Citation[31]), was shown by Hajiro et al. (Citation[11]) to have the same discriminant power as baseline dyspnea index (BDI), oxygen cost diagram (OCD), activity of St. George's respiratory questionnaire (SGRQ), and dyspnea of chronic respiratory questionnaire (CRQ) in patients with COPD and a wide spectrum of severity.
Grades of the MRC scale of dyspnea were also shown by Bestall et al. (Citation[12]) to reflect accurately the results of the 6-minute walk test in patients with COPD. Finally, Eltayara et al. (Citation[10]), testing the role of expiratory flow limitation at rest against that of FEV1 in patients with COPD, consider the MRC dyspnea scale (Citation[31]) as the gold standard to set up a table of sensitivity analysis. Thus, the MRC dyspnea score seems adequate to characterize the functional status of patients with COPD. Limitations stem from the composition of the study population, differences in the CT technique, and the use of multivariate analysis. By univariate analysis, e.g., correlation of expiratory low attenuation area (% lung area) (LAA% exp) with DLCO (% pred) is significant and similar to LAA% insp in the 4 quoted studies: Knudson et al. (Citation[18]) (LAA % insp −0.32, p = 0.004; L AA% exp −0.63, p < 0.001, n = 64); Miniati et al. (Citation[20]) (L AA% insp −0.65, p < 0.001; L AA% exp −0.71, p < 0.001, n = 25); Gevenois et al. (Citation[25]) (L AA% insp −0.43, p < 0.01; L AA% exp −0.50, p < 0.001, n = 59); and Camiciottoli et al. (Citation[1]) (L AA% insp −0.73, p < 0.0001; L AA% exp −0.72, p < 0.0001, n = 51).
To have an idea of the extent of effort airflow limitation and reduction in diffusing capacity, FEV1 (% pred) and DLCO (% pred) in the 4 studies were: 60, 34, 73, and 52 and 80, 58, 66, and 68, respectively. In the last of these studies (Citation[1]), multivariate analysis, either by logistic or ordinary regression, selects LAA% insp, namely [RAI950 (% lung area)], among the four HRCT lung density variables, as the one with most predictive value for DLCO (% pred) not further modified by the other three; it is expected that in the first three studies LAA% exp would have been selected. Distributions of effort airflow limitation and diffusing capacity in a sample of patients with COPD, as reported by Gelb et al. (Citation[22]), influence the correlation between CT emphysema score and DLCO (% pred) or lung function tests by and large.
For example, on one side, there are few COPD patients with large or very large lung volumes with nearly normal FEV1 (% pred) and DLCO (% pred) and, on the other side, there are COPD patients, not so few, with marked reduction in FEV1 (% pred) and some reduction in DLCO (% pred) with no or trivial emphysema (Citation[22]). The former are patients occasionally encountered who perform quite well (as yet), whereas the latter may be those patients with COPD who develop PAH and/or frequent exacerbations in the course of chronic bronchitis or who may develop bronchiectasis in the frame of severe bronchial obstruction and progressive impairment of diffusing capacity, obliterative bronchiolitis, etc. Such COPD patients are bound to be outliers in any comparison regarding the characterization of emphysema.
The level of the correlation in the study by Camiciottoli et al. (Citation[1]) might be ascribed, besides the patients composition of the study sample (hopefully, with few outliers), to accuracy of the HRCT technique, which is spirometrically gated. At any rate, according to standard criteria of airflow limitation, our patients [FEV1 (% pred): mean 52, standard deviation 20, median 52, range 15–106] cover the full spectrum of functional derangement. If we consider a different sample of COPD patients, e.g., that by Eltayara et al. (Citation[10]) [FEV1 (% pred) in men: mean 34, standard deviation 14] somewhat different results in the multivariate analyses are expected. Therefore, the results should be compared only for groups of patients in the same range of functional derangement. On the other hand, the explanatory models for MRC score of dyspnea in and appear stable and backward stepwise regression seem s to obviate some shortcomings of the forward approach.
CONCLUSIONS
In a group of 51 outpatients with mild to moderate COPD, the MRC dyspnea score shows, by univariate analysis (), a similar strength of association to a HRCT index of expiratory lung density [RAE910 (% lung area)] and to a functional index of lung parenchymal loss [DLCO (% pred)]. It shows a somewhat less strength of association () to a plain CXR finding of small vertical heart. Taken one at a time, indexes of HRCT, LFT, and CXR account at most for 37, 36, and 19%, respectively (), of MRC dyspnea variance.
In separate multivariate analysis (1 group of explanatory variables at a time), significant explanatory variables are: for lung function, DLCO (% pred), FRC (% pred), and PaCO2 (mmHg); for HRCT lung density, Mean CT exp (HU) and RAE910 (% lung area); and for plain CXR findings, small Vertical heart and increased lung volumes. In separate multivariate analysis, indexes of HRCT, LFT, and CXR account for 40, 50, and 29%, respectively, of the variance of MRC dyspnea scale.
In a combined multivariate analysis with lung function and HRCT lung density as explanatory variables (), the model where DLCO (% pred) replaces RAE910 (% lung area) shows a correlation coefficient slightly higher, suggesting that it reflects slightly better the emphysematous component of hyperinflation, associated with lung tissue loss, as distinct from the air trapping component, to be ascribed to airway obstruction, present in FRC (% pred). In both models, PaCO2 (mmHg) reflects the ventilatory components (ventilatory drive and tidal volume constraints) of pulmonary gas exchange. In combined multivariate analysis, the better model is the same as that for LFT in separate analysis and accounts for 50% of the MRC dyspnea variance, but the other model has an explanatory power (r2 = 0.48), which is only slightly lower and, depending on the characteristics of the patients included in the sample, may even be expected to become higher.
In combined multivariate analysis with LFT, HRCT, and CXR patterns as explanatory variables (), HRCT lung density variables behave as in the multivariate analysis not containing CXR findings, thus confirming the stability of the model based on variables reflecting various components of lung hyperinflation and pulmonary gas exchange. As to CXR findings (), interestingly, Increased lung volumes replaces FRC (% pred) in the forward model and Pulmonary hypertension enters the backward one, thus suggesting that hypoxic vascular changes, reflecting V/Q mismatch and giving rise to PAH, add to the explanation of dyspnea in patients with COPD.
The forward model accounts for 49% and the backward one for 58% of MRC dyspnea variance. The latter figure represents the highest estimate of the proportion of MRC dyspnea variance accounted for by lung function tests, HRCT lung density indexes, and CXR findings (taken together). Thus, about 42% of the MRC dyspnea variance remains unexplained (by the backward model). On the other hand, dyspnea ascertainment is dependent on subjective behavior and evaluation and in tests is influenced by individual performance and perception. A similar proportion of the overall variance may remain unexplained as reported by Enright and Sherrill (Citation[32]) who found that about 60% of the variance in the 6-minute walk test remained unexplained in their models in healthy adults. The results of this study need to be prospectively evaluated in a different series of patients.
REFERENCES
- Camiciottoli G, Bartolucci M, Maluccio N M, Moroni C, Mascalchi M, Giuntini C, Pistolesi M. Spirometrically-gated high-resolution CT findings in COPD: lung attenuation vs. lung function and dyspnea severity. Chest 2006; 129: 558–564
- American Thoracic Society. Lung function testing: selection of reference values and interpretative strategies. Am Rev Respir Dis 1991; 144: 1202–1208
- Siafakas N M, Vermeire P, Pride N B, Paoletti P, Gibson J, Howard P, Yernault J C, Decramer M, Higenbottam T, Postma D S, Rees J on behalf of the European Respiratory Society Task Force. Optimal assessment and management of chronic obstructive pulmonary disease (COPD). Eur Respir J 1995; 8: 1398–1420
- Fletcher C M, Elmes P C, Fairbairn A S, Wood C H. The significance of respiratory symptoms and diagnosis of chronic bronchitis in a working population. Br Med J 1959; 5147: 257–266
- American Thoracic Society. Single breath carbon monoxide diffusing capacity (transfer factor): recommendations for a standard technique. Am J Respir Crit Care Med 1995; 152: 2185–2198
- Milne E NC, Pistolesi M. Reading the Chest Radiograph. A Physiologic Approach. Mosby, St Louis 1993; 267–310
- Kalender W A, Rienmüller R, Seissler W, Behr J, Welke M, Fichte H. Measurement of pulmonary parenchymal attenuation: use of spirometric gating with quantitative CT. Radiology 1990; 175: 265–268
- Kalender W A, Fichte H, Bautz W, Skalej M. Semiautomatic evaluation procedures for quantitative CT of the lung. J Comput Assist Tomogr 1991; 15: 248–255
- Mahler D A, Weinberg D H, Wells C K, Feinstein A R. The measurement of dyspnea: contents, interobserver agreement, and physiologic correlates of two new clinical indexes. Chest 1984; 85: 751–758
- Eltayara L, Becklacke M R, Volta C A, Milic-Emili J. Relationship between chronic dyspnea and expiratory flow limitation in patients with chronic obstructive pulmonary disease. Am J Respir Crit Care Med 1996; 154: 1726–1734
- Hajiro T, Nishimura K, Tsukino M, Ikeda A, Koyama H, Izumi T. Analysis of clinical methods used to evaluate dyspnea in patients with chronic obstructive pulmonary disease. Am J Respir Crit Care Med 1998; 158: 1185–1189
- Bestall J C, Paul E A, Garrod R, Garnham R, Jones P W, Wedzicha J A. Usefulness of the Medical Research Council (MRC) dyspnoea scale as a measure of disability in patients with chronic obstructive pulmonary disease. Thorax 1999; 54: 581–586
- Sakai F, Gamsu G, Im J G, Ray C S. Pulmonary function abnormalities in patients with CT- determined emphysema. J Comput Assist Tomogr 1987; 11: 963–968
- Gould G A, MacNee W, McLean A, Warren P M, Redpath A, Best J JK, Lamb D, Flenley D C. CT measurements of lung density in life can quantitate distal airspace enlargement – an essential defining feature of human emphysema. Am Rev Respir Dis 1988; 137: 380–392
- Sanders C, Nath P H, Bailey W C. Detection of emphysema with computed tomography: correlation with pulmonary function tests and chest radiography. Invest Radiol 1988; 23: 262–266
- Morrison N J, Abboud R T, Ramadan F, Miller R R, Gibson N N, Evans K G, Nelems B, Müller N L. Comparison of single breath carbon monoxide diffusing capacity and pressure volume curves in detecting emphysema. Am Rev Respir Dis 1989; 139: 1179–1187
- Kinsella M, Müller N L, Abboud R T, Morrison N J, DyBuncio A. Quantitation of emphysema by computed tomography using a “density mask” program and correlation with pulmonary function tests. Chest 1990; 97: 315–321
- Knudson R J, Standen J R, Kaltenborn W T, Knudson D E, Rehm K, Habib M P, Newell J D. Expiratory computed tomography for assessment of suspected pulmonary emphysema. Chest 1991; 99: 1357–1366
- Klein J S, Gamsu G, Webb W R, Golden J A, Müller N L. High-resolution C T diagnosis of emphysema in symptomatic patients with normal chest radiographs and isolated low diffusing capacity. Radiology 1992; 182: 817–821
- Miniati M, Filippi E, Falaschi F, Carrozzi L, Milne E NC, Sostman H D, Pistolesi M. Radiologic evaluation of emphysema in patients with chronic obstructive pulmonary disease: chest radiography versus high resolution computed tomography. Am J Respir Crit Care Med 1995; 151: 1359–1367
- Gevenois P A, De Vuyst P, de Maertelaer V, Zanen J, Jacobovitz D, Cosio M G, Yernault J C. Comparison of computed density and microscopic morphometry in pulmonary emphysema. Am J Respir Crit Care Med 1996; 154: 187–192
- Gelb A F, Hogg J C, Müller N L, Schein M J, Kuei J, Tashkin D P, Epstein J D, Kollin J, Green R H, Zamel N, Elliot W M, Hadjiaghai L. Contribution of emphysema and small airways in COPD. Chest 1996; 109: 353–359
- Mishima M, Hirai T, Itoh H, Nakano Y, Sakai H, Muro S, Nishimura K, Oku Y, Chin K, Ohi M, Nakamura T, Bates J HT, Alencar A M, Suki B. Complexity of terminal airspace geometry assessed by lung computed tomography in normal subjects and patients with chronic obstructive pulmonary disease. Proc Natl Acad Sci U SA 1999; 96: 8829–8834
- Baldi S, Miniati M, Bellina C R, Battolla L, Catapano G, Begliomini E, Giustini D, Giuntini C. Relationship between extent of pulmonary emphysema by high-resolution computed tomography and lung elastic recoil in patients with chronic obstructive pulmonary disease. Am J Respir Crit Care Med 2001; 164: 585–589
- Gevenois P A, De Vuyst P, Sy M, Scillia P, Chaminade L, de Maertelaer V, Zanen J, Yernault J C. Pulmonary emphysema: quantitative CT during expiration. Radiology 1996; 199: 825–829
- Hosmer D W, Lemeshow S. Applied Logistic Regression. John Wiley & Sons, New York 1989; 83
- Heath D, Smith P. Pulmonary vascular disease. Med Clin North Am 1977; 61: 1279–1307
- Barst R J, McGoon M, Torbicki A, Sitbon O, Krowka M J, Olschewski H, Gaine S. Diagnosis and differential assessment of pulmonary arterial hypertension. J Am Coll Cardiol 2004; 43: 40S–47S
- Rubin L J, Badesch D B, Barst R J, Galiè N, Black C M, Keogh A, Pulido T, Frost A, Roux S, Leconte I, Landzberg M, Simonneau G, for the Bosentan Randomized Trial of Endothelin Antagonist Therapy Study Group. Bosentan therapy for pulmonary arterial hypertension. N Engl J Med 2002; 346: 896–903
- Hoeper M M, Oudiz R J, Peacock A, Tapson V F, Haworth S G, Frost A E, Torbicki A. End points and clinical trial designs in pulmonary arterial hypertension. Clinical and regulatory perspectives. J Am Coll Cardiol 2004; 43: 48S–55S
- Speizer F, Comstock G. Recommended respiratory disease questionnaires for use with adults and children in epidemiological research, In: Ferris B G, ed. Epidemiology standardization project. Am Rev Respir Dis 1978; 118: 7–53
- Enright P L, Sherrill D L. Reference equations for the six-minute walk in healthy adults. Am J Respir Crit Care Med 1998; 158: 1384–1387