ABSTRACT
In this study, we present a new automatic deep learning-based network named Road Vectorization Network (RoadVecNet), which comprises interlinked UNet networks to simultaneously perform road segmentation and road vectorization. Particularly, RoadVecNet contains two UNet networks. The first network with powerful representation capability can obtain more coherent and satisfactory road segmentation maps even under a complex urban set-up. The second network is linked to the first network to vectorize road networks by utilizing all of the previously generated feature maps. We utilize a loss function called focal loss weighted by median frequency balancing (MFB_FL) to focus on the hard samples, fix the training data imbalance problem, and improve the road extraction and vectorization performance. A new module named dense dilated spatial pyramid pooling, which combines the benefit of cascaded modules with atrous convolution and atrous spatial pyramid pooling, is designed to produce more scale features over a broader range. Two types of high-resolution remote sensing datasets, namely, aerial and Google Earth imagery, were used for road segmentation and road vectorization tasks. Classification results indicate that the RoadVecNet outperforms the state-of-the-art deep learning-based networks with 92.51% and 93.40% F1 score for road surface segmentation and 89.24% and 92.41% F1 score for road vectorization from the aerial and Google Earth road datasets, respectively. In addition, the proposed method outperforms the other comparative methods in terms of qualitative results and produces high-resolution road segmentation and vectorization maps. As a conclusion, the presented method demonstrates that considering topological quality may result in improvement of the final road network, which is essential in various applications, such as GIS database updating.
GRAPHICAL ABSTRACT
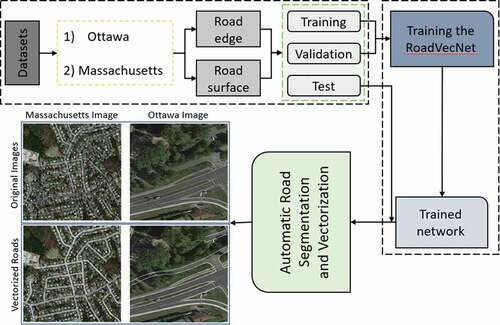
Highlights
A new RoadVecNet is applied for road segmentation and vectorization simultaneously.
SE and DDSPP modules are used to improve the accuracy.
Sobel edge detection method is used to obtain complete and smooth road edge networks
Two different Massachusetts and Ottawa datasets are used for road vectorization.
MFB_FL loss function is utilized to overcome highly unbalanced training dataset.
Data availability
The Massachusetts and Ottawa datasets and developed code that is uploaded at Github can be downloaded from the online versions at https://www.cs.toronto.edu/~vmnih/data/, https://github.com/gismodelling/RoadVecNet, and https://github.com/yhlleo/RoadNet.
Disclosure statement
No potential conflict of interest was reported by the author(s).
Author contributions
Author Contributions: A.A. carried out the investigations, analyzed the data, and drafted the article; B.P. conceptualized, supervised, visualized, project administered, resource allocated, wrote, reviewed, edited, and reorganized the article; B.P. and A.A.A. effectively enhanced the article, along with the funding. All authors have read and consented to the published version of the article.