1. Introduction
Structure-based drug design (SBDD) heavily relies on the production of high-resolution (<2Å) three-dimensional (3D) structures of the drug target in the presence or absence of the drug candidate [Citation1]. X-ray crystallography is the predominant technique accounting for higher than 94% of the structures in Protein Data Bank (PDB), considering the complexes involving protein and inhibitors [Citation2–Citation4]. Macromolecular X-ray crystallography provides an important and powerful technique in studying the specific interactions of a particular drug with its protein target at the atomic level that can help improve the drug design process [Citation5]. It is the main technique used to obtain 3D information for binary complexes involving protein and drugs [Citation6].
One of the major ‘bottlenecks’ in X-ray crystallography is the lack of generalized methods for high quality crystal production. Since protein crystallization mechanism details remain unknown, protein crystallization is a complicated and time-consuming process and requires performing a significant number of trial-and-error experiments involving systematic testing of variable chemical and physical parameters [Citation7]. High-throughput (HT) structural biology coincided with the dawn of the genomic era in biology requires the automation, miniaturization, and parallelization of protein crystallization in order to reach the capacity necessary for large-scale structure determination efforts [Citation8]. HT protein crystallization screening (HTPCS) technologies appeared on the protein crystallization scene more than three decades ago and have since allowed accessing hundreds to thousands of protein crystallization conditions, thereby greatly impacting HT structural biology [Citation9]. HTPCS has helped to identify critical components required for HT crystallization efforts [Citation7]. In recent years, the rapid developments of manipulation techniques and devices provide effective and reliable solutions for protein crystallization screening with HT and low consumption. In spite of their advances, HTPCS has suffered from two main handicaps, namely, poor hit rate in protein crystallization screening and lack of predictive power of the scoring functions. To overcome these handicaps, several projects have recently been initiated to construct ‘smart systems’ that are not only capable of rapidly performing a large number of crystallization trials, but also scripting and triggering certain events based on the collected data used in predict the outcome of a protein x-ray crystallization trial [Citation10]. In this contest, we will outline recent efforts in HTPCS that could improve the success rate of the structural pipeline. We will discuss the challenge and some of the possible avenues in that direction.
2. Recent advances in HTPCS technologies
Obtaining high-quality protein crystals suitable for X-ray crystallography largely relies on HT screening of hundreds to thousands of protein crystallization conditions. Recently, the rapid developments of automation techniques, devices, and algorithm provided effective and reliable HT methods for protein crystallization and screening. In the following five aspects are discussed, corresponding to different stages of the HTPCS pipeline ().
Figure 1. A flow diagram showing the overall process for sample characterization, starting from the PCS to obtaining experimental justification for a smart HTPCS system. Please see text for details.
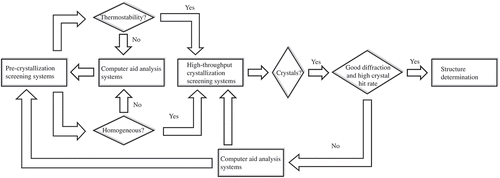
2.1. Pre-crystallization screening
Pre-crystallization screening (PCS) refers to experiments conducted before crystallization experiments are performed. PCS is widely used to provide ‘quality-control’ data prior to performing crystallization screening experiments. PCS has helped to identify critical components required for HT crystallization efforts: select the best protein sample for crystallization trials; determined the appropriate protein concentration for crystallization screening; evaluated the thermostability of proteins in an HT manner; limited proteolysis [Citation11], surface manipulation [Citation12], use of function based additives, such as compatible ligands, inhibitors, or detergents [Citation13,Citation14], etc. In the recent years, the concept of systems biology and synthetic biology was used to rapidly evolve protein or screen suitable small parts (proteins or chemicals) which form the complex with target protein to facilitate protein crystallization. PCS has been proved that significantly increases the chances of obtaining initial crystal hits, expediting efforts in generating high-resolution structures of challenging protein targets [Citation15].
2.2. Protein crystallization methods
Traditional crystallization methods can be classified into the three basic categories of batch diffusion, vapor diffusion and liquid diffusion, which have been developed and utilized with HTPCS technologies [Citation16]. In recent years, new crystallization methods such as in vivo protein crystallization, counter-diffusion technique, supercritical fluid crystallization, electric field, magnetic field, microgravity controlled precipitation, agarose gel solution crystallization can be used to produce nanocrystals [Citation17]. The most remarkable improvement is being done on the microfluidic array chip with micrometer-sized crystallization space [Citation18].
In spite of advances in protein crystallization methods, obtaining high-quality protein crystals suitable for X-ray crystallography largely relies on HT screening of hundreds to thousands of protein crystallization conditions. Since the 1990s, many sparse matrix screening systems have been developed not only for soluble proteins but also for membrane proteins [Citation19–Citation21]. In recent years, with the rapid developments of automated liquid handling techniques and instrumentation, the crystallization process has the benefit of increasing speed and decreasing sample volume [Citation22].
2.3. Automated techniques and instrumentation
Recently, benefiting from the rapid development of technologies such as mechanical automation, control technology, and microfluidics, researchers have developed a series of fluid manipulation technologies and devices which provide effective and reliable solutions for protein crystallization screening with HT and low consumption [Citation23]. Generally, a sophisticated liquid-handling system is used to combine and mix the reservoir solutions and a second system sets up the sample droplets. In order to improve the liquid handling accuracy and reliability of the pipette, many companies have developed a number of automated liquid handling workstations and laboratory automation technologies to the scientific community, such as Douglas Instruments company’s Oryx systems, TTP Labtech’s mosquito series, Art Robbins Instruments’ Crystal Phoenix and Crystal Gryphon LCP, Digilab’s HoneyBee series, Tecan’s Freedom EVO workstation. Acoustic droplet ejection (ADE) using a pulse of ultrasound to move low volumes of fluids (typically nanoliters or picoliters) without any physical contact were used to improve protein crystal quality, facilitate protein crystallization, and improve HT structural biology [Citation24]. Teplitsky and coworker described a HT method for screening up to 1728 distinct chemicals to co-position 2.5nL of protein, precipitant, and chemicals with protein crystals on a single microplate using ADE [Citation22]. Microfluidics is a technique for manipulating fluids in microstructures on the micron scale, with conventional liquid handling volumes ranging from femtoliters to nanoliters. This technique can greatly reduce sample consumption in protein crystallization [Citation18]. In recent years, the microfluidic technology used in HT protein crystal screening has developed rapidly, such as Formulatrix’s Formulator and Mantis, Protein BioSolutions’ Plug Maker systems. These devices are striving to increase the efficiency of the hit-identification process and/or diffraction data collection.
2.4. HTPCS platforms
A fully automated HT crystallization facility is still expensive to set up and maintain. They are therefore typically shared by a consortium and/or publicly funded and hence open to the national or international user community. In recent years, HTPCS platforms have been set up and provide HTPCS services for the scientific community, which has promoted the great development of structural biology and drug discovery. These HTPCS platforms, such as EMBL Hamburg HTP protein crystallization, SGC HT Protein Crystallization, SLS crystallization platform, have established a fully automated platform for the HT crystallization of protein and offer a wide range of crystallization screening conditions and flexible methods have been developed to adapt each step to individual project requirements. For the individual laboratories who have not automated HT crystallization facility, just need to send their samples to platforms who perform crystal-growth screening experiments in microassay plates with a predetermined set of crystallization cocktails for both soluble and membrane biological macromolecules. The rapid development and popularization of internet technology allow us to remotely control the HT crystallization instrumentation for performing crystallization screening and optimization.
2.5. Smart HTPCS system
Recently, computer-aided design (CAD) has been used to generate optimized cocktails based on the initial screening results to lead to higher scoring outcomes, crystals [Citation25]. The computational methods can provide additional information which is the new driving force for improvement in HTPCS. The computational methods analyze initial crystal screening results to determine those screening factors in chemical space that are most likely to lead to higher scoring outcomes, crystals. The computational methods also can provide the optimization screens, based on the results found in the initial and adjusted screens, to obtain large single crystals suitable for X-ray diffraction [Citation26]. As artificial intelligence (AI) advances by the day, HTPCS is beginning to combine it with automated self-learning approaches (smart HTPCS system). Current state-of-the-art solutions of fully integrated crystallization workstation are already providing a blueprint of what could be achieved with smart HTPCS. These workstations integrate multiple functions including the camera, bar coding, plate handling, plate storage, environmental control and software management. In hopes of creating better access to protein crystallization, researchers are doing to develop algorithms and hardware devices, which provide the ability to design smarter and faster experiments by scripting and triggering certain events based on the collected data, for example, automated image collection used in predict the outcome of a protein x-ray crystallization trial [Citation10].
3. Conclusions
Whilst improving the reliability and validity of the automated techniques and instrumentation for HTPCS, there have been significant improvements in crystallization success rate, and producing crystals of greater diffraction quality and quantity. The amount of protein required for crystallization screening has been gradually reduced. The recent years have witnessed increasing HT crystallization screening platforms with a strong focus on no only HT and low consumption but also intelligent screening. Currently, the new developed ‘smart systems,’ computer-aided crystallization screening, are typically applied to increase the efficiency of HTPCS. In order to meet the diverse requirements of HT structural biology, more innovative technical solutions, which can not only directly address traditional HT shortcomings, but also provide additional information content to be used for a more successful protein crystallization process are required.
4. Expert opinion
Bigger focus on cost reduction and efficiency improvements within drug discovery plays nowadays an important role for the application of X-ray crystallography for high-resolution 3D structures of the drug target in the presence or absence of the drug candidate. HTPCS approaches are capable of providing crystalline material that covers from the nano to the millimeter sizes range produced, which meets the requirement of 3D model determination. Although there are already a growing number of structures published using HTPCS, the technology is not yet a mature, fully automated, and still presents a number of unique challenges as well as opportunities for structural biologists.
SBDD requires accurate structural information with the native conformation of a protein. However, scientists frequently select the protein that is suitable for crystallization but far from the physiological condition. It has been well known that protein conformation may be sensitive to the crystallization solution, and the different crystallization conditions or buffer selection can be a source of irreproducibility [Citation27]. It is a big challenge to find crystallization conditions that are close to the physiological conditions of a protein. To guide drug design with the native conformation of a protein, the developments of the proprietary crystallization screen kits with soft features for the protein to maintain its native conformation for drug target protein crystallization and new methods that can help select the best protein sample for crystallization trials are required.
Taking advantage of computer science techniques, the previous crystallization trials have been used to develop tools that computationally predict the crystallization outcome and define mutational approaches that enhance the likelihood of crystallization [Citation28]. Achieving its full potential requires a significant increase in the amount and quality of available data [Citation28]. However, the fact is that we do not have a publicly available universal database of crystallization conditions or that it currently may not be possible to create such a database because the crystallization condition data within the PDB is incomplete and often inconsistent with the associated publication. Recently, a couple of dedicated crystallization condition databases, such as the TargetTrack database that kept all crystallization condition for all structural genomics targets, have been closed due to lack of funding. Although a number of crystallization facilities have also been gathering datasets that contain the experimental details for each crystallization attempt, for both positive and negative results [Citation28], these databases are incomplete and always don’t open to entire scientific community or pharmaceutical companies. The full potential of crystallization screening information is limited by the amount and quality of available data. Thus, it will be necessary to establish a publicly available universal database of crystallization conditions such that each individual has the same chance to use this source to facilitate protein crystallization, which and then promotes the development of structural biology and drug discovery.
Recently, HT crystallization screening systems are getting smarter with developed software and devices. Due to lack of skill or knowledge and high maintenance costs, the smart screening systems will not achieve maximum performance in individual laboratories. The most effective way to increase efficiency would be to set up HT crystallization screening platforms in large institutions, which can provide screening services for each individual. As reported by the Structural Genomics Consortium (SGC) in 2016, more than 200 scientists used the facility over the ten-year period, generating over 60 000 crystallization plates from 2339 targets and over 24 000 protein purifications, and of all targets for which purified protein was ever available, 43% eventually yielded diffracting crystals [Citation20]. However, the large facilities require good and sustained funding to not only maintain the facility but also to constantly update the hardware and software. An HT crystallization facility will not generate enough money from service and therefore it would be necessary to provide a mechanism of funding similar to the one implemented for synchrotron facilities. Another issue is the confidentiality if these facilities would be used by pharmaceutical companies. It will be necessary to consider several legal responses to this issue as it relates to the promotion and protection of pharmaceutical companies, especial of small and medium-sized pharmaceutical companies.
Current state-of-the-art solutions for fully integrated crystallization workstation are already providing a blueprint of what could be achieved with smart HTPCS. The smart HTPCS is a pervasive trend that is rapidly accelerating thanks to vast amounts of data and progress in both algorithms and the processing capacity of modern devices. The design of smarter experiments will be a required first step toward improving HT crystallization screening in the long term. Thus, it will be necessary to develop more efficient algorithms and to establish a hierarchy of methods such that most conditions can be rapidly ruled out while still delivering accurate predictions for the most promising candidates. We are still far away from the accurate calculation of protein crystallization conditions, but the time is right to increase the success rate of going from a new protein to a structure.
Declaration of interest
The author has no relevant affiliations or financial involvement with any organization or entity with a financial interest in or financial conflict with the subject matter or materials discussed in the manuscript. This includes employment, consultancies, honoraria, stock ownership or options, expert testimony, grants or patents received or pending, or royalties. Peer reviewers on this manuscript have no relevant financial or other relationships to disclose
Acknowledgments
The author thanks Ms. Aiping Qian for her critical reading and artwork.
Additional information
Funding
References
- Grey JL, Thompson DH. Challenges and opportunities for new protein crystallization strategies in structure-based drug design. Expert Opin Drug Discov. 2010;5:1039–1045.
- Heck GS, Pintro VO, Pereira RR, et al. Supervised machine learning methods applied to predict ligand- binding affinity. Curr Med Chem. 2017;24:2459–2470.
- Burley SK, Berman HM, Christie C, et al. Rcsb protein data bank: sustaining a living digital data resource that enables breakthroughs in scientific research and biomedical education. Protein Sci. 2018;27:316–330.
- Burley SK, Berman HM, Kleywegt GJ, et al. Protein data bank (PDB): the single global macromolecular structure archive. Methods Mol Biol. 2017;1607:627–641.
- de Azevedo WF Jr., Canduri F, Basso LA, et al. Determining the structural basis for specificity of ligands using crystallographic screening. Cell Biochem Biophys. 2006;44:405–411.
- Canduri F, de Azevedo WF. Protein crystallography in drug discovery. Curr Drug Targets. 2008;9:1048–1053.
- Luft JR, Snell EH, Detitta GT. Lessons from high-throughput protein crystallization screening: 10 years of practical experience. Expert Opin Drug Discov. 2011;6:465–480.
- Elsliger MA, Deacon AM, Godzik A, et al. The jcsg high-throughput structural biology pipeline. Acta Crystallogr Sect F Struct Biol Cryst Commun. 2010;66:1137–1142.
- Skarina T, Xu X, Evdokimova E, et al. High-throughput crystallization screening. Methods Mol Biol. 2014;1140:159–168.
- Lekamge B, Sowmya A, Newman J. Prediction of protein x-ray crystallisation trial image time-courses. In: Marsico DM, Baja GS, Fred A, editors. Proceedings of the 6th International Conference on Pattern Recognition Applications and Methods. Setúbal: SciTePress; 2017. p. 663–668.
- Dong A, Xu X, Edwards AM, et al. In situ proteolysis for protein crystallization and structure determination. Nat Methods. 2007;4:1019–1021.
- Derewenda ZS, Vekilov PG. Entropy and surface engineering in protein crystallization. Acta Crystallogr D Biol Crystallogr. 2006;62:116–124.
- McPherson A, Cudney B. Searching for silver bullets: an alternative strategy for crystallizing macromolecules. J Struct Biol. 2006;156:387–406.
- Chruszcz M, Zimmerman MD, Wang S, et al. Function-biased choice of additives for optimization of protein crystallization - the case of the putative thioesterase PA5185 from pseudomonas aeruginosa pao1. Cryst Growth Des. 2008;8:4054–4061.
- Milic D, Veprintsev DB. Large-scale production and protein engineering of g protein-coupled receptors for structural studies. Front Pharmacol. 2015;6:66.
- Russo Krauss I, Merlino A, Vergara A, et al. An overview of biological macromolecule crystallization. Int J Mol Sci. 2013;14(6):11643–11691.
- Moreno A. Advanced methods of protein crystallization. Methods Mol Biol. 2017;1607:51–76.
- Schieferstein JM, Pawate AS, Sun C, et al. X-ray transparent microfluidic chips for high-throughput screening and optimization of in meso membrane protein crystallization. Biomicrofluidics. 2017;11:024118.
- Jancarik J, Kim S-H. Sparse matrix sampling: a screening method for crystallization of proteins. J Appl Cryst. 1991;24:409–411.
- Ng JT, Dekker C, Reardon P, et al. Lessons from ten years of crystallization experiments at the SCG. Acta Crystallogr D Struct Biol. 2016;72:224–235.
- Gorrec F. Protein crystallization screens developed at the MRC laboratory of molecular biology. Drug Discov Today. 2016;21:819–825.
- Teplitsky E, Joshi K, Ericson DL, et al. High throughput screening using acoustic droplet ejection to combine protein crystals and chemical libraries on crystallization plates at high density. J Struct Biol. 2015;191:49–58.
- Stewart PS, Mueller-Dieckmann J. Automation in biological crystallization. Acta Crystallogr F Struct Biol Commun. 2014;70:686–696.
- Yin X, Scalia A, Leroy L, et al. Hitting the target: fragment screening with acoustic in situ co-crystallization of proteins plus fragment libraries on pin-mounted data-collection micromeshes. Acta Crystallogr D Biol Crystallogr. 2014;70:1177–1189.
- Dinc I, Pusey ML, Aygun RS. Optimizing associative experimental design for protein crystallization screening. IEEE Trans Nanobiosci. 2016;15:101–112.
- Wang H, Feng L, Zhang Z, et al. Crysalis: an integrated server for computational analysis and design of protein crystallization. Sci Rep. 2016;6:21383.
- Majorek KA, Kuhn ML, Chruszcz M, et al. Double trouble-buffer selection and his-tag presence may be responsible for nonreproducibility of biomedical experiments. Protein Sci. 2014;23:1359–1368.
- Altan I, Charbonneau P, Snell EH. Computational crystallization. Arch Biochem Biophys. 2016;602:12–20.