Abstract
This study attempts to assess whether canopy spectra of mangrove species and mudflat spectra recorded under field conditions contain adequate spectral information for discerning mangroves at species rank and mudflats at the class level. This article highlights the hyperspectral characteristics of canopies of 17 tropical mangrove species, belonging to nine families, creek water and four mudflat classes found in the tidal forests of Indian Sundarbans. Hyperspectral observations were recorded using a field spectroradiometer, and pre-processed and averaged reflectance values of samples were subjected to various statistically tests such as k-means cluster analysis, one-way analysis of variance (ANOVA), stepwise linear discrimination and factor analysis. k-means cluster analysis showed highest Euclidean distance between Rhizophoraceae and Myrsinaceae/Plumbaginaceae. ANOVA results indicated that all the canopy spectra were statistically different at all the spectral locations except one with majority of the bands exhibiting 99% confidence level, and for the mudflat classes and creek water, all bands showed p < 0.01. Discriminant analysis was performed in different combinations/cases to identify the bands for maximum separability. Optimal Wilks' Lambda (L) were achieved with two, six, four, eight, one, four, two and three for differentiating canopies of the species of Avicennia, Sonneratia, Xylocarpus, Bruguiera, Ceriops, Bruguiera and Rhizophora, all species of Rhizophoraceae, two species of Arecaceae, respectively. For mudflat classes and creek water, the best possible Wilks' Lambda were attained with four, five and two for discriminating upper, intermediate and mud lower, mud lower and water, mud upper with and without roots, correspondingly. Factor analysis was the tool used to identify the wavelengths that were uncorrelated and contained maximum information in the combination of selected wavelengths. The most significant bands for canopy discrimination were 960, 970, 1000, 1070, 1120, 1160, 2070, 2080, 2150, 2200, 2240 and 2340 nm; for discrimination amongst mudflat classes and creek water, the bands were 540, 550, 730, 740, 770, 780, 880, 1190, 1290, 2010 and 2150 nm. Overall, hyperspectral data showed potential for discriminating between mangrove canopies of different species and for discerning mudflat classes. The outcomes of the study also indicated the efficacy of the applied statistical tools for discrimination.
1. Introduction
Mangrove forests are found in the intertidal zones of tropical and subtropical coastlines (Kathiresan and Bingham Citation2001) and exist as an ecosystem, comprising estuaries, lagoons, creeks and intertidal mudflats. The forests are expanses of spreading trees with numerous prop roots, stilt roots, knee roots and pneumatophores. The system is sensitive to changes in the local hydrological environment, and the changes are typically manifested through alterations in the species or community composition. Of late, mangroves have received much ecological attention, partly because of the alarming rate at which they are being modified or completely destroyed and partly because of their recognition as highly productive ecosystems (Mann Citation1982). Remote sensing has now emerged as a sustainable tool for mapping and monitoring of mangrove areas (Blasco et al. Citation1998), primarily because this technology allows information to be collected from locations that would be otherwise difficult to survey. Multispectral broadband optical sensor data have been widely used to map and monitor the mangrove areas of the world (Sulong et al. Citation2002; Guebas et al. Citation2004; Myint et al. Citation2008; Berlanga-Robles and Ruiz-Luna Citation2011; Giri et al. Citation2011). India contains a large number of mangrove swamps. Mapping and monitoring of these areas using multispectral data from IRS sensors has been done periodically (Reddy, Pattanaik, and Murthy Citation2007; SAC Citation2010; FSI Citation2011). However, information on the floristic composition of the mangroves using remote sensing data is still at developmental stage. The broadband multispectral remote sensing sensors have been found to be inadequate to discriminate the mangrove classes at genus or species level (Holmgren and Thuresson Citation1998). In this context, hyperspectral remote sensing has a significant role to play because of its ability to differentiate subtle differences in biophysical and biochemical attributes of plants (Schmidt and Skidmore Citation2003). Many studies have been carried out in this direction using airborne and satellite hyperspectral data (Held et al. Citation2003; Hirano, Madden, and Welch Citation2003; Lucas et al. Citation2003; Jusoff Citation2006; Jensen et al. Citation2007; Yang et al. Citation2009; Chakravortty and Chakrabarti Citation2011; Kamal and Phinn Citation2011; Kumar et al. Citation2013). The potential of in situ hyperspectral data for discriminating species composition of terrestrial plants (Schmidt and Skidmore Citation2003) and hydrophytes (Sun et al. Citation2008) have been reported.
In terms of the continuity trait of hyperspectral data, it becomes possible to apply many statistical tests to analyse hyperspectral data (Thenkabail et al. Citation2004). Since a large number of bands are available, the selection of the optimum bands is required for vegetation identification. Use of J-M distance indices for separation of leaves of some mangroves species has been reported. Vaiphasa et al. (Citation2005, Citation2007) also used a genetic search algorithm-based selector for selecting a subset of bands that maintained spectral separability between mangrove species classes of Sawi Bay, Chumporn province, Thailand. Discrimination of four mangrove species and one mangrove associate from Tok Bali, Malaysia, using hyperspectral leaf reflectance measurements was carried out by Kamaruzaman and Kasawani (Citation2007). A similar experiment was conducted by Wang and Sousa (Citation2009) for discerning three mangrove species from Caribbean coast of Panama. The authors also used some ratio indices for detecting stress in these mangroves. Leaves of four mangrove species of Indian Sundarbans and Gulf of Kachchh, Gujarat, India, were discriminated using different statistical protocols (Panigrahy, Kumar, and Manjunath Citation2012). All these studies clearly demonstrated the use of foliar spectral measurements. However, canopy spectra are of greater importance than leaf spectra in the context of satellite remote sensing data. In the present study, canopy spectra was used for discrimination of mangrove species.
Mudflat is an integral part of a mangrove ecosystem and represents the first physiographic zone in a mangrove ecosystem where phyto-succession begins (Naskar and Guha Bakshi Citation1987). This zone exhibits great variability in composition, being flanked by river or creek water on one side and mangrove forest slope on the other. Thus, characterizing the mudflats is also an important requirement in remote sensing studies.
The objective of this study was to evaluate the utility of hyperspectral data for discrimination of canopies of mangrove species and mudflat classes (and creek water) using ground-based hyperspectral data and to apply different statistical tools to harness essential information from the collected data.
2. Study area and targets
Sundarbans (21° 31′ N to 22° 30′ N and 88° 10′ E to 89° 51′ E), one of the world's largest blocks of mangrove ecosystem lying in the delta of two rivers, the Ganges and the Brahmaputra of the Indian subcontinent, is the study area. Around 37 species of obligate mangroves and 32 mangrove associates have been reported in this area (Banerjee, Sastry, and Nayar Citation1989). The study area of this work was the mangrove habitat of Bonnie Camp (21° 49′ 45′′–21° 49′ 49′′ N and 88° 37′ 32′′–88° 37′ 36′′ E) at Raidighi range of the Indian Sundarbans (). The targets for spectral measurements were canopies of different mangrove species, mudflat classes and creek water. Altogether, 17 mangrove species (from both natural forests and plantations nurtured under natural conditions) were investigated in this study (). Mudflat classes considered were lower zone mudflat/mud lower (zones or areas adjacent to creek water), mud intermediate, mud upper (without exposed roots) and mud upper with roots (), with the amount of water being of the order mud lower > intermediate > upper. Reflectance measurements were also taken for creek water (having salinity ranging from 10 to 15 ppt). Mud upper with roots are the transitional areas between mudflats (mud upper) and slopes.
Figure 1. Location of the study area – Bonnie Camp area at Raidighi Range, Indian Sundarbans, West Bengal.
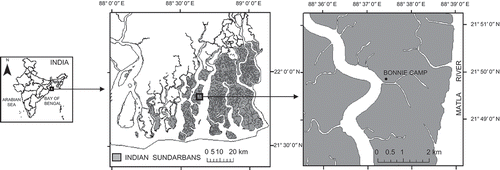
Table 1. List of mangrove species covered under this study
Figure 2. Profile showing different physiographic zones, mudflat classes (b–e), creek water, tide levels and phyto-association in the Sundarbans Reserve Forest area. The figure also shows field photographs (dated 10.12.09 and 11.12.09) of creek water (a), mud lower (b), mud intermediate (c), mud upper without roots (d) and mud upper with roots (e).
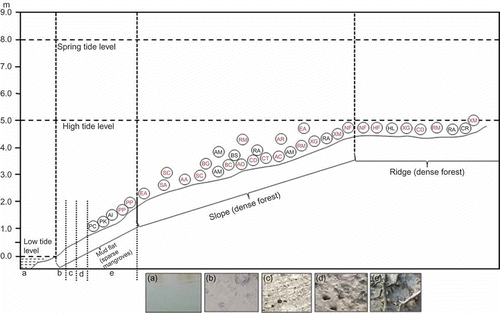
3. Methodology
3.1. Spectral measurements
Spectral measurements of targets (canopies of mangrove species, mudflat classes and creek water) were taken during low tide conditions in December 2009 and on cloud-free days from 10 to 11 a.m. (local daytime hours). Based on the availability of the mangrove species, trees were randomly selected from every physiographic zone (e.g. mudflat, slope and ridge). Measurements were taken using a hyperspectral spectroradiometer having 2151 channels in the range of 350–2500 nm (ASD Citation2008). The instrument acquired hyperspectral data at the spectral resolution of 3 nm. The sampling interval was 1.4 nm for the spectral region 350–1500 nm and 2 nm for 1000–2500 nm, and resampling the instrument delivered data with 1 nm interval. The sensor, equipped with a field-of-view (FOV) 25°, was positioned 1 m above the target at the nadir position. During canopy measurements, it was ensured that the FOV was fully covered by the canopy of the concerned species. At FOV of 25°, the ground range is 35 cm (diameter). The aim was to make measurements under natural conditions. The instrument was positioned 1 m above the canopy/branch of the canopy; approximately it was about 3 m (2 m for Phoenix paludosa and Nypa fruticans) above the ground [total (3 or 2) + 1 m]. Since the tree species were more than 10 years old and had good canopy cover with multiple layers, the background was more or less completely covered with leaves, twigs and branches. Hence, the contribution from background on the reflectance was negligible for most of the species. However, owing to the presence of large compound leaves in P. paludosa and N. fruticans, the background effect is unavoidable in these two species.
The spectral response of each target (canopy of a single tree/mudflat class/creek water at a particular place) was recorded 10 times. The 10 readings were averaged to construct a radiance curve; the radiance was converted to a reflectance curve, using a Spectralon reference panel and the correction of the spectroradiometer dark current. The reflectance of a target is the ratio of energy reflected off the target to that incident on the target. In this study, 30 reflectance curves were constructed per mangrove species canopy, mudflat class and creek water (in total 510 curves for canopies, 120 for mudflat classes and 30 for creek water). Window-based software View Spec Pro (FieldSpec® 3 Citation2008) was used for viewing, analysing and exporting the spectral data.
3.2. Data treatments and statistical tests
3.2.1. Hyperspectral data pre-processing
Reflectance profiles containing spectral wavelengths from 350 to 2500 nm with 1 nm interval were subjected to t-tests for outlier removal. Moreover, measurements near the water absorption bands and the extreme short-wave infra-red (SWIR) bands yielded only noisy data. As a result, spectral bands from 391 nm to 1340 nm, from 1431 nm to 1790 nm and from 1971 nm to 2340 nm were used for further analysis. However, previous studies (Broge and Leblanc Citation2000; Thenkabail et al. Citation2004) have shown that wavebands in immediate neighbourhood of one another provide similar information, hence become redundant. Given these facts, the spectral data were averaged over 10 nm; thus, reducing the number of data points. The pre-processed data were subjected to the following statistical tests, which were performed using statistical software SPSS 16.0.
3.2.2. k-means cluster analysis for grouping the mangrove families
k-means cluster analysis was performed to classify the spectra of nine mangrove families into clusters or groups and distances were computed using Euclidean distance. To assess the stability of a given solution, results were compared from analyses with different permutations of the initial centre values. This procedure was attempted to identify relatively homogeneous groups of families (Anderberg Citation1973). Cluster membership of the families and distance information were tabulated.
3.2.3. ANOVA tests
The averaged reflectance values of canopies of the 17 mangrove species (belonging to nine families) and four mudflat classes (and creek water) were subjected to one-way analysis of variance (ANOVA) separately to see whether at least one couple of reflectance was statistically different at every spectral band.
3.2.4. Spectral separability
Spectral separability test was used to minimize the chance of TYPE I error (Vaiphasa et al. Citation2005) found in the ANOVA test. The method adopted for spectral separability in this study was stepwise linear discriminant analysis (SDA). SDA was performed to identify the bands for maximum separability in the cases/pairs/combinations of the species/mudflat classes (including creek water) ( and ) taken separately. The Wilks' Lambda (L), a measure of discrimination used in the selection of bands, is given by (Green and Caroll Citation1978).
Table 2. Cluster membership of the nine mangrove families
Table 3. Distances between the final cluster centres
Table 4. Stepwise linear discriminant analysis (SDA), combination of selected wavelengths (λ), Wilks' Lambda (L) and F statistics in the steps of SDA in different cases for canopies
Table 5. Stepwise linear discriminant analysis (SDA), combination of selected wavelengths (λ), Wilks' Lambda (L) and F statistics in the steps of SDA for different cases of mudflat classes and creek water
A combination of low Wilks' Lambda and high F-value showed higher discrimination, and a zero value of Lambda indicated perfect separability. This method also helped in the reduction of dimensionality of the data.
3.2.5. Factor analysis
The averaged data were also subjected to factor analysis [separately for the two sets of data, viz., canopies and mudflat classes (with creek water)], which attempts to identify underlying variables (here wavelengths) that explain the pattern of correlations within a set of observed variables. In this study, this analysis was not used for data reduction, but as an added tool to identify the wavelengths that were uncorrelated and contained maximum information in the combination of selected wavelengths obtained by means of SDA. The method used for extraction was principal component analysis, which forms uncorrelated linear combinations of the observed variables. The first component has maximum variance. Successive components explain progressively smaller portions of the variance and are all uncorrelated to each other. The technique adopted for rotation was Varimax method with Kaiser Normalization, which is an orthogonal rotation method that minimizes the number of variables that have high loadings on each factor (Norusis Citation2004). This method simplifies the interpretation of the factors. Eigenvalues over one were only extracted, and principal components were analysed on the basis of correlation matrix.
A flowchart for the methodology is provided in .
4. Results
4.1. k-means cluster analysis for grouping the mangrove families
Reflectance spectra of canopies of 17 mangrove species belonging to nine families are presented in . k-means cluster analysis of the spectra revealed that the families could be broadly grouped into three clusters (). Rhizophoraceae (comprising members of – Bruguiera, Ceriops and Rhizophora), and Mysinaceae (including single species – Aegiceras corniculatum) with Plumbaginaceae (Aegialitis rotundifolia) formed two separate clusters (cluster 1 and 3, respectively). Avicenniaceae, Euphorbiaceae, Sterculiaceae and Arecaceae could be grouped into a single cluster (cluster 2), while Meliaceae and Sonneratiaceae could be assigned to either of the clusters – 1 or 2 and 2 or 3, respectively. The distance between the final cluster centres was of the order 1 and 3 > 1 and 2 > 2 and 3 ().
4.2. ANOVA tests
In case of the canopy spectra, all the wavelengths except one (wavelength 2010 nm – p > 0.05) registered 99% confidence level (p < 0.01). For the mudflat classes and creek water (reflectance spectra given in ), all bands showed p < 0.01. Reflectance canopy spectra of 13 species (species of Avicennia, Sonneratia, Xylocarpus, species belonging to Arecaceae and members of Rhizophoraceae) are presented in –e to provide a view of the mangrove canopy spectrum continuum after pre-processing.
4.3. SDA
The best possible Wilks' Lambda was achieved with two, six, four, eight, one, four, two and three bands for differentiating canopies of the species of Avicennia, Sonneratia, Xylocarpus, Bruguiera, Ceriops, Bruguiera and Rhizophora, all the species of Rhizophoraceae, the two species of Arecaceae, respectively (). The discrimination between the two species of Sonneratia was more significant (L = 0.004) than the other cases. In case of the mudflat classes and creek water, the optimal Wilks' Lambda values were attained with four, five and two for discriminating upper, intermediate and mud lower (0.000, 12,77,951.33), mud lower and water (0.000, 17,97,875.91), mud upper with and without roots (0.001, 6554.99), respectively ().
4.4. Factor analyses
The screen plots (a and c) of the factor analyses revealed that the inherent dimensionality of the canopies and mudflat (and water) data were five and three, respectively. The information content reduced from component 1 to 2 and became very low in 3 for mudflat classes and creek water and onwards for canopies. Factor loadings (correlation coefficients) for the main factors resulting from principal component analysis and rotation component matrix are presented in and d. Out of 166, 103 and 152 wavelengths exhibited correlation coefficients ≥0.9 in the rotation component matrices for canopy and mudflat (and creek water), correspondingly. The combination of wavelengths in bold ( and ) could be considered as the uncorrelated and more important wavelengths out of the selected combination of bands for discrimination amongst the canopies of mangrove species and amongst the mudflat classes and creek water.
Figure 6. Pair-wise (species of the same genus) elucidation of reflectance spectra of canopies (a, b, c and d). Canopy reflectance profiles of the members of Rhizophoraceae (e).
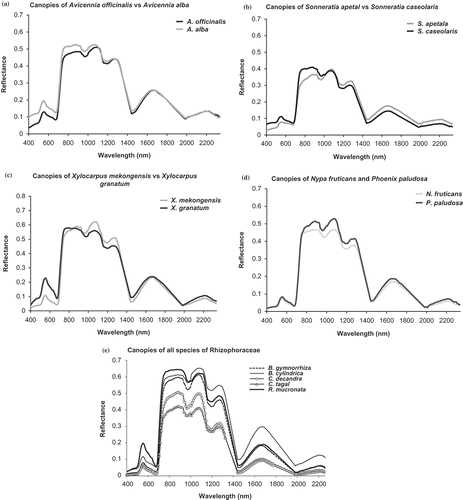
Figure 7. Scree plot of eigenvalues plotted against component numbers – for canopies (a) and mudflat classes and creek water (c). Rotation component matrix of component 1, 2, 3, 4 [canopies (b)] and 1, 2, 3 [mudflat classes and water (d)]. Filled triangles/squares/circles depict wavelengths exhibiting correlation coefficients ≥0.9.
![Figure 7. Scree plot of eigenvalues plotted against component numbers – for canopies (a) and mudflat classes and creek water (c). Rotation component matrix of component 1, 2, 3, 4 [canopies (b)] and 1, 2, 3 [mudflat classes and water (d)]. Filled triangles/squares/circles depict wavelengths exhibiting correlation coefficients ≥0.9.](/cms/asset/7be4fc43-cfec-4e4c-bdd7-5c4f56fe3bc1/tgrs_a_814275_o_f0007g.gif)
5. Discussion
The general shape of spectra was found to be similar in all the 17 species of mangroves. This may be attributed to the similar biochemical and anatomical composition of vegetation across species. The leaf biochemical information is transmitted virtually unchanged from the leaf to the canopy in near-infrared wavelengths (Asner Citation1998). There is also multiple scattering within the leaves and canopy that causes broadening of absorption features, and reflectance from absorption features tend towards an asymptote when saturation is reached (Curran Citation1989). However, as pigment concentrations, biochemical contents, leaf characteristics and canopy structure vary between species, so does absorption and reflectance (Asner Citation1998; Martin et al. Citation1998). As a result, generalizations cannot be made across different vegetation types as a whole and mangrove species in particular (Knapp and Carter Citation1998).
Dawson and Curran (Citation1998) reported that canopy spectra remain sensitive to variations in foliar biochemical content, leaf area index, canopy closure, under storey reflectance, foliar water and lignin-cellulose content. Kumar et al. (Citation2001) represented vegetation canopy reflectance as a function of leaf biochemistry, leaf morphology (as represented by leaf scattering properties), leaf thickness and anatomy, soil reflectance and canopy architecture.
The reflectance characteristics of the canopies of the species with isolateral leaves (Sonneratia sp. and Phoenix paludosa) are very different from the dorsiventral ones (the remaining species). This may be mainly attributed to the differences in cellular structure and pigmentation. A similar fact was also pointed out by Panigrahy, Kumar, and Manjunath (Citation2012). Reflectance in the green domain of the spectrum was most pronounced in X. granatum (>0.2), followed by Rhizophora sp. and A. alba (∼0.2) []. This may be attributed to the thickness of the assimilatory zone containing chlorenchymatous tissue per leaf, which is greater in X. granatum and Rhizophora sp. in comparison to the other species (Tomlinson Citation1994). The reflectance in the near infra-red (NIR) domain (particularly from 900 to 1300 nm) was higher in the species of Rhizophora, Bruguiera and Xylocarpus, thereby indicating that scattering because of multiple refractions and reflections at the boundary between cellular walls and air spaces in the mesophyll tissue are more in these species. Higher reflectances in the NIR region for these species might also be attributed to denser canopies (with minimum exposure of background) than those in the other species. In the middle infra-red (MIR) domain (1300–2340 nm), B. cylindrica showed highest reflectance. This could be explained by the fact that the leaves of this species register least thickness of colourless tissue storing water than the leaves of the remaining species (Das and Ghose Citation1996). Amongst the members of Rhizophoraceae, the thickness of this achlorophyllous tissue is maximum in Ceriops; similarly, Sonneratia also contains considerable amount of this water storage tissue (Seshavatharam and Srivalli Citation1989; Nandy (Datta), Das, and Ghose Citation2005; Yuangue, Zhongbao, and Peng Citation2009). Hence, low reflectance values were observed in the MIR regions for these two genera. Because of the presence of colourless water storage tissue at different levels of mesophyll and hypodermal layers, mangrove leaves become thick and succulent, which can be correlated with the extra water storage capacity. Comparatively, succulent leaves occur in those plants, such as in C. decandra and Sonneratia sp., which usually grow in the slope regions, where salinity is quite high (Das and Ghose Citation1996). Wehe (Citation1964) has experimentally proven that succulent leaves are a common feature of most mangroves, and leaf succulence increases with the increase of substrate salinity.
The species under the same genus and in some cases under the same family [e.g. the members of Rhizophoraceae (–e)] visually exhibit similar phyllotaxy and branching pattern, thus, virtually the same canopy structure. This is also supported by the outputs of k-means cluster analysis, in which this family has been grouped into a separate cluster. The grouping of Meliaceae with either cluster 1 or cluster 2 might be attributed to the similarity in the canopy architecture of Xylocarpus and members of Rhizophoraceae, Avicenniaceae, Euphorbiaceae and Sterculiaceae. Inclusion of Arecaceae in cluster 2 might be due to comparable amount of soil/background reflectance with the members of cluster 2 as both Nypa and Phoenix are characterized by large compound leaves in a terminal crown. Grouping of Sonneratiaceae to cluster 2 might be due to the isolateral nature of Sonneratia and Phoenix (Arecaceae) leaves; again, assignment of Sonneratiaceae to cluster 3 might be due to similar proportions of background reflectance (though negligible). Since the species under the same genus exhibit more or less the same canopy structure, the statistical tools applied in the present work could provide supplementary information for species discrimination at the canopy level.
Water showed lowest reflectance values beyond 700 nm, and the values for the mudflat classes were of the order mud upper (without roots) > mud intermediate > mud lower > mud upper with roots. The general pattern of the spectra was similar, and the spectral response could be directly correlated to the content of water in the soils (absorption peaks at ∼1450 and ∼1950 nm, ). This observation supports the fact that increasing moisture content generally decreases soil reflectance across the entire spectrum (de Jong and Epema Citation2001). Thus, mud lower, intermediate and upper are typical examples of wet soils. The selected bands (), which could be reconciled with the locations of the spectral responses, were 1190 and 1290 nm for water (Pieters and Englert Citation1993) and 550 nm for iron (Baumgardner et al. Citation1985). Out of all the mudflat classes, mud upper with roots recorded lowest reflectance values. This could be attributed to the presence of more organic matter in the soils contributed by way of decay and decomposition of dead roots. Moreover, available literatures confirm that Sundarban soils are characterized by high organic matter (Kumar and Ghose Citation2007). Furthermore, an increase in the organic matter content of a soil causes a decrease in the reflectance over the entire spectrum that is similar to moisture (Iron, Weismiller, and Petersen Citation1989). Overall, from the outputs of SDA and the maximum number of selected bands in a particular case ( and ), it is evident that the factors that influence soil reflectance seem to act over less specific spectral bands than vegetation.
The combinations of selected uncorrelated bands for species discrimination could be documented for future reference. ( provides a summary of the outcomes of the present work.) The selected wavelengths for differentiating between mudflat classes and creek water could be used as an added protocol for discerning wet soils of different inundation levels.
Table 6. Summary table for the major outcomes of the present work
6. Conclusion
Hyperspectral remote sensing is now the thrust area of research to improve the information content of the target. Detailed inventory of mangrove ecosystem, including species composition and characterization of mudflats, is one of the potential applications of hyperspectral remote sensing. Ground-based field observations provide an insight to the utility of hyperspectral data in this direction. However, in the context of satellite remote sensing, it is essential to study the canopy spectra, rather than the leaf spectra. This study analysed the spectral responses of canopies of 17 mangrove species belonging to 9 families, 4 mudflat classes and creek water generally associated with mangrove ecosystem, with the aim of best band selection for discrimination of the targets. On the one hand, based on k-means cluster analysis at the family level, Rhizophoraceae had highest discrimination from Myrsinaceae and Plumbaginaceae; on the other hand, based on SDA at the species level, discrimination amongst the studied species of Rhizophoraceae was lowest. Out of all the pairs/cases/combinations, discrimination between S. alba and S. caseolaris was highest. Around 25 spectral bands got selected for mangrove species discrimination. Frequently, 400, 1000 and 1730 nm spectral bands were part of the combinations of selected bands in discrimination. The most important bands were in the NIR (960, 970, 1000, 1070, 1120 and 1160 nm) and MIR (2070, 2080, 2150, 2200, 2240 and 2340 nm) regions of the spectra. Discrimination within mudflat classes and creek water required about 11 bands, distributed in the visible (540 and 550 nm), NIR (730, 740, 770, 780, 880, 1190 and 1290 nm) and MIR (2010 and 2150 nm) regions.
This article, thus, deals with the potentials of some of the statistical techniques for discrimination amongst the targets using ground-collected hyperspectral data; especially, it focuses on the use of stepwise linear discrimination for obtaining a combination of selected bands and factor analysis as an added tool for identification of wavelengths that are uncorrelated and contain maximum information in the combination of selected bands. Simultaneous application of both the tools may be carried out to harness essential information from the in situ hyperspectral data.
Acknowledgements
The work has been carried out under the ISRO-EOAM project “Hyperspectral Studies” at Space Applications Centre. We are grateful to Shri Pradeep Vyas, IFS, Director, Sunderban Biosphere Reserve, Kolkata, for granting permission to take scientific measurements for this study and logistics support. We are thankful to Dr. J. S. Parihar, Deputy Director, SAC, for the encouragement received to take up this assignment. We are also grateful to the two anonymous reviewers for their critical comments.
Notes
References
- Anderberg , M. R. 1973 . Cluster Analysis for Applications , 359 New York : Academic Press .
- ASD. 2008. RS3™ User Manual ASD Document 600545 Rev. E © 2008. Boulder, CO: Analytical Spectral Device Inc. http://www.asdi.com (http://www.asdi.com)
- Asner , G. P. 1998 . Biophysical and Biochemical Sources of Variability in Canopy Reflectance . Remote Sensing of Environment , 64 ( 3 ) : 234 – 253 .
- Banerjee , L. K. , Sastry , A. R. K. and Nayar , M. P. 1989 . Mangroves in India, Identification Manual , 110 Kolkata : Botanical Survey of India .
- Baumgardner , M. F. , Stoner , E. R. , Silva , L. F. and Biehl , L. L. 1985 . “ Reflectance Properties of Soils ” . In Advances of Agronomy , Edited by: Brady , N. 1 – 44 . New York : Academic Press .
- Berlanga-Robles , C. A. and Ruiz-Luna , A. 2011 . Integrating Remote Sensing Techniques, Geographical Information Systems (GIS), and Stochastic Models for Monitoring Land Use and Land Cover (LULC) Changes in the Northern Coastal Region of Nayarit, Mexico . GIScience & Remote Sensing , 48 ( 2 ) : 245 – 263 .
- Blasco , F. , Gauquelin , T. , Rasolofoharinoro , M. , Denis , J. , Aizpuru , M. and Caldairou , V. 1998 . Recent Advances in Mangrove Studies Using Remote Sensing Data . Marine and Freshwater Research , 49 ( 4 ) : 287 – 296 .
- Broge , N. H. and Leblanc , E. 2000 . Comparing Prediction Power and Stability of Broadband and Hyperspectral Vegetation Indices for Estimation of Green Leaf Area Index and Canopy Chlorophyll Density . Remote Sensing of Environment , 76 ( 2 ) : 156 – 172 .
- Chakravortty , S. and Chakrabarti , S. 2011 . Pre-processing of Hyperspectral Data: A Case Study of Henry and Lothian Islands in Sunderban Region, West Bengal, India . International Journal of Geomatics and Geosciences , 2 ( 2 ) : 490 – 501 .
- Curran , P. J. 1989 . Remote Sensing of Foliar Chemistry . Remote Sensing of Environment , 30 ( 2 ) : 271 – 278 .
- Das , S. and Ghose , M. 1996 . Anatomy of Leaves of Some Mangroves and their Associates from Sundarbans (West Bengal) . Phytomorphology , 46 ( 2 ) : 139 – 150 .
- Dawson , T. P. and Curran , P. J. 1998 . A New Technique for Interpolating the Reflectance Red Edge Position . International Journal of Remote Sensing , 19 ( 11 ) : 2133 – 2139 .
- de Jong , S. M. and Epema , G. F. 2001 . “ Imaging Spectrometry for Surveying and Modeling Land Degradation ” . In Imaging Spectrometry , Edited by: van de Meer , F. and de Jong , S. M . 65 – 86 . Dordrecht : Kluwer Academic Press .
- FieldSpec® 3. 2008. User Manual ASD Document 600540 Rev. H © 2008. Boulder, CO: Analytical Spectral Device Inc. http://www.asdi.com (http://www.asdi.com)
- FSI. 2011. State of Forest Report. Dehradun: Forest Survey of India, Ministry of Environment and Forests. http://www.fsi.org.in/final_2011.pdf (http://www.fsi.org.in/final_2011.pdf)
- Giri , C. , Ochieng , E. , Tieszen , L. L. , Zhu , Z. , Singh , A. , Loveland , T. , Masek , J. and Duke , N. 2011 . Status and Distribution of Mangrove Forests of the World Using Earth Observation Satellite Data . Global Ecology and Biogeography , 20 ( 1 ) : 154 – 159 .
- Green , P. E. and Caroll , J. D. 1978 . Mathematical Tools for Applied Multivariate Analysis , New York : Academic Press .
- Guebas , D. F. , van Hiel , J. Elly , Chan , C. W. , Jayatissa , L. P. and Koedam , N. 2004 . Qualitative Distinction of Congeneric and Introgressive Mangrove Species in Mixed Patchy Forest Assemblages Using High Spatial Resolution Remotely Sensed Imagery (IKONOS) . Systematics and Biodiversity , 2 ( 2 ) : 113 – 119 .
- Held , A. , Ticehurst , C. , Lymburner , L. and Williams , N. 2003 . High Resolution Mapping of Tropical Mangrove Ecosystems Using Hyperspectral and Radar Remote Sensing . International Journal of Remote Sensing , 24 ( 13 ) : 2739 – 2759 .
- Hirano , A. , Madden , M. and Welch , R. 2003 . Hyperspectral Image Data for Mapping Wetland Vegetation . Wetlands , 23 ( 2 ) : 436 – 448 .
- Holmgren , P. K. and Thuresson , T. T. 1998 . Satellite Remote Sensing for Forestry Planning . Journal of Forestry Research , 13 ( 1 ) : 90 – 110 .
- Iron , J. R. , Weismiller , R. A. and Petersen , G. W. 1989 . “ Soil Reflectance ” . In Theory and Applications in Optical Remote Sensing , Edited by: Asrar , G. 66 – 106 . New York : Wiley .
- Jensen , R. , Mausel , P. , Dias , N. , Gonser , R. , Yang , C. , Everitt , J. and Fletcher , R. 2007 . Spectral Analysis of Coastal Vegetation and Land Cover Using AISA+ Hyperspectral Data . Geocarto International , 22 ( 1 ) : 17 – 28 .
- Jusoff , K. 2006 . Individual Mangrove Species Identification and Mapping in Port Klang Using Airborne Hyperspectral Imaging . Journal of Sustainability Science and Management , 1 ( 2 ) : 27 – 36 .
- Kamal , M. and Phinn , S. 2011 . Hyperspectral Data for Mangrove Species Mapping: A Comparison of Pixel-Based and Object-Based Approach . Remote Sensing , 3 ( 10 ) : 2222 – 2242 .
- Kamaruzaman , J. and Kasawani , I. 2007 . Imaging Spectrometry on Mangrove Species Identification and Mapping in Malaysia . WSEAS Transactions on Biology and Biomedicine , 4 ( 8 ) : 118 – 126 .
- Kathiresan , K. and Bingham , B. L. 2001 . Biology of Mangroves and Mangrove Ecosystems . Advances in Marine Biology , 40 : 81 – 251 .
- Knapp , A. K. and Carter , G. A. 1998 . Variability in Leaf Optical Properties Among 26 Species from a Broad Range of Habitats . American Journal of Botany , 85 ( 7 ) : 940 – 946 .
- Kumar , L. , Schmidt , K. , Durry , S. and Skidmore , A. K. 2001 . “ Imaging Spectrometry And Vegetation Science ” . In Imaging spectrometry , Edited by: van de Meer , F. and de Jong , S. M. 111 – 155 . Dordrecht : Kluwer Academic Press .
- Kumar , T. and Ghose , M. 2007 . Effect of Tidal Inundation on Some Chemical Properties of Soil of the Sundarbans (India) . Annals of Tropical Research , 29 ( 2 ) : 137 – 147 .
- Kumar , T. , Panigrahy , S. , Kumar , P. and Parihar , J. S. 2013 . Classification of Floristic Composition of Mangrove Forests Using Hyperspectral Data: Case Study of Bhitarkanika National Park . Journal of Coastal Conservation Planning and Management , 17 ( 1 ) : 121 – 132 .
- Lucas , R. M. , Mitchell , A. , Donnelly , B. and Milne , T. 2003 . “ Characterising and Mapping Mangroves in Northern Australia Using Stereo Aerial Photography and Hyperspectral CASI Data ” . In Geoscience and Remote Sensing Symposium Proceedings IGARSS Vol. 4 , 2380 – 2382 . 2003
- Mann , K. H. 1982 . Ecology of Coastal Waters: A Systems Approach , 322 Berkeley , CA : University of California Press .
- Martin , M. E. , Newman , S. D. , Aber , J. D. and Congalton , R. G. 1998 . Determining Forest Species Composition Using High Spectral Resolution Remote Sensing Data . Remote Sensing of Environment , 65 ( 3 ) : 249 – 254 .
- Myint , S. W. , Giri , C. P. , Wang , L. , Zhu , Z. and Gillette , S. C. 2008 . Identifying Mangrove Species and their Surrounding Land Use and Land Cover Classes Using an Object-Oriented Approach with a Lacunarity Spatial Measure . GIScience and Remote Sensing , 45 ( 2 ) : 188 – 208 .
- Nandy (Datta) , P. , Das , S. and Ghose , M. 2005 . Relation of Leaf Micromorphology with Photosysnthesis and Water Efflux in Some Indian Mangroves . Acta Botanica Croatica , 64 ( 2 ) : 331 – 340 .
- Naskar , K. R. and Bakshi , D. N. Guha . 1987 . Mangrove Swamps of the Sundarbans: An Ecological Perspective , 264 Kolkata : Naya Prokash .
- Norusis , M. 2004 . SPSS 13.0 Statistical Procedures Companion , Upper Saddle River , NJ : Prentice Hall .
- Panigrahy , S. , Kumar , T. and Manjunath , K. R. 2012 . Hyperspectral Leaf Signature as an Added Dimension for Species Discrimination: Case Study of Four Tropical Mangroves . Wetlands Ecology and Management , 20 ( 2 ) : 101 – 110 .
- Pieters , C. M. and Englert , P. A. J. 1993 . Remote Geochemical Analysis: Elemental and Mineralogical Composition , 594 New York : Cambridge University Press .
- Reddy , C. S. , Pattanaik , C. and Murthy , M. S. R. 2007 . Assessment and Monitoring of Mangroves of Bhitarkanika Wildlife Sanctuary, Orissa, India Using Remote Sensing and GIS . Current Science , 92 ( 10 ) : 1409 – 1415 .
- SAC . 2010 . Coastal Zone Studies , Ahmedabad : Space Applications Centre, SAC/EPSA/MPSG/TR/01 . 559
- Schmidt , K. S. and Skidmore , A. K. 2003 . Spectral Discrimination of Vegetation Types in a Coastal Wetland . Remote Sensing of Environment , 85 ( 1 ) : 92 – 108 .
- Seshavatharam , V. and Srivalli , M. 1989 . Systematic Leaf Anatomy of Some Indian Mangroves . Proceedings of the Indian Academy of Sciences (Plant Sciences) , 99 ( 6 ) : 557 – 565 .
- Sulong , I. , Mohd–Lokman , H. , Mohd–Tarmizi , K. and Ismail , A. 2002 . Mangrove Mapping Using Landsat Imagery and Aerial Photographs: Kemaman District, Terengganu . Malaysia Environment, Development and Sustainability , 4 : 135 – 152 .
- Sun , Y. , Liu , X. , Wu , Y. and Liao , C. 2008 . Identifying Hyperspectral Characters of Wetland Species Using in-situ Data . The International Archives of the Photogrammetry, Remote Sensing and Spatial Information Sciences , 107 : 459 – 465 .
- Thenkabail , P. S. , Enclona , E. A. , Ashton , M. S. and van der Meer , V. 2004 . Accuracy Assessments of Hyperspectral Waveband Performance for Vegetation Analysis Applications . Remote Sensing of Environment , 91 ( 3–4 ) : 354 – 376 .
- Tomlinson , P. B. 1994 . The Botany of Mangroves , 419 Cambridge : Cambridge University Press .
- Vaiphasa , C. , Ongsomwang , S. , Vaiphasa , T. and Skidmore , A. K. 2005 . Tropical Mangrove Species Discrimination Using Hyperspectral Data: A Laboratory Study . Estuarine, Coastal and Shelf Science , 65 ( 1–2 ) : 371 – 379 .
- Vaiphasa , C. , Skidmore , A. K. , de Boer , W. F. and Vaiphasa , T. 2007 . A Hyperspectral Band Selector for Plant Species Discrimination . ISPRS Journal of Photogrammetry and Remote Sensing , 62 : 225 – 235 .
- Wang , L. E. and Sousa , W. P. 2009 . Distinguishing Mangrove Species with Laboratory Measurements of Hyperspectral Leaf Reflectance . International Journal of Remote Sensing , 30 ( 5 ) : 1267 – 1281 .
- Wehe , V. 1964 . Beitrage zur Okologie der mittle und westeuropaischen Salzgetation (Gezeitenkusten) . Biet Biol Pflanzen , 39 : 189 – 237 .
- Yang , C. , Everitt , J. H. , Fletcher , R. S. , Jensen , R. R. and Mausel , P. W. 2009 . Evaluating AISA+ Hyperspectral Imagery for Mapping Black Mangrove Along the South Texas Gulf Coast . Photogrammetric Engineering and Remote Sensing , 75 ( 4 ) : 425 – 435 .
- Yuangue , L. , Zhongbao , L. and Peng , L. 2009 . “ The Study on the Leaf Anatomy of Some Mangrove Species of China ” . In Proceedings of the International Conference on Environmental Science and Information Application Technology , Vol. 3 , 47 – 51 . Washington , DC : IEEE Computer Society .