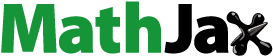
ABSTRACT
For antibody-drug conjugates (ADCs) that carry a cytotoxic drug, doses that can be administered in preclinical studies are typically limited by tolerability, leading to a narrow dose range that can be tested. For molecules with non-linear pharmacokinetics (PK), this limited dose range may be insufficient to fully characterize the PK of the ADC and limits translation to humans. Mathematical PK models are frequently used for molecule selection during preclinical drug development and for translational predictions to guide clinical study design. Here, we present a practical approach that uses limited PK and receptor occupancy (RO) data of the corresponding unconjugated antibody to predict ADC PK when conjugation does not alter the non-specific clearance or the antibody-target interaction. We used a 2-compartment model incorporating non-specific and specific (target mediated) clearances, where the latter is a function of RO, to describe the PK of anti-CD33 ADC with dose-limiting neutropenia in cynomolgus monkeys. We tested our model by comparing PK predictions based on the unconjugated antibody to observed ADC PK data that was not utilized for model development. Prospective prediction of human PK was performed by incorporating in vitro binding affinity differences between species for varying levels of CD33 target expression. Additionally, this approach was used to predict human PK of other previously tested anti-CD33 molecules with published clinical data. The findings showed that, for a cytotoxic ADC with non-linear PK and limited preclinical PK data, incorporating RO in the PK model and using data from the corresponding unconjugated antibody at higher doses allowed the identification of parameters to characterize monkey PK and enabled human PK predictions.
Introduction
The promise of antibody-drug conjugates (ADCs) lies in their ability to efficiently deliver their payload (generally a cytotoxic drug) to tumor cells while minimizing delivery to non-target sites. ADCs are expected to enhance the anti-tumor activity of monoclonal antibodies and widen the therapeutic index (i.e., ratio of doses or exposures at the maximum tolerated dose versus the efficacious dose) of the cytotoxic drugs. During the development of ADCs, mathematical pharmacokinetic (PK) models are used to capture the relationship between dose and exposure and to inform cross-species translation. In the preclinical space, PK models can be used to estimate the therapeutic index of various drug candidates and support candidate selection. When entering the clinic, predictions of human PK (typically based on preclinical observations) may play a critical role in the selection of early stage clinical doses that must balance safety of patients while minimizing the number of subjects who receive sub-therapeutic treatments.
As clinical experience with monoclonal antibodies has grown over the past few decades, characterization of their PK properties in non-human primates and subsequent translation to humans has been fairly well studied for antibodies that are predominantly cleared by non-specific mechanisms.Citation1-Citation6 In the case of antibodies that demonstrate significant target-mediated drug disposition (TMDD), PK characterization in non-human primates requires antibody concentration-time profiles over a wide concentration range to capture saturation of target-mediated clearance that frequently manifests in PK non-linearities. This information can only be obtained if the test article is well tolerated in preclinical in vivo studies over a wide dose range. Even when this is possible, a study with multiple groups is required to evaluate different dose levels, resulting in a higher animal usage than that needed to characterize an antibody with linear PK. To predict human PK of antibodies that undergo TMDD, species differences of target expression levels and turnover as well as antibody interaction with target need to be considered. Scale up of the target-dependent component of antibody PK has been tackled with varying degrees of success using TMDDCitation 7 , Citation 8 and Michaelis-Menten (MM)Citation 9 non-linear PK models. In both cases, appropriate scale up of the parameters that describe the PK non-linearity is critical to capture the differences across species. For ADCs carrying cytotoxic drugs, preclinically evaluable doses are restricted by tolerability, limiting concentration-time measurements to a range that may not be sufficient for robust characterization of the PK non-linearity. To date, various PK modeling efforts that support the drug development have been focused on complexities unique to ADCs, such as capturing the PK driven by the de-conjugation processesCitation10-Citation12 or integrating the complex processes occurring at cellular, tissue and systemic levels using multi-scale models.Citation13-Citation15 For newer generation ADCs, advances in conjugation technologies have reduced the rates of payload loss by improving linker stability compared to the first-generation ADCs.Citation16-Citation19 Moreover, understanding the effect of the site of conjugation, drug loading, and drug-linker design on ADC PK has enabled mitigation of accelerated clearance observed with some ADCs.Citation20-Citation22 Mechanistic PK/pharmacodynamic (PD)Citation23-Citation26 and multi-scale modelsCitation13-Citation15 have been proposed to improve translatability from preclinical species to the clinic. However, implementation and calibration of these multi-scale models require a substantial amount of in vitro and in vivo data that may not be available when human PK predictions are first required, which is typically during early stages of drug development. Here, we present a practical approach to predict ADC PK using limited PK and receptor occupancy (RO) data of the corresponding unconjugated antibody under conditions when conjugation does not alter the antibody-target interaction or the non-specific clearance of the antibody.
An anti-CD33 ADC with dose-limiting neutropenia in monkeys was used as a case study to illustrate our approach. CD33, a glycoprotein expressed on most myeloid leukemia cells as well as on normal myeloid and monocytic precursors, has been pursued clinically as a target for drugs intended as treatments for acute myeloid leukemia (AML).Citation 27 A non-linear 2-compartment model incorporating non-specific and specific (target-mediated) clearances, where the latter is a function of RO, was used to describe the PK. We tested our model by comparing PK predictions based on the unconjugated antibody (referred to here as anti-CD33 mAb) to observed conjugated antibody (referred to here as anti-CD33 ADC) PK data that was not utilized for model development, and subsequently translated the model to predict human PK. Additionally, we used this approach to compare model predictions for other previously tested anti-CD33 molecules with published clinical data.
Results
CD33 expression levels in cynomolgus monkey and human cells
Evaluation of CD33 expression in cynomolgus monkey and human cells showed the expected myeloid-specific expression pattern for CD33.Citation 28 Similar levels of CD33 were observed for both human and cynomolgus monkey mature myeloid cells (monocytes / granulocytes) (). It is worth noting that the slightly lower levels of CD33 on monocytes of cynomolgus monkey compared to human might be attributed to the small number of animals (n = 2) evaluated by flow cytometry, as there was a wide range of CD33 expression for human monocytes (n = 6). Furthermore, the levels of CD33 on cynomolgus CD34+ hematopoietic progenitor cells were equivalent to human, which further supports this as a suitable preclinical model for evaluating CD33 targeting therapeutics.
Cell binding of anti-CD33-mAb and anti-CD33 ADC to monkey and human CD33
Anti-CD33 mAbs bound to human embryonic kidney (HEK) 293 cells over-expressing recombinant human- or cynomolgus-CD33 within 2-fold as determined by fluorescence-activated cell sorting (FACS) with EC50s of 0.60 µg/ml (human CD33) and 0.35 µg/ml (cynomolgus CD33) (). Additionally, a competitive binding assay with Scatchard analysis using the HEK 293 stable cell lines was conducted confirming that the affinity of the anti-CD33 mAb for human and cynomolgus CD33 are comparable (0.71 nM and 0.32 nM, respectively, ). Together, both analyses suggest that the binding of the anti-CD33 mAb is similar for human and cynomolgus CD33. The binding affinity of anti-CD33 ADC was not measured in this study, and it is assumed to be similar to the unconjugated anti-CD33 mAb based on our previous experience with other antibodies targeting tumor antigens conjugated at position LC K149C on the antibody.Citation 29
Table 1. Binding affinities of anti-CD33 mAb to human and cynomolgus monkey CD33 positive cells.
Figure 2. Characterization of anti-CD33 mAb binding to stable 293 HEK cells overexpressing recombinant human (red) and cynomolgus monkey (black) CD33. Incubations performed on ice for 30 min followed by detection with a goat-anti-human IgG-PE secondary reagent. Approximate EC50 for human and monkey are 0.60 µg/ml and 0.35 µg/ml, respectively.

Anti-CD33 mAb and anti-CD33-ADC PK in monkey studies
Serum anti-CD33 mAb concentrations were measured following single dose intravenous (IV) administration of 0.5 and 15 mg/kg in the monkey PKPD study. Dosing solution concentrations were measured as 64% and 86% of their nominal value for the low and high dose groups, respectively. Therefore, actual measured doses were used in the PK and modeling analysis. Anti-CD33 mAb exhibited non-linear PK in this dose range with overall clearances ranging from 15.5 ± 1.34 mL/day/kg at the low-dose (0.5 mg/kg) to 8.63 ± 2.39 mL/day/kg at the high-dose (15 mg/kg). Serum concentration-time profiles in the monkey PKPD study are shown in . Non-compartmental PK parameters are reported in . The maximum concentration (Cmax) increased roughly dose proportionally from 11.5 ± 0.658 µg/mL at 0.5 mg/kg to 272 ± 13.4 µg/mL at 15 mg/kg. Positive anti-drug antibody (ADA) responses were detected in 5 of 6 animals (83%). ADA did not appear to have a significant effect on the total antibody (TAB) concentrations. TAB concentration from all animals was used in the PK evaluation and modeling analysis. Concentrations below the lower limit of quantification (LLOQ) of the assay were treated as missing.
Table 2. Mean and standard deviation (SD) non-compartmental plasma PK parameters following administration of a 0.5 and 15 mg/kg IV bolus of anti-CD33 mAb to cynomolgus monkeys.
Figure 3. (a) Anti-CD33 mAb serum concentration following administration of a 0.5 mg/kg (in green) and 15 mg/kg (in blue) IV bolus of anti-CD33 mAb to cynomolgus monkeys. Symbols and bars represent mean and standard deviation, n = 3 unless indicated otherwise; missing values were below of the LLOQ of the assay (7.8 ng/mL). Solid lines correspond model fitted serum TAB curve using both PK and RO data sets. (b) Receptor availability/occupancy in granulocytes and monocytes following administration of a 0.5 mg/kg (in green) and 15 mg/kg (in blue) IV bolus of anti-CD33 mAb to cynomolgus monkeys. Symbols and bars represent mean and standard deviation (n = 3). Solid lines correspond fitted RO curve using both PK and RO data sets.
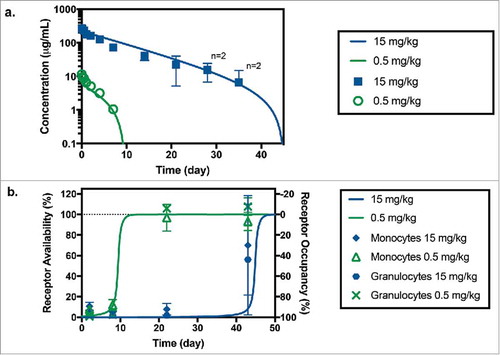
Limited serum toxicokinetic samples were taken during the toxicology study following the administration of 0.1, 0.2, 0.4 and 1 mg/kg of anti-CD33-ADC to measure TAB levels. All measured dosing solutions concentrations were within ±10% of their nominal values; therefore, nominal doses were used for PK and modeling analysis. Doses of 0.4 or 1 mg/kg were not well tolerated and resulted in mortalities for all animals in those groups. Anti-CD33-ADC concentrations measured using TAB assay are shown in . Missing symbols at sample time points (10 minutes; 1, 7, 10, 12 and 14 days post-dose) indicate that concentrations fell below the LLOQ (1 µg/mL). Minimal deconjugation was confirmed by affinity capture measurements (data not shown). These results are similar to those reported in previous publications,Citation 34 which showed that the average drug-to-antibody-ratio declined roughly 10% over the complete duration of the study and support the use of TAB concentrations for PK characterization of the anti-CD33 ADC. The TAB concentrations of anti-CD33 ADC observed in this toxicology study were consistent with PK observations in the monkey PKPD study. Non-compartmental PK parameters could not be estimated due to the limited number of samples with measurable concentrations. Detectable post-baseline ADA responses were observed in 7 of 8 animals (88%) with post-baseline evaluable samples. One animal in the group receiving 0.4 mg/kg of anti-CD33 ADC only had baseline collections. ADA did not appear to have a significant impact on TAB concentrations. All samples with detectable TAB concentrations were included in the comparison to model predictions.
Figure 4. (a) Predicted and measured concentrations of TAB after administration of 0.1 mg/kg (n = 4), 0.2 mg/kg (n = 4), 0.4 mg/kg (n = 1) and 1 mg/kg (n = 1) of anti-CD33 ADC in toxicology study in cynomolgus monkey (LLOQ = 1 µg/mL). (b) Corresponding model predicted receptor availability/occupancy for the same study.
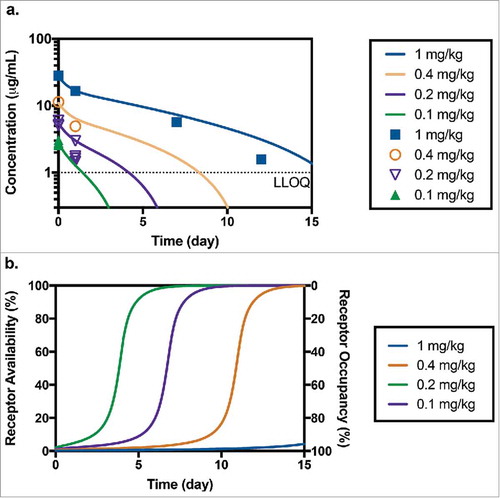
CD33 receptor occupancy in monkey PKPD study
RO was measured in the monkey PKPD study after the administration of anti-CD33-mAb. The results showed rapid saturation of CD33 on both monocytes and granulocytes beginning as early as one day following administration of anti-CD33 mAb (). Higher doses of anti-CD33-mAb resulted in more prolonged RO as observed by the increased binding by the test-article-competing CD33 antibody. The RO for both monocytes and granulocytes was similar.
PK-RO model development based on monkey PKPD study with anti-CD33 mAb
Modeling based on PK and RO data
The serum TAB concentration and RO data following the administration of 0.5 mg/kg and 15 mg/kg of unconjugated anti-CD33 antibody in the monkey PKPD study and shown in were used to calibrate the model described in equations 1-3. Both PK and RO data were well described by the proposed model. Estimated model parameters with their standard error values can be found in . Values for the non-specific clearance (CL = 4.92 mL/day/kg) and volume of central compartment (V1 = 36.7 mL/kg) are comparable to the values historically observed for other human IgG1 antibodies in monkeys.Citation 1 The value of intrinsic clearance (CLINT), defined as the ratio Vmax/KM,Citation 35 was 372 mL/kg/day. The target-mediated clearance for low concentrations (i.e., when C1<<KM) is thus roughly 75-fold the non-specific clearance (CL). The estimated KM value (0.0602 µg/mL ∼ 0.40 nM) was comparable to the affinity value of the anti-CD33 ADC for the monkey CD33 receptor (KD ∼ 0.32 nM), suggesting that the target uptake rate is primarily determined by binding and that internalization and antibody degradation rates do not represent rate-limiting steps in the overall observed target-mediated clearance. Significant values of standard error respect to the estimated value suggest a high degree of uncertainty, especially in those characterizing the PK non-linearity, Vmax and KM.
Table 3. Model parameters for the presented PK-RO model. The model was fitted to cynomolgus monkey data using both PK and RO data sets. Scaled-up parameters used for prediction for humans are also shown.
Modeling utilizing only PK data
To evaluate the significance of including RO data in the modeling approach, calibration of the model was attempted using only PK data; however, in this case, the model parameters were not identifiable, most likely due to the limited number of dose levels tested in the study. Alternatively, when the PK is not sufficient to identify all parameters, some of these may be fixed if an estimation of their values (or their potential range) is available. For, example, based on the TMDD model,Citation 36 we expect the value of KM to be larger or equal to KD (i.e., the antibody concentration that results in an elimination rate equal to 50% of Vmax must be at least equal to the concentration required to bind 50% of the target when antibody is in excess). Based on this, the value of KD can be used to provide a lower bound for the range of possible values of KM. For illustration, we fixed the value of KM to 1, 10, or 100 times the value of KD. Resulting parameter fits are shown in Table S1 (Supplementary material). The resulting values of non-specific clearance (5.9 – 6 mL/kg/day) seem to be independent of the value of KM used and are comparable to the value obtained using PK and RO data (4.92 mL/kg/day), indicating that at high doses all model fits result in a comparable prediction of TAB PK. However, the estimated values of intrinsic clearance differ significantly (164, 18.6 and 3.2 mL/kg/day, for KM equal to 1, 10, 100 times KD, respectively, versus 372 mL/kg/day using PK and RO data), indicating that at lower doses, PK predictions for each of these model fits will be significantly different. More importantly, for all model fits using PK only, the standard error of the Vmax estimation is very high (resulting in large standard errors with respect to the estimated parameter values, see Table S1), indicating a high degree of uncertainty in the parameter estimates.
Using PK-RO model to predict ADC PK in the monkey toxicology study
Assuming that both unconjugated and conjugated antibody interact with the CD33 receptor in a similar manner, we can use our PK-RO model (developed based on anti-CD33 mAb data from the PKPD study) to predict the corresponding ADC PK in the toxicology study. This assumption is supported by our prior experience with ADC constructs where conjugation of different payloads at the same position (K149C) in the antibody did not appear to affect this interaction.Citation 30 Note here that no ADC data were used in model development. As observed in , the PKPD model of the unconjugated antibody does a fair job describing this limited PK dataset of the conjugate, and the deviations are within range of typical variability in concentration (see for example ). For the two lowest dose groups, the model predicts the concentration to fall below the limit of quantitation for samples taken at 7-days post dose and later. For the groups receiving 0.4 mg/kg and 1 mg/kg, predicted concentrations are above the LLOQ for one and two weeks, respectively. While serum TAB levels were detectable in the top dose group (n = 1) up to day 12, the group receiving 0.4 mg/kg (n = 1) had undetectable serum TAB levels on day 7 and onwards. Corresponding RO predictions are shown in suggesting that, even at the lowest dose (0.1 mg/kg), a RO level of almost 90% was achieved at Cmax. A dose of 1 mg/kg is predicted to maintain >90% RO for more than 15 days.
Model scale-up from monkey to human
For human PK predictions, parameters associated with the linear terms of the model (CL, CLD, V1 and V2) were unchanged from monkey to human () because the scaling factor was equal to 1 based on prior in-house data (not shown). To scale the parameters describing the target-mediated elimination (Vmax and KM), differences in target expression and the antibody-target interaction across species were taken into account. The magnitude of the CD33 target sink in AML patients may be larger than that in normal monkeys either due to high receptor expression of the CD33 target on patient's blastsCitation 31 or increased number of CD33-expressing cells.Citation 32 In addition, heterogeneity in levels of CD33 expression is anticipated in patients.Citation33-Citation35 In the PK-RO model, the Vmax parameter represents the amount of antibody that is cleared by target when the target is fully saturated and can be associated with the target expression level. Based on this, a range of values for Vmax was used to assess the impact of possible differences in antigenic burden in patients.Citation 36 As shown in , CD33 expression levels measured by flow cytometry appear to be comparable or higher in human AML versus monkey monocytes, granulocytes and normal hematopoietic stem cell (HSCs). However, circulating levels of CD33-positive cells have been reported to be a few fold higher (< 5-fold) in patients with myeloid malignancies.Citation 32 Hence, the value for Vmax for human predictions was scaled up using a range of 1- to 10-fold the observed value in monkeys. The KM in humans was assumed to be equal to the monkey value based on: 1) comparable binding affinities of anti-CD33-ADC to human and monkey CD33, 2) the high degree of homology across monkeys and human CD33 (87% by sequence alignment, in house measurement by PCR) and, 3) the fact that the estimated KM value in monkey was comparable to the binding affinity KD (concentration to drive 50% of the maximum capacity of the target-mediated elimination rate is comparable to the concentration required to bind 50% of the target receptor), suggesting that binding of antibody to target is the rate-limiting step.
Human PK and RO predictions
Simulations of TAB concentration and RO after doses of 0.25, 2.4 and 21 µg/kg of anti-CD33 ADC (corresponding to doses from 17 µg to 1.5 mg for a 70 kg patient) are shown in Figure S1 (Supplementary material). These doses were chosen to result in approximately 10%, 50% and 90% RO at Cmax to explore a full range of predictions from the model and to include doses comparable to first-in-human doses administered in the clinic for other ADCs bearing pyrrolobenzodiazepine (PBD, a DNA binding agent) payloads.Citation37-Citation39 To evaluate the impact of the uncertainty of the parameters characterizing the PK nonlinearity on the human PK and RO predictions, we generated a multivariate normal distribution of 1000 pairs of values for Vmax and log(KM) using the model estimations and their covariance matrix. The predicted Cmax values for these simulated doses were 6.81, 65.4 and 572 ng/mL, respectively. Since these correspond to the estimated concentration of drug immediately after administration assuming no drug binding to target has taken place, the Cmax values increase dose proportionally (i.e., Cmax = dose/V1) () and do not depend on the non-linear parameters. Note that RO predictions at Cmax are not Vmax dependent, but the RO versus time profile is strongly dependent on its value. In Figure S1 (Supplementary material), predictions of the PK and RO at the estimated parameters together with their 5th and 95th percentile of the simulated distribution of parameters are shown. The corresponding values of AUC0-t, Cmax and RO at Cmax are in . As expected, the simulated AUCs increased more than dose proportionally and were highly dependent on the Vmax value used for translation. Moreover, for each Vmax chosen, the uncertainty on the estimated parameters results in a large range (sometimes more than an order of magnitude) of values for AUC0-t predictions, especially for the lower dose. The uncertainty in the exposure predictions needs to be considered during the selection of the early clinical doses.
Table 4. Predicted human AUC0-t using the estimated parameters as well as the 5th and 95th percentile of the simulated distribution for doses of 0.25, 2.4 and 21 µg/kg. The maximum concentration (Cmax) and the predicted values of RO at Cmax are also reported.
Comparison to prior clinical anti-CD33 molecules
Both unconjugatedCitation 40 , Citation 41 and conjugated antibodiesCitation42-Citation44 targeting CD33 have been previously studied in the clinic for treatment of AML. Lintuzumab is an unconjugated humanized IgG1 anti-CD33 antibody that was terminated during clinical development because of lack of activity as a single agent and failure to improve survival when used in combination with conventional chemotherapy.Citation 45 The binding affinity for lintuzumab to CD33 on human promyelocytic leukemia cells (HL-60) cells has been reported as KD = 3.9 ± 1.4 nM,Citation 46 an approximately 20-times greater KD (i.e., with poorer affinity) than that for our conjugated anti-CD33 ADC (KD = 0.2 nM). RO levels seven days after the administration of lintuzumab to CD33-positive patients with myeloid malignancies (n = 23) have been reported.Citation 47 We used our PK-RO model to predict RO of lintuzumab at those time points after the administered doses. We modified the value of KM to be 20 times that of our anti-CD33 ADC (i.e., 0.0602 ug/mL × 20 ∼ 1.2 ug/mL) to account for the lower binding affinity of lintuzumab. Since the CD33 target level is independent of the administered antibody, the same range of Vmax values was explored, as shown as a shaded area in . Reported measurementsCitation 47 () showed that the measured receptor availability values fall approximately within the predicted range when Vmax in humans is equal to 3- to 10- times the Vmax value in monkey.
Figure 5. (a) Predicted levels of receptor availability using the PK-RO model for lintuzumab for a range of Vmax values. Symbols and error bars correspond to the standard deviations as reported in Ref. Citation54. (b) Comparison of predicted clearance values a function of dose when the target capacity in humans is 1-fold (solid line), 3-fold (dashed line) and 10-fold (dotted line) the observed value of Vmax in cynomolgus monkey; symbols represent clinical data using AVE9633Citation 59 and GO.Citation 57 , Citation 58
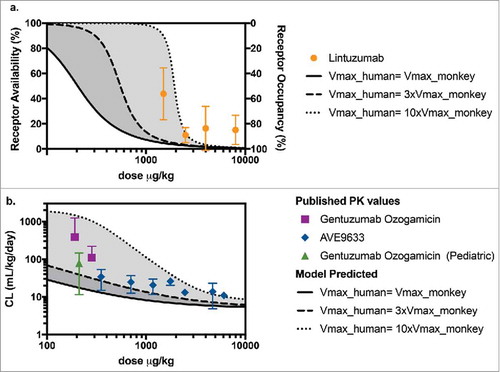
Published PK data were also available for gemtuzumab ozogamicin (GO, Mylotarg™) and AVE9633. GO is a humanized IgG4κ conjugate of the antibody hp67.6 with approximately four calicheamicin (a DNA binding agent) payload molecules in each ADC molecule. Mylotarg is currently approved for the treatment of AML. AVE9633 is a humanized IgG1 anti-CD33 conjugate, whose development was terminated after Phase 1 due to very modest activity as a single agent.Citation 45 AVE9633 carries an average of 3.5 molecules of DM4, a microtubule inhibitor, as payload. Both GO and AVE9633 bind to the CD33 human receptor with comparable or tighter affinity than anti-CD33 mAb (approximately 0.025 nMCitation 48 and 0.1 nM,Citation 49 respectively). The reported clearance values for GOCitation 50 , Citation 51 and AVE9633Citation 43 as a function of administered dose in µg/kg are shown in . The shaded area corresponds to the predicted range of clearance values calculated assuming Vmax in humans is 1 to 10 times Vmax in monkeys. For GO, the data were highly variable, but measured clearance values were closer to those predicted based on the higher end of target capacity (10-fold Vmax relative to monkey). For both GO and AVE9633, published clearance values in humans fall within the range predicted by our PK-RO model. Note that while in both cases the target is CD33, the antibodies can differ in epitope, internalization, specific and non-specific uptake rates, and the model parameters presented here may not apply, so the PK-RO prediction must be taken as an exploratory comparison only.
Discussion
Non-linear PK is commonly seen with antibodies undergoing significant TMDD, such as the unconjugated anti-CD33 mAb and the corresponding anti-CD33-ADC. Similarly, in humans, non-linear PK has been observed after the administration of CD33-targeted therapies.Citation 40 , Citation 41 , Citation 44 , Citation52-Citation54 Characterization of the target sink that may be driving the non-linear PK usually requires concentration-time profiles obtained over a range of administered doses. In the case of an ADC bearing a cytotoxic payload, the feasible dose range for a PK study may be limited by the drug's overall tolerability in animals and the sensitivity of the analytical methods. Here, we present the use of limited PK and RO data of the unconjugated antibody to inform predictions for the conjugated antibody PK. We used a two-compartment model with specific and non-specific clearance from the central compartment to capture the antibody PK. The specific elimination term can be used to capture the target-mediated component of the clearance driven by the whole-body target sink (i.e., the effect of circulating target as well as of target expressed in tissues), and the observed systemic PK reflects its overall level of saturation. Since CD33 is expressed largely in circulation, in this analysis, we assumed that the target-mediated uptake in tissues is negligible and that the RO in circulating myeloid cells is representative of the level of whole-body target saturation. This may not be a valid assumption for targets that are heavily expressed in tissues for which concentrations required to saturate circulating target may be substantially lower than those required to saturate the whole-body target capacity. The MM approach to describe non-linear PK model has previously been used as an approximation of the TMDD model;Citation 54 however, the use of RO to support the PK characterization for targets present mostly in circulation had yet not been explored.
Translation of non-linear PK from preclinical species to human requires consideration of various differences in target properties between species (e.g., total target expression levels, target tissue profiles, rate of target turnover, disease state), as well as differences in the interaction of the drug candidate with the animal and human receptors (e.g., binding affinity, trafficking of the ADC-receptor complex).Citation55-Citation57 Successful translation of PKPD models relies on the selection of the appropriate assumptions based on our best knowledge of these properties. A key assumption in this work is that the value of the MM constant (KM) is equal across species, i.e., the interactions of anti-CD33 mAb and anti-CD33 ADC are similar with the monkey and human receptor in terms of binding affinity, binding kinetics, internalization rate and efficiency. This assumption has not been probed in vivo for the antibodies examined here, and conclusions cannot be extended from our experience with other targets because the rate of these processes depend on many factors, such as receptor-mediated endocytosis, accessory modes of endocytosis, linker drug, cell lines.Citation 58 In general, for the MM approximation of the TMDD model, the KM is not equivalent to the binding affinity of the antibody receptor interaction, but represents a combination of parameters characterizing the drug-receptor interaction, including binding affinity, association and dissociation kinetics, and internalization rate. The fact that the estimated value of KM of anti-CD33-ADC (0.0602 µg/mL ≈ 0.40 nM) is comparable to the measured affinity to human promyelocytic leukemia cells (HL-60) cells (0.2 nM) and monkey CD33+ cells (0.32 nM) suggests that antibody uptake rate by target may governed by affinity and not limited by other factors such as internalization rate.
We anticipate that AML patients may have a higher and more variable antigen burden than that observed in normal cynomolgus monkey.Citation 31 Hence, a range of values of target capacity was studied, based on measured levels of expression. The underlying assumption is that antigen expression levels are related to maximum target capacity, Vmax, by the signal measured by FACS analysis. Here, we explored potential scenarios where patient's blasts have higher target load than normal cells. Human PK predictions have been provided for cases when Vmax in humans is equal to 3-fold or 10-fold the Vmax value estimated for monkeys. The resulting range of exposures (AUC) has been reported in . Here, the impact of partial or full CD33+ cells depletion (as may be expected for patients receiving repeated doses of anti-CD33 ADC), has not been considered for the prediction of clinical PK. However, for repeated doses or for patients who are anticipated to have depleted levels of CD33 target in circulation, the effect of CD33 expression in the PK of following doses would need to be considered. Dose proportionality has been assumed for the calculation of Cmax and receptor availability at Cmax. In monkeys, observed Cmax () increased roughly dose proportionally from 0.5 mg/kg to 15 mg/kg. In humans, some anti-CD33 therapies appear to show more than dose proportional increases in Cmax. For example, Cmax values (1-h post administration, Cycle 1 Week 5) after the administration of lintuzumab showed more than dose proportional increases (Cmax values were: 34.74 ± 11.71 μg/mL (n = 5), 71.65 μg/mL (n = 1), 158.33 ± 19.43 μg/mL (n = 3), 355.25 ± 141.63 μg /mL (n = 4), after 5 weekly doses of 1.5, 2.5, 4 and 8 mg/kg, respectivelyCitation 47 ). This can be explained by the presence of target in circulation such that, at lower doses, a non-negligible fraction of the total drug is bound to the target before the Cmax sample is taken. The extent of deviation from dose-proportionality can be used to infer the level of target expression in circulation.Citation 59 To address this, the initial condition of the differential equation can be corrected.Citation 60 account for the amount of drug that binds to target immediately after administration. This correction may be used to describe the non-linearity in Cmax and to correct the level of RO at Cmax.
While our comparison with lintuzumab, GO and AVE9633 is not rigorous due to the differences between both conjugates and our anti-CD33 antibodies, we highlight the use of the current industry knowledge with respect to CD33 to benchmark our calculations. Our predicted exposures indeed fall within the ranges reported from clinical observations. Although predictions of human PK cannot entirely be based or validated on prior experience with similar constructs or modalities using the same target, such information should be leveraged when possible to build confidence around PK predictions, and confirm that the overall approach is consistent with our current knowledge of this target in the clinical space.
In conclusion, characterization of non-linear PK typically requires the administration of the antibody at multiple dose levels to examine the PK over a wide range of concentrations. In the case of ADCs containing cytotoxic payloads, this may not be feasible if tolerability limits preclinical dose exploration to adequately cover the necessary concentration range. Here, we have proposed a modeling approach that utilizes limited PK and RO data obtained with the unconjugated antibody to predict the non-linear PK of an anti-CD33 ADC. Our translational assumptions from monkey to human examine a range of values of target capacity to account for antigen burden variability expected in patients. Additionally, we provided a theoretical estimation of the target coverage achieved at various dose levels. In all, the inclusion of RO measurements and data obtained using the unconjugated antibody reduced the number of animals required to characterize the non-linear PK and enabled the implementation of this translational approach that could potentially be applied to other antibodies that bind circulating targets.
Materials and methods
CD33 expression levels in cynomolgus monkey and human cells
The basal expression of CD33 was determined on normal and AML-diagnosed humans and normal cynomolgus monkey blood. Individual donor samples were evaluated by flow cytometry after co-staining with CD33 antibody, clone p67.6, and additional antibodies to identify the different blood subsets (CD14, CD11b, CD1c, CD123, BDCA3, BDCA2, CD3, CD19, CD56, CD34 and CD38; BD Biosciences, San Jose, CA) as previously described.Citation 61 Samples included normal human whole blood donors (n = 6 from Genentech, Inc. South San Francisco, CA; for phenotyping lymphocyte and myeloid subsets), normal human bone marrow donors (n = 5 from ALLCells, Alameda, CA; for phenotyping hematopoietic stem cells or HSCs, defined as lineage negative, CD34 positive and CD38 negative), AML patient bone marrow (n = 18 from patients with confirmed AML diagnosis at the Stanford Cancer Center, Stanford, CA; for phenotyping AML blasts, defined as CD45 low and CD34 positive), normal cynomolgus monkey whole blood (n = 2, BioreclamationIVt, New York, NY) and normal bone marrow donors (n = 3, Covance, Princeton, NJ).
Unconjugated and conjugated anti-CD33 antibody
The unconjugated monoclonal antibody examined here, referred to as ‘anti-CD33 mAb’, is a human IgG1 that binds to the CD33 receptor. Anti-CD33 mAb was generated at Genentech, Inc. and was provided in a clear liquid form to be administered in in vivo monkey studies. The actual concentration of anti-CD33 mAb was 11.8 mg/mL in the stock solutions. The diluent (200 mM arginine, 137 mM succinic acid, pH 5.0) was used as the vehicle control and as the diluent for unconjugated antibody. The conjugated antibody, referred here as ‘anti-CD33 ADC’, was designed to deliver a cytotoxic pyrrolobenzodiazepine (PBD, a DNA binding agent) payload to CD33-positive cells. Chemical structure and conjugation for this class of payload has been described elsewhere.Citation 62 , Citation 63 To generate anti-CD33-ADC, two payload molecules were conjugated to each anti-CD33 mAb using engineered cysteine residues at position LC K149C.Citation 63 The actual concentration of anti-CD33 ADC was 6.43 mg/mL. The same diluent used for the unconjugated antibody (200 mM arginine, 137 mM succinic acid, pH 5.0) was used as the vehicle control and as the diluent for dosing solution preparation.
Cell binding of anti-CD33-ADC to human and monkey CD33 receptor
Binding of anti-CD33-ADC to recombinant membrane-bound human- and monkey- CD33 on HEK 293 cells was measured by flow cytometry, as previously described by Leong et al.Citation 61 The test articles were tested in the range of 0, 0.003, 0.01, 0.03, 0.1, 0.3, 1 and 3 µg/ml and incubated for 30 minutes on ice followed by detection with a F(ab')2 goat-anti-human IgG (H+L) PE secondary antibody (Jackson ImmunoResearch Laboratories, West Grove, PA).
PKPD study in monkeys with anti-CD33 antibody
The PKPD study in monkeys was approved by the Institutional Animal Care and Use Committee and conducted at Charles River Laboratories (Reno, NV). Animals were administered doses of 0.5 mg/kg (n = 3) or 15 mg/kg (n = 3) of anti-CD33 mAb via a single IV dose. Blood samples for TAB analysis were taken by venipuncture and processed to collect serum at pre-dose; 15 minutes; 4 and 12 hours; and 1, 2, 4, 7, 14, 21, 28, 35 and 42 days post-dose. Serum samples collected pre-dose and 14, 28 and 42 days post-dose were also analyzed for ADA. Additionally, blood samples were taken for flow cytometry analysis one week prior to the study initiation (pre-dose) and 1, 6, 21, and 42 days post-dose to measure the occupancy of the CD33 receptor.
Toxicology study in monkey with anti-CD33 ADC
The toxicology study in monkeys was approved by the Institutional Animal Care and Use Committee and conducted at Charles River Laboratories (Reno, NV). The animals received a single IV dose of 0.1 mg/kg (n = 4), 0.2 mg/kg (n = 4), 0.4 mg/kg (n = 1), or 1 mg/kg (n = 1) of anti-CD33-ADC or vehicle control (n = 5). Blood samples were taken by venipuncture and processed to obtain serum for TAB analysis one week before study initiation (pre-dose), 10 minutes; 1, 7, 14, and 21 days post-dose. Serum samples taken prior study initiation, on Day 22, and 43 were also analyzed for ADA. RO analysis was not conducted on samples collected in this study.
PK assay: Total antibody assay
Two different assays to detect TAB (which includes fully conjugated, partially deconjugated and fully deconjugated antibodies) were used to analyze samples from the monkey PKPD study and the toxicology study. Samples from monkey PKPD study was analyzed with an ELISA assay using a CD33 extracellular domain (ECD) generated by Genentech, Inc. as the capture reagent and a goat anti-human IgG-horseradish peroxidase (HRP) (A80-319A, Bethyl Labratories, Inc. Montgomery, TX) as the detection reagent. The assay had a minimum quantitation value of 7.8 ng/mL in serum with a 1:100 minimum dilution. Samples from the monkey toxicity study were analyzed using a liquid chromatography-mass spectrometry assay. Here, to measure TAB concentration, the samples underwent affinity capture via protein-A magnetic beads. Isolated ADCs were subsequently treated with denaturation, reduction, alkylation, and trypsin digestion. A signature peptide from the human Fc region of the ADCs was selected as the surrogate to quantify the TAB concentrations in animal samples. The LLOQ of the assay was determined to be 1 μg/mL. Details of the method(s) can be found elsewhere.Citation 64
Antibody-drug-antibody assay
Two immunoassays were used to detect ADAs in the non-clinical studies. Serum samples from the monkey PKPD study were analyzed for ADA responses using an exploratory screening ELISA by the Biochemical and Cellular Pharmacology department at Genentech. The ADA ELISA utilized anti-CD33 at 0.5 ug/mL as a capture reagent and biotinylated goat anti-monkey IgG (A140-118B, Bethyl Laboratories Inc., Montgomery, TX, USA) at 5 ng/mL and streptavidin (SA) conjugated to HRP at 1:10K as the detection reagents. Study samples and control pool serum samples were diluted 50-fold. A naïve pooled cynomolgus monkey serum was used as control (n = 4) and the cutoff for the assay was assigned as two times the signal of the control serum pool. Samples with an optical density higher than two times that of the control serum pool signal were reported as ADA positive. Serum samples from the monkey toxicology study were analyzed by the BioAnalytical Sciences Department at Genentech, using a qualified generic immuno-complex ADA immunoassay in cynomolgus monkey serum.Citation 65 In this assay, serum samples were first incubated with the drug to form immune complexes with potential ADA in the sample. Then the drug:ADA complexes were captured with an immobilized mouse monoclonal anti-human Fc gamma antibody (R10Z8E9, generated at Genentech, South San Francisco, CA) followed by detection using a goat anti-monkey IgG conjugated to HRP (A140-102P, Bethyl Laboratories Inc., Montgomery, TX, USA). For each animal, the ratios between the signal of each post-baseline sample and the signal at baseline were calculated, with all baseline samples being negative by definition, regardless of signal in the assay. For each animal, post-baseline samples with signals equal to or above 1.16 of their corresponding baseline sample signal were reported as positive. The multiplication factor of 1.16 was determined to yield a false-positive rate of approximately 5%. The relative sensitivity using a human IgG-cynomolgus monkey IgG fusion was approximately 40.7 ng/mL.
PD assay: Receptor occupancy
Test articles, anti-CD33 mAb and anti-CD33 ADC, target the CD33 surface receptor on myeloid cells. To assess the occupancy of the CD33 receptor by each test article, a test article-competing commercially available anti-CD33 monoclonal antibody, AC104.3E3 (109-136-088, Miltenyi, Auburn, CA), that binds both human and monkey CD33 was used in conjunction with CD11b labeling to determine the percentage of circulating blood monocytes (high CD11b expression) and granulocytes (intermediate CD11b expression) expressing unbound CD33 receptor. Total levels of CD33 are assumed to remain unchanged throughout the duration of the study. Flow cytometry analysis was conducted using Beckman Coulter XL System II™ and BD DIVA® cytometers. The isotype for AC10E4.3E3-APC was a mIgG1-APC and appropriate isotype controls were used for blood phenotype markers.
PKPD modeling
TAB concentrations were used to characterize the PK of anti-CD33 mAb and anti-CD33 ADC. The serum concentration-time data of the monkey PKPD study were fit to a nonlinear, two-compartment model comprising specific (target-mediated) and non-specific clearance pathways as shown in . The MM approximation of the full TMDD model was selected as the simplest approach capable of capturing the system's dynamics and is appropriate for the desired model applications in this manuscript. The PK model was described by the following equations:
Figure 6. Model scheme used to describe PK-RO relationship in cynomolgus monkey and humans. The model consists of two compartments: central and peripheral. Drug concentrations are noted by C1 and C2 in the central and peripheral compartment, respectively. From the central compartment there are two main elimination mechanisms: non-specific (accounted by a CL term) and target mediated (represented by Vmax and KM). Receptor occupancy is related to concentrations by the Michaelis-Menten constant, KM.
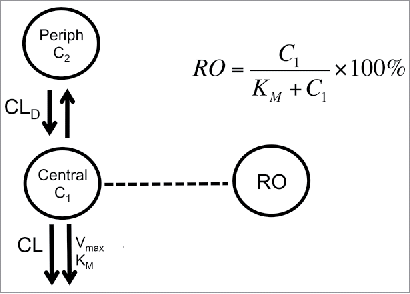
were CL is the clearance from the central compartment (i.e., the non-specific clearance pathway), CLD is distributional clearance, V1 and V2 are the volumes of distribution of the central compartment peripheral compartments, respectively. The parameters of the specific clearance pathway are: maximum target-mediated elimination rate under conditions of target saturation (Vmax) and the concentration for reaching 50% of Vmax (i.e., KM).Citation 66 , Citation 67 The last parameter, KM, was fitted using its logarithmic transformation to be able to explore a significant range of variability in the subsequent analyses. In the case of target-mediated PK, the observed clearance reflects the level of target saturation at a given concentration. Typically, PK models use only systemic concentration-time profiles to estimate the target-mediated component of the overall clearance and infer the level of target saturation. When the target is mostly expressed in circulation, levels of target occupancy in blood are an additional measure of the saturation. Based on receptor theory,Citation 68 the amount of antibody being cleared by the target is proportional to the RO that is given by:
Prediction of human PKPD
Human PK and RO were described by a model with the same mathematical structure that was developed for monkey. The specific and distributional clearances were scaled allometrically using a weight-based scaling exponent of 1. This was based on historical in-house data in monkeys and humans from ADCs conjugated using a different technology (random conjugation resulting in a heterogeneous population of ADCs versus site specific conjugation resulting in a homogeneous population in the case of anti-CD33 ADC) and a different payload (MMAE, an anti-mitotic agent versus PBD, a DNA damaging agent, used to make anti-CD33 ADC). Because we will use our PK-RO model to predict human PK at low doses, where we expect the non-linear component of the ADC clearance to be dominating, the choice of the scaling factor for the non-specific component of the clearance does not appreciably affect the predicted PK. Human PK predictions at higher doses may be more sensitive to these values, but are in general less relevant for the case study examined here, a cytotoxic ADC with doses limited by tolerability. Similarly, the volumes of the central and peripheral compartment were scaled using an exponent of 1. The parameters of target-mediated elimination were also scaled: KM and Vmax were assumed to be proportional to antibody-target affinity and levels of target expression between monkeys and humans, respectively.
To evaluate the effect of the uncertainty of the estimated parameters, human model predictions were conducted for varying values of Vmax and KM. A multivariate normal distribution of 1000 values was generated based on estimated parameters and their covariance matrix using Matlab 2016a (Mathworks Inc., Natick, MA). The AUC predictions using the mean values, as well as the 5th and 95th percentile were reported.
Abbreviations
ADC | = | antibody-drug conjugate |
AML | = | acute myeloid leukemia |
AUC | = | area under the curve |
Cmax | = | maximum concentration |
MM | = | Michaelis Menten |
PK | = | pharmacokinetics |
RA | = | receptor availability |
RO | = | receptor occupancy |
SD | = | standard deviation |
TAB | = | total antibody |
TMDD | = | target-mediated drug disposition |
Disclosure of potential conflicts of interest
All authors are employees of Genentech, a member of the Roche Group, and hold financial interest in Hoffman-La Roche.
2017MABS1645R1-file002.docx
Download MS Word (407.4 KB)References
- Deng R, Iyer S, Theil F-P, Mortensen DL, Fielder PJ, Prabhu S. Projecting human pharmacokinetics of therapeutic antibodies from nonclinical data. mAbs. 2011;3(1):61–6. doi:10.4161/mabs.3.1.13799. PMID:20962582.
- Mordenti J, Chen SA, Moore JA, Ferraiolo BL, Green JD. Interspecies scaling of clearance and volume of distribution data for five therapeutic proteins. Pharm Res. 1991;8(11):1351–9. doi:10.1023/A:1015836720294. PMID:1798669.
- Mahmood I. Interspecies scaling of protein drugs: prediction of clearance from animals to humans. J Pharm Sci. 2004;93(1):177–85. doi:10.1002/jps.10531. PMID:14648647.
- Mahmood I. Pharmacokinetic allometric scaling of antibodies: Application to the first-in-human dose estimation. J Pharm Sci. 2009;98(10):3850–61. doi:10.1002/jps.21682. PMID:19177515.
- Oitate M, Nakayama S, Ito T, Kurihara A, Okudaira N, Izumi T. Prediction of human plasma concentration-time profiles of monoclonal antibodies from monkey data by a species-invariant time method. Drug Metab Pharmacokinet. 2012;27(3):354–9. doi:10.2133/dmpk.DMPK-11-SH-059. PMID:22146109.
- Wang W, Prueksaritanont T. Prediction of human clearance of therapeutic proteins: Simple allometric scaling method revisited. Pharm. Drug Dispos. 2010;31(4):253–63.
- Luu KT, Bergqvist S, Chen E, Hu-Lowe D, Kraynov E. A model-based approach to predicting the human pharmacokinetics of a monoclonal antibody exhibiting target-mediated drug disposition. J Pharmacol Exp Ther. 2012;341(3):702–8. doi:10.1124/jpet.112.191999. PMID:22414855.
- Singh AP, Krzyzanski W, Martin SW, Weber G, Betts A, Ahmad A, Abraham A, Zutshi A, Lin J, Singh P. Quantitative prediction of human pharmacokinetics for mabs exhibiting target-mediated disposition. AAPS J. 2015;17(2):389–99. doi:10.1208/s12248-014-9690-8. PMID:25445845.
- Dong JQ, Salinger DH, Endres CJ, Gibbs JP, Hsu CP, Stouch BJ, Hurh E, Gibbs MA. Quantitative prediction of human pharmacokinetics for monoclonal antibodies. Clin Pharmacokinet. 2011;50(2):131–42. doi:10.2165/11537430-000000000-00000. PMID:21241072.
- Bender B, Leipold DD, Xu K, Shen B-Q, Tibbitts J, Friberg LE. A Mechanistic pharmacokinetic model elucidating the disposition of trastuzumab emtansine (T-DM1), an Antibody-Drug Conjugate (ADC) for Treatment of Metastatic Breast Cancer. AAPS J. 2014;16(5):994–1008. doi:10.1208/s12248-014-9618-3. PMID:24917179.
- Sukumaran S, Zhang C, Leipold DD, Saad OM, Xu K, Gadkar K, Samineni D, Wang B, Milojic-Blair M, Carrasco-Triguero M, et al. Development and translational application of an integrated, mechanistic model of antibody-drug conjugate pharmacokinetics. AAPS J. 2017;19(1):130–40. doi:10.1208/s12248-016-9993-z. PMID:27679517.
- Sukumaran S, Gadkar K, Zhang C, Bhakta S, Liu L, Xu K, Raab H, Yu SF, Mai E, Fourie-O'Donohue A, et al. Mechanism-based pharmacokinetic/pharmacodynamic model for THIOMAB™ drug conjugates. Pharm Res. 2015;32(6):1884–93. doi:10.1007/s11095-014-1582-1. PMID:25446772.
- Singh AP, Maass KF, Betts AM, Wittrup KD, Kulkarni C, King LE, Khot A, Shah DK. Evolution of Antibody-drug conjugate tumor disposition model to predict preclinical tumor pharmacokinetics of Trastuzumab-Emtansine (T-DM1). AAPS J. 2016;18(4):861–75. doi:10.1208/s12248-016-9904-3. PMID:27029797.
- Shah DK, Haddish-Berhane N, Betts A. Bench to bedside translation of antibody drug conjugates using a multiscale mechanistic PK/PD model: A case study with brentuximab-vedotin. J Pharmacokinet Pharmacodyn. 2012;39(6):643–59. doi:10.1007/s10928-012-9276-y. PMID:23151991.
- Shah DK, King LE, Han X, Wentland JA, Zhang Y, Lucas J, Haddish-Berhane N, Betts A, Leal M. A Priori Prediction of tumor payload concentrations: Preclinical case study with an Auristatin-Based Anti-5T4 Antibody-Drug Conjugate. AAPS J. 2014;16(3):452–63. doi:10.1208/s12248-014-9576-9. PMID:24578215.
- Perez HL, Cardarelli PM, Deshpande S, Gangwar S, Schroeder GM, Vite GD, Borzilleri RM. Antibody-drug conjugates: Current status and future directions. Drug Discov Today. 2014;19(7):869–81. doi:10.1016/j.drudis.2013.11.004. PMID:24239727.
- Sievers EL, Senter PD. Antibody-drug conjugates in cancer therapy. Annu Rev Med. 2013;64:15–29. doi:10.1146/annurev-med-050311-201823. PMID:23043493.
- McCombs JR, Owen SC. Antibody drug conjugates: Design and selection of linker, payload and conjugation chemistry. AAPS J. 2015;17(2):339–51. doi:10.1208/s12248-014-9710-8. PMID:25604608.
- Panowski S, Bhakta S, Raab H, Polakis P, Junutula JR. Site-specific antibody drug conjugates for cancer therapy. mAbs. 2014;6(1):34–45. doi:10.4161/mabs.27022. PMID:24423619.
- Lyon RP, Bovee TD, Doronina SO, Burke PJ, Hunter JH, Neff-LaFord HD, Jonas M, Anderson ME, Setter JR, Senter PD. Reducing hydrophobicity of homogeneous antibody-drug conjugates improves pharmacokinetics and therapeutic index. Nat Biotech. 2015;33(7):733–5. doi:10.1038/nbt.3212.
- Boswell CA, Mundo EE, Zhang C, Bumbaca D, Valle NR, Kozak KR, Fourie A, Chuh J, Koppada N, Saad O, et al. Impact of drug conjugation on pharmacokinetics and tissue distribution of Anti-STEAP1 Antibody-Drug Conjugates in Rats. Bioconjug Chem. 2011;22(10):1994–2004. doi:10.1021/bc200212a. PMID:21913715.
- Kamath AV, Iyer S. Preclinical pharmacokinetic considerations for the development of antibody drug Conjugates. Pharm Res. 2015;32(11):3470–9. doi:10.1007/s11095-014-1584-z. PMID:25446773.
- Haddish-Berhane N, Shah DK, Ma D, Leal M, Gerber HP, Sapra P, Barton HA, Betts AM. On translation of antibody drug conjugates efficacy from mouse experimental tumors to the clinic: A PK/PD approach. J Pharmacokinet Pharmacodyn. 2013;40(5):557–71. doi:10.1007/s10928-013-9329-x. PMID:23933716.
- Singh AP, Shin YG, Shah DK. Application of pharmacokinetic-pharmacodynamic modeling and simulation for antibody-drug conjugate development. Pharm Res. 2015;32(11):3508–25. doi:10.1007/s11095-015-1626-1. PMID:25666843.
- Sapra P, Betts A, Boni J. Preclinical and clinical pharmacokinetic/pharmacodynamic considerations for antibody-drug conjugates. Expert Rev Clin Pharmacool. 2013;6(5):541–55. doi:10.1586/17512433.2013.827405.
- Betts AM, Haddish-Berhane N, Tolsma J, Jasper P, King LE, Sun Y, Chakrapani S, Shor B, Boni J, Johnson TR. Preclinical to clinical translation of antibody-drug conjugates using PK/PD Modeling: A retrospective analysis of inotuzumab ozogamicin. AAPS J. 2016;18(5):1101–16. doi:10.1208/s12248-016-9929-7. PMID:27198897.
- Jurcic JG. What happened to anti-CD33 therapy for acute myeloid leukemia? Curr Hematol Malig Rep. 2012;7(1):65–73. doi:10.1007/s11899-011-0103-0. PMID:22109628.
- Walter RB, Appelbaum FR, Estey EH, Bernstein ID. Acute myeloid leukemia stem cells and CD33-targeted immunotherapy. Blood. 2012;119(26):6198–208. doi:10.1182/blood-2011-11-325050. PMID:22286199.
- Pillow TH. Novel linkers and connections for antibody-drug conjugates to treat cancer and infectious disease. Pharmaceutical Patent Analyst. 2017;6(1):25–33. doi:10.4155/ppa-2016-0032. PMID:28155578.
- Junutula JR, Raab H, Clark S, Bhakta S, Leipold DD, Weir S, Chen Y, Simpson M, Tsai SP, Dennis MS, et al. Site-specific conjugation of a cytotoxic drug to an antibody improves the therapeutic index. Nat Biotechnol. 2008;26(8):925–32. doi:10.1038/nbt.1480. PMID:18641636.
- Robillard N, Fau - Wuilleme S, Wuilleme S, Fau - Lode L, Lode L, Fau - Magrangeas F, Magrangeas F, Fau - Minvielle S, Minvielle S, Fau - Avet-Loiseau H, Avet-Loiseau H. CD33 is expressed on plasma cells of a significant number of myeloma patients, and may represent a therapeutic target. ( 0887-6924 (Print)).
- Diaz-Montero CM, Salem ML, Nishimura MI, Garrett-Mayer E, Cole DJ, Montero AJ. Increased circulating myeloid-derived suppressor cells correlate with clinical cancer stage, metastatic tumor burden, and doxorubicin-cyclophosphamide chemotherapy. Cancer Immunol Immunother. 2009;58(1):49–59. doi:10.1007/s00262-008-0523-4. PMID:18446337.
- Walter RB, Gooley TA, van der Velden VHJ, Loken MR, van Dongen JJ, Flowers DA, Bernstein ID, Appelbaum FR. CD33 expression and P-glycoprotein-mediated drug efflux inversely correlate and predict clinical outcome in patients with acute myeloid leukemia treated with gemtuzumab ozogamicin monotherapy. Blood. 2007;109(10):4168–70. doi:10.1182/blood-2006-09-047399. PMID:17227830.
- Pollard JA, Alonzo TA, Loken M, Gerbing RB, Ho PA, Bernstein ID, Raimondi SC, Hirsch B, Franklin J, Walter RB. Correlation of CD33 expression level with disease characteristics and response to gemtuzumab ozogamicin containing chemotherapy in childhood AML. Blood. 2012;119(16):3705–11. doi:10.1182/blood-2011-12-398370. PMID:22378848.
- Walter RB, Raden BW, Kamikura DM, Cooper JA, Bernstein ID. Influence of CD33 expression levels and ITIM-dependent internalization on gemtuzumab ozogamicin-induced cytotoxicity. Blood. 2005;105(3):1295–302. doi:10.1182/blood-2004-07-2784. PMID:15454492.
- Jilani I, Estey E, Huh Y, Joe Y, Manshouri T, Yared M, Giles F, Kantarjian H, Cortes J, Thomas D, et al. Differences in CD33 Intensity between various myeloid neoplasms. Am J Clin Pathol. 2002;118(4):560–6. doi:10.1309/1WMW-CMXX-4WN4-T55U. PMID:12375643.
- Bea K. First clinical results of ADCT-402, a novel pyrrolobenzodiazepine-based Antibody Drug Conjugate (ADC), in Relapsed/refractory B-cell lineage non-hodgkin lymphoma. 14th International Conference on Malignant Lymphoma (ICML); 2017.
- Horwitz SM, Fanale MA, Spira AI, et al. Interim data from the first clinical study of ADCT-30, a novel pyrrolobenzodiazapine-based antibody drug conjugate, in relapsed/refractory hodgkin/non-hodgkin lymphoma. Hematol Oncol. 2017;35:270–1. doi:10.1002/hon.2438_143.
- Stein AS, Walter RB, Erba HP, Fathi AT, Advani AS, Lancet JE, Ravandi F, Kovacsovics T, DeAngelo DJ, Bixby D, et al. A phase 1 trial of SGN-CD33A As monotherapy in patients with CD33-Positive Acute Myeloid Leukemia (AML). Blood. 2015;126(23):324.
- Feldman EJ, Brandwein J, Stone R, Kalaycio M, Moore J, O'Connor J, Wedel N, Roboz GJ, Miller C, Chopra R, et al. Phase III randomized multicenter study of a humanized Anti-CD33 monoclonal antibody, lintuzumab, in combination with chemotherapy, versus chemotherapy alone in patients with refractory or first-relapsed acute myeloid leukemia. J Clin Oncol. 2005;23(18):4110–6. doi:10.1200/JCO.2005.09.133. PMID:15961759.
- Kossman SE, Scheinberg Da, Fau - Jurcic JG, Jurcic Jg, Fau - Jimenez J, Jimenez J, Fau - Caron PC, Caron PC. A phase I trial of humanized monoclonal antibody HuM195 (anti-CD33) with low-dose interleukin 2 in acute myelogenous leukemia. ( 1078-0432 (Print)).
- Hamann PR, Hinman LM, Hollander I, Beyer CF, Lindh D, Holcomb R, Hallett W, Tsou HR, Upeslacis J, Shochat D, et al. Gemtuzumab ozogamicin, a potent and selective anti-CD33 antibody-calicheamicin conjugate for treatment of acute myeloid leukemia. ( 1043-1802 (Print)).
- Lapusan S, Vidriales MB, Thomas X, de Botton S, Vekhoff A, Tang R, Dumontet C, Morariu-Zamfir R, Lambert JM, Ozoux ML, et al. Phase I studies of AVE9633, an anti-CD33 antibody-maytansinoid conjugate, in adult patients with relapsed/refractory acute myeloid leukemia. Invest New Drugs. 2012;30(3):1121–31. doi:10.1007/s10637-011-9670-0. PMID:21519855.
- Kung Sutherland MS, Walter RB, Jeffrey SC, Burke PJ, Yu C, Kostner H, Stone I, Ryan MC, Sussman D, Lyon RP, et al. SGN-CD33A: A novel CD33-targeting antibody-drug conjugate using a pyrrolobenzodiazepine dimer is active in models of drug-resistant AML. Blood. 2013;122(8):1455. doi:10.1182/blood-2013-03-491506. PMID:23770776.
- Laszlo GS, Estey EH, Walter RB. The past and future of CD33 as therapeutic target in acute myeloid leukemia. ( 1532-1681 (Electronic)).
- Chen P, Wang J, Fau - Hope K, Hope K, Fau - Jin L, et al. Nuclear localizing sequences promote nuclear translocation and enhance the radiotoxicity of the anti-CD33 monoclonal antibody HuM195 labeled with 111In in human myeloid leukemia cells. ( 0161-5505 (Print)).
- Raza A, Jurcic JG, Roboz GJ, Maris M, Stephenson JJ, Wood BL, Feldman EJ, Galili N, Grove LE, Drachman JG, et al. Complete remissions observed in acute myeloid leukemia following prolonged exposure to lintuzumab: A phase 1 trial. Leukemia Lymphoma. 2009;50(8):1336–44. doi:10.1080/10428190903050013. PMID:19557623.
- Chang J. Biologic therapy of leukemia. Br J Cancer. 2004;90(1):281-. doi:10.1038/sj.bjc.6601432.
- Giles F, Morariu-Zamfir R, Lambert J, et al. Phase I Study of AVE9633, an AntiCD33-Maytansinoid immunoconjugate, administered as an intravenous infusion in patients with refractory/relapsed CD33-positive acute myeloid leukemia (AML). Blood. 2006;108(11):4548.
- Buckwalter M, Dowell JA, Korth-Bradley J, Gorovits B, Mayer PR. Pharmacokinetics of gemtuzumab ozogamicin as a single-agent treatment of pediatric patients with refractory or relapsed acute myeloid leukemia. J Clin Pharmacool. 2004;44(8):873–80. doi:10.1177/0091270004267595.
- Dowell JA, Korth-Bradley J, Liu H, King SP, Berger MS. Pharmacokinetics of gemtuzumab ozogamicin, an antibody-targeted chemotherapy agent for the treatment of patients with acute myeloid leukemia in first relapse. J Clin Pharmacool. 2001;41(11):1206–14. doi:10.1177/00912700122012751.
- Heider K-H, Konopitzky R, Ostermann E, et al. A Novel Fc-Engineered Antibody to CD33 with Enhanced ADCC Activity for Treatment of AML. Blood. 2012;120(21):1363.
- Hamann PR, Hinman LM, Hollander I, Beyer CF, Lindh D, Holcomb R, Hallett W, Tsou HR, Upeslacis J, Shochat D, et al. gemtuzumab ozogamicin, a potent and selective Anti-CD33 Antibody−calicheamicin conjugate for treatment of acute myeloid leukemia. Bioconjug Chem. 2002;13(1):47–58. doi:10.1021/bc010021y. PMID:11792178.
- Gibiansky L, Gibiansky E. Target-mediated drug disposition model: Approximations, identifiability of model parameters and applications to the population pharmacokinetic-pharmacodynamic modeling of biologics. Expert Opin Drug Metab Toxicol. 2009;5(7):803–12. doi:10.1517/17425250902992901. PMID:19505189.
- Tabrizi MA, Bornstein GG, Klakamp SL. Development of antibody-based therapeutics: Translational considerations. New York: Springer; 2012.
- Bornstein GG. Antibody drug conjugates: Preclinical considerations. AAPS J. 2015;17(3):525–34. doi:10.1208/s12248-015-9738-4. PMID:25724883.
- Tabrizi MA, Bornstein GG, Klakamp SL, Drake A, Knight R, Roskos L. Translational strategies for development of monoclonal antibodies from discovery to the clinic. Drug Discov Today. 2009;14(5):298–305. doi:10.1016/j.drudis.2008.12.008. PMID:19152840.
- Xu S. Internalization, trafficking, intracellular processing and actions of antibody-drug conjugates. ( 1573-904X (Electronic)).
- Grimm HP. Gaining insights into the consequences of target-mediated drug disposition of monoclonal antibodies using quasi-steady-state approximations. J Pharmacokinet Pharmacodyn. 2009;36(5):407. doi:10.1007/s10928-009-9129-5. PMID:19728050.
- Yan X, Krzyzanski W. Dose correction for the Michaelis-Menten approximation of the target-mediated drug disposition model. J Pharmacokinet Pharmacodyn. 2012;39(2):141–6. doi:10.1007/s10928-011-9233-1. PMID:22215144.
- Leong SR, Sukumaran S, Hristopoulos M, Totpal K, Stainton S, Lu E, Wong A, Tam L, Newman R, Vuillemenot BR, et al. An anti-CD3/anti-CLL-1 bispecific antibody for the treatment of acute myeloid leukemia. Blood. 2017;129(5):609. doi:10.1182/blood-2016-08-735365. PMID:27908880.
- Flygare JA, Gunzner-Toste JL, Pillow T, Howard PW, Masterson L. Pyrrolobenzodiazepines and conjugates thereof. Google Patents. 2013.
- Pillow TH, Schutten M, Yu S-F, Ohri R, Sadowsky J, Poon KA, Solis W, Zhong F, Del Rosario G, Go MAT, et al. Modulating therapeutic activity and toxicity of pyrrolobenzodiazepine antibody-drug conjugates with self-immolative disulfide linkers. Mol Cancer Ther. 2017. doi:10.1158/1535-7163.MCT-16-0641. PMID:28223423.
- Kaur S, Liu L, Cortes DF, Shao J, Jenkins R, Mylott WR Jr, Xu K. Validation of a biotherapeutic immunoaffinity-LC-MS/MS assay in monkey serum: ‘Plug-and-play’ across seven molecules. Bioanalysis. 2016;8(15):1565–77. doi:10.4155/bio-2016-0117. PMID:27396481.
- Carrasco-Triguero M, Davis H, Zhu Y, Coleman D, Nazzal D, Vu P, Kaur S. Application of a plug-and-play immunogenicity assay in cynomolgus monkey serum for ADCs at Early stages of drug development. ( 2314-7156 (Electronic)).
- Yan X, Mager DE, Krzyzanski W. Selection between Michaelis-Menten and target-mediated drug disposition pharmacokinetic models. J Pharmacokinet Pharmacodyn. 2010;37(1):25–47. doi:10.1007/s10928-009-9142-8. PMID:20012173.
- Gibiansky L, Gibiansky E, Kakkar T, Ma P. Approximations of the target-mediated drug disposition model and identifiability of model parameters. J Pharmacokinet Pharmacodyn. 2008;35(5):573–91. doi:10.1007/s10928-008-9102-8. PMID:19005743.
- Ploeger BA, Van der Graaf PH, Danhof M. Incorporating receptor theory in mechanism-based pharmacokinetic-pharmacodynamic (PK-PD) modeling. Drug Metab Pharmacokinet. 2009;24(1):3–15. doi:10.2133/dmpk.24.3. PMID:19252332.