ABSTRACT
Lower fine motor performance in childhood has been associated with poorer cognitive development and neurodevelopmental conditions such as autism spectrum disorder, yet, biological underpinnings remain unclear. DNA methylation (DNAm), an essential process for healthy neurodevelopment, is a key molecular system of interest. In this study, we conducted the first epigenome-wide association study of neonatal DNAm with childhood fine motor ability and further examined the replicability of epigenetic markers in an independent cohort. The discovery study was embedded in Generation R, a large population-based prospective cohort, including a subsample of 924 ~ 1026 European-ancestry singletons with available data on DNAm in cord blood and fine motor ability at a mean (SD) age of 9.8 (0.4) years. Fine motor ability was measured using a finger-tapping test (3 subtests including left-, right-hand and bimanual), one of the most frequently used neuropsychological instruments of fine motor function. The replication study comprised 326 children with a mean (SD) age of 6.8 (0.4) years from an independent cohort, the INfancia Medio Ambiente (INMA) study. Four CpG sites at birth were prospectively associated with childhood fine motor ability after genome-wide correction. Of these, one CpG (cg07783800 in GNG4) was replicated in INMA, showing that lower levels of methylation at this site were associated with lower fine motor performance in both cohorts. GNG4 is highly expressed in the brain and has been implicated in cognitive decline. Our findings support a prospective, reproducible association between DNAm at birth and fine motor ability in childhood, pointing to GNG4 methylation at birth as a potential biomarker of fine motor ability.
Introduction
Fine motor skills involve the fine control of fingers and hands and are important for activities such as playing an instrument, writing, cutting, and opening boxes. Efficient fine motor control requires a cascade of neuronal activities in order to manipulate objects or perform specific tasks appropriately. These motor movements also entail the age-appropriate development of related physical skills, such as core trunk control and shoulder strength, as these provide a stable base from which the arm and hand can then move with control, as well as the smaller muscles of the hands. In turn, the efficiency of fine motor skills influences the quality of manual task outcomes as well as the speed of task performance [Citation1]. Children with poor fine motor skills have difficulties developing appropriate independence in ‘life’ skills, for instance, getting dressed and feeding themselves, which has social implications within the family and peer relationships.
The early development of fine motor ability underlies and precedes cognitive functioning later in life [Citation2–8]. Repetitive rapid finger tapping is a standard test of fine motor control of the upper extremities. Hubel et al. refer to the finger-tapping test (FTT) as an efficient and precise measure of tapping speed and kinetics [Citation9], and therefore one of the most frequently used neuropsychological instruments of utility in research and clinical studies of motor performance [Citation10]. Lower performance in fine motor ability is a neuropsychological deficit that transcends diagnostic boundaries. Abnormal fine motor skills have been associated with a range of neurodevelopmental disorders, such as autism spectrum disorder (ASD) and attention deficit hyperactivity disorder (ADHD), as well as cognitive decline in ageing adults [Citation11–16]. Mental disorders, including ASD and ADHD, can be considered as the extremes of quantitative traits rather than dichotomizing the continuous variation of symptoms [Citation17–19]. Identifying the underlying mechanisms that give rise to individual differences in fine motor ability, in particular during developmentally sensitive periods of childhood, provides opportunities to understand typical neurodevelopment and cognitive function. It can also lead to new insights into the molecular processes underlying risks for related neurodevelopmental disorders in the general population [Citation20,Citation21].
Previous research has shown that fine motor ability is partially under genetic influence, with twin and familial heritability ranging from 41% to 86% on different fine motor tests [Citation22,Citation23]. Environmental factors that shape motor development may occur very early in life, for example, exposure to adverse intra-uterine environments, such as higher placental vascular resistance, malnutrition, and stress, are associated with poor motor ability, including FTT performance [Citation24–30]. Yet, how these influences affect early fine motor development at a molecular level remains unclear. Epigenetic processes, such as DNA methylation (DNAm), have emerged as a potential molecular system of interest, as they regulate gene activity in response to both genetic and environmental influences [Citation31]. DNAm at birth is particularly of interest, as it (i) may be a good proxy of genetic, biological, or prenatal environmental factors relevant to the fine motor development [Citation31,Citation32], while the epigenetic signal becomes ‘noisier’ in later life; and (ii) growing evidence shows that DNAm at birth associates more strongly with certain neurodevelopmental outcomes that also feature fine motor deficits, such as ADHD and social communication deficits [Citation33,Citation34], compared to DNAm patterns measured concurrently in childhood (i.e., prospective > cross-sectional associations). As such, DNAm patterns at birth – but not in childhood – may also mediate risk for developmental outcomes in later life (e.g., DNAm alterations at birth may impact early neurodevelopment and downstream behavioural phenotypes that persist despite changes in DNAm patterns per se).
Previous epigenetic studies have linked DNAm of candidate genes at birth, such as the glucocorticoid receptor gene NR3C1, to poorer motor performance during early development [Citation35–37]. However, evidence from epigenome-wide association studies (EWASs) is limited. We are aware of only one EWAS, which identified associations between cord blood DNAm at a cytosine-phosphate-guanine (CpG) site in SPTBN4 and infant motor function, measured using a composite score of general (fine and gross) motor skills [Citation38]. Given that research to date implicates more fine then gross motor skills as indicators of cognitive development [Citation39,Citation40], it is important to focus specifically on fine motor ability. Yet, to our knowledge, no study has examined epigenome-wide DNAm patterns associated with fine motor ability in childhood. Furthermore, it is unclear to what extent current findings replicate in independent samples, which is important for identifying reliable biomarkers.
In light of these gaps, we performed an EWAS examining long-term prospective associations between DNAm at birth (cord blood) and fine motor ability in childhood, measured by FTT at age 10 years, leveraging data from a large population-based prospective cohort, the Generation R Study (GenR). We further tested whether our findings can be replicated in an independent birth cohort. To verify the relevance of FTT to higher-order cognition in the general paediatric population, we also examined the prospective association between FTT performance and later cognitive function in adolescence (age 14 years). Finally, we investigated whether slower FTT performance mediated the association between lower DNAm at birth and lower cognitive functioning in the general paediatric population.
Methods
Study population
Detailed information for each cohort is described in the Supplementary Methods. Primary analyses were conducted using data from GenR, a population-based prospective cohort from early foetal life onwards, based in Rotterdam, the Netherlands. The design and sample characteristics of GenR have been described in detail elsewhere [Citation41]. The EWAS analyses included a subsample of 1,396 European-ancestry singletons with available DNAm data at birth. Of these, 924 ~ 1069 children had data on fine motor ability at a mean (SD) age of 9.8 (0.3) years and relevant covariates. In addition, we included 2813 participants with cognitive assessment at a mean (SD) age of 13.6 (0.3) years. A flowchart of sample selection is described in Figure S1.
The replication analyses were performed in an independent population-based prospective cohort, the INfancia Medio Ambiente (INMA) study in Spain [Citation42]. We included 326 European singletons with complete data on cord blood DNAm at birth and fine motor ability at a mean (SD) age of 6.8 (0.4) years.
DNA methylation
Briefly, DNAm from cord blood was measured using the Illumina Infinium HumanMethylation450 BeadChip in both cohorts. Pre-processing and normalization were performed in R with the CPACOR [Citation43] workflow in GenR and the Bioconductor package minfi [Citation44] in INMA. DNAm levels are characterized by beta (β) values ranging from 0 (no methylation) to 1 (full methylation). CpG sites with values outside the 25th percentile − 3 × interquartile range (IQR) and the 75th percentile+3 × IQR of the distribution were identified as outliers and winsorized.
Finger Tapping Test
In GenR, the fine motor ability was measured using a computerized finger-tapping task during an assessment visit at the age-10 years. The task was programmed in Python using modules from the PsychoPy toolbox (version 1.90.3), as previously described [Citation45,Citation46]. Briefly, children were instructed to perform five 10-second finger tapping trials in which they tapped their index fingers on a button as quickly as possible using their (1) right, (2) left, (3) both (alternating), (4) right and (5) then left hand. For the right- and left-hand conditions, we calculated the average number of taps across two trials on the same hand. For the alternating condition, the total number of taps was calculated as bimanual outcome.
In INMA, fine motor ability was measured during the age-7 assessment visit using a computerized finger-tapping task administered with E-Prime 2.0 [Citation47], first completing two trials with the preferred hand for practice, then two more trials with the non-preferred hand. The children used a standardized method by pressing a key in a joystick board; it was attached to the desk in order to avoid slips while doing the task. We calculated the mean number of taps of same-hand trials.
Cognitive Functions
Cognitive functions were measured by the Wechsler Intelligence Scale for Children-Fifth Edition (WISC-V) at 14 years of age in GenR [Citation48]. Four core subtests (i.e., Vocabulary, Matrix Reasoning, Digit Span, and Coding) were selected to produce four domain-specific index scores: verbal comprehension, fluid reasoning, working memory, processing speed. Trained research assistants administered four subtests. T-scores based on Dutch norm for all subtests were summed and converted to an estimated Full-Scale Intelligence Quotient (FSIQ) score.
Covariates
Covariates for epigenetic associations in both cohorts included: child sex, maternal age at delivery (in years), smoking during pregnancy (binary categorization of ‘no smoking/quit in early pregnancy’ vs. ‘smoked throughout pregnancy’), gestational age at delivery (in weeks), child age at the fine motor assessment (in years), and estimated cell-type proportions estimated using a cord blood cell type reference panel [Citation49]. In addition, we adjusted for technical covariates (i.e., batch effect only in GenR, as ComBat was applied to remove batch effect in INMA), and handedness as measured by the Edinburgh Handedness Inventory (EHI) in GenR. In INMA, handedness was defined as a laterality index calculated with the relative difference between scores obtained with preferred and non-preferred hand, expressed as a percent of the sum.
Further details on DNAm, finger tapping test, cognitive functions, and handedness are provided in Supplementary Methods
Statistical analysis
Epigenome-wide association study (EWAS) analysis
Robust linear regression was applied to investigate the prospective associations between neonatal DNAm and fine motor ability in childhood. DNA methylation β values at each CpG site were specified as the predictor and right-, left-hand or bimanual FTT score as the outcome adjusted for all covariates. In order to DNAm values were z-standardized to facilitate interpretation and comparison across two cohorts. Both DNAm β value and FTT outcomes were z-standardized to facilitate interpretation and comparison across two cohorts. Probes were annotated using the meffil R package [Citation50], enhanced using the University of California Santa Cruz (UCSC) Genome Browser, based on genome build hg19. P-values were adjusted for genome-wide significance using false discovery rate (FDR) adjustment using the Benjamini–Hochberg method [Citation51] and only sites with FDR-corrected q < 0.05 were considered statistically significant.
Follow-up analyses
Follow-up analyses were first performed to further characterize identified top CpGs (FDR q < 0.05) as follows. (1) We examined the concordance between methylation in peripheral blood and brain using two independent online tools based on post-mortem data: the blood–brain concordance tool (http://epigenetics.essex.ac.uk/bloodbrain/) from 75 adults characterizing whole blood versus 4 brain regions (superior temporal gyrus, prefrontal cortex, entorhinal cortex, and cerebellum); and BECon (https://redgar598.shinyapps.io/BECon/) from 16 adults with 3 brain regions (inferior temporal gyrus, anterior prefrontal cortex, and parietal cortex). (2) Gene expression profiles were probed across 53 human tissues using GTEx database from the FUMA portal (https://fuma.ctglab.nl/). (3) Look-up of methylation quantitative trait loci (mQTL) was performed using the largest mQTL database to date to explore potential genetic influences (https://mqtldb.godmc.org.uk/).
Additionally, to verify the relevance of FTT to cognitive functions, we examined the associations of the FTT score with FSIQ and 4 subdomain index scores of cognition using linear regression. The analyses were adjusted for sex, age of cognitive assessment, handedness, child ethnicity, maternal age, and education. Last, a candidate gene follow-up analysis was performed on results extracted from a previous EWAS of infants’ motor ability for CpG sites annotated to SPTBN4, in order to examine associations with DNAm at this previously implicated gene (n = 36 CpGs). P-values were adjusted for gene-level significance using the Benjamini-Hochberg method for false discovery rate (FDR), and only methylation sites with FDR-corrected q < 0.05 were considered statistically significant.
Replication analyses in INMA
Last, we attempted to replicate our findings in an independent sample (n = 326) with complete data on cord blood DNAm at birth and FTT at age 7 from INMA. Robust linear models were run in INMA on the top CpGs identified in GenR, adjusting for covariates as described above.
Mediation analysis
In a post hoc analysis, we examined whether fine motor development mediates the association between DNAm at birth (specifically in CpG sites showing significant replication in INMA) and cognition in adolescence. To this end, we ran a mediation model using R (package mediation) with 99% bias-corrected bootstraps confidence intervals (CIs) using 1000 bootstrapped samples. We partitioned the total effect of replicated CpGs on cognition into a direct and an indirect effect through fine motor development. The indirect effect is the estimated effect of DNAm on cognition through fine motor development, while the direct effect represents the effect of DNAm on cognition scores that is independent of fine motor development.
Data availability
The summary statistics of the EWAS will be made available on figshare https://figshare.com/.
Results
Participants characteristics
Sample characteristics are described in . Differences in characteristics between children included in our EWAS sample and those who were not included were evaluated using chi-square tests for the categorical and independent t-tests for the continuous variables. Children in the current EWAS sample had a higher gestational age and lower performance on left-hand and bimanual FTT than those not included. Mothers of children in the study were on average older, had higher education, and were less likely to smoke throughout pregnancy compared to mothers of children who were excluded. Children from INMA showed, on average, higher left- and right-hand FTT performance compared to children in GenR, likely due to differences in the duration of FTT assessment between cohorts (15-second per trial in INMA and 10-second per trial in GenR).
Table 1. Descriptive statistics in two cohorts.
Epigenome-wide Association Analysis
At birth, lower methylation at one CpG (cg07783800) site was prospectively associated with slower childhood right and left hand FTT after FDR correction (q < 0.05; and ). The top site is located in the gene body of GNG4, which is a member of the G-protein family and has been associated with cognitive decline [Citation52]. For left-hand FTT, four CpGs surpassed the FDR-correction, including (a) the same site cg07783800 in GNG4; (b) cg05136476, annotated to the gene body of PRKCD, a gene previously linked to several psychiatric disorders by genome-wide association studies (GWAS), including ASD, ADHD, and schizophrenia [Citation53,Citation54]; (c) cg07638797, annotated to the promotor of DYRK1A, a gene localized in the Down syndrome critical region of chromosome 21; variations in this gene have also been linked to neuroticism and Parkinson’s disease by GWAS at a genome-wide significant level [Citation55,Citation56]; (d) cg16705073, annotated to ST3GAL2, a sialyltransferase gene responsible for sialylation of gangliosides and glycoproteins; knockout of this gene results in cognitive deficits in mice [Citation57]. We did not observe any significant association for bimanual FTT scores (Table S1).
Figure 1. Manhattan plots (Fig.1a. Left-hand; Fig.1b. Right-hand). showing EWAS between cord blood DNA methylation and finger tapping test at 10 years (N = 925). The horizontal line indicates a FDR threshold of q < 0.05. Top CpG sites are highlighted in green.
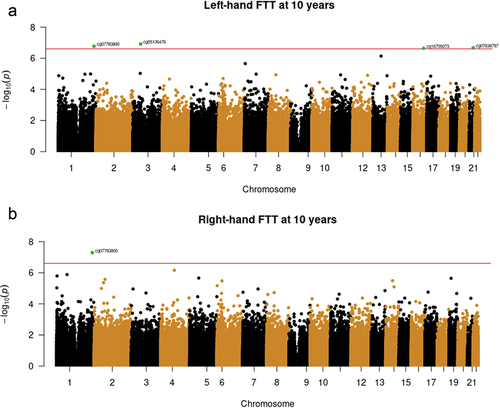
Table 2. Top 10 CpGs derived from the EWAS of the association between neonatal blood DNAm and right and left hand finger tapping test at 10 years in GenR; sorted by ascending p-value (N = 925).
Follow-up analyses
Blood–brain concordance
Using two independent tools, we found modest and inconsistent DNAm patterns across blood versus different brain regions for the four identified CpG sites (Table S2). For example, cg07783800 showed a positive correlation between blood and entorhinal cortex (r = 0.30) from the blood–brain DNAm comparison tool, but negative correlations across blood versus parietal cortex and inferior temporal gyrus (r = -0.39) from BECon. Despite partial overlap between frontal cortex and anterior prefrontal cortex across tools, cg05136476 showed a positive correlation between blood and frontal cortex (r = 0.11), but a negative correlation between blood and anterior prefrontal cortex (r = −0.23). These findings suggest that DNAm patterns on FTT-associated CpGs vary across tissues (blood vs. brain tissue) and across specific brain regions.
Gene expression profile across tissues
Next, we assessed gene expression levels across 53 tissues including blood and several brain regions from public GTEx data (Figure S2). The GNG4 gene showed higher expression in the ovary, pituitary gland, and brain, but with low specificity in brain regions. PRKCD and DYRK1A were highly expressed across different tissues, with lower but consistent expression in brain-related tissues.
Methylation quantitative trait loci (mQTL)
Of the four significant CpGs identified at birth, three (cg05136476 in PRKCD, cg16705073 in ST3GAL2, cg07638797 in the promoter of DYRK1A) were all associated with several known mQTLs (Table S3). Only cg07783800 (GNG4) was unrelated to known mQTLs. This was further supported by a heritability tool based on data from monozygotic and dizygotic twins [Citation58], showing low additive genetic and low shared environmental influences on DNAm levels at this site (r = 0.04 and 0.10, respectively), but high nonshared environmental influences (r = 0.86).
Associations between FTT and cognitive functions
We found that slower FTT performance for both right- and left-hand at age 10 years were associated with the lower total score of cognitive function in adolescence (right-hand, β = .20, p < .001); left-hand, β = .20, p < .001). Slower performance in right-hand FTT was associated with less-optimal functioning in all cognitive subdomains except for verbal comprehension, while lower performance in left-hand FFT was associated with poorer processing speed only (Table S4).
Mediation analysis
We examined the potential mediation (indirect effect) and found that the association between lower methylation at the cg07783800 site and lower cognitive functioning was mediated by less optimal fine motor development; the indirect effect was significant for the right (β = 0.027, 95% CI: 0.01, 0.05, p = 0.009) and for the left hand (β = 0.019, 95% CI: 0.01, 0.03, p = 0.007). We did not observe a significant direct effect of cg07783800 on cognition.
Candidate gene follow-up analysis
In candidate gene follow-up analysis, none of the CpGs annotated to SPTBN4 reached gene-level significance after FDR correction (Table S5).
Replication analyses in INMA
We sought to replicate the four top CpG sites identified in GenR in an independent birth cohort. Lower methylation at the Cg07783800 site (see ) was associated with a lower FTT score in both the left- and right-hand in GenR (βleft = 0.16, SEleft = 0.03, pleft = 1.70E–07; βright = 0.17, SEright = 0.03, pright = 5.11E–08) and was significantly associated with right-hand FTT in the same direction in INMA (β = 0.12, SE = 0.05, p = 0.023). This means that a 1 SD decrease in methylation at cg07783800 was associated with a 0.17 SD lower right-hand FTT score in the discovery cohort and a 0.12 SD lower FTT score in the replication cohort. No associations were replicated with left-hand FTT in INMA (Table S6).
Figure 2. Regional Association Plot for the Top DNA Methylation (CpG) Site cg07783800.
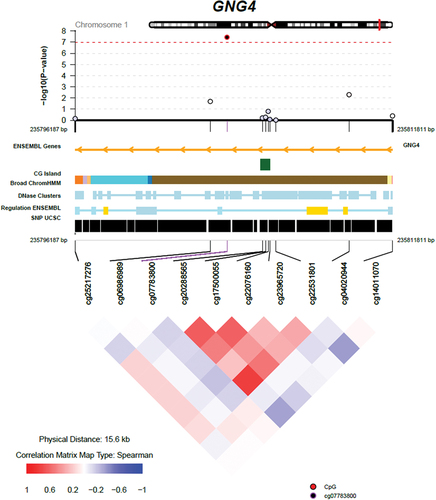
Discussion
This is the first study to characterize epigenome-wide associations between DNA methylation at birth and fine motor ability in childhood. We highlight three key findings. First, we identified 4 CpG sites in cord blood that were prospectively associated with a child’s fine motor ability, as measured by the Finger Tapping Test (FTT), after genome-wide correction in a large population-based birth cohort. These FTT-associated CpGs map to genes that have been implicated in cognitive functions, neurodevelopmental and neurodegenerative diseases. Second, one CpG annotated to the GNG4 gene, cg07783800, was replicated in an independent birth cohort, suggesting it might be a valid early epigenetic marker of fine motor ability. Our findings highlight the importance of employing multi-cohort approaches to replicate epigenetic associations and reduce the risk of false positive discoveries [Citation59]. Third, we found an association between slower FTT and worse cognitive function measured at 14 years of age, supporting the functional relevance of this neuropsychological measure of fine motor control to higher-order cognitive function in the general paediatric population. Furthermore, slower FTT performance in childhood mediated the association between lower DNAm at birth of our replicated CpG site and delayed cognition in adolescence.
Our EWAS at birth identified four CpGs after genome-wide correction. For the top site, cg07783800, we observed that the lower DNAm in this site was associated with less optimal fine motor performance on both hands, which was supported by the replication for right hand results in an independent cohort. This CpG is annotated to the body of the GNG4 gene, a modulator and transducer of several transmembrane signalling systems that play a role in haemostasis and glucagon response. It has been suggested that its expression variability in utero influences cognitive trajectories in ageing [Citation52] GNG4 is mostly expressed in the cerebellum, hypothalamus, hypophysis (pituitary gland), amygdala, basal ganglia, and cortex. In addition, GNG4 is expressed in the ovaries and may play a role in the hypothalamus-pituitary-ovarian axis. GNG4 expression changes over time, with the highest expression during foetal development, decreasing with ageing [Citation52]. Interestingly, cg07783800 was unrelated to known mQTLs, and as such unlikely to be under strong genetic control – a finding further supported by twin data: variation in this site was mainly explained by non-shared environmental influences. Future studies are needed to elucidate whether DNAm at this site reflects and potentially propagates the influence of prenatal environmental exposures on fine motor development.
The other three top CpGs identified in GenR did not replicate in INMA. We thus only briefly mention them here. The CpG cg05136476 is located in the PRKCD gene, which regulates the processing of the amyloid precursor protein (APP), a central element in Alzheimer’s disease pathophysiology; however, PRKCD has also been implicated in ASD [Citation60]. Cg07638797 is annotated to the promotor of DYRK1A, a gene localized in the Down syndrome critical region of chromosome 21. This gene plays a critical function in the central nervous system during development and ageing. It controls the differentiation of prenatally formed neurons and has effects on synaptic plasticity. Cg16705073 is annotated to ST3GAL2 gene products sialyltransferase, which are largely responsible for ganglioside terminal α2–3 sialylation in the brain, synthesizing the major brain gangliosides GD1a and GT1b [Citation61]. Taken altogether, all genes to which the four CpG sites were mapped are expressed in the brain and have been linked to neurodevelopmental and neurodegenerative diseases, including autism and Down syndrome. Impaired neuromotor development is implicated in all of these neurodevelopmental disorders.
We observed a relationship between fine motor skills in childhood and cognitive performance in adolescence. This converges with previous epidemiological studies as well as other lines of evidence, for example neuroimaging studies, which have shown that maturation of the dorsolateral prefrontal cortex and cerebellum may underlie both motor experience and active exploration of the world shaping these cognitive functions [Citation62]. In samples of typically developing children, neuromimaging studies have also shown that regions of the brain that underpin motor functioning are among the first to mature [Citation63]. While difficult to tease apart the directionality of associations, several researchers have posited that fine motor abilities may precede the development of cognitive abilities, starting with Piaget’s theory of developmental stages and recently supported by a longitudinal cross-lagged study [Citation7,Citation8]. It is possible that epigenetic mechanisms partly explain the association between less optimal fine development and cognitive functioning. In line with this hypothesis, we found that DNAm levels at birth of our replicated CpG (cg07783800) prospectively associated with cognition in adolescence through fine motor development. Specifically, slower FTT mediated the association between lower DNAm at birth and poorer cognitive functioning during adolescence.
Overall, these findings point to GNG4 methylation as a promising candidate for future studies investigating the development of fine motor abilities. It will be important in future to test whether GNG4 methylation may be causally related to motor and cognitive function, through for example the use of advanced causal inference approaches in humans (e.g., Mendelian randomization) as well as experimental models (e.g., epigenetic editing). Furthermore, it will be useful to examine whether information on GNG4 methylation may add predictive value on top of known risk factors for poor motor development. Of note, the identified effect sizes were small, and it is unlikely that information on single CpG sites will sufficiently improve predictive performance, in the same way that single genetic variants are insufficient to predict complex traits or disorders. However, the use of polyepigenetic scores (also referred to as methylation risk scores – akin to polygenic risk scores) may help in future to capturing broader DNAm patterns associated with motor ability and help improve predictive power.
Strengths and limitations
This study presents a number of strengths, including its prospective design, objective performance-based measures of child fine motor ability, and the replication in an independent cohort. However, several limitations should be noted. Since subjects included in the discovery phase were not representative of the whole cohort concerning maternal education and ethnicity, the generalizability of findings from the current study may be limited. However, we replicated the top CpG in an independent cohort (INMA), where the education of mothers is quite different than in GenR. While not impossible, reverse causality at this age is unlikely to explain our results, as hand fine motor ability only manifests at later stages of child development. We could not perform causal inference analysis as genome-wide association studies of motor development are unavailable. In addition, epigenetic mechanisms are tissue-specific, and the functional relevance of blood-based DNAm for brain-based phenotypes, such as motor ability, is unclear. As we do not have individual gene expression data in Generation R, we utilized publicly available tools to explore the expression of the annotated genes in other relevant tissues (e.g., the brain). The sample sizes of these tissue databases are often small, limited to adults, and available post-mortem tissue; therefore, caution is warranted when interpreting these results.
Regarding the blood–brain correlations, it is important to note that although currently available blood–brain tools are helpful in exploring cross-tissue concordance, they have several caveats that limit their interpretation, including the use of small samples with mixed clinical presentation and incomplete phenotyping, limited data on relevant brain regions, and the reliance on mainly adult post-mortem samples, which may not generalize to neurodevelopmental processes such as fine motor ability and cognition in childhood. We also note that peripheral DNAm patterns may still be useful and valid markers even in the absence of significant cross-tissue concordance. To illustrate, similarly to our study, DNAm at the cg05575921 site in blood (annotated to the AHRR) – a strong, widely replicated biomarker of smoking exposure which is associated with poor cognitive function and structural brain integrity in adults [Citation64] – shows null-to-weak positive associations with DNAm in the brain using the blood–brain comparison tool, whereas it shows negative correlations with the brain regions tagged by the BECon tool. Finally, this study focused on DNA methylation, which is one of several types of epigenetic mechanisms (e.g., histone or RNA modifications), which may contribute to motor development and the pathogenesis of motor diseases [Citation65].
Conclusions
To our knowledge, we report the first EWAS of fine motor ability. At four CpGs, lower DNAm at birth was prospectively associated with poorer fine motor ability in childhood, as indexed by slower performance on the finger tapping test. All genes corresponding to the identified CpGs have been involved in brain pathways regulating neurodevelopment and neurodegeneration. One site annotated to the GNG4 gene, cg07783800, showed consistent results for both hands in the Generation R study and was replicated for the right hand in the INMA study, showing potential as an epigenetic marker of fine motor development. Furthermore, lower DNAm at cg07783800 was associated with poor cognitive functioning through non-optimal fine motor development. Our findings highlight the importance of employing multi-cohort approaches to replicate epigenetic associations and reduce the risk of false positive discoveries. The results from this study contribute to a better understanding of epigenetic factors associated with fine motor development in childhood and later cognitive function.
Supplemental Material
Download Zip (204.6 KB)Acknowledgments
The Generation R Study is conducted by the Erasmus Medical Center in close collaboration with the School of Law and Faculty of Social Sciences at Erasmus University Rotterdam, the Municipal Health Service Rotterdam area, the Rotterdam Homecare Foundation, and the Stichting Trombosedienst & Artsenlaboratorium Rijnmond (STAR-MDC), Rotterdam. We gratefully acknowledge the contribution of children and parents, general practitioners, hospitals, midwives, and pharmacies in Rotterdam. The generation and management of the Illumina 450K methylation array data (EWAS data) for the Generation R Study was executed by the Human Genotyping Facility of the Genetic Laboratory of the Department of Internal Medicine, Erasmus MC, the Netherlands. We thank Mr. Michael Verbiest, Ms. Mila Jhamai, Ms. Sarah Higgins, Mr. Marijn Verkerk and Dr. Lisette Stolk for their help in creating the EWAS database. We thank Dr. A.Teumer for his work on the quality control and normalization scripts. The general design of the Generation R Study is made possible by financial support from the Erasmus Medical Center Rotterdam, Erasmus University Rotterdam, the Netherlands Organization for Health Research and Development and the Ministry of Health, Welfare and Sport.
Disclosure statement
No potential conflict of interest was reported by the authors.
Supplementary material
Supplemental data for this article can be accessed online at https://doi.org/10.1080/15592294.2023.2207253
Additional information
Funding
References
- Seo SM. The effect of fine motor skills on handwriting legibility in preschool age children. J Phys Ther Sci. 2018;30(2):324–13.
- Emck C, Bosscher R, Beek P, et al. Gross motor performance and self-perceived motor competence in children with emotional, behavioural, and pervasive developmental disorders: a review. Dev Med Child Neurol. 2009;51(7):501–517.
- Martin R, Tigera C, Denckla MB, et al. Factor structure of paediatric timed motor examination and its relationship with IQ. Dev Med Child Neurol. 2010;52(8):e188–194.
- Alamiri B, Nelson C, Fitzmaurice GM, et al. Neurological soft signs and cognitive performance in early childhood. Dev Psychol. 2018;54(11):2043–2052.
- Piek JP, Dawson L, Smith LM, et al. The role of early fine and gross motor development on later motor and cognitive ability. Hum Mov Sci. 2008;27(5):668–681.
- Bloch MH, Sukhodolsky DG, Dombrowski PA, et al. Poor fine-motor and visuospatial skills predict persistence of pediatric-onset obsessive-compulsive disorder into adulthood. J Child Psychol Psychiatr. 2011;52(9):974–983. DOI:10.1111/j.1469-7610.2010.02366.x
- Piaget J. The development of time concepts in the child. Proc Annu Meet Am Psychopathol Assoc. 1954-1955;34–44. discussion, 45-55.
- Mohring W, Frick A. Touching up mental rotation: effects of manual experience on 6-month-old infants’ mental object rotation. Child Dev. 2013;84(5):1554–1565.
- Hubel KA, Yund EW, Herron TJ, et al. Computerized measures of finger tapping: reliability, malingering and traumatic brain injury. J Clin Exp Neuropsychol. 2013;35(7):745–758.
- Rabin LA, Barr WB, Burton LA. Assessment practices of clinical neuropsychologists in the United States and Canada: a survey of INS, NAN, and APA Division 40 members. Arch Clin Neuropsychol. 2005;20(1):33–65.
- Damme KSF, Schiffman J, Ellman LM, et al. Sensorimotor and activity psychosis-risk (SMAP-R) scale: an exploration of scale structure with replication and validation. Schizophr Bull. 2021;47(2):332–343.
- Behere A, Shahani L, Noggle CA, et al. Motor functioning in autistic spectrum disorders: a preliminary analysis. J Neuropsychiatry Clin Neurosci. 2012;24(1):87–94.
- Mostofsky SH, Rimrodt SL, Schafer JGB, et al. Atypical motor and sensory cortex activation in attention-deficit/hyperactivity disorder: a functional magnetic resonance imaging study of simple sequential finger tapping. Biological Psychiatry. 2006;59(1):48–56. DOI:10.1016/j.biopsych.2005.06.011
- Valera EM, Spencer RMC, Zeffiro TA, et al. Neural substrates of impaired sensorimotor timing in adult attention-deficit/hyperactivity disorder. Biological Psychiatry. 2010;68(4):359–367. DOI:10.1016/j.biopsych.2010.05.012
- Travers BG, Bigler ED, Duffield TC, et al. Longitudinal development of manual motor ability in autism spectrum disorder from childhood to mid-adulthood relates to adaptive daily living skills. Dev Sci. 2017;20(4):e12401. DOI:10.1111/desc.12401
- Liou WC, Chan L, Hong CT, et al. Hand fine motor skill disability correlates with dementia severity. Arch Gerontol Geriatr. 2020;90:104168.
- Plomin R, Haworth CMA, Davis OSP. Common disorders are quantitative traits. Nat Rev Genet. 2009;10(12):872–878.
- Lundström S, Chang Z, Råstam M, et al. Autism spectrum disorders and autisticlike traits: similar etiology in the extreme end and the normal variation. Arch Gen Psychiat. 2012;69(1):46–52. DOI:10.1001/archgenpsychiatry.2011.144
- Larsson H, Anckarsater H, Råstam M, et al. Childhood attention‐deficit hyperactivity disorder as an extreme of a continuous trait: a quantitative genetic study of 8,500 twin pairs. J Child Psychol Psyc. 2012;53(1):73–80.
- Bernard JA, Mittal VA. Updating the research domain criteria: the utility of a motor dimension. Psychol Med. 2015;45(13):2685–2689.
- Mittal VA, Wakschlag LS. Research domain criteria (RDoC) grows up: strengthening neurodevelopment investigation within the RDoC framework. J Affect Disord. 2017;216:30–35.
- Francks C, Fisher SE, Marlow AJ, et al. Familial and genetic effects on motor coordination, laterality, and reading-related cognition. Am J Psychiatry. 2003;160(11):1970–1977. DOI:10.1176/appi.ajp.160.11.1970
- Williams LRT, Gross JB. Heritability of motor skill. Acta geneticae medicae et gemellologiae: twin research. 1980;29(2):127–136.
- Mountford HS, Hill A, Barnett AL, et al. Genome-wide association study of motor coordination. Front Hum Neurosci. 2021;15:669902.
- Doney R, Lucas BR, Jones T, et al. Fine motor skills in children with prenatal alcohol exposure or fetal alcohol spectrum disorder. J Dev Behav Pediatr. 2014;35(9):598–609.
- Darling AL, Rayman MP, Steer CD, et al. Association between maternal vitamin D status in pregnancy and neurodevelopmental outcomes in childhood: results from the avon longitudinal study of parents and children (ALSPAC). Br J Nutr. 2017;117(12):1682–1692.
- Crous-Bou M, Gascon M, Gispert JD, et al. Impact of urban environmental exposures on cognitive performance and brain structure of healthy individuals at risk for Alzheimer’s dementia. Environ Int. 2020;138:105546.
- Trasti N, Vik T, Jacobsen G, et al. Smoking in pregnancy and children’s mental and motor development at age 1 and 5 years. Early Hum Dev. 1999;55(2):137–147.
- Golding J, Emmett P, Iles-Caven Y, et al. A review of environmental contributions to childhood motor skills. J Child Neurol. 2014;29(11):1531–1547.
- Sammallahti S, Tiemeier H, Louwen S, et al. Fetal–placental blood flow and neurodevelopment in childhood: population‐based neuroimaging study. Ultrasound Obstet Gynecol. 2021;58(2):245–253. DOI:10.1002/uog.22185
- Barker ED, Walton E, Cecil CAM. AnnuaL research review: DNA methylation as a mediator in the association between risk exposure and child and adolescent psychopathology. J Child Psychol Psychiatry. 2018;59(4):303–322.
- Martin EM, Fry RC. Environmental Influences on the Epigenome: Exposure- Associated DNA methylation in human populations. Annu Rev Public Health. 2018;39(1):309–333.
- Neumann A, Walton E, Alemany S, et al. Association between DNA methylation and ADHD symptoms from birth to school age: a prospective meta-analysis. Transl Psychiatry. 2020;10(1):398. DOI:10.1038/s41398-020-01058-z
- Rijlaarsdam J, Cecil CAM, Relton CL, et al. Epigenetic profiling of social communication trajectories and co-occurring mental health problems: a prospective, methylome-wide association study. Dev Psychopathol. 2022;34(3):1–10.
- Bromer C, Marsit CJ, Armstrong DA, et al. Genetic and epigenetic variation of the glucocorticoid receptor (NR3C1) in placenta and infant neurobehavior. Dev Psychobiol. 2013;55(7):673–683.
- Marsit CJ, Maccani MA, Padbury JF, et al. Placental 11-beta hydroxysteroid dehydrogenase methylation is associated with newborn growth and a measure of neurobehavioral outcome. PloS one. 2012;7(3):e33794. doi:10.1371/journal.pone.0033794.
- Rygiel CA, Dolinoy DC, Bakulski KM, et al. DNA methylation at birth potentially mediates the association between prenatal lead (Pb) exposure and infant neurodevelopmental outcomes. Environ Epigenet. 2021;7(1):dvab005. DOI:10.1093/eep/dvab005
- Hüls A, Wedderburn CJ, Groenewold NA, et al. Newborn differential DNA methylation and subcortical brain volumes as early signs of severe neurodevelopmental delay in a South African birth cohort study. World J Biol Psychiatry. 2022;2022(8):1–12. DOI:10.1080/15622975.2021.2016955
- Roebers CM, Röthlisberger M, Neuenschwander R, et al. The relation between cognitive and motor performance and their relevance for children’s transition to school: a latent variable approach. Hum Mov Sci. 2014;33:284–297.
- Suggate S, Stoeger H. Fine motor skills enhance lexical processing of embodied vocabulary: a test of the nimble-hands, nimble-minds hypothesis. Q J Exp Psychol. 2017;70(10):2169–2187.
- Kooijman MN, Kruithof CJ, van Duijn CM, et al. The Generation R Study: design and cohort update 2017. Eur J Epidemiol. 2016;31(12):1243–1264. DOI:10.1007/s10654-016-0224-9
- Guxens M, Ballester F, Espada M, et al. Cohort Profile: the INMA–INfancia y Medio Ambiente–(Environment and Childhood) Project. Int J Epidemiol. 2012;41(4):930–940. DOI:10.1093/ije/dyr054
- Lehne B, Drong AW, Loh M, et al. A coherent approach for analysis of the Illumina HumanMethylation450 BeadChip improves data quality and performance in epigenome-wide association studies. Genome Bio. 2015;16(1):1–12. DOI:10.1186/s13059-015-0600-x
- Aryee MJ, Jaffe AE, Corrada-Bravo H, et al. Minfi: a flexible and comprehensive bioconductor package for the analysis of infinium DNA methylation microarrays. Bioinformatics. 2014;30(10):1363–1369. DOI:10.1093/bioinformatics/btu049
- White T, Muetzel RL, El Marroun H, et al. Paediatric population neuroimaging and the Generation R Study: the second wave. Eur J Epidemiol. 2018;33(1):99–125. DOI:10.1007/s10654-017-0319-y
- Lopez-Vicente M, Lamballais S, Louwen S, et al. White matter microstructure correlates of age, sex, handedness and motor ability in a population-based sample of 3031 school-age children. Neuroimage. 2021;227:117643.
- Lezak MD. Neuropsychological assessment, 3rd ed. New York, NY, US: Oxford University Press; 1995.
- Kaufman AS, Raiford SE, Coalson DL. Intelligent testing with the WISC-V. New Jersey: John Wiley & Sons; 2015.
- Gervin K, Salas LA, Bakulski KM, et al. Systematic evaluation and validation of reference and library selection methods for deconvolution of cord blood DNA methylation data. Clin Epigenetics. 2019;11(1).
- Min JL, Hemani G, Davey Smith G, et al. Meffil: efficient normalization and analysis of very large DNA methylation datasets. Bioinformatics. 2018;34(23):3983–3989.
- Benjamini Y, Hochberg Y. Controlling the false discovery rate: a practical and powerful approach to multiple testing. J Royal Stat Soc Ser B (Methodological). 1995;57(1):289–300.
- Bonham LW, Evans DS, Liu Y, et al. Neurotransmitter pathway genes in cognitive decline during aging: evidence for GNG4 and KCNQ2 Genes. Am J Alzheimers Dis Other Demen. 2018;33(3):153–165.
- Anney RJL, Ripke S, Anttila V, et al. Meta-analysis of GWAS of over 16,000 individuals with autism spectrum disorder highlights a novel locus at 10q24.32 and a significant overlap with schizophrenia. Mol Autism. 2017;8(1):21.
- Peyrot WJ, Price AL. Identifying loci with different allele frequencies among cases of eight psychiatric disorders using CC-GWAS. Nature Genet. 2021;53(4):445–454.
- Nalls MA, Blauwendraat C, Vallerga CL, et al. Identification of novel risk loci, causal insights, and heritable risk for Parkinson’s disease: a meta-analysis of genome-wide association studies. Lancet Neurol. 2019;18(12):1091–1102. DOI:10.1016/S1474-4422(19)30320-5
- Wendt FR, Pathak GA, Lencz T, et al. Multivariate genome-wide analysis of education, socioeconomic status and brain phenome. Nat Human Behav. 2021;5(4):482–496.
- Yoo SW, Motari MG, Susuki K, et al. Sialylation regulates brain structure and function. Faseb J. 2015;29(7):3040–3053. DOI:10.1096/fj.15-270983
- Hannon E, Knox O, Sugden K, et al. Characterizing genetic and environmental influences on variable DNA methylation using monozygotic and dizygotic twins. PLoS Genet. 2018;14(8):e1007544. DOI:10.1371/journal.pgen.1007544
- Rijlaarsdam J, Pappa I, Walton E, et al. An epigenome-wide association meta-analysis of prenatal maternal stress in neonates: a model approach for replication. Epigenetics. 2016;11(2):140–149. DOI:10.1080/15592294.2016.1145329
- Park YH, Hodges A, Risacher SL, et al. Dysregulated Fc gamma receptor-mediated phagocytosis pathway in Alzheimer’s disease: network-based gene expression analysis. Neurobiol Aging. 2020;88:24–32.
- Sturgill ER, Aoki K, Lopez PH, et al. Biosynthesis of the major brain gangliosides GD1a and GT1b. Glycobiology. 2012;22(10):1289–1301. DOI:10.1093/glycob/cws103
- Diamond A. Close interrelation of motor development and cognitive development and of the cerebellum and prefrontal cortex. Child Dev. 2000;71(1):44–56.
- Casey BJ, Tottenham N, Liston C, et al. Imaging the developing brain: what have we learned about cognitive development? Trends Cogn Sci. 2005;9(3):104–110.
- Corley J, Cox SR, Harris SE, et al. Epigenetic signatures of smoking associate with cognitive function, brain structure, and mental and physical health outcomes in the Lothian Birth Cohort 1936. Transl Psychiatry. 2019;9(1):248. DOI:10.1038/s41398-019-0576-5
- Yang Y, Yamada T, Hill KK, et al. Chromatin remodeling inactivates activity genes and regulates neural coding. Science. 2016;353(6296):300–305. DOI:10.1126/science.aad4225