ABSTRACT
Explicable AI in education (XAIED) has been proposed as a way to improve trust and ethical practice in algorithmic education. Based on a critical review of the literature, this paper argues that XAI should be understood as part of a wider socio-technical turn in AI. The socio-technical perspective indicates that explicability is a relative term. Consequently, XAIED mediation strategies developed and implemented across education stakeholder communities using language that is not just ‘explicable’ from an expert or technical standpoint, but explainable and interpretable to a range of stakeholders including learners. The discussion considers the impact of XAIED on several educational stakeholder types in light of the transparency of algorithms and the approach taken to explaination. Problematising the propositions of XAIED shows that XAI is not a full solution to the issues raised by AI, but a beginning and necessary precondition for meaningful discourse about possible futures.
Introduction
AI was predicted to disrupt human society and productivity as an aspect of the ‘4th Industrial Revolution’ (Schwab Citation2016; Timms Citation2016) and the effects of this are already being observed in education. The pace of AI uptake is increasing, and 2023 sees an explosion of interest in language-based tools like ChatGPT (OpenAI Citation2023) while AI tools for large scale learning are also being developed (Kiecza Citation2022). According to the AI in Education Market Research Report (Market Research Future Citation2020), the global market reached $1.1 billion in 2019 and is predicted to generate $25.7 billion in 2030. Statista (Citation2020) estimates the AI market as a whole will be worth $126 billion by 2025. Contemporary applications of AIED include adaptive learning systems, tailored assessments, automated feedback and tutoring tools, and learning analytics dashboards (Khosravi et al. Citation2022).
The Covid-19 crisis catalysed uptake of learning management systems, incentivizing higher education institutions to move towards online learning and automation, though AI tools evidently did not prove to be especially useful during the pandemic (Heaven Citation2021). One high profile use of algorithms in education during this time in the UK saw automated grading of the General Certificate of Education (GCE) Advanced (A) Level exams when in-person exams could not take place (Ehsan et al. Citation2021). Huge outcry among educators, learners and institutions over the perceived unfairness of grades allocated resulted in a government u-turn and the resignation of the chief executive of the UK exams regulator Ofqual. The UK Prime Minister blamed a ‘mutant algorithm’ for the debacle (Everett Citation2021). AI proliferation is thus often presented as progress despite falling short of targeted or imputed standards (Baur Citation2020; Chatfield Citation2020).
There is a growing awareness of the profound ethical implications of AIED. AI is seen as a potential route to boosting job markets, lifelong learning and democratic participation but is also open to heinous misuse (European Parliament Citation2022; European Commission Citation2018). Algorithmic bias has been the focus of many critiques of AI (e.g., Baker and Hawn Citation2021; Birhane et al. Citation2022; Noble Citation2018; Samuel Citation2021; Wachter Citationforthcoming; Zuboff Citation2019). Bulathwela et al. (Citation2021, 6) found in their review that ‘AI will impact education greatly. However, virtually no research has been undertaken, no guidelines have been agreed, no policies have been developed, and no regulations have been enacted to address the use of AI in education’.
There is consequently much debate on how to manage the risks that are potentially introduced to democracy and accountability in teaching and learning systems. The emerging consensus is that there needs to be adequate transparency and explicability for the use of algorithms (Floridi, Cowls, and Beltrametti Citation2018; Gunning et al. Citation2019; Kiourti et al. Citation2019; Panigutti, Perotti, and Pedreschi Citation2020). Explicability is intended to make it easier to reconstruct actions taken by AI programs and to show who might be responsible for consequences. The three distinctive features of XAI are ‘algorithmic transparency; explainer generalizability; and explanation granularity’ (Antoniadi et al. Citation2021). However, there are few detailed descriptions of what this will look like or aspire to be in educational contexts (e.g., Khosravi et al. Citation2022). The goal of this paper is to understand the nature of XAIED; determine what might make it effective, and identify any ethical or practical limits to such transparency in teaching and learning processes.
Materials and methods
The claims of this paper are based on a thematic literature search at the intersection of several disciplines relating to XAIED. A purposive, emergent snowballing approach (Wohlin Citation2014; Lecy and Beatty Citation2012) was used to compile resources, supplemented by keyword searches on Google Scholar (Citationn.d.) and question queries submitted to the Elicit (Citationn.d.) database. 58 items published in 2020 or later were selected for review. Additional relevant references were drawn from these and added to the dataset. The total number of resources consulted was 102. The method of presentation below is summative, thematic, synthetic, reflective and analytical. No statistical claim is about the choice of literature, which was guided by inquiry. The review took place between October 2021 and October 2022.
Results
Artificial intelligence in education (AIED)
Thousands of institutions are already using AI technologies to shape and plan the delivery of education (Zawacki-Richter et al. Citation2019; Luckin et al. Citation2016; Dignum Citation2021). AIED is often presented as a pragmatic tool which simply delivers existing tasks more efficiently, and therefore has benefits for both learners and educators. The conviction that AI presents a route to improving many services associated with teaching and learning is a clear driver of activity and reflects the optimistic view that innovation in techniques like machine learning and deeper learning will lead to tangible benefits in practice. As an extension of the move towards digitalisation in higher education institutions (Orr, Weller, and Farrow Citation2018) the use of AI has become a focus for innovation and competitive edge (Khosravi et al. Citation2022). Applications of algorithmic intelligence are anticipated in areas such as profiling learners; intelligent tutoring systems; assessment; evaluation; adaptive systems and personalised learning (Luckin et al. Citation2016). Natural language processing can be used to connect learners and educators with relevant information in a more timely way. Personalisation (Fiok et al. Citation2022) can draw on data external to and generated by the learner to suggest interventions. Automated models are being built to analyse the social and emotional moods of learners; provide feedback; create authentic learning simulations and offer personal support through AI tutoring, writing assistance, and chatbots (Sharples and Pérez y Pérez Citation2022). Educators can be supported by delegating administrative tasks to machines, freeing time for more creative activity. Algorithmic data mining has been shown to produce an increase in student enrolment of more than 20% and thus a significant uplift in revenue (Aulck, Nambi, and West Citation2019). Thus, the strategic value of AI in education is only partly determined by a focus on learning and teaching.
Many of the anticipated uses of AIED rely on the assumption that mass data collection and analysis will take place. This can include data about learner progress through a virtual learning environment and which pedagogical approaches have been most effective for different learner profiles; but includes tracking biometric data, taking voice samples, and using eye-tracking software (Luckin et al. Citation2016, 34). Already there is considerable reliance on the use of controversial tracking technologies in proctoring and assessment (Coghlan, Miller, and Paterson Citation2021). Institutional planning is increasingly data-driven and based on harvesting increasing amounts of information from virtual learning environments and combining these with other data sets as an expanded neural network. Beetham et al. (Citation2022, 18) describe the key aspects of surveillance in higher education as ‘the rendering of student and educator activities as behaviours that can be “datafied”; inequalities of power that exist between data owners/companies and the people whose data is being collected, analysed, managed and shared; the insertion and intensification of data-based and data-generating digital platforms into the core activities of universities, and the normalisation of vendor-university relationships’. There is no way to separate the use of analytics and surveillance. However, the scale and penetration of machine learning data collection can be unsettling: a recent study found that 146 of 164 EdTech products recommended, mandated or procured by governments during the Covid-19 pandemic harvested the data of millions of children (Human Rights Watch Citation2022).
As AIED becomes increasingly mainstream attention is shifting from the technical to the socio-technical perspective. The majority of legacy AIED literature is based in quantitative computer science and there is little expertise in AI in the humanities (Zawacki-Richter et al. Citation2019; Dignum Citation2021) leading to calls that AI would benefit from greater interdisciplinarity (Gilpin et al. Citation2018; Dignum Citation2021). More generally, differences in contexts of application complicate attempts to assess the impact of AI as a whole. Xuesong et al. (Citation2021) suggest a threefold categorisation of the challenges facing AIED. Firstly, arising from the attempt to apply AI techniques from one context of application into another; secondly, the disruptive effects on the traditional roles and activities of learner and teacher; and thirdly the wider social impacts that can emerge when things go wrong (such as the inappropriate exposure or use of data).
The ‘Black box’ problem
Pasquale (Citation2020, 225) has described how advanced socio-technical systems can appear ‘humanly inexplicable’ or even ‘magical’. The key structural feature of the ‘black box’ model of computation is the non-transparency of the processes and workings that convert input to output. Tjoa and Guan (Citation2021) find that ‘the black box nature of [deeper learning] is still unresolved, and many machine decisions are still poorly understood’. Machine learning has made little progress with representing higher order thoughts, higher levels of abstraction, being creative with language, or ‘common sense’ (Russell and Norvig Citation2021). Dramatic progress has been made in recent years with respect to functional or “weak” applications using natural language programming, many of which are often branded in the unrestrained language of AI marketing.
Guidotti et al. (Citation2018) propose a universal typology for understanding issues around ‘black box’ computation: the model explanation problem; the outcome explanation problem; the inspection problem; and the transparent box design problem. These vary based on the specific explanation problem addressed, the type of explanator adopted, the black box model opened, and the type of data used as input by the black box model. Markus, Kors, and Rijnbeek (Citation2021) similarly propose three types of explanations: model-based explanations (where a simplified model is presented to explain the workings of the AI model), attribution-based explanations (which explain the task model in terms of input features), and example-based explanations (which involve looking at specific instances or cases to explain how a model works – or doesn’t work). Páez (Citation2019) supports the idea that interpretative models present the best route to understanding but the purely functional approach doesn’t really explain the actual XAI part at all: ‘The task ahead for XAI is thus to fulfil the double desiderata of finding the right fit between the interpretative and the black box model, and to design interpretative models and devices that are easily understood by the intended users.’ ()
Table 1. Four challenges for XAI (based on Felten Citation2017; cited in Mueller et al. Citation2019, 18).
The explicability turn
Ethics is weakly represented in contemporary discourse around AI, with ethics, critical reflection and pedagogy all requiring further exploration in the field (Zawacki-Richter et al. Citation2019; Crawford Citation2021, 115–119). Nonetheless, a range of overlapping ethical frameworks have been proposed for dealing with emergent ethical issues (e.g., Future of Life Citation2017; Montréal Declaration for a Responsible Development of Artificial Intelligence Citation2017; EU Citation2018; IEEE Citationn.d.; HoL Citation2018; Partnership on AI Citation2018). AI4People reduced 47 proposed principles to four traditional ethical principles as well as one new principle which relates to AI implementation: explicability (Floridi, Cowls, and Beltrametti Citation2018; Floridi and Cowls Citation2019) ().
Figure 1. An ethical framework for AI, formed of four traditional principles and a new one (Floridi, Cowls, and Beltrametti Citation2018).
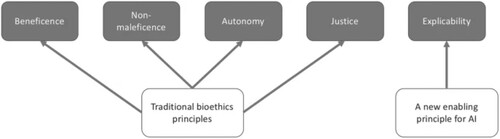
XAI addresses four traditional moral principles (beneficence; non-maleficence; autonomy; and justice) through two key questions: how does [the algorithm] work? and who is responsible for the way it works? Through greater accountability and legibility, Floridi, Cowls, and Beltrametti (Citation2018) anticipate more open ethical deliberations supported by training more engineers in ethical and legal perspectives, new qualification programmes in the ethics of AI, greater public awareness of AI, and promotion of computer science. From this perspective, XAI is a retort to the ‘black box’ problem which responds with transparency to foster trust (Hanif, Zhang, and Wood Citation2021).
Notably, not all agree that XAI is a solution. Robbins (Citation2019) argues that many uses for AI are low risk and don’t require explication; in some cases XAI could prevent the advantages of AI being realised. According to this view “a principle of explicability for AI makes the use of AI redundant” because it is not the algorithm (process) or designer/decision maker but the underlying principle that determines ethical value (ibid.). Jiang, Kahai, and Yang (Citation2022) further argue that XAI can overwhelm and introduce epistemic uncertainty. There remains considerable debate and ambiguity around terms like explicability, explainability, interpretability, comprehensibility, intelligibility, transparency, and understandability. Some (e.g., Páez Citation2019) consequently argue that explicability remains a vague and under-theorised term with no definitive meaning. Nonetheless, XAI remains the most common response to criticisms of algorithmic bias, unwanted impacts, and lack of scrutiny.
Adadi and Berrada (Citation2018) propose that ‘[e]xplainability provides insights to a targeted audience to fulfil a need, whereas interpretability is the degree to which the provided insights can make sense for the targeted audience’s domain knowledge.’ XAI cannot result in a single form of explanation since different stakeholders require different kinds of explanations which are commensurate with their own baseline understanding and ability to interpret. In the case of education this means providing XAI at the most generalisable level (Antoniadi et al. Citation2021) although this might look different for learners, educators and developers, for instance. The key consideration for XAI is the question ‘what makes for a good explanation?’ (Mueller et al. Citation2019) but good explanations are relative. A simple distinction here could differentiate the domain of technical expertise from the knowledge of the layperson. Markus, Kors, and Rijnbeek (Citation2021) suggest that the quintessential XAI distinction is between those accounts which emphasise intelligibility to a human and those which faithfully reconstruct and represent the tasks performed by an algorithm ().
Figure 2. Proposed definitions for explainability and related terms. (Markus, Kors, and Rijnbeek Citation2021).
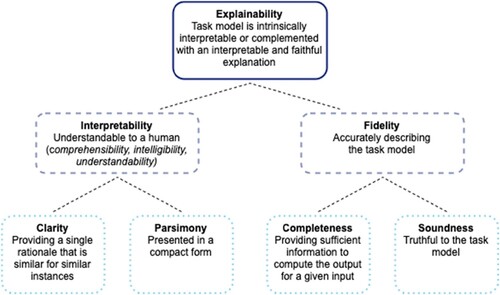
This typology distinguishes interpretability which is human readable and fidelity which is the accurate, technical description of what happens in the ‘black box’. The technical explanation of an algorithm might include things like exploratory or statistical analysis; evaluation of machine learning models; periodic iterations of concepts and validation of results; user testing; and producing documentation for datasets and models. For stakeholders lacking expert knowledge such transparency presumably has limited value without simplified explanations nor a trusted broker who can interpret on their behalf. As Khosravi et al. (Citation2022) note, this is particularly apt in the case of educational administrators, institutional leaders and legal officers who have responsibility for governance. Bloch-Wehba (Citation2020) thus argues for greater transparency in the use of automated systems of governance. Tutt (Citation2020) similarly contends that algorithms should be directly regulated by new governmental agencies which work in partnership with industry to develop common standards of acceptable practice. XAI supports the uptake and operation of machine learning in education since non-transparency negatively affects trust (Hanif, Zhang, and Wood Citation2021).
Socio-Technical perspectives on xaied
Birhane et al. (Citation2022) argue that although AI ethics is a rapidly growing field it cannot keep pace with the rapid development and rollout of AI systems into all parts of society, and as a result most work in this area is shallow. They describe AI ethics as characterised by agnosticism about existing forms of oppression and insufficiently focused on the structures and institutions that perpetuate inequality. Hickok (Citation2021) also calls for greater diversity amid a need to progress from high-level abstractions and concepts in favour of applied ethics which establish accountability. Chatfield (Citation2020) similarly points out that we can’t think about the ethics of AI distinctly from the ethics of our society.
Attempting to fully understand the socio-technical scale of AI implementations is challenging. Crawford and Joler (Citation2018) have described the interconnected nature of such systems through primary production and processing of raw materials; manufacturing; logistics; assembly; data preparation; programming; AI training; infrastructure, platformisation, user interfaces; and devices. Each stage involves various forms of human labour (much of which is ethically questionable though ‘invisible’ to the end user). To focus on the AI-user dichotomy is to overlook many socio-technical and context-dependent aspects (Vera Liao, Gruen, and Miller Citation2020).
Antoniadi et al. (Citation2021) reviewed 121 papers, finding that explainability is an important part of building trust in AI systems but that introducing XAI features can add significantly to the cost of systems. They found that there is a significant amount of work to be done in studying applications of XAI in ethically important contexts (such as medicine). Notably, the bigger the dataset – and AI requires ever bigger datasets – the less connection there is to the individual. We are increasingly affected by algorithms which one has not intentionally engaged with: shadow profiling is common on social networks and for advertisers and interlinked systems sharing data means isolating systems is difficult. Viljoen (Citation2021, 37) notes that such ‘horizontal’ data relations within our technological infrastructures are designed to facilitate and monetise data flows rather than regulate responsibilities or prevent injustices. There is also a need for human data curation to support machine learning which can lead to exploitation of the most marginalised who are most at risk when algorithmic systems fail (Hao and Hernández Citation2022; Birhane et al. Citation2022; Ricaurte Citation2022; Carman and Rosman Citation2020).
Accordingly, Ehsan et al. (Citation2021) propose the concept of social transparency for XAI. This approach adjusts the algorithmic centrality of AI decision-making towards ‘a socio-technically informed perspective that incorporates the socio-organizational context’. AI systems can be understood as human-AI assemblages which are already socio-technically embedded. Hence, a socially situated XAI needs to prioritise the complexity of human-AI assemblage over technical solutionism. Selbst et al. (Citation2018) identify five ‘traps’ for AI systems that fail to adequately recognise the socio-technical context for AI decision-making ().
Table 2. Socio-technical AI risks and ameliorations (based on Selbst et al. Citation2018).
Socio-technical approaches inherently acknowledge the range of stakeholder perspectives. For instance, Prinsloo, Slade, and Khalil (Citation2022) propose a cautious, non-binary, granular approach to human-algorithmic decision-making across areas like admissions, student support, pedagogy and assessment based on specific conditions and contexts. Hu et al. (Citation2021) propose an XAI toolkit (XAITK) which comprises an open-source collection of XAI tools and resources which can be applied across multiple domains and systems. This approach emphasises transparency and greater sharing of data across disciplines and domains of application. Similarly, the XAI-ED Framework (Khosravi et al. Citation2022) consists of critical questions about stakeholders, XAI benefits and user experience, approach to AI explanation, and pitfalls/risks. Here the recommendation is to distinguish the global and local forms of explanation that use proxies which are less complex to understand: global forms explain the entire AI model while local forms relate to individual predictions, and each is associated with particular mathematical models. The XAI-ED model confers a flexible lens on issues of XAI and suggests pragmatic routes to aligning different stakeholder groups with appropriate proxies and communication strategies (see ).
Figure 3. Common explainability approaches (Khosravi et al. Citation2022).
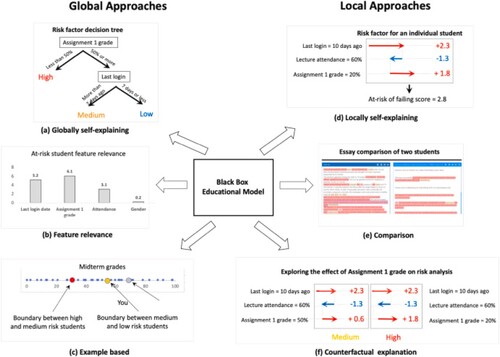
Systems of feedback and evaluation are needed for understanding the impact of AI. Morley et al. (Citation2021) argue that existing approaches to closing the gap between ethical theory and the practical design of AI systems are ineffective, meaning that regular re-evaluation of AI systems is necessary. Markus, Kors, and Rijnbeek (Citation2021) recommend that trust in XAI be built through reporting data quality (so that issues around bias and low quality data can be explored); performing extensive, external evaluation (to interrogate and optimise models); and through regulation. One potential solution is to develop regularised approaches to assessing the impact via a combination of experts and public scrutiny (Moss et al. Citation2021). Crucially, these audits could typically take place before implementation and use public transparency to ensure further accountability.
Discussion: the possibilities and limits of xaied
XAI should help educators to understand the algorithms that will influence their practice as AIED becomes more common. Similarly, learners stand to benefit from XAI when it helps them to comprehend how decisions are made that affect their learning with AIED. Other stakeholders involved in educational processes (managers, administrators, technicians, librarians, designers, etc.) are also potentially empowered. An explainable account of the same AIED system might look quite different from these alternative perspectives.
Educators and learners are likely to use different tools and services within AIED. Learners might use adaptive learning management systems, augmented interfaces, and receive support from chat bots or intelligent tutoring systems (ITS). Educators might make use of automated assessment, plagiarism checkers and administrative tools, as well as reviewing dashboards of predictive analytics. Institutions can use an overview of the data to monitor, manage and plan activity. We can consider each of these XAIED perspectives by way of Antoniadi et al. (Citation2021) as ‘algorithmic transparency; explainer generalizability; and explanation granularity’. As pedagogical experts, educators have an interest in a high-level of AIED explicability and need to have a good awareness of the role of AIED in the design of learning. Finer granularity of explanation might be needed where AIED plays a more central role, but educators should be able to explain AIED processes. By contrast, the learner might only require a simple to understand model for the role of AI in learning systems but this may limit algorithmic transparency. Providing a detailed account of how the algorithm influences the learning process might also influence how a learner behaves. This could be a distraction from the authentic learning process, or even attempts to manipulate algorithms. Tong et al. (Citation2021) found that while AI feedback can be of high quality it can be perceived negatively by learners. Having human educators deliver AIED feedback may be beneficial to learning but also potentially limits explicability. Many traditional pedagogies rely on a degree of authority and are rarely fully transparent. XAIED threatens to disrupt traditional pedagogical structures by laying bare aspects of the learning process, especially at scale. From a learner's point of view there can be a benefit to ‘forgetting’ past performance and not being judged by previous performance (Luckin et al. Citation2016).
The demands that AIED systems will make of future learners remained underexplored. If AI systems require learner data to be effective, will learners be permitted to withhold their data? Recommendations made to learners will require some understanding of how such computations work and a degree of critical reflectiveness to make sense of. Failure to ensure that learners have these skills risks another form of the digital divide. Similarly, little attention has been paid to the demands that AIED enhanced systems will make of learners and how they will acquire the required skills in areas like communication, self-assessment, reflection, remote work and self-management.
There is every indication that AI algorithms do more to exacerbate structural inequality than act as a corrective as a result of bias. Furthermore, personalised learning threatens to exacerbate inequality in educational experience. The solution proposed by Bulathwela et al. (Citation2021, 7) is that we embrace diversity and dialogue to ‘collectively design a global education revolution that will help us solve educational inequity’ by addressing the political and social context which engenders unequal access to quality education. AIED can contribute to this, but not through the typical forms of AI solutionism where every machine learning issue is ‘solved’ through more machine learning (Chatfield Citation2020). Maintaining explicability as a principle of organisation encourages participation and balancing dialogue around human-AI assemblages, but XAI alone cannot engage with underlying socioeconomic conditions.
Pedagogies are rarely fully transparent and so there is a need to retain the possibility of non-transparency to different stakeholders. However, this does not preclude the possibility of making those systems and algorithms transparent for auditing purposes or external examination. Making exemptions subject to scrutiny from an expert regulator would constitute a limited form of transparency that could protect stakeholders. A key goal of such audits would be to minimise the differences between XAI descriptions for various stakeholders in the presentation of socio-technical AI systems. Controlled sharing could allow audit information to be shared selectively with the public while commercially sensitive details remain opaque (Morten Citation2022).
Pasquale (Citation2020, 19) argues that “as soon as algorithms [have] effects in the world, they must be regulated and their programmers subject to ethical and legal responsibility for the harms they cause”. However, the inscrutability and complexity of machine learning has impeded attempts to regulate it, and AI lacks an agreed professional code or ethical framework (Crawford Citation2021, 214–224). Legislative moves are underway. Regulatory force in cases of AIED could include the destruction of algorithmic data, models and algorithms themselves (Kaye Citation2022). The United Nations has called for a moratorium on the sale and use of AI on the basis of risk to human rights (United Nations Citation2021). Expressing concerns about the application of AI tools to areas like law enforcement, national security, criminal justice and border management, they call for cross-sectoral regulation and a drastic increase in transparency to ameliorate the ‘black box’ problem of AI informed decision-making where algorithmic recommendations are made but it is not possible to reconstruct or explicate the process through which recommendations were generated.
In the most recent recommendations made by the UN High Commissioner to member states there is a call to ban any applications that cannot be run in full compliance with human rights legislation (ibid., 15). The USA has similarly proposed a bill of rights for AI systems (White House Citation2022) which foregrounds explanation of why ‘an automated system is being used and understand how and why it contributes to outcomes that impact you’. The bill recommends plain language reporting which is technically valid, meaningful and should be shared publicly where possible.
The forthcoming AI Act (European Commission Citation2021) proposes a regulatory framework for the exploitation of AI technologies which aims to be consistent with existing rights and values. According to the AI Act, key to building trust in AI systems is to introduce higher degrees of oversight, monitoring and transparency which are greater in higher-risk scenarios (such as those involving vulnerable groups, biometric data, social scoring, or manipulative generated content like deep fake images). The key regulatory challenge going forward is finding non-reductive ways to make socio-technical AI processes not just transparent, but understandable.
Conclusion
XAI is often portrayed as a route to ameliorating fears about the mechanisation of society. Being able to explain what is happening to those affected requires careful messaging. In educational contexts, it should always be possible to provide accounts of AIED which are interpretable to the layperson alongside more technical accounts which can be made available to specialist auditors or external examiners. Furthermore, appropriate governance measures can be put in place so that it is always possible to identify a human being who takes responsibility for what an algorithm has done or recommended (cf. Floridi, Cowls, and Beltrametti Citation2018).
It is likely that educational institutions will not in fact be the gatekeepers of AI technologies as they begin to proliferate consumer devices. Educators are already starting to integrate language processors like ChatGPT in their teaching as students increasingly use them to overcome the parameters of traditional assessments like essays. It is essential that educators engage with the impact of generative AI on existing delivery and assessment systems. It is possible that we will see the introduction of new roles that support this (such as the brokering and auditing roles described above) by drawing on the distinctively human aspects of sentience and moral agency (Véliz Citation2021; Weizenbaum Citation1976).
Greater transparency and explicability indicates a route to critical reflection upon the application of algorithms in education and AI in social life more generally. This critical review of literature has shown that a socio-technical perspective for XAIED is essential. For educators and learners to participate in AIED they need to be able to understand and meaningfully consent to AI interventions, and trust must be built as transparently as possible. The risks and impacts of AIED are in the process of becoming: XAIED is necessary for AIED, not least because the only alternatives are opaque AI or no AI. For promoting trust, ameliorating risk and the exchange of stakeholder perspectives, XAIED could even be considered a kind of default position for educational institutions. However, it is also necessary to acknowledge that radical transparency is potentially disruptive to traditional pedagogical approaches, and AIED introduces risks (such as algorithmic manipulation; bias; modifying rather than measuring behaviour; and disincentivizing learning). For learners to participate in AIED they need to be able to understand and meaningfully consent to the processes and effects of algorithmic intervention. It is hard to see how this can happen unless those who support learners also understand what is happening and all the ethical implications. Even if one could render all algorithms transparent and fully explicable, the socio-technical ecosystems of production, assembly, programming, training, using and maintaining AI systems is so diffuse as to be obscured in its entirety from any one individual view. Problematising the proposition of XAIED from a socio-technical view shows that XAI is not a full solution to the issues raised by AI, but both a beginning of and a necessary precondition for meaningful discourse about our possible futures.
Acknowledgements
Earlier versions of this paper were presented at the Computers and Learning Research Group (CALRG) and the OpenAIED group at The Open University (UK) in 2020 and 2021. The author thanks participants for their feedback and contributions.
Disclosure statement
No potential conflict of interest was reported by the author(s).
References
- Adadi, A., and M. Berrada. 2018. “Peeking Inside the Black-Box: A Survey on Explainable Artificial Intelligence (XAI).” IEEE Access 6: 52138–52160. doi:10.1109/ACCESS.2018.2870052.
- Adams, R. 2021. “Can Artificial Intelligence be Decolonized?” Interdisciplinary Science Reviews 46 (1-2): 176–197. doi:10.1080/03080188.2020.1840225.
- Antoniadi, A. M., Y. Du, Y. Guendouz, L. Wei, C. Mazo, B. A. Becker, and C. Mooney. 2021. “Current Challenges and Future Opportunities for XAI in Machine Learning-Based Clinical Decision Support Systems: A Systematic Review.” Applied Sciences 11 (11): 5088. doi:10.3390/app11115088. MDPI AG.
- Aulck, L., D. Nambi, and J. West. 2019. “Using Machine Learning and Genetic Algorithms to Optimize Scholarship Allocation for Student Yield.” In SIGKDD ‘19: ACM SIGKDD Conference on Knowledge Discovery and Data Mining, August 4–8, 2019, Anchorage, AK. ACM, New York, NY, USA. doi:10.1145/1122445.1122456.
- Baker, R. S., and A. Hawn. 2021. “Algorithmic Bias in Education.” doi:10.35542/osf.io/pbmvz.
- Baur, D. 2020. “Four Reasons Why Hyping AI is an Ethical Problem.” Medium. https://dorotheabaur.medium.com/four-reasons-why-hyping-ai-is-an-ethical-problem-8db47b17bf43.
- Beetham, H., A. Collier, L. Czerniewicz, B. Lamb, Y. Lin, J. Ross, A.-M. Scott, and A. Wilson. 2022. “Surveillance Practices, Risks and Responses in the Post Pandemic University.” Digital Culture & Education 14 (1): 16–37. https://www.digitalcultureandeducation.com/volume-14-1.
- Birhane, A., E. Ruane, T. Laurent, M. S. Brown, J. Flowers, A. Ventresque, and C. L. Dancy. 2022. “The Forgotten Margins of AI Ethics.” FAccT ‘22: Proceedings of the 2021 ACM Conference on Fairness, Accountability, and Transparency (Forthcoming). doi:10.1145/3531146.3533157.
- Bloch-Wehba, H. 2020. “Access to Algorithms.” Fordham Law Review 1265. https://ir.lawnet.fordham.edu/flr/vol88/iss4/2.
- Bulathwela, S., M. Pérez-Ortiz, C. Holloway, and J. Shawe-Taylor. 2021. “Could AI Democratise Education? Socio-Technical Imaginaries of an EdTech Revolution.” 35th Conference on Neural Information Processing Systems (NeurIPS 2021). ArXiv, abs/2112.02034. doi:10.48550/arXiv.2112.02034.
- Byrne, R. M. J. 2019. “Counterfactuals in Explainable Artificial Intelligence (XAI): Evidence from Human Reasoning.” In Proceedings of the Twenty-Eighth International Joint Conference on Artificial Intelligence Survey track. 6276–6282. doi:10.24963/ijcai.2019/876.
- Carman, M., and B. Rosman. 2020. “Applying a Principle of Explicability to AI Research in Africa: Should We Do It?” Ethics and Information Technology. doi:10.1007/s10676-020-09534-2.
- Chatfield, T. 2020. “There’s No Such Thing As ‘Ethical A.I.’.” Medium. https://onezero.medium.com/theres-no-such-thing-as-ethical-a-i-38891899261d.
- Coghlan, S., T. Miller, and J. Paterson. 2021. “Good Proctor or “Big Brother”? Ethics of Online Exam Supervision Technologies.” Philosophy and Technology 34: 1581–1606. doi:10.1007/s13347-021-00476-1.
- Cole, S. 2022. “Google’s AI-Powered ‘Inclusive Warnings’ Feature Is Very Broken.” Vice (19th April). https://www.vice.com/en/article/v7dk8m/googles-ai-powered-inclusive-warnings-feature-is-very-broken.
- Crawford, K. 2021. The Atlas of AI: Power, Politics, and the Planetary Costs of Artificial Intelligence. Yale University Press. doi:10.2307/j.ctv1ghv45t.
- Crawford, K. and Joler, V. (2018). “Anatomy of an AI System: The Amazon Echo As An Anatomical Map of Human Labor, Data and Planetary Resources.” AI Now Institute and Share Lab. https://anatomyof.ai/
- Čyras, K., A. Rago, E. Albini, P. Baroni, and F. Toni. 2021. “Argumentative XAI: A Survey.” In 30th International Joint Conference on Artificial Intelligence, edited by Z.-H. Zhou, 4392–4399. Montreal: IJCAI.
- Dignum, V. 2021. “The Role and Challenges of Education for Responsible AI.” London Review of Education 19 (1): 1–11. doi:10.14324/LRE.19.1.01.
- Ehsan, U., Q. Vera Liao, M. Muller, M. O. Riedl, and J. D. Weisz. 2021. “Expanding Explainability: Towards Social Transparency in AI Systems.” In Proceedings of the 2021 CHI Conference on Human Factors in Computing Systems (CHI ‘21). Association for Computing Machinery, New York, NY, USA, Article 82, 1–19. doi:10.1145/3411764.3445188.
- Elicit. n.d. https://elicit.org/search.
- European Commission. 2018. “Statement on Artificial Intelligence, Robotics and ‘Autonomous’ Systems.” European Commission Directorate-General for Research and Innovation, European Group on Ethics in Science and New Technologies, Brussels, 9 March 2018, Publications Office. doi:10.2777/531856.
- European Commission. 2021. Proposal for a REGULATION OF THE EUROPEAN PARLIAMENT AND OF THE COUNCIL LAYING DOWN HARMONISED RULES ON ARTIFICIAL INTELLIGENCE (ARTIFICIAL INTELLIGENCE ACT) AND AMENDING CERTAIN UNION LEGISLATIVE ACTS. 2021/0106(COD). https://eur-lex.europa.eu/legal-content/EN/TXT/?uri = CELEX%3A52021PC0206.
- European Parliament. 2022. “REPORT on Artificial Intelligence in a Digital Age (2020/2266(INI)).” Special Committee on Artificial Intelligence in a Digital Age. Rapporteur: Axel Voss. European Parliament. https://www.europarl.europa.eu/doceo/document/A-9-2022-0088_EN.pdf.
- EU. 2018. “Statement on Artificial Intelligence, Robotics and ‘Autonomous' Systems.” European Union. https://op.europa.eu/en/publication-detail/-/publication/dfebe62e-4ce9-11e8-be1d-01aa75ed71a1
- Everett, J. 2021. “From A-Levels to Pensions, Algorithms Make Easy Targets – But They Aren’t to Blame.” Guardian (17th August). https://www.theguardian.com/commentisfree/2021/aug/17/a-levels-pensions-algorithms-easy-targets-blame-mutant-maths.
- Fazelpour, S., and M. De-Arteaga. 2022. “Diversity in Sociotechnical Machine Learning Systems.” Big Data & Society, doi:10.1177/20539517221082027.
- Feathers, T. 2021. “AI Can Guess Your Race Based On X-Rays, and Researchers Don't Know How.” Motherboard. https://www.vice.com/en/article/wx5ypb/ai-can-guess-your-race-based-on-x-rays-and-researchers-dont-know-how.
- Felten, E. 2017. “What Does It Mean to Ask for an “Explainable” Algorithm?” Accessed 29 August, 2017. https://freedom-to-tinker.com/2017/05/31/what-does-it-mean-to-ask-for-anexplainable-algorithm/.
- Fiok, K., F. V. Farahani, W. Karwowski, and T. Ahram. 2022. “Explainable Artificial Intelligence for Education and Training.” The Journal of Defense Modeling and Simulation 19 (2): 133–144. doi:10.1177/15485129211028651.
- Floridi, L., and J. Cowls. 2019. “A Unified Framework of Five Principles for AI in Society.” Harvard Data Science Review 1 (1), doi:10.1162/99608f92.8cd550d1.
- Floridi, L., and J. Cowls. 2019. “A Unified Framework of Five Principles for AI in Society.” Harvard Data Science Review 1 (1), doi:10.1162/99608f92.8cd550d1.
- Floridi, L., J. Cowls, M. Beltrametti, et al. 2018. “AI4People—An Ethical Framework for a Good AI Society: Opportunities, Risks, Principles, and Recommendations.” Minds & Machines 28: 689–707. doi:10.1007/s11023-018-9482-5.
- Floridi, L., M. Holweg, M. Taddeo, J. Amaya Silva, J. Mökander, and Y. Wen. 2022. “capAI - A Procedure for Conducting Conformity Assessment of AI Systems in Line with the EU Artificial Intelligence Act (March 23, 2022).” Available at SSRN: https://ssrn.com/abstract = 4064091. doi:10.2139/ssrn.4064091.
- Future of Life. 2017. “Asilomar AI Principles.” https://futureoflife.org/ai-principles/.
- Gilpin, L. H., D. Bau, B. Z. Yuan, A. Bajwa, M. Specter, and L. Kagal. 2018. “Explaining Explanations: An Overview of Interpretability of Machine Learning.” In 2018 IEEE 5th International Conference on Data Science and Advanced Analytics (DSAA), 2018, pp. 80-89, doi:10.1109/DSAA.2018.00018.
- Google Scholar. n.d. https://scholar.google.com/.
- Guidotti, R., Monreale, A., Ruggieri, S., Turini, F., Giannotti, F. and Pedreschi, D. (2018, August). “A Survey of Methods for Explaining Black Box Models.” ACM Computing Surveys 51 (5): Article 93. doi:10.1145/3236009.
- Gunning, D., M. Stefik, J. Choi, T. Miller, S. Stumpf, and G. Z. Yang. 2019. “XAI—Explainable Artificial Intelligence.” Science Robotics 4 (37), doi:10.1126/scirobotics.aay7120.
- Hanif, A., X. Zhang, and S. Wood. 2021. “A Survey on Explainable Artificial Intelligence Techniques and Challenges.” In IEEE 25th International Enterprise Distributed Object Computing Workshop (EDOCW). pp. 81–89, doi:10.1109/EDOCW52865.2021.00036.
- Hao, K., and A. P. Hernández. 2022. “How the AI Industry Profits From Catastrophe.” MIT Technology Review (April 20th). https://www.technologyreview.com/2022/04/20/1050392/ai-industry-appen-scale-data-labels.
- Heaven, H. W. 2021. “Hundreds of AI Tools Have Been Built to Catch Covid. None of Them Helped.” MIT Technology Review. https://www.technologyreview.com/2021/07/30/1030329/machine-learning-ai-failed-covid-hospital-diagnosis-pandemic/.
- Hickok, M. 2021. “Lessons Learned from AI Ethics Principles for Future Actions.” AI Ethics 1: 41–47. doi:10.1007/s43681-020-00008-1.
- HoL. 2018. “AI in the UK: ready, willing and able? House of Lords Select Committee on Artificial Intelligence Report of Session 2017–19.” HL Paper 100. https://publications.parliament.uk/pa/ld201719/ldselect/ldai/100/100.pdf.
- Hu, B., P. Tunison, B. Vasu, N. Menon, R. Collins, and A. Hoogs. 2021. “XAITK: The Explainable AI Toolkit.” Applied AI Letters 2 (4), doi:10.1002/ail2.40.
- Human Rights Watch. 2022. “How Dare They Peep into My Private Life?” Children’s Rights Violations by Governments that Endorsed Online Learning During the Covid-19 Pandemic. Human Rights Watch. https://www.hrw.org/report/2022/05/25/how-dare-they-peep-my-private-life/childrens-rights-violations-governments.
- IEEE. n.d. Ethically Aligned Design: A Vision for Prioritizing Human Well-Being with Autonomous and Intelligent Systems. Institute of Electrical and Electronics Engineers. https://standards.ieee.org/content/dam/ieee-standards/standards/web/documents/other/ead_v2.pdf.
- Jardas, E., D. Wasserman, and D. Wendler. 2022. “Autonomy-based Criticisms of the Patient Preference Predictor.” Journal of Medical Ethics 48 (5): 304–10. doi:10.1136/medethics-2021-107629.
- Jiang, J., S. Kahai, and M. Yang. 2022. “Who Needs Explanation and When? Juggling Explainable AI and User Epistemic Uncertainty.” International Journal of Human-Computer Studies 165), doi:10.1016/j.ijhcs.2022.102839.
- Kaye, K. 2022. “The FTC’s New Enforcement Weapon Spells Death for Algorithms.” Protocol (March 14th). https://www.protocol.com/policy/ftc-algorithm-destroy-data-privacy.
- Khosravi, H., S. Buckingham Shum, G. Chen, C. Conati, Y.-S. Tsai, J. Kay, S. Knight, R. Martinez-Maldonado, S. Sadiq, and D. Gašević. 2022. “Explainable Artificial Intelligence in Education.” Computers and Education: Artificial Intelligence 3), doi:10.1016/j.caeai.2022.100074.
- Kiecza, D. 2022. “Practice Sets: A More Personal Path to Learning.” Google Classroom Blog. https://blog.google/outreach-initiatives/education/introducing-practice-sets/.
- Kiourti, P., K. Wardega, S. Jha, and W. Li. 2019. “TrojDRL: Trojan Attacks on Deep Reinforcement Learning Agents.” ArXiv, abs/1903.06638.
- Lecy, J., and K. Beatty. 2012. “Representative Literature Reviews Using Constrained Snowball Sampling and Citation Network Analysis.” SSRN Electronic Journal, doi:10.2139/ssrn.1992601.
- Luckin, R., W. Holmes, M. Griffiths, and L. B. Forcier. 2016. Intelligence Unleashed. An Argument for AI in Education. London: Pearson. https://discovery.ucl.ac.uk/id/eprint/1475756/.
- Market Research Future. 2020. “Artificial Intelligence Education Market Research Report.” https://www.marketresearchfuture.com/reports/artificial-intelligence-education-market-6365.
- Markus, A. F., J. A. Kors, and P. R. Rijnbeek. 2021. “The Role of Explainability in Creating Trustworthy Artificial Intelligence for Health Care: A Comprehensive Survey of the Terminology, Design Choices, and Evaluation Strategies.” Journal of Biomedical Informatics 113), doi:10.1016/j.jbi.2020.103655.
- Montréal Declaration for a Responsible Development of Artificial Intelligence. 2017. https://www.montrealdeclaration-responsibleai.com/the-declaration.
- Morley, J., A. Elhalal, F. Garcia, L. Kinsey, J. Mökander, and L. Floridi. 2021. “Ethics as a service: a pragmatic operationalisation of AI Ethics (February 11th).” Available at SSRN: https://ssrn.com/abstract = 3784238. doi:10.2139/ssrn.3784238.
- Morten, C. 2022. “Publicizing Corporate Secrets for Public Good.” University of Pennsylvania Law Review, Vol. 171, Forthcoming. doi:10.2139/ssrn.4041556.
- Moss, E., E. A. Watkins, R. Singh, M. C. Elish, and J. Metcalf. 2021. “Assembling Accountability: Algorithmic Impact Assessment for the Public Interest.” Data & Society. https://datasociety.net/library/assembling-accountability-algorithmic-impact-assessment-for-the-public-interest/.
- Mueller, S. T., R. R. Hoffman, W. Clancey, A. Emrey, and G. Klein. 2019. “Explanation in human-AI systems: A literature meta-review, synopsis of key ideas and publications, and bibliography for explainable AI [Preprint].” DARPA XAI Literature Review. February 9. https://arxiv.org/pdf/1902.01876.pdf.
- Noble, S. U. 2018. Algorithms of Oppression. NYU Press.
- OpenAI. 2023. “ChatGPT.” https://chat.openai.com/auth/login.
- Orr, D., M. Weller, and R. Farrow. 2018. “Models for Online, Open, Flexible and Technology-Enhanced Higher Education Across the Globe – A Comparative Analysis.” International Council for Open and Distance Education (ICDE). Oslo, Norway. https://oofat.oerhub.net/OOFAT/ CC-BY-SA.
- Páez, A. 2019. “The Pragmatic Turn in Explainable Artificial Intelligence (XAI).” Minds & Machines 29: 441–459. doi:10.1007/s11023-019-09502-w.
- Panigutti, C., A. Perotti, and D. Pedreschi. 2020. “Doctor XAI: An Ontology-Based Approach to Black-Box Sequential Data Classification Explanations.” In Conference on Fairness, Accountability, and Transparency (FAT* ‘20), January 27–30, 2020, Barcelona, Spain. ACM, New York, NY, USA, 11 pages. doi:10.1145/3351095.3372855.
- Partnership on AI. 2018. “About Us.” https://partnershiponai.org/about/.
- Pasquale, F. 2020. New Laws of Robotics: Defending Human Expertise in the Age of AI. Harvard University Press.
- Peterson, D., K. Goode, and D. Gehlhaus. 2021. AI Education in China and the United States: A Comparative Assessment. Center for Security and Emerging Technology.
- Prinsloo, P., S. Slade, and M. Khalil. 2022. “At the Intersection of Human and Algorithmic Decision-Making in Distributed Learning.” Journal of Research on Technology in Education, doi:10.1080/15391523.2022.2121343.
- Ricaurte, P. 2022. “Artificial Intelligence and the Feminist Decolonial Imagination.” Bot Populi (March 4th). https://botpopuli.net/artificial-intelligence-and-the-feminist-decolonial-imagination/.
- Robbins, S. A. 2019. “Misdirected Principle with a Catch: Explicability for AI.” Minds & Machines 29: 495–514. doi:10.1007/s11023-019-09509-3.
- Roio, D. 2018. “Algorithmic Sovereignty.” PhD diss., The University of Plymouth. http://hdl.handle.net/10026.1/11101.
- Russell, S. J., and P. Norvig. 2021. Artificial Intelligence: A Modern Approach. 4th ed. Prentice Hall.
- Saeed, W., and C. Omlin. 2021. “Explainable AI (XAI): A Systematic Meta-Survey of Current Challenges and Future Opportunities.”. doi:10.48550/arXiv.2111.06420.
- Samuel, S. 2021. “AI’s Islamophobia problem.” Vox. https://www.vox.com/future-perfect/22672414/ai-artificial-intelligence-gpt-3-bias-muslim.
- Schlegel, U., H. Arnout, M. El-Assady, D. Oelke, and D. A. Keim. 2019. “Towards A Rigorous Evaluation Of XAI Methods On Time Series.” In 2019 IEEE/CVF International Conference on Computer Vision Workshop (ICCVW) pp. 4197–4201, doi:10.1109/ICCVW.2019.00516.
- Schwab, K. 2016. The Fourth Industrial Revolution. World Economic Forum.
- Searle, J. 1980. “Minds, Brains and Programs.” Behavioral and Brain Sciences 3 (3): 417–457.
- Selbst, A. D., D. Boyd, F. Sorelle, V. Suresh, and J. Vertesi. 2018. “Fairness and Abstraction in Sociotechnical Systems (August 23, 2018).” In 2019 ACM Conference on Fairness, Accountability, and Transparency (FAT*), 59-68, Available at SSRN: https://ssrn.com/abstract = 3265913.
- Sharples, M., and R. Pérez y Pérez. 2022. Story Machines: How Computers Have Become Creative Writers. Routledge.
- Statista. 2020. “Artificial Intelligence (AI) worldwide - Statistics & Facts.” https://www.statista.com/topics/3104/artificial-intelligence-ai-worldwide/.
- Timms, M. J. 2016. “Letting Artificial Intelligence in Education Out of the Box: Educational Cobots and Smart Classrooms.” International Journal of Artificial Intelligence in Education 26: 701–712. doi:10.1007/s40593-016-0095-y.
- Tjoa, E., and C. Guan. 2021. “A Survey on Explainable Artificial Intelligence (XAI): Toward Medical XAI.” IEEE Transactions on Neural Networks and Learning Systems 32 (11): 4793–4813. DOI:10.1109/tnnls.2020.3027314. PMID: 33079674.
- Tong, S., N. Jia, X. Luo, and Z. Fang. 2021. “The Janus Face of Artificial Intelligence Feedback: Deployment Versus Disclosure Effects on Employee Performance.” Strategic Management Journal 42), doi:10.1002/smj.3322.
- Tutt, A. 2020. “An FDA for Algorithms.” Administrative Law Review, 69(1). https://administrativelawreview.org/wp-content/uploads/sites/2/2019/09/69-1-Andrew-Tutt.pdf.
- United Nations. 2021. “The Right to Privacy in the Digital Age.” Report of the United Nations High Commissioner for Human Rights. A/HRC/48/31. https://documents-dds-ny.un.org/doc/UNDOC/GEN/G21/249/21/PDF/G2124921.pdf.
- Véliz, C. 2021. “Moral Zombies: Why Algorithms are not Moral Agents.” AI & Society 36: 487–497. doi:10.1007/s00146-021-01189-x.
- Vera Liao, Q., D. Gruen, and S. Miller. 2020. “Questioning the AI: Informing Design Practices for Explainable AI User Experiences.” In Proceedings of the 2020 CHI Conference on Human Factors in Computing Systems. Association for Computing Machinery, New York, NY, USA, 1–15. doi:10.1145/3313831.3376590.
- Viljoen, S. 2021. “A Relational Theory of Data Governance.” The Yale Law Journal (Forthcoming), doi:10.2139/ssrn.3727562.
- Wachter, S. forthcoming. “The Theory of Artificial Immutability: Protecting Algorithmic Groups under Anti-Discrimination Law (February 15, 2022).” Tulane Law Review. Available at SSRN: https://ssrn.com/abstract = 4099100. doi:10.2139/ssrn.4099100.
- Weitz, K. 2022. “Towards Human-Centered AI: Psychological Concepts as Foundation for Empirical XAI Research.” it - Information Technology 64 (1-2): 71–75. doi:10.1515/itit-2021-0047.
- Weizenbaum, J. 1976. Computer Power and Human Reason: From Judgment to Calculation. W. H. Freeman and Company.
- White House. 2022. “Blueprint for an AI Bill of Rights – Making Automated Systems work for the American People.” https://www.whitehouse.gov/ostp/ai-bill-of-rights/.
- Whittlestone, J., R. Nyrup, A. Alexandrova, K. Dihal, and S. Cave. 2019. Ethical and Societal Implications of Algorithms, Data, and Artificial Intelligence: A Roadmap for Research. London: Nuffield Foundation. https://www.nuffieldfoundation.org/sites/default/files/files/Ethical-and-Societal-Implications-of-Data-and-AI-report-Nuffield-Foundat.pdf.
- Wohlin, C. 2014. “Guidelines for snowballing in systematic literature studies and a replication in software engineering.” In EASE ‘14: Proceedings of the 18th International Conference on Evaluation and Assessment in Software Engineering (May 2014). Article 38, Pages 1–10. doi:10.1145/2601248.2601268.
- Xuesong, Z., X. Chu, C. S. Chai, M. S. Y. Jong, A. Istenic, M. Spector, J.-B. Liu, J. Yuan, and Y. Li. 2021. “A Review of Artificial Intelligence (AI) in Education from 2010 to 2020.” Complexity 2021: 18. doi:10.1155/2021/8812542.
- Zawacki-Richter, O., V. I. Marín, M. Bond, and F. Gouveneur. 2019. “Systematic Review of Research on Artificial Intelligence Applications in Higher Education – Where are the Educators?” International Journal of Educational Technology in Higher Education 16: 39. doi:10.1186/s41239-019-0171-0.
- Zhang, Y., and X. Chen. 2020. “Explainable Recommendation: A Survey and New Perspectives.” Foundations and Trends® in Information Retrieval 14 (1): 1–101. doi:10.1561/1500000066.
- Zuboff, S. 2019. The Age of Surveillance Capitalism. Public Affairs Books.