Abstract
Although contact network models have yielded important insights into infectious disease transmission and control throughout the last decade, researchers have just begun to explore the dynamic nature of contact patterns and their epidemiological significance. Most network models have assumed that contacts are static through time. Developing more realistic models of the social interactions that underlie the spread of infectious diseases thus remains an important challenge for both data gatherers and modelers. In this article, we review some recent data-driven and process-driven approaches that capture the dynamics of human contact, and discuss future challenges for the field.
1. Introduction
Many pathogens spread through host populations via close interactions. The interpersonal contact patterns that underlie disease transmission among hosts can naturally be thought to form a network, where links join individuals who interact with each other. All epidemiological models make assumptions about the underlying network of interactions, often without explicitly stating them. Contact network models, however, mathematically formalize this intuitive concept so that epidemiological calculations can explicitly consider complex patterns of interactions. A node in a contact network represents an individual host, and an edge between two nodes represents an interaction (that has occurred at any point in time for any period) which may allow disease transmission. A node's degree is the number of edges (i.e. contacts) attached to it.
Much of the current work in network epidemiology is based on static contact networks, which are graphs where nodes and edges remain fixed for the duration of the epidemic in the population. Static networks assume that contacts are permanent and provide a useful tool for predicting the spread of disease through relatively stable populations Citation5 Citation31. However, social interactions are often quite fluid: new connections form, while others dissolve, providing transient opportunities for disease transmission. A dynamic representation of a contact network, in which nodes and edges shift according to changes in the population, would reflect this reality more. Dynamic and evolving networks have been studied in the context of social or technological networks in many instances. Most have concentrated on how nodes create or change their links based on either popularity of other nodes in the network Citation8, social distances from other nodes Citation33, or interaction payoffs in a game-theoretic framework Citation57.
In the context of epidemiology, the dynamic nature of host contacts and related properties, such as the order in which contacts occur and the concurrency (i.e. temporal overlap) of contacts, can significantly influence the spread of disease through a population and the efficacy of various intervention strategies. In this paper, we explore recent and ongoing efforts to characterize and study the relationship between dynamic contact networks and disease transmission. In Section 2, we consider a data-driven approach to dynamic network modelling. Specifically, we discuss available dynamic contact data and methods for estimating dynamic network structure from such data. Section 3 describes mechanistic approaches to modelling dynamic contact networks. Finally, in Section 4, we discuss future challenges for the field of dynamic contact network epidemiology.
2. Building models from dynamic contact data
The dynamics of disease transmission through a networked population has received much (deserved) attention in the field of mathematical epidemiology over the last two decades. Here, we shift the focus from the dynamics of disease spreading through a network to the dynamics of the underlying host network itself. In particular, we consider changes in individual contacts and broad-scale flux in contact patterns that govern the structure of the network through which disease spreads.
The number of longitudinal data sets that provide information about the timing, identity and duration of contacts across a diverse host population is still limited, but there are many more on the horizon. An example of a well-characterized dynamic contact network is one built from records of cattle movement in the UK, which represents the movement of every animal between cattle premises on a daily scale. For human beings, there have been several network-based studies addressing the transmission of HIV and other sexually transmitted diseases (STDs) that have revealed dynamic contact behaviour Citation54. Also, a study by Read et al. Citation51 records both close contacts (as measured by physical touch) and casual contacts (as measured by conversations) for a small sample of individuals over 14 non-consecutive days, and thus provides information about the network transmission of non-STDs.
Below, we discuss the representation of dynamic contact data and features of such data that are relevant to the study of infectious disease. Although not the focus of this article, the field of statistical network modelling has advanced the modelling of longitudinal network data using both continuous-time and discrete-time Markov approaches such as dynamic exponential random graph models Citation59 and latent space models Citation55. Although motivated by sociological applications, these methods can also help study the epidemiological consequences of dynamic contact patterns. There are also useful algorithmic approaches to analyse the evolution of network structure through time that were originally developed in the context of technological and information networks Citation1 Citation36.
2.1. Representations of dynamic networks
A key question for epidemiological modelling is whether the interactions that underlie disease transmission can be adequately captured by a static network model or whether their complex dynamics must be modelled explicitly. The importance of network dynamics depends on both the timescale over which a pathogen spreads and the particular aspects of disease dynamics one wishes to study Citation52 Citation62. For rapidly spreading pathogens that can cause acute infection, it is often assumed that a static network description will suffice (e.g. Citation25 Citation40). This assumption enables more straightforward mathematical calculations of disease dynamics and has been the basis for much work in the field, to date. Specifically, static networks have provided insight into problems of disease ecology Citation3 Citation49, animal health problems related to foot and mouth disease and scrapie Citation25, and public health problems related to severe acute respiratory syndrome (SARS), walking pneumonia, influenza, and gonorrhea Citation4 Citation6 Citation19 Citation39 Citation40 among many others.
One common method for integrating dynamic contact information into a static network model is to construct ‘time-integrated networks’ Citation16 Citation40 Citation43. These models consider all contacts that may have occurred within a relevant period of time (e.g. an average infectious period) and aggregate these contacts into a single static contact network. However, different dynamic data can give rise to the same aggregated static contact network (). In addition, static network-based simulation studies often assume that host individuals have contacts with a unique, fixed set of individuals at all times during the simulation. This assumption not only takes identity of contacts to be identical but also that contacts are repeated at every time step, allowing multiple opportunities for transmission. In a recent study, Mossong et al. Citation41 showed that in a large-scale survey study on contact behaviour, less than 50% of all contacts occurred on a daily basis, while approximately 10% of all contacts occurred for the first time (and possibly the last time). Similarly, Read et al. Citation51 showed that approximately three quarters of the individuals met by study participants during a 14-day period were encountered only on one of those days.
Figure 1. Static network models of dynamic networks. (a)–(d) are sequential ‘snapshots’ of a dynamic network of contacts (lines) between individuals (circles). (e) A static network representation of the network, where edges denote the existence of a contact in the dynamic network. (f) A weighted network representation, where the edge weights (line thickness) indicate the number of times any two individuals are in contact. (g) Another weighted network representation, where weights represent the number of consecutive times any two individuals are in contact.
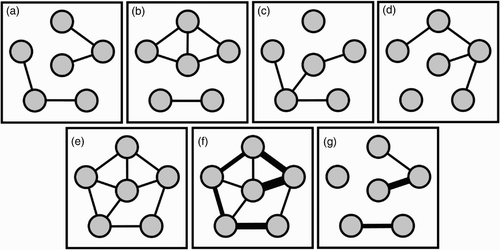
Using UK cattle movement data, Vernon and Keeling Citation60 compare several static network representations as approximations to a full dynamic network (some of which we illustrate in ). For livestock movement, the most common representation models cattle premises as graph nodes, and movements between premises as edges. A natural static network approximation of the full dynamic network of cattle movement is a weighted static network in which movements are aggregated over some period of time and the frequency of movements are represented as weights on edges. The authors find that this simple approximation fails to reproduce the distribution of epidemic sizes produced by the corresponding full dynamic network model (which produces a wider distribution) and thus caution against such simplifications when making detailed epidemiological predictions.
Another approach which incorporates intrinsic temporal structure into static network epidemic models is the use of line graphs. This approach was developed in the context of human STDs (sexually transmitted diseases) to represent concurrency of sexual relationships between sexually active individuals, and it was applied more recently in Citation28 to incorporate the temporal sequence of movements between cattle premises into a static graph representation of UK cattle movement. In this case, nodes represent movements (not premises) characterized by source, target, and date of movement; and edges between these movement nodes occur when the movement target for one node is the movement source for another on the same or later date. These network models not only capture spatial relationships in the data (similar to other models), but also represent temporal sequence relationships, and prove to be computationally more efficient than other models Citation28.
Although the methods just described provide valuable steps towards adequate and tractable representations of dynamic network data, none are able to incorporate all of the important structural properties. Below, we discuss some of these properties and consider their importance for infectious disease epidemiology.
2.2. Epidemiological significance of dynamic contact patterns
For acute, close-contact infections of human beings, the dynamics of physical contacts may critically influence the extent and rate of spread. A contact, or series of contacts, can be characterized by the following features: the duration of one contact between two specific individuals; the frequency of contact events between two specific individuals; the order of contacts between pairs of individuals within a larger group; the concurrency (that is, temporal overlap) contacts between a particular individual and a set of other individuals; and the rate at which the identity of a contact partner changes.
Several studies have investigated the impacts of such temporal features on epidemiological processes. The work of Smieszek et al. Citation58 suggests that higher frequencies of repeated contacts reduces epidemic size, and that this effect is stronger with low numbers of contacts or a low-transmission probability. Read et al. Citation51 demonstrated that both high regularity and duration of contacts have the same effect, using an empirical data set of physical contacts. On the other hand, high concurrency of contacts, which has been studied intensely in the context of sexually transmitted infections, leads to both faster-growing outbreaks and larger final epidemic sizes. (Recent work, however, has sparked controversy on the role of concurrency in driving HIV epidemics in Africa Citation37). Traditional static network models predict a direct relationship between the probability of transmission between individuals and the extent of an outbreak. The results of Volz and Meyers Citation62, however, suggest that the evolution of network structure through contact turnover can produce more complex patterns of disease spread, changing our fundamental understanding of disease dynamics Citation22.
These studies indicate that the dynamics of physical contacts can profoundly influence the spread of disease and should thus be incorporated into our epidemiological models. In a sense, contact patterns lie on a spectrum. At one extreme is infinitely fluid populations in which contacts are instantaneous and chosen randomly, in other words, mass-action populations. At the other extreme is rigid populations in which all contacts are permanent, in other words, static network populations. In between lie a range of dynamic networks in which contacts may be of variable duration and concurrency Citation62.
3. Modelling dynamic contact processes
How can a contact network change? What can drive these changes? The alternative to a data-driven approach to modelling dynamic contacts is a mechanistic process-driven approach. In this section, we identify three classes of network dynamics () that are relevant to infectious disease epidemiology and review modelling approaches that incorporate these processes mechanistically. There are several ways in which network topology can change: the complete addition or removal of a node along with all of its edges, the addition or removal of an edge, or the rewiring of nodes by switching the destination(s) of one or more edges. While these changes occur at the scale of interaction between individuals, they may also have farther reaching impacts on larger-scale network structures and properties (such as clustering and centrality measures, or path length).
Figure 2. Classes of network dynamics. Extrinsic changes: (a) Births add new nodes and edges to a network and deaths remove them, while migration events can add and remove nodes and edges; (b) changes in social behaviour or seasonal patterns in mixing can add or remove edges, or rewire existing edges. Pathogen-mediated changes: (c) if infection confers subsequent immunity, recovered and immune individuals are removed from the epidemiologically active portion of the network; (d) when individuals withdraw from social interaction due to infection, their contacts (edges) decrease. Public health-mediated changes: (e) vaccination removes nodes and their connecting edges from the population; (f) avoidance of infected individuals may reduce contacts or replace contacts to infected nodes with contacts to susceptible nodes; (g) while school or workplace closures directly reduce contacts that would have otherwise occurred, they may inadvertently decrease social distances by causing new contacts to form elsewhere.
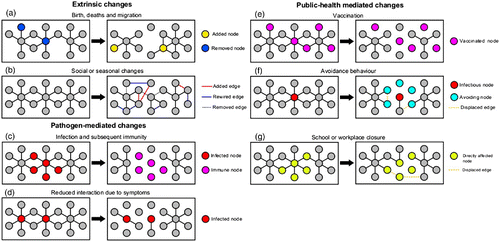
3.1. Extrinsic forces on network structure
Extrinsic processes, independent of the host-pathogen interaction, can reshape a host population. These include: (a) demographic changes, such as births and deaths that create and remove nodes and edges, respectively, or aging which can rewire a network through behavioural changes; (b) changes in social relationships which may add, remove, or rewire edges; (c) migration or host mobility, which may add or remove nodes and/or rewire edges; (d) seasonal changes in host social behaviour and contact rates, such as school sessions and holidays; (e) long-term socially or economically driven changes, such as changes that can be observed in livestock movement patterns over time Citation53 or the proliferation of air travel and subsequent changes in global transport networks. These changes may occur over a range of timescales; and their importance depends on the relative timescale of within-host infection dynamics and between-host disease spread and recurrence.
The most well-studied example of an extrinsic change driving disease dynamics is the replenishment of susceptibles by new births. Following a large disease outbreak which depletes a susceptible host population to a level where disease transmission is no longer sustained (that is, below an epidemic threshold), a subsequent outbreak may become possible only after an influx of new births. The apparently regular recurrence of childhood disease outbreaks is thought to be maintained by the constant replenishment of susceptible populations through new births (e.g. Citation15 Citation26).
Recently, infectious disease modellers have focused on the epidemiological impacts of mobility and travel patterns (particularly due to air travel). The work of Colizza et al. Citation17 and Viboud et al. Citation61 has shown that global connectivity patterns drive the diffusion of infectious diseases on large scales. Most of these models, however, do not consider dynamic changes in individual-level network connectivity.
There have been numerous studies investigating the network structures underlying the spread for sexually transmitted infectious diseases. Early work modelling STI (sexually transmitted infection) dynamics involved stochastic models which included partnership dynamics and transmission probabilities that depended on both the contact rate in a pair and the duration of partnership Citation18
Citation34. The work of Altman Citation2 extends the classical susceptible-infections-recovered (SIR) epidemic model to allow for disease transmission through a population with dynamic extended duration partnerships. This model did not allow for heterogeneous network structure, but did incorporate varying numbers of concurrent partners across the population. Eames and Keeling Citation19 have developed a pair-approximation framework to study serial monogamy in a population and includes heterogeneity in numbers of concurrent contacts. Their model is based on the novel idea of potential versus active partnerships: individuals are embedded in a fixed set of potential sexual partnerships, which may or may not be active; passive partnerships become active and occur one at a time. In addition to the variables counting numbers of individuals (e.g. [S] for susceptible individuals) and pairs of individuals (e.g. [SI] for susceptible-infected pairs) as in the standard SIS pair-approximation model Citation30, Eames and Keeling further label individuals with numbers of potential partners and the type of partnership (i.e. active or passive). So, for example, susceptible individuals with n potential partners who are in an active relationship would be tracked by . In addition, relationships activate and deactivate at heterogeneous rates α
n, m
and ρ
n, m
between individuals with n and m partners. This approach cleverly incorporates partnership formation and dissolution as well as disease transmission and recovery, but is limited by the high dimensionality of the mathematical system (which has on the order of
equations, where d
max
is the highest number of contacts for any individual in the population).
Recently, Volz and Meyers Citation62
Citation63 have developed a simple ODE framework called the neighbour exchange (NE) model to model SIR dynamics in a class of dynamic networks where individuals have a characteristic behaviour (i.e. contact number) but the identities of their contacts change over time (at a constant rate ρ). The NE model incorporates a continuous-time version of the concept of edge-rewiring Citation38
Citation65: an edge rewiring consists of removing two edges (A–B and C–D) and replacing them with the reciprocal pair of edges (A– and B–D). Altering the rate, ρ, at which random edge rewirings occur allows the model to capture different levels of mixing and to smoothly interpolate between static network models and mass-action models. Volz and Meyers Citation63 have used the model to rigorously explore the use of static versus dynamic networks, and found that a static network approach offers a reasonable approximation only for relatively static populations (low ρ). Their paper also demonstrates how one might estimate empirical contact and mixing rates from sociological data, using a sexual contact data set from a high school in Atlanta, Georgia that experienced a syphilis outbreak Citation62. They define t
ij
to be the duration of contact between individual i and individual j, and estimate a mean contact duration from the data, . The NE model assumes that contacts turnover at a constant rate, ρ, and thus that the duration of contact should be exponentially distributed with mean 1/ρ which they estimate by
. Extensions of the Volz and Meyers model might include heterogeneity in the number of concurrent contacts as well as correlations in edge rewiring.
3.2. Impacts of public health intervention on network structure
The second class of structural changes are those caused by the public or animal health interventions and control. Changes may be mediated either by individual choice or health policy and can have local and or population scale effects. Interventions such as closing schools and other public places attempts to eliminate potential contacts between individuals in a population, thereby pruning a large number of edges from the underlying contact network. Interventions such as immunization and the use of antibacterial or antiviral drugs can potentially remove immunized or treated individuals, along with all their contacts, from the chains of transmission. The structural impacts and effectiveness of such intervention strategies has been studied extensively using static network approaches Citation4 Citation6 Citation19 Citation35 Citation39 Citation40 Citation47 Citation48. For infectious respiratory diseases, Pourbohloul et al. Citation48 describe a general network-based framework for evaluating large-scale mitigation strategies. They focus on the epidemic threshold T c , which is a critical transmission probability, above which epidemics are possible and below which epidemics are highly unlikely. The value of the threshold depends on the structure of the host contact network. In general, sparser networks have higher epidemic thresholds and are thus less vulnerable to the spread of disease. The paper asserts that a primary goal of public health measures should be to raise the epidemic threshold of the population above the transmissibility of the circulating pathogen, thereby mitigating the epidemic or averting it entirely. This can be achieved through transmission-reducing interventions, such as the use of face masks, gloves, gowns, and handwashing, which reduce the probability of transmission along each edge of the network where the control is carried out but do not fundamentally alter the topology of the network; or it can be achieved through contact-reducing interventions, such as school closures, social distancing, or vaccination, that result in pruning the network through the removal of contacts (i.e. edges) for individuals participating in the intervention.
Individual-level avoidance behaviour has also been studied using epidemiological network models with edge rewiring, and has been shown to impact epidemic size and timing Citation27 Citation56 Citation66. These models mimic illness avoidance by replacing infected contacts of susceptible individuals with other randomly chosen susceptible individuals. Edge rewiring is not done completely randomly as in the NE model, but instead depends on the changing epidemiological status of nodes in the network. Using a pair-approximation framework, Gross et al. Citation27 show that such adaptive rewiring leads to modular network structure (consisting of highly intraconnected but only loosely interconnected sub-networks), a wide degree distribution and degree assortavity (a positive correlation in degree between pairs of linked nodes). This reveals two means by which avoidance behaviour can counterintuitively exacerbate epidemics: degree correlations can decrease the effectiveness of targeted vaccination; and high intraconnectivity of the susceptible cluster enables the persistence of epidemics even below the epidemic threshold Citation27. However, Shaw and Schwartz Citation56 show that infections are reduced due to self-isolation, and that for an appropriate choice of parameters, bistability between the disease-free equilibrium and endemic state can be observed, in contrast to SIS dynamics in static networks where either a single attracting endemic or disease-free state exists. In another model Citation66, susceptible-infective contacts are broken (rather than rewired) and later reconnected at random. This also leads to bistability and other dynamics not observed in static network models. While these models are quite interesting, it is not yet clear if and how they pertain to real-world behavioural responses to ongoing epidemics.
3.3. Pathogen-mediated changes in network structure
While extrinsic processes and public health interventions can alter the contact network over which a pathogen spreads, and can have significant impact on disease consequences, pathogen spread itself can also alter the host contact structure. We differentiate pathogen-mediated changes from interventions such as avoidance behaviour (which are clearly also driven by the disease spread) by defining them in terms of change occurring at infected nodes and their edges. This focus on infected rather than susceptibles proves to have a crucially different impact on network topology (). Immunizing pathogens such as measles and influenza in human beings or foot and mouth disease virus in livestock confer resistance to reinfection by the same pathogen, making recovered individuals irrelevant in future chains of transmission. In the simplest case of a naive population and a fully immunizing pathogen, disease outbreaks restructure the epidemiologically active portion of their host population by effectively removing infected individuals (the node and all connecting edges) from the network. This particular change in network topology is mediated by pathogen spread and is preferentially removes highly connected individuals in the network Citation3 Citation10 Citation23 Citation45. This and more complex structural change due to naturally acquired immunity can be studied by calculating the structures of the residual networks made up of remaining susceptible individuals and the contacts between them Citation3 Citation23 Citation49. Ferrari et al. Citation23 characterized the structural evolution of a network due to an epidemic in terms of frailty (the degree to which highly connected individuals are more vulnerable to infection) and interference (the extent to which the epidemic cuts off connectivity among the susceptible population that remains following an epidemic). Specifically, at the end of an epidemic, frailty is given by the scaled difference between the mean degree of remaining susceptible nodes and the mean degree of the original network; and interference is the scaled difference in the mean degree of remaining susceptible nodes and the average number of connections among the remaining susceptible nodes. The evolution of the susceptible network over the course of an epidemic differs among the classes of networks; frailty, relative to interference, accounts for an increasing component of network evolution on networks with greater variance in contacts.
Figure 3. The topological impact of various dynamic processes in an urban contact network. We measure structural impact in terms of node degree (i.e. number of edges) (left panels) and clustering coefficient (i.e. the propensity of triangles, measured as the fraction of triples for a node that are closed) (right panels) across the network. In the top panels, we compare random vaccination and school closure. In the bottom panels, we compare infection-acquired immunity (a pathogen-mediated change) to avoidance-behaviour (a public-health measure).
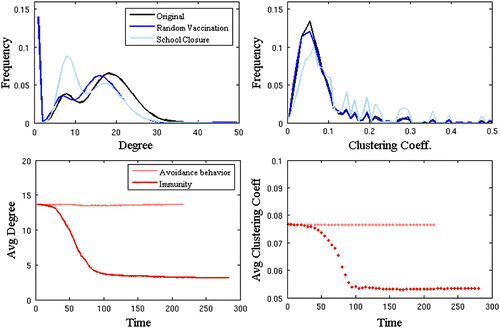
An epidemic can profoundly reshape a contact network, and thereby impact the potential for future disease emergence as well as the disease dynamics and the viability of various intervention strategies should future outbreaks occur Citation3 Citation44. A recent analysis of historical data from twentieth century influenza pandemics demonstrated that infection-acquired immunity can indeed lead to a significant structural change in the host contact structure and shift the burden of disease to different portions of the population Citation6. In the longer term, repeated epidemics and demographic processes as well as adaptation of the pathogen may lead to the co-evolution of pathogen and host network structure Citation3 Citation49 Citation50.
Pathogens may also restructure their host contact network by mediating changes in host behaviour. A well-known example is that of rabies causing infected hosts to be aggressive and more likely to bite (thus increasing chances of transmission). In contrast, the common cold is thought to cause infected hosts to refrain ‘voluntarily’ from social interaction (thus decreasing chances of transmission). Although alterations of host behaviour have been widely studied from a disease ecology perspective, little data exist about the individual-level impact of this change on network structure. It is unknown, for example, if change in contact behaviour due to disease symptoms leads to a reduction in contacts or a rewiring of contacts, or both. A better understanding of such behaviours and of their impacts on network structure might allow us to design more effective public health measures.
3.4. Topological impact of changes
Each of the dynamical processes described above causes one or more topological changes to the underlying: node additions/deletions, edge addition/deletions, or edge rewirings. The magnitude and nature of the changes, however, can significantly impact large-scale topological features of the network (and thereby influence disease dynamics). In , we consider a few of these perturbations using a synthetic respiratory-disease contact network based on data from Vancouver, Canada Citation40. We measure structural impact in terms of the distribution of node degrees (i.e. number of edges) and clustering coefficients (i.e. the propensity of triangles) across the network. In the top panel, we consider the effect of random vaccination versus school closure as a targeted contact-reducing intervention. While random vaccination makes the degree of all vaccinated nodes zero and shifts the entire degree distribution to the left, it has only a small impact on the clustering coefficient distribution. School closure, on the other hand, affects the network structure through high-degree individuals such as children and school staff Citation4 and thus dramatically alters the degree distribution and shifts the clustering coefficient distribution slightly to the right: high contact individuals lose contacts and switch into lower degree classes and existing contacts appear relatively more clustered due to the reduction in edges. In the lower panel of , we compare two processes driven by disease spread, avoidance behaviour, and infection-acquired immunity. The network change centred at susceptible nodes (avoidance behaviour) has little effect on network topology, while the one focused on infected nodes (infection-acquired immunity) drastically breaks down network connectivity, reducing both average degree and clustering.
Although provides some insight into the topological signatures left behind by each of these dynamic processes, we stress that the epidemiological consequences of each process is less obvious. Results showed in Citation23, for example, demonstrate that infection-acquired immunity due to a prior epidemic results in greater population protection in a heterogeneously structured population, while random vaccination leads to smaller epidemics in highly structured small-world populations. While much of the discussion of network epidemiology has focused on connectivity as measured by degree, these results suggest that higher order structural properties, such as clustering, can have important implications for the epidemiological evolution and robustness of populations.
4. Discussion
The field of network epidemiology has matured in the last decade; and dynamic network modelling stands as a major challenge for both data gatherers and modellers going forward. On the data collection side, there are very few studies that provide data on contact patterns relevant to the spread of human infectious disease Citation12 Citation13 Citation20 Citation41 Citation46 Citation51 Citation64. Collecting detailed and longitudinal contact data sufficient to construct a dynamic network represents a significantly greater challenge Citation29 Citation51. A unique example of a data set sufficient for estimating dynamic network structure includes extensive records of livestock movement among agricultural premises throughout the UK and other European nations Citation7 Citation14 Citation32 Citation42. This has resulted from a large-scale effort to trace livestock movements for the purposes of disease surveillance and control, and has been made possible by a technological passport system for livestock. Similarly rich data sets may emerge from the development and use of human tracking devices (e.g. cell phones, GPS, RFID, etc.) Citation9 Citation24. These technologies provide population scale data from which dynamic interaction patterns can be inferred, and the use of such data for epidemiological modelling may be very informative if disease-causing contacts are inferred appropriately from the proximity data.
As data revealing to dynamic contact patterns are slowly becoming available, modellers need to improve the analytical and computational toolkit with which we study disease dynamics in complex populations. Recent analytic models of infectious diseases spreading through particular classes of dynamic networks (e.g. Citation11 Citation52 Citation62) provide important initial steps in this direction, but we still lack a mathematical framework that tractably handles a broad range of realistic dynamic networks. While some have argued that agent-based simulations may reduce the need for analytical models of this kind Citation21, agent-based approaches face significant algorithmic and computational problems with respect to network representation, measurement of topological features, and dynamical models for pathogen spread. Advances in data-driven analytic modelling would avert some of these challenges while providing a general understanding of the relationship between contact dynamics and disease spread.
Through collection and analysis of better data, and analytic modelling of disease dynamics in dynamic networks, the field can address two relevant questions: (1) how does network topology evolve in response to various intrinsic and extrinsic processes? and (2) how do these topological changes influence the spread and evolution of infectious disease pathogens? In addition, such work will allow us to identify situations in which the dynamic nature of a contact network will matter and thus should be explicitly estimated, modelled, and/or targeted by public health measures.
Acknowledgements
The authors acknowledge support from the RAPIDD program of the Science & Technology Directorate, Department of Homeland Security, and the Fogarty International Center, National Institutes of Health to S.B.; from the Fogarty International Center, US National Institutes of Health (R01 TW008246) and from the UK Economic and Social Research Council and Medical Research Council (RES-355-25-0019) to J.R.; from the Canadian Institutes of Health Research (PTL97125 and PAP93425) and the Michael Smith Foundation for Health Research to B.P.; and from the National Science Foundation (DEB-0749097) to L.M.
References
- Aizen , J. , Huttenlocher , D. , Kleinberg , J. and Novak , A. 2004 . Traffic-based feedback on teh web . 101 : 5254 – 5260 . PNAS
- Altman , M. 1995 . Susceptible-infection-removed epidemic models with dynamic partnerships . J. Math. Biol. , 33 ( 6 ) : 661 – 675 .
- S. Bansal and L.A Meyers, The impact of past epidemics on future disease dynamics, arxiv.org 0910.2008, (2009)
- Bansal , S. , Pourbohloul , B. and Meyers , L. A. 2006 . Comparative analysis of influenza vaccination programs . PLoS Med. , 3 : e387
- Bansal , S. , Grenfell , B. and Meyers , L. A. 2007 . When individual behaviour matters: homogeneous and network models in epidemiology . J.R. Soc. Interface , 4 ( 16 ) : 879 – 891 .
- Bansal , S. , Pourbohloul , B. , Hupert , N. , Grenfell , B. and Meyers , L. A. 2010 . The shifting demographic landscape of pandemic influenza . PLoS One , 5 ( 2 ) : 9360
- Baptista , F. M. , Nunes , T. , Almeida , V. and Louza , A. 2008 . Cattle movements in Portugal: An insight into the potential use of network analysis . 103 : 35 – 40 . RPCV
- Barabasi , A. L. and Albert , R. 1999 . Emergence of scaling in random networks . Science , 286 : 509 – 512 .
- A. Barrat, C. Cuttuto, V. Colizza, J. Pinton, W. Van den Broeck, and A. Vespignani, High resolution dynamical mapping of social interactions with active RFID, arxiv.org (2008), 0811.417v2
- Barthelemy , M. , Barrat , A. , Pastor-Satorras , R. and Vespignani , A. 2004 . Velocity and hierarchical spread of epidemic outbreaks in scale-free networks . Phys. Rev. Lett. , 92 ( 17 ) : 178701
- Bauch , C. T. 2002 . A versatile ode approximation to a network model for the spread of sexually transmitted diseases . J. Math. Biol. , 45 : 375 – 395 .
- Bearman , P. S. , Moody , J. and Stovel , K. 2004 . Chains of affection . Am. J. Sociol. , 110 : 44 – 91 .
- Beutels , P. , Shkedy , Z. , Aerts , M. and Van Damme , P. 2006 . Social mixing pattern of transmission models of close contact infections . Epidemiol. Infect. , 134 : 1158 – 1166 .
- Bigras-Poulin , M. , Thompson , R. A. , Chriel , M. , Mortensen , S. and Greiner , M. 2006 . Network analysis of Danish cattle industry trade patterns as an evaluation of risk potential for disease spread . Prev. Vet. Med. , 76 : 11 – 39 .
- Broutin , H. , Viboud , C. , Grenfell , B. T. , Miller , M. A. and Rohani , P. 2010 . Impact of vaccination and birth rate on the epidemiology of pertussis: A comparative study in 64 countries . Proc. Biol. Sci. , doi:10.1098/rspb.2010.0994
- Christley , R. M. , Robinson , S. E. , Lysons , R. and French , N. P. Network analysis of cattle movements in Great Britain . Proceedings of a Meeting Held at Nairn, Inverness . Scotland. pp. 234 – 44 .
- Colizza , V. , Barrat , A. , Barthelemy , M. and Vespignani , A. 2005 . The role of the airline transportation network in the prediction and predictability of global epidemics . 103 ( 7 ) : 2105 – 2120 . PNAS
- Dietz , K. and Hadeler , J. P. 1988 . Epidemiological models for sexually transmitted diseases . J. Math. Biol. , 26 : 1 – 25 .
- Eames , K. T.D. and Keeling , M. J. 2002 . Modeling dynamic and network heterogeneities in the spread of sexually transmitted diseases , 99 : 13330 – 13335 . PNAS
- Edmunds , W. J. , O'Callaghan , C. J. and Nokes , D. J. 1997 . Who mixes with whom? A method to determine the contact patterns of adults that may lead to the spread of airborne infection . Proc. Biol. Sci. , 264 : 949 – 957 .
- Epstein , J. 2009 . Modelling to contain pandemics . Nature , 460 ( 7256 ) : 687
- Fefferman , F. and Ng , K. L. 2007 . How disease models in static networks can fail to approximate disease in dynamic networks . Phys. Rev. E , 76 ( 3 ) : 031919
- Ferrari , M. , Bansal , S. , Meyers , L. A. and Bjornstad , O. 2006 . Network frailty and the geometry of herd immunity . Proc. Biol. Sci. Lond. B , 273 : 2743 – 2748 .
- Gonzalez , M. C. , Hidalgo , C. A. and Barabasi , A. L. 2008 . Understanding individual human mobility patterns . Nature , 453 : 779 – 782 .
- Green , D. , Kao , R. , Johnson , J. and Kiss , I. Z. 2007 . Disease dynamics over very different time-scales: Foot-and-mouth disease and scrapie on the network of livestock movements in the UK . J. R. Soc. Interface , 4 : 907 – 916 .
- Grenfell , B. T. , Bjornstad , O. N. and Finkenstadt , B. 2002 . Dynamics of measles epidemics: Scaling noise, determinism and predictability with the TSIR model . Ecol. Monogr. , 72 : 185 – 202 .
- Gross , T. , Dommar D'Lima , C. J. and Blasius , B. 2006 . Epidemic dynamics on an adaptive network . Phys. Rev. Lett. , 96 ( 20 ) : 208701
- Heath , M. F. , Vernon , M. C. and Webb , C. R. 2008 . Construction of networks with intrinsic temporal structure from UK cattle movement data . BMC Vet. Res. , 4 ( 11 )
- Kao , R. , Danon , L. , Green , D. and Kiss , I. Z. 2006 . Demographic structure and pathogen dynamics on the network of livestock movements in Great Britain . Proc. Biol. Sci. , 273 : 1999 – 2007 .
- Keeling , M. J. 1999 . The effects of local spatial structure on epidemiological invasions . Proc. R. Soc. B , 266 : 859 – 867 .
- Keeling , M. J. and Eames , K. T.D. 2005 . Networks and epidemic models . J. R. Soc. Interface , 2 : 295 – 307 .
- Kiss , I. , Green , D. M. and Kao , R. R. 2006 . The network of sheep movements within Great Britain: Network properties and their implications for infectious disease spread . J. R. Soc. Interface , 3 : 669 – 677 .
- Kossinets , G. and Watts , D. J. 2006 . Empirical analysis of an evolving social network . Science , 311 : 88 – 90 .
- Kretzschmar , M. 1995 . Deterministic and stochastic pair formation models for the spread of sexually transmitted diseases . J. Biol. Syst. , 3 : 789 – 901 .
- Kretzschmar , M. 2004 . Sexual network structure and sexually transmitted disease prevention: A modeling perspective . Sex. Transm. Dis. , 27 ( 10 ) : 627 – 635 .
- Leskovec , J. , Kleinberg , J. and Faloutsos , C. Graphs over time: Densification laws, shrinking diameters and possible explanations . Proc 11th ACM Chicago . IL. pp. 177 – 187 . SIGKDD
- Lurie , M. N. and Rosenthal , S. 2010 . Concurrent partnerships as a driver of the HIV epidemic in Sub-Saharan Africa? The evidence is limited . AIDS Behav. , 14 : 17 – 24 .
- Maslov , S. and Sneppen , K. 2002 . Specificity and stability in topology of protein networks . Science , 296 ( 5569 ) : 910 – 913 .
- Meyers , L. A. , Newman , M. E.J. , Martin , M. and Schrag , S. 2003 . Applying network theory to epidemics: Control measures for Mycoplasma pneumoniae outbreaks . Emerg. Infect. Dis. , 9 ( 2 ) : 204 – 210 .
- Meyers , L. A. , Pourbohloul , B. , Newman , M. E.J. , Skowronski , D. M. and Brunham , R. C. 2005 . Network theory and SARS: Predicting outbreak diversity . J. Theor. Biol. , 232 : 71 – 81 .
- Mossong , J. , Hens , N. , Jit , M. , Beutels , P. , Auranen , K. , Mikolajczyk , R. , Massari , M. , Salmaso , S. , Tomba , G. S. Wallinga , J. 2008 . Social contacts and mixing patterns relevant to the spread of infectious diseases . PLoS Med. , 5 ( 3 ) : e74
- Natale , F. , Giovannini , A. , Savini , L. , Palma , D. , Possenti , L. , Fiore , G. and Calistri , P. 2009 . Network analysis of Italian cattle trade patterns and evaluation of risks for potential disease spread . Prev. Vet. Med. , 92 : 341 – 350 .
- Newman , M. E.J. 2002 . Spread of epidemic disease on networks . Phys. Rev. E. , 66 ( 1 ) : 016128
- Newman , M. E.J. 2004 . Threshold effects for two pathogens spreading on a network . Phys. Rev. Lett. , 95 ( 10 ) : 108701
- Noel , P.-A. , Davoudi , B. , Brunham , R. C. , Dube , L. J. and Pourbohloul , B. 2009 . Time evolution of epidemic disease on finite and infinite networks . Phys. Rev. E. , 79 ( 2 ) : 026101
- Potterat , J. J. , Phillips-Plummer , L. , Muth , S. Q. , Rothenberg , R. B. , Woodhouse , D. E. , Maldonado-Long , T. S. , Zimmerman , H. P. and Muth , J. B. 2002 . Risk network structure in the early epidemic phase of HIV transmission in Colorado springs . Sex. Transm. Infect. , 78 : 159 – 163 .
- Pourbohloul , B. and Brunham , R. 2004 . Network models and transmission of sexually transmitted diseases . Sex. Transm. Dis. , 31 ( 6 ) : 388 – 390 .
- Pourbohloul , B. , Meyers , L. A. , Skowronski , D. M. , Krajden , M. and Patrick , D. M. 2005 . Modeling control strategies of respiratory pathogens . Emerg. Infect. Dis. , 11 : 1249 – 1256 .
- Read , J. M. and Keeling , M. J. 2003 . Disease evolution on networks: The role of contact structure . Proc. Biol. Sci. , 270 ( 1516 ) : 699 – 708 .
- Read , J. M. and Keeling , M. J. 2006 . Disease evolution across a range of spatio-temporal scales . J. Popul. Biol. , 70 : 201 – 213 .
- Read , J. M. , Eames , K. T.D. and Edmunds , W. J. 2008 . Dynamic social networks and the implications for the spread of infectious disease . J. R. Soc. Interface , 5 ( 26 ) : 1001 – 1007 .
- S. Risau-Gusman, Influence of network dynamics on the spread of sexually transmitted diseases, arxiv.org (2010) 1378
- Robinson , S. E. , Everett , M. G. and Christley , R. M. 2007 . Recent network evolution increases the potential for large epidemics in the British cattle population . J. R. Soc. Interface , 4 : 669 – 674 .
- Rothenberg , R. and Muth , S. Q. 2007 . Large-network concepts and small-network characteristics: Fixed and variable factors . Sex. Transm. Dis. , 34 ( 8 ) : 604 – 612 .
- Sarkar , P. and Moore , A. W. 2005 . Dynamic social network analysis using latent space models . 7 ( 2 ) : 31 – 40 . SIGKDD Explorations: Special Edition on Link Mining SIGKDD Explor. Newsl
- Shaw , L. and Schwartz , I. 2008 . Fluctuating epidemics on adaptive networks . Phys. Rev. E , 6 ( 2 ) : 066101 77
- Skyrms , B. and Pemantle , R. 2000 . A dynamic model of social network formation . 97 : 9340 – 9346 . PNAS
- Smieszek , T. , Fiebig , L. and Scholz , R. W. 2009 . Models of epidemics: When contact repetition and clustering should be included . Theor. Biol. Med. Model. , 6 ( 11 )
- Snijders , T. A.B. 2005 . “ Models for longitudinal network data, in Models and Methods in Social Network Analysis ” . Edited by: Peter , J. , Carrington , John , Scott and Stanley , Wasserman . New York : Cambridge University Press .
- Vernon , M. and Keeling , M. J. 2009 . Representing the UK cattle herd as static and dynamic networks . Proc. Biol. Sci. , 276 : 469 – 476 .
- Viboud , C. , Bjornstad , O. N. , Smith , D. , Simonsen , L. , Miller , M. A. and Grenfell , B. T. 2006 . Synchrony, waves, and spatial hierarchies in the spread of influenza . Science , 312 : 447 – 451 .
- Volz , E. and Meyers , L. A. 2007 . Susceptible-infected-recovered epidemics in dynamic contact networks . Proc. R. Soc. B , 274 : 2925 – 2933 .
- Volz , E. and Meyers , L. A. 2008 . Epidemic thresholds in dynamic contact networks . J. R. Soc. Interface , 6 : 233 – 241 .
- Wallinga , J. , Edmunds , W. J. and Kretzschmar , M. 1999 . Perspective: Human contact patterns and the spread of airborne infectious diseases . Trends Microbiol. , 7 : 372 – 377 .
- Watts , D. and Strogatz , S. H. 1998 . Collective dynamics of small world networks . Nature , 393 ( 6684 ) : 440 – 442 .
- Zanette , D. and Risau-Gusman , S. 2008 . Infection spreading in a population with evolving contacts . J. Biol. Phys. , 34 ( 1–2 ) : 135 – 148 .