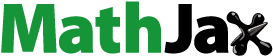
Abstract
The discovery of highly effective hepatitis C virus (HCV) treatments has led to discussion of elimination and intensified interest in models of HCV transmission. In developed settings, HCV disproportionally affects people who inject drugs (PWID), and models are typically used to provide an evidence base for the effectiveness of interventions such as needle and syringe programs, opioid substitution therapy and more recently treating PWID with new generation therapies to achieve specified reductions in prevalence and / or incidence. This manuscript reviews deterministic compartmental S-I, deterministic compartmental S-I-S and network-based transmission models of HCV among PWID. We detail typical assumptions made when modeling injecting risk behavior, virus transmission, treatment and re-infection and how they correspond with available evidence and empirical data.
Introduction
Hepatitis C virus (HCV) is a major global health problem affecting approximately 170 million people worldwide causing significant morbidity and mortality.Citation1 Treatment for chronic HCV has been available for some time, however with low cure rates (30–80%, depending on genotype) and significant side effects,Citation2,3 treatment uptake has been low.Citation4-7 Recently, ‘direct-acting antiviral’ (DAA) treatments have become available that are highly tolerable and have greater than 90% efficacy,Citation8-10 offering hope to reduce the future burden of disease. At the same time, a number of HCV vaccines are also currently in development,Citation11 creating discussion on what their role might be in eliminating HCV transmission as a public health problem.Citation11,12
HCV is transmitted via exposure to infected blood, most commonly through intravenous drug use, blood transfusions, organ transplants, from mother-to-child or through sexual contact,Citation13 although the sexual transmission of HCV is very inefficient outside the setting of HIV.Citation14,15 In developed settings blood screening and healthcare practices have virtually eliminated iatrogenic spread, meaning that HCV transmission occurs almost exclusively among people who inject drugs (PWID).Citation1,16-18 Compared to the estimated world-wide HCV prevalence of 2–3%,Citation19 the prevalence of HCV among PWID ranges from 10% to 97% globally,Citation18,20 and is estimated to be greater than 50% in many countries.Citation20 As a result, modeling studies that investigate ways to slow the HCV epidemic or reduce the number of incident cases focus on PWID, because this is where interventions are likely to have the most significant impact on the overall future burden of disease.Citation21
Once infected with HCV, an estimated 26% of people spontaneously clear the virus,Citation22 typically within a 6 month asymptomatic ‘acute’ phase.Citation23 Individuals who fail to clear their HCV infections may remain asymptomatic for many years, yet face substantial risk of liver disease.Citation24 Chronic HCV infection has been attributable to approximately 27% of cirrhosis cases and 25% of hepatocellular carcinoma cases worldwide,Citation25 and given the slow rates of liver disease progression and peaks in transmission that occurred in the 1990s, many countries are yet to experience the full burden of HCV-related liver disease.Citation26
Since its was first isolated in 1989,Citation27 HCV has been the topic of many modeling studies. This review looks at transmission models of HCV among PWID, focusing on assumptions that are made about the population at risk and transmission of the virus and how they correspond with empirical data. These models are particularly relevant for developed settings, and are typically used to provide an evidence base for the effectiveness of public health interventions such as needle and syringe programs (NSPs), opioid substitution therapy (OST) and more recently treating PWID with DAAs.
S-I Models
Deterministic compartmental S-I models of HCV transmission among people who inject drugs
One of the most basic models of HCV transmission involves classifying PWID into 2 compartments: susceptible (S), or chronically infected (I). PWID enter this model (start their injecting career) in the S compartment, possibly move from the S to the I compartment (if they become infected), and eventually leave (end their injecting career) from either compartment. These movements—in particular becoming infected—can be described by either an ordinary differential equation (ODE) or by a probabilistic (‘stochastic’) mechanism. Models described by ODEs are referred to as deterministic, since for given initial conditions the outcomes are always the same. Stochastic models are appropriate for modeling the initial spread of a disease through a population or for modeling small numbers of people—for example an infectious disease outbreak within a single hospital, where probabilistic transmission plays an important role—while deterministic models are appropriate for well-established epidemics and larger scales. Because HCV is approximately endemic among PWID in most countries, deterministic approaches are suitable and hence typically used.
Population characteristics
Deterministic compartmental models of HCV transmission among PWID necessarily include, sometimes implicitly, a minimum of 3 features describing the population: 1) the duration PWID are at risk of infection or transmission (the ‘length of injecting career’); 2) the injecting risk behavior of PWID during this period (assumptions about the frequency of injecting, frequency of sharing and the characteristics of injecting networks); and 3) the transmissibility of HCV among PWID (the transmission risk per ‘sharing’ event between discordant individuals). The third feature is typically used, in conjunction with the first 2, to calibrate chronic prevalence in the model and is discussed in the next section. The illicit nature and stigmatisation of injecting drug use make these parameters challenging to determine through epidemiological studies: it is difficult to recruit representative samples of PWID; sharing of injecting paraphernalia may be underreported; and drug markets frequently change, leading to different consumption patterns.Citation28-30 Moreover, where estimates are available they are unlikely to extend to different settings due to cultural or socio-demographic differences.
There is considerable variation in the length of injecting careers around the world, ranging from a short time period up to 30 years prior to cessation.Citation31,32 Individuals may also go through periods of cessation and relapse within their injecting careers.Citation33 This variation in injecting length and cycling in and out of injecting is an issue, as many deterministic models assume a constant rate of flow out of the model that is based on a single estimate for the length of injecting career—usually assumed to be an average of between 7 and 14 years, depending on setting—producing outcomes that are sensitive to this parameter.Citation34,35
Injecting risk behavior is also difficult to measure. Firstly, individuals cycles through periods of high and low injecting frequency.Citation36 Secondly, injecting paraphernalia is mostly shared through social networks,Citation37 in particular with current sex partners.Citation38 Both of these factors make the risk of transmission per injection highly heterogeneous and dynamic across the population. To address this, deterministic models often approximate risk heterogeneity dichotomously (or categorically) by including ‘high risk’ and ‘low risk’ PWID.Citation35,39,40 This involves estimating a relative risk factor and allowing individuals to continuously cycle between risk groups. Some degree of assortative mixing is also often used to approximate social groups, but ultimately deterministic models are based on restrictive assumptions about risk behaviours and mixing of PWID. This may be reasonable for estimating injecting frequency: in the absence of treatment programs such as OST, which have been shown to reduce injecting frequencyCitation41 and sharing risk,Citation42 the average injecting frequency may be fairly constant.Citation30 However, deterministic models are limited in their ability to approximate injecting risk (i.e. the number and turnover of sharing partners), as they are unable to capture the properties of injecting networks that have been shown to be important for blood borne virus transmission.Citation43-45
Transmission
Transmission is generally modeled to occur only through ‘sharing’, the quantitation of which can be imprecise given the number of ways in which injecting equipment can be shared or (imperfectly) cleaned before re-use.Citation46 Clinically the risk of acquiring HCV per needle stick injury is at least roughly known (0.31% risk per event,Citation47 however this is also likely to depend on the strain of the virus),Citation48 but the ambiguity of a sharing event and difficulties estimating the frequency and characteristics of ‘sharing’ partners limit how these estimates can be used. However unlike transmission risk, it is much simpler to estimate chronic HCV prevalence—a model outcome. Data sources such as NSPsCitation49 and cohort studies can objectively determine HCV prevalence among PWID where blood samples are taken, and estimates are available for many countries.Citation20 Although sometimes the infectivity per sharing event is assumed and the resulting prevalence predicted,Citation50 the more common approach is the reverse: the model is solved for a transmission parameter that—given the assumptions about the length of injecting career and injecting risk behavior—produces an equilibrium HCV prevalence matching what has been observed in epidemiological studies.
As an illustrative example, consider the following open deterministic S-I model. Let S be the proportion of the population who are susceptible, I be the proportion of the population who are infectious (so that S + I = 1), and assume an average length of injecting career 1/μ. This model is described by the equations(Equation 1)
(Equation 1) that can be solved for an infection parameter β that gives correct equilibrium prevalence
: namely
.
A consequence of calibrating transmission this way is that the HCV epidemic is assumed to be in equilibrium prior to the implementation of any interventions. Modeling suggests that in developed settings HCV incidence has greatly reduced from a peak in the 1990s due to a combination of blood supply screening and harm reduction interventions such as NSPs,Citation26 so that even if prevalence currently approximates an equilibrium, liver fibrosis and disease stage among the aging chronically infected population does not.Citation51,52 For models that compare strategies for reducing prevalence by means of reducing incidence—such as NSP or OST implementation or scale up—this may be appropriate. However many cost-effectiveness analyses require further disaggregation of chronic infection, given the slow disease progression and the differences in care and quality of life for later stages of fibrosis. Where these models estimate the total costs of future healthcare they should consider how the burden of disease is changing.Citation26
S-I-S Models
Deterministic compartmental S-I-S models: including treatment
Deterministic compartmental S-I-S models extend the S-I model by allowing the possibility of infected individuals returning to the susceptible compartment, for example through treatment. Although the S-I-S model assumes treated individuals are identical to infection naïve individuals, there are many variations that do not. In general, complete or partial immunity after treatment can be modeled by including a recovered (R) compartment (the S-I-R or S-I-R-S models), but for HCV even though some immunity has been observed following clearance of infectionCitation53-55 (either spontaneously or through treatment), the percentage of patients likely to experience immunity and for how long is currently unclear, making the S-I-S model an appropriate way to include treatment.
Modeling treatment numbers
With the advent of DAAs, models must realistically include a treatment feature—firstly to reduce the morbidity and mortality associated with HCV, and secondly because of the potential impact of ‘treatment as prevention’ (TasP). The usual approach is to implement treatment rates that are proportional to the total PWID population size (which may include a period of scale up) rather than the total infected population. For example, treatment rates of 40/1000 PWID per year might be assumed, which represents the maximum number of PWID (less if there are not enough people infected) who are moved from the I compartment to the S compartment each year. This is a more plausible assumption than the alternative: if treatment rates were assumed to be proportional to the size of the infected population, for example 2% of infected PWID per year, this would imply the bulk of infrastructure requirements would be in initial rather than later years, but the generally low engagement of PWID in care makes this scenario unrealistic for HCV treatment. A consequence of assuming treatment rates that are proportional to the total population is that the epidemic can collapse quickly once treatment numbers pass a critical threshold, compared to if treatments were given to a proportion of the infected population which has slower and more asymptotic effects.Citation34
Modeling re-infection
Models that include treatment must make assumptions about re-infection. High rates of re-infection have been documented among PWID,Citation56 however it is difficult to estimate as it requires frequent testing of large cohorts of PWID.Citation57,58 Further, it is not always clear what is meant by re-infection. Biologically re-infection is defined as an initial infection being completely resolved prior to a subsequent infection,Citation59 and requires discrimination from an individual's infection relapsing.Citation60 Viral sequencing can be necessary to distinguish examples such as an individual having only one strain of a co-infection (simultaneous acquisition of 2 or more HCV strains)Citation59 cured. Deterministic compartmental S-I-S models rarely include this level of complexity, and as a result re-infection is usually assumed to occur at the same rate as initial infection. These rates should be interpreted with caution, since as discussed above, models often use the infection parameter to calibrate the model prevalence.
Network models
Network models portray individuals as a set of vertices (‘nodes’) of a graph, with edges connecting 2 nodes if the corresponding individuals have some non-zero probability of disease transmission. For example, for HCV transmission among PWID edges may exist between partners, members of the same social network or current inmates of the same prison. The network structure removes many of the restrictive assumptions of deterministic models: network models no longer rely on perfect mixing and can incorporate large amounts of heterogeneity among individuals. This has been a successful approach to modeling the HIV epidemic, establishing the importance of social ties and networks among PWID for prevention strategies.Citation61
Estimating network structure
Estimating the network structure underlying these types of models remains difficult. Surveys of PWID struggle to gain information beyond the nodal degree of the respondent (the number edges a person in the network has, indicating the number of people they have shared equipment with) and the basic characteristics of nearest neighbors, and can experience reporting bias due to the stigmatisation of injecting risk behaviors. Further, the ‘hidden’ nature and high incarceration rate of PWID means that disruptions and changes to social networks are substantial. This dynamic nature of injecting networks is thought to play an important role in transmission,Citation62 in addition to its structure.Citation63
It is often assumed that the injecting networks of PWID can be modeled using Exponential Random Graph Models with social circuit dependence.Citation64 With this assumption, survey data collected through cohort studies and samples of PWID can be used to estimate features of the larger injecting networks from which the respondents come,Citation65 and this technique has been successfully used to model the spread of infectious disease among PWID.Citation66 However, given the underreporting of sharing and imperfect abilities of surveys to capture network structure, modeling closed networks of PWID is unrealistic, and efforts to calibrate transmission to achieve initial prevalence have previously relied on some ‘infection import’ probabilities.Citation67 The importation of infections is required because the closed network cannot explain the incidence data and the imported cases represent unobserved processes not captured by the network data. Currently these type of models also assume a static network structure, and further work is required to test how the temporal nature of social networks influences transmission and interventions.Citation68 Nevertheless, network models of HCV transmission have shown that the injecting network itself plays an important role in transmission,Citation69 and that significant reductions can be made if needle sharing is confined to one person.Citation70
Implementing interventions
Assumptions about how interventions are implemented are also important for network models. For example, interventions such as TasP have been shown to be more effective when network dependent approaches are taken, such as when PWID and their immediate injecting network are treated together—a ‘treat-your-friends’ strategy—rather than treating PWID individually.Citation43 Using network-based strategies for public health interventions in this way is not new, and underpins the contact tracing strategy used to treat and control sexually transmitted infections (STIs). The difference is that instead of aiming to protect the health of an individual's past network, treat-your-friends aims to protect an individual from re-infection through their current network. Interventions involving current injecting networks of PWID are likely to be more effective for HCV than for STIs because firstly, the prevalence of HCV among PWID is much higher than the prevalence of many STIs in the community—meaning that reinfection plays a much more significant role in the HCV epidemic that it does for STIs—and secondly, the hidden nature and stigmatisation of injecting drug use make contact tracing a difficult exercise in practice. Network models are an extremely useful tool for testing these ideas.
Sensitivities
Outcomes of models and their sensitivities to underlying assumptions
Models of HCV transmission are typically used to estimate the benefits of interventions, for example the effects of reducing syringe sharing,Citation71 or combinations of OST and NSP.Citation72 The high cost of new treatments has led to much recent focus on the benefits that can be gained by treating PWID, in particular given the possibility of re-infection and need for re-treatment. This has been looked at from a healthcare cost-effectiveness perspective,Citation73,74 and alternatively by estimating the number of onwards transmissions that a cured individual will no longer cause.Citation39 The latter is a part of the TasP concept that has featured prominently in global HIV policy. Typically, TasP models for HCV have been used to estimate the treatment rates required to achieve meaningful reductions in overall prevalence.Citation40
Although some vaccines are currently in development,Citation11 their properties are unclear, as are their costs. Understanding how a vaccine's properties and cost influence which HCV elimination strategies are the most cost-effective will be vital for future decision making, and models have been used to estimate how effective a vaccine would need to be to be useful,Citation35,75 what a vaccine might mean for the future burden of disease,Citation76 what settings it would be most useful in,Citation35 and whether or not this would be cost-effective compared to treatment.Citation77
Sensitivity to length of injecting career
Most of these outputs are sensitive to assumptions about the length of injecting career, since the method described for calibrating transmission means that the rate of infection is a function of the length of injecting career. For example, in Equation Equation1(Equation 1)
(Equation 1) ,
= 1/length of injecting career. Hence doubling (or halving) the length of injecting career leads to a halving (or doubling) of the infection parameter, and hence of the incidence, while achieving the same equilibrium prevalence. The assumption of a long injecting career causes the epidemic in the model to be driven by longer chronic infections rather than by new cases, and has important implications for interventions: anything designed to reduce prevalence by reducing incidence, such as NSP and OST, will be more effective when the length of injecting career is shorter;Citation72 while interventions for chronic infections such as treatment will be more effective where injecting careers are longer.Citation35,40 Similarly, models that allow new population members to enter the model at a stage in which they are already infected require a lower force of infection to achieve equilibrium prevalence, and so will predict greater benefits for interventions such as treatment and reduced benefits for OST and NSP. This may be particularly evident in models that include injecting cessation and subsequent relapse, where a person can enter the model already HCV infected from their previous period of injecting, as opposed to new or naive PWID assumed to be HCV negative.
Sensitivity to heterogeneity
Model outputs are often sensitive to risk heterogeneity, both in terms of relative risk difference and the length of cycles between high and low risk behavior. For example, consider the following open deterministic S-I transmission model with injecting risk heterogeneity:
Here, PWID are categorised dichotomously as high or low injecting risk; with relative difference in transmission risk ; are assumed to mix proportionally and not cycle between risk categories; and
is the force of infection with transmission parameter β (to be calibrated). In this example
represents the number of individuals who are infected in the effective population,
. This can also been interpreted as the number of contaminated syringes among all shared syringes.Citation78 Calibrating β to achieve the desired equilibrium prevalence gives β as a decreasing function of both the length of injecting career and
().
Figure 1. If the initial prevalence of heterogeneous S-I deterministic compartment models is calibrated by varying the transmission risk (effective contact) parameter (β), then for a fixed initial prevalence and proportion of the population at high risk β will be a decreasing function of the average length of injecting career (1/μ) and the relative difference in transmission risk ().
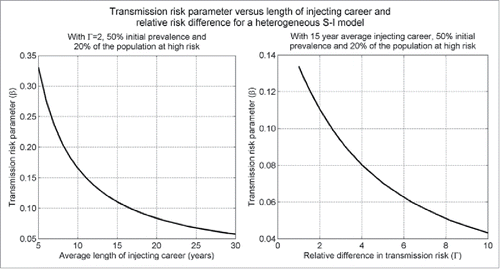
Thus for a fixed population prevalence, as the injecting risk heterogeneity in the model increases, the transmission parameter decreases, which shifts the epidemic toward more chronic infections and again predicts greater benefits for treatment but reduced benefits for OST and NSPs. Conversely, if the above model were modified to allow PWID to cycle between risks categories, as the time spent in each category decreases, so does the heterogeneity of the model (as the risk is more evenly spread throughout the population and the virus can more easily reach the whole population). This has been found to predict reduced benefits of treatment and increased benefits of injecting risk targeted interventions.Citation35,40,79
There is some benefit in models being sensitive to these parameters. Since the length of injecting career and any heterogeneity is often implemented as an approximation to highly heterogeneous human behavior, they are almost always included in sensitivity analyses, and therefore have their impacts tested against the conclusions of the study.
Challenges For The Future
Strains
Since HCV was first isolated, 7 different genotypes and more than 50 subtypes have been identified circulating worldwide.Citation80 Different strains of HCV have different subtype-specific transmission patterns,Citation48 yet very little attention has been paid to multi-strain models. In particular, co-infection and superinfection (individuals with chronic HCV infection, who, after re-exposure to HCV, present with a new and different HCV viral strain),Citation59 can have implications for the effectiveness of treatment interventions, which will become increasingly important with the emergence of drug-resistance.Citation81
HCV and HIV co-infection
HCV infected individuals are at high risk of acquiring HIV since both infections share transmission routes. Although the prevalence of HCV/HIV co-infection among HCV infected PWID varies considerably globally, it is estimated to be as high as 95% in many Asian countries.Citation82 This is important because HCV/HIV co-infection can significantly change the dynamics of the HCV epidemic: infection with HIV reduces the likelihood of spontaneously clearing a newly acquired HCV infection,Citation54,83 accelerates the progression of HCV-related liver cirrhosis,Citation84 and leads to more rapid death from end stage liver disease.Citation85 Given the current lack of empirical data on the dynamics of co-infection, the best approach to simultaneously model HCV and HIV is not so clear; however, with increasingly sophisticated surveillance systems being implemented in many countries this is likely to be an important area for future work.Citation86 In particular, co-infection models have already provided insight into the extent of the sexual transmission of HIV,Citation87 and have helped to better understand the impacts of NSPsCitation71 on prevalence and the effectiveness of HCV treatments.Citation88
Compartmental versus network based models
Compartmental models are typically used because network level data is expensive to collect and often unavailable for the desired setting. This makes it important to understand when they make similar predictions and whether it is possible to make reasonable adjustments so that the models are more comparable. This is an active field of research and the relationships between different types of models are highly dependent on the characteristics of the disease and population being modeled;Citation89-92 for example, compartmental models have been developed that approximate the effects of a sexual partner network on STI transmission,Citation90 but the approximation breaks down as population heterogeneity increases. For this reason there would be benefit to investigating, in the specific context of HCV, the size and direction of errors when compartmental models are used to approximate what is predominately a network problem. Although this would require extensive data to parametrise both types of models, it may enable future studies to know when compartmental models could be used to approximate the results of network models without having to collect empiric network data. This could significantly increase the accuracy and reduce the costs of future projects.
Conclusion
With HCV recently considered ‘curable’ due to advancements in DAA therapy, modeling public health responses to HCV among PWID is, and will continue to be, an active area of research. Better understanding of injecting careers, injecting equipment sharing and injecting networks is required in order to improve the accuracy of these models: empirical studies suggest that these parameters are enormously heterogeneous, yet they are often implemented as point values. Little is known of what this means for the predictions of the simple deterministic models that are commonly used. It is important to investigate what the potential inclusion of more accurate empirical data and more sophisticated mathematical models could mean for previous models' predictions of HCV transmission.
Disclosure of Potential Conflicts of Interest
No potential conflicts of interest were disclosed.
References
- Hajarizadeh B, Grebely J, Dore GJ. Epidemiology and natural history of HCV infection. Nat Rev Gastroenterol Hepatol 2013; 10(9):553-62; PMID:23817321
- Okanoue T, Sakamoto S, Itoh Y, Minami M, Yasui K, Sakamoto M, Nishioji K, Katagishi T, Nakagawa Y, Tada H. Side effects of high-dose interferon therapy for chronic hepatitis C. J Hepatol 1996; 25:283-91; PMID:8895006
- Manns MP, McHutchison JG, Gordon SC, Rustgi VK, Shiffman M, Reindollar R, Goodman ZD, Koury K, Ling MH, Albrecht JK. Peginterferon alfa-2b plus ribavirin compared with interferon alfa-2b plus ribavirin for initial treatment of chronic hepatitis C: a randomised trial. Lancet 2001; 358:958-65; PMID:11583749
- Dore GJ. The changing therapeutic landscape for hepatitis C. Medical J Australia 2012; 196:629-32
- Walsh N, Lim M, Hellard M. Using a surveillance system to identify and treat newly acquired hepatitis C infection. J Gastroenterol Hepatol 2008; 23:1891-4; PMID:19120877
- Grebely J, Oser M, Taylor LE, Dore GJ. Breaking down the barriers to hepatitis C virus (HCV) treatment among individuals with HCV/HIV coinfection: action required at the system, provider, and patient levels. J Infect Dis 2013; 207:S19-S25; PMID:23390301
- Robaeys G, Grebely J, Mauss S, Bruggmann P, Moussalli J, De Gottardi A, Swan T, Arain A, Kautz A, Stöver H. Recommendations for the management of hepatitis C virus infection among people who inject drugs. Clin Infect Dis 2013; 57:S129-S37; PMID:23884061
- Lawitz E, Poordad FF, Pang PS, Hyland RH, Ding X, Mo H, Symonds WT, McHutchison JG, Membreno FE. Sofosbuvir and ledipasvir fixed-dose combination with and without ribavirin in treatment-naive and previously treated patients with genotype 1 hepatitis C virus infection (LONESTAR): an open-label, randomised, phase 2 trial. Lancet 2014; 383:515-23
- Gane EJ, Stedman CA, Hyland RH, Ding X, Svarovskaia E, Subramanian GM, Symonds WT, McHutchison JG, Pang PS. Efficacy of nucleotide polymerase inhibitor sofosbuvir plus the NS5A inhibitor ledipasvir or the NS5B non-nucleoside inhibitor GS-9669 against HCV genotype 1 infection. Gastroenterol 2014; 146:736-43.e1
- Poordad F, Lawitz E, Kowdley KV, Cohen DE, Podsadecki T, Siggelkow S, Heckaman M, Larsen L, Menon R, Koev G. Exploratory study of oral combination antiviral therapy for hepatitis C. N Engl J Med 2013; 368:45-53; PMID:23281975
- Baumert TF, Fauvelle C, Chen DY, Lauer GM. A prophylactic hepatitis C virus vaccine: A distant peak still worth climbing. J Hepatol 2014; 61:S34-S44; PMID:25443345
- Shi C, Ploss A. Hepatitis C virus vaccines in the era of new direct-acting antivirals. Expert Rev Gastroenterol Hepatol 2013; 7(2):171-85
- Chen SL, Morgan TR. The Natural History of Hepatitis C Virus (HCV) Infection. Int J Med Sci 2006; 3:47-52; PMID:16614742
- Dienstag JL. Sexual and perinatal transmission of hepatitis C. Hepatol 1997; 26:66S-70S; PMID:9305667
- Terrault NA. Sexual activity as a risk factor for hepatitis C. Hepatol 2002; 36:S99-S105; PMID:12407582
- The Kirby Institute. HIV, viral hepatitis and sexually transmissible infections in Australia Annual Surveillance Report 2014. The University of New South Wales, Sydney NSW 2052 2014
- Shepard CW, Finelli L, Alter MJ. Global epidemiology of hepatitis C virus infection. Lancet Infect Dis 2005; 5:558-67; PMID:16122679
- Nelson PK, Mathers BM, Cowie B, Hagan H, Des Jarlais D, Horyniak D, Degenhardt L. Global epidemiology of hepatitis B and hepatitis C in people who inject drugs: results of systematic reviews. Lancet 2011; 378:571-83; PMID:21802134; http://dx.doi.org/10.1016/S0140-6736(11)61097-0
- Lavanchy D. The global burden of hepatitis C. Liver Int 2009; 29:74-81; PMID:19207969; http://dx.doi.org/10.1111/j.1478-3231.2008.01934.x
- Aceijas C, Rhodes T. Global estimates of prevalence of HCV infection among injecting drug users. Int J Drug Policy 2007; 18:352-8; PMID:17854722; http://dx.doi.org/10.1016/j.drugpo.2007.04.004
- Hellard M, Doyle JS, Sacks‐Davis R, Thompson AJ, McBryde E. Eradication of hepatitis C infection: The importance of targeting people who inject drugs. Hepatol 2014; 59:366-9; PMID:23873507; http://dx.doi.org/10.1002/hep.26623
- Micallef JM, Kaldor JM, Dore GJ. Spontaneous viral clearance following acute hepatitis C infection: a systematic review of longitudinal studies. J Viral Hepatitis 2006; 13:34-41; http://dx.doi.org/10.1111/j.1365-2893.2005.00651.x
- Mondelli MU, Cerino A, Cividini A. Acute hepatitis C: diagnosis and management. J Hepatol 2005; 42:S108-S14; PMID:15777565; http://dx.doi.org/10.1016/j.jhep.2004.10.017
- Grebely J, Dore GJ. What is killing people with hepatitis C virus infection? Semin Liver Dis 2011:31(4):331-9
- Alter MJ. Epidemiology of hepatitis C virus infection. World J Gastroenterol 2007; 13:2436; PMID:17552026; http://dx.doi.org/10.3748/wjg.v13.i17.2436
- Razavi H, Waked I, Sarrazin C, Myers R, Idilman R, Calinas F, Vogel W, Correa M, Hézode C, Lázaro P. The present and future disease burden of hepatitis C virus (HCV) infection with today's treatment paradigm. J Viral Hepatitis 2014; 21:34-59; http://dx.doi.org/10.1111/jvh.12248
- Choo Q-L, Kuo G, Weiner AJ, Overby LR, Bradley DW, Houghton M. Isolation of a cDNA clone derived from a blood-borne non-A, non-B viral hepatitis genome. Science 1989; 244:359-62; PMID:2523562; http://dx.doi.org/10.1126/science.2523562
- Scott N, Caulkins JP, Ritter A, Dietze P. Patterns of drug preference and use among people who inject drugs in Melbourne, Australia. Addiction Research Theory 2015:1-10; http://dx.doi.org/10.3109/16066359.2015.1031118
- Scott N, Caulkins JP, Ritter A, Quinn C, Dietze P. High‐frequency drug purity and price series as tools for explaining drug trends and harms in Victoria, Australia. Addiction 2015; 110:120-8; PMID:25220170; http://dx.doi.org/10.1111/add.12740
- Scott N, Caulkins JP, Ritter A, Dietze P. How patterns of injecting drug use evolve in a cohort of people who inject drugs. Trends Issues Crime Criminal Justice 2015; 502.
- Oppenheimer E, Tobutt C, Taylor C, Andrew T. Death and survival in a cohort of heroin addicts from London clinics: a 22‐year follow‐up study. Addiction 1994; 89:1299-308; PMID:7804091; http://dx.doi.org/10.1111/j.1360-0443.1994.tb03309.x
- Henderson N, Ross J, Darke S, Teesson M, Lynskey M. Longitudinal studies of dependent heroin users in Australia: Feasibility and benefits. 2002.
- Fazito E, Cuchi P, Mahy M, Brown T. Analysis of duration of risk behaviour for key populations: a literature review. Sex Transmitted Infect 2012; 88:i24-i32; PMID:23172343; http://dx.doi.org/10.1136/sextrans-2012-050647
- Martin NK, Vickerman P, Hickman M. Mathematical modelling of hepatitis C treatment for injecting drug users. J Theoretical Biol 2011; 274:58-66; http://dx.doi.org/10.1016/j.jtbi.2010.12.041
- Scott N, McBryde E, Vickerman P, Martin N, Stone J, Drummer H, Hellard M. The role of a hepatitis C virus vaccine: modelling the benefits alongside Direct-Acting Antiviral treatments. In submission 2015.
- Kimber J, Copeland L, Hickman M, Macleod J, McKenzie J, De Angelis D, Robertson JR. Survival and cessation in injecting drug users: prospective observational study of outcomes and effect of opiate substitution treatment. BMJ 2010; 341:c3172; PMID:20595255; http://dx.doi.org/10.1136/bmj.c3172
- Iversen J, Maher L. Australian Needle and Syringe Program National Data Report 2007–2011. The Kirby Institute, University of New South Wales 2012.
- Fraser S, Treloar C, Bryant J, Rhodes T. Hepatitis C prevention education needs to be grounded in social relationships. Drugs: Education, Prevention and Policy 2013; 21:88-92.
- de Vos A, Prins M, Kretzschmar M. Hepatitis C virus treatment as prevention among injecting drug users: who should we cure first? Addiction 2015; 110(6):975-83; PMID:25586171
- Martin NK, Vickerman P, Grebely J, Hellard M, Hutchinson SJ, Lima VD, Foster GR, Dillon JF, Goldberg DJ, Dore GJ, Hickman M. Hepatitis C virus treatment for prevention among people who inject drugs: Modeling treatment scale-up in the age of direct-acting antivirals. Hepatol 2013; 58:1598-609; http://dx.doi.org/10.1002/hep.26431
- Gowing L, Farrell MF, Bornemann R, Sullivan LE, Ali R. Oral substitution treatment of injecting opioid users for prevention of HIV infection. Cochrane Database Syst Rev 2011; 8:CD004145; PMID:21833948
- Gibson DR, Flynn NM, McCarthy JJ. Effectiveness of methadone treatment in reducing HIV risk behavior and HIV seroconversion among injecting drug users. Aids 1999; 13:1807-18; PMID:10513638; http://dx.doi.org/10.1097/00002030-199910010-00002
- Hellard M, Rolls DA, Sacks-Davis R, Robins G, Pattison P, Higgs P, Aitken C, McBryde E. The impact of injecting networks on hepatitis C transmission and treatment in people who inject drugs. Hepatol 2014; 60:1861-70; http://dx.doi.org/10.1002/hep.27403
- Koopman J. Modeling infection transmission. Annu Rev Public Health 2004; 25:303-26; PMID:15015922; http://dx.doi.org/10.1146/annurev.publhealth.25.102802.124353
- Welch D, Bansal S, Hunter DR. Statistical inference to advance network models in epidemiology. Epidemics 2011; 3:38-45; PMID:21420658; http://dx.doi.org/10.1016/j.epidem.2011.01.002
- Palmateer N, Hutchinson S, McAllister G, Munro A, Cameron S, Goldberg D, Taylor A. Risk of transmission associated with sharing drug injecting paraphernalia: analysis of recent hepatitis C virus (HCV) infection using cross-sectional survey data. J Viral Hepatitis 2014; 21:25-32; http://dx.doi.org/10.1111/jvh.12117
- Gabriella De Carli M, Puro V, Ippolito G. Risk of hepatitis C virus transmission following per-cutaneous exposure in healthcare workers. Infection 2003; 31.
- Pybus OG, Charleston MA, Gupta S, Rambaut A, Holmes EC, Harvey PH. The epidemic behavior of the hepatitis C virus. Science 2001; 292:2323-5; PMID:11423661; http://dx.doi.org/10.1126/science.1058321
- Iversen J, Maher L. Australian Needle and Syringe Program National Data Report 2008–2012. The Kirby Institute, University of New South Wales. ISBN: 1448-5915 2013.
- Mather D, Crofts N. A computer model of the spread of hepatitis C virus among injecting drug users. Eur J Epidemiol 1999; 15:5-10; PMID:10098989; http://dx.doi.org/10.1023/A:1007548307196
- Law MG, Dore GJ, Bath N, Thompson S, Crofts N, Dolan K, Giles W, Gow P, Kaldor J, Loveday S. Modelling hepatitis C virus incidence, prevalence and long-term sequelae in Australia, 2001. Int J Epidemiol 2003; 32:717-24; PMID:14559738; http://dx.doi.org/10.1093/ije/dyg101
- Davis GL, Albright JE, Cook SF, Rosenberg DM. Projecting future complications of chronic hepatitis C in the United States. Liver Transplantation 2003; 9:331-8; PMID:12682882; http://dx.doi.org/10.1053/jlts.2003.50073
- Osburn WO, Fisher BE, Dowd KA, Urban G, Liu L, Ray SC, Thomas DL, Cox AL. Spontaneous control of primary hepatitis C virus infection and immunity against persistent reinfection. Gastroenterol 2010; 138:315-24; http://dx.doi.org/10.1053/j.gastro.2009.09.017
- Mehta SH, Cox A, Hoover DR, Wang X-H, Mao Q, Ray S, Strathdee SA, Vlahov D, Thomas DL. Protection against persistence of hepatitis C. Lancet 2002; 359:1478-83; PMID:11988247; http://dx.doi.org/10.1016/S0140-6736(02)08435-0
- Dahari H, Feinstone SM, Major ME. Meta-analysis of hepatitis C virus vaccine efficacy in chimpanzees indicates an importance for structural proteins. Gastroenterol 2010; 139:965-74; http://dx.doi.org/10.1053/j.gastro.2010.05.077
- Aitken CK, Lewis J, Tracy SL, Spelman T, Bowden DS, Bharadwaj M, Drummer H, Hellard M. High incidence of hepatitis C virus reinfection in a cohort of injecting drug users. Hepatol 2008; 48:1746-52; http://dx.doi.org/10.1002/hep.22534
- Vickerman P, Grebely J, Dore GJ, Sacks-Davis R, Page K, Thomas DL, Osburn WO, Cox AL, Aitken CK, Hickman M. The more you look, the more you find: effects of hepatitis C virus testing interval on reinfection incidence and clearance and implications for future vaccine study design. J Infect Dis 2012; 205:1342-50; PMID:22457292; http://dx.doi.org/10.1093/infdis/jis213
- Sacks-Davis R, McBryde E, Grebely J, Hellard M, Vickerman P. Many hepatitis C reinfections that spontaneously clear may be undetected: Markov-chain Monte Carlo analysis of observational study data. J Royal Society Interface 2015; 12:20141197; http://dx.doi.org/10.1098/rsif.2014.1197
- Blackard JT, Sherman KE. Hepatitis C virus coinfection and superinfection. J Infect Dis 2007; 195:519-24; PMID:17230411; http://dx.doi.org/10.1086/510858
- Grady BP, Schinkel J, Thomas XV, Dalgard O. Hepatitis C virus reinfection following treatment among people who use drugs. Clinical Infect Dis 2013; 57:S105-S10; http://dx.doi.org/10.1093/cid/cit301
- Kretzschmar M, Wiessing LG. Modelling the spread of HIV in social networks of injecting drug users. Aids 1998; 12:801-11; PMID:9619813; http://dx.doi.org/10.1097/00002030-199807000-00017
- Aitken CK, McCaw RF, Bowden DS, Tracy SL, Kelsall JG, Higgs PG, Kerger MJ, Nguyen H, Crofts JN. Molecular Epidemiology of Hepatitis C Virus in a Social Network of Injection Drug Users. J Infect Dis 2004; 190:1586-95; PMID:15478062; http://dx.doi.org/10.1086/424678
- Aitken C, Lewis J, Hocking J, Bowden D, Hellard M. Does information about IDUs' injecting networks predict exposure to the hepatitis C virus. Hepat Mon 2009; 9:17-23
- Robins G, Snijders T, Wang P, Handcock M, Pattison P. Recent developments in exponential random graph (< i> p*) models for social networks. Social Networks 2007; 29:192-215; http://dx.doi.org/10.1016/j.socnet.2006.08.003
- Pattison PE, Robins GL, Snijders TA, Wang P. Conditional estimation of exponential random graph models from snowball sampling designs. J Mathematical Psychol 2013; 57:284-96; http://dx.doi.org/10.1016/j.jmp.2013.05.004
- Rolls DA, Wang P, Jenkinson R, Pattison PE, Robins GL, Sacks-Davis R, Daraganova G, Hellard M, McBryde E. Modelling a disease-relevant contact network of people who inject drugs. Social Networks 2013; 35:699-710; http://dx.doi.org/10.1016/j.socnet.2013.06.003
- Rolls DA, Sacks-Davis R, Jenkinson R, McBryde E, Pattison P, Robins G, Hellard M. Hepatitis C transmission and treatment in contact networks of people who inject drugs. PloS one 2013; 8:e78286; PMID:24223787; http://dx.doi.org/10.1371/journal.pone.0078286
- Snijders TA, Pattison PE, Robins GL, Handcock MS. New specifications for exponential random graph models. Sociological Methodol 2006; 36:99-153; http://dx.doi.org/10.1111/j.1467-9531.2006.00176.x
- Sacks-Davis R, Daraganova G, Aitken C, Higgs P, Tracy L, Bowden S, Jenkinson R, Rolls D, Pattison P, Robins G. Hepatitis C virus phylogenetic clustering is associated with the social-injecting network in a cohort of people who inject drugs. PloS One 2012; 7:e47335; PMID:23110068; http://dx.doi.org/10.1371/journal.pone.0047335
- Hutchinson SJ, Bird SM, Taylor A, Goldberg DJ. Modelling the spread of hepatitis C virus infection among injecting drug users in Glasgow: implications for prevention. Int J Drug Policy 2006; 17:211-21; http://dx.doi.org/10.1016/j.drugpo.2006.02.008
- Vickerman P, Hickman M, Judd A. Modelling the impact on Hepatitis C transmission of reducing syringe sharing: London case study. Int J Epidemiol 2007; 36:396-405; PMID:17218325; http://dx.doi.org/10.1093/ije/dyl276
- Martin NK, Hickman M, Hutchinson SJ, Goldberg DJ, Vickerman P. Combination interventions to prevent HCV transmission among people who inject drugs: modeling the impact of antiviral treatment, needle and syringe programs, and opiate substitution therapy. Clinical Infect Dis 2013; 57:S39-S45; http://dx.doi.org/10.1093/cid/cit296
- Visconti AJ, Doyle JS, Weir A, Shiell AM, Hellard ME. Assessing the cost‐effectiveness of treating chronic hepatitis C virus in people who inject drugs in Australia. J Gastroenterol Hepatol 2013; 28:707-16; PMID:23173753; http://dx.doi.org/10.1111/jgh.12041
- Martin NK, Vickerman P, Miners A, Foster GR, Hutchinson SJ, Goldberg DJ, Hickman M. Cost-effectiveness of hepatitis C virus antiviral treatment for injection drug user populations. Hepatol 2012; 55:49-57; http://dx.doi.org/10.1002/hep.24656
- Hahn JA, Wylie D, Dill J, Sanchez MS, Lloyd-Smith JO, Page-Shafer K, Getz WM. Potential impact of vaccination on the hepatitis C virus epidemic in injection drug users. Epidemics 2009; 1:47-57; PMID:20445816; http://dx.doi.org/10.1016/j.epidem.2008.10.002
- Chaib E, Oliveira MCFd, Galvão FHF, Silva FD, D’Albuquerque LAC, Massad E. Theoretical impact of an anti-HCV vaccine on the annual number of liver transplantation. Medical Hypotheses 2010; 75:324-7
- Krahn MD, John-Baptiste A, Yi Q, Doria A, Remis RS, Ritvo P, Friedman S. Potential cost-effectiveness of a preventive hepatitis C vaccine in high risk and average risk populations in Canada. Vaccine 2005; 23:1549-58; PMID:15694507; http://dx.doi.org/10.1016/j.vaccine.2004.09.023
- de Vos A, Kretzschmar M. Benefits of hepatitis C virus treatment: A balance of preventing onward transmission and re-infection. Mathematical Biosciences 2014; 258:11-8; PMID:25242609; http://dx.doi.org/10.1016/j.mbs.2014.09.006
- De Vos AS, Kretzschmar ME. The efficiency of targeted intervention in limiting the spread of HIV and Hepatitis C Virus among injecting drug users. J Theoretical Biol 2013; 333:126-34; http://dx.doi.org/10.1016/j.jtbi.2013.05.017
- Smith DB, Bukh J, Kuiken C, Muerhoff AS, Rice CM, Stapleton JT, Simmonds P. Expanded classification of hepatitis C virus into 7 genotypes and 67 subtypes: Updated criteria and genotype assignment web resource. Hepatol 2014; 59:318-27; http://dx.doi.org/10.1002/hep.26744
- Roberts M, Andreasen V, Lloyd A, Pellis L. Nine challenges for deterministic epidemic models. Epidemics 2014; 10:49-53
- Matthews GV, Dore GJ. HIV and hepatitis C coinfection. J Gastroenterol Hepatol 2008; 23:1000-8; PMID:18707597; http://dx.doi.org/10.1111/j.1440-1746.2008.05489.x
- Thomas DL, Astemborski J, Rai RM, Anania FA, Schaeffer M, Galai N, Nolt K, Nelson KE, Strathdee SA, Johnson L. The natural history of hepatitis C virus infection: host, viral, and environmental factors. JAMA 2000; 284:450-6; PMID:10904508; http://dx.doi.org/10.1001/jama.284.4.450
- Benhamou Y, Bochet M, Di Martino V, Charlotte F, Azria F, Coutellier A, Vidaud M, Bricaire F, Opolon P, Katlama C. Liver fibrosis progression in human immunodeficiency virus and hepatitis C virus coinfected patients. Hepatol 1999; 30:1054-8; http://dx.doi.org/10.1002/hep.510300409
- Graham C, Baden L, Yu E, Mrus J, Carnie J, Heeren T, Koziel M. Influence of human immunodeficiency virus infection on the course of hepatitis C virus infection: a meta-analysis. Clinical Infect Dis 2001; 33:562-9; http://dx.doi.org/10.1086/321909
- Kretzschmar M, Wiessing L. New challenges for mathematical and statistical modeling of HIV and hepatitis C virus in injecting drug users. Aids 2008; 22:1527-37; PMID:18670211; http://dx.doi.org/10.1097/QAD.0b013e3282ff6265
- `Vickerman P, Martin NK, Roy A, Beattie T, Group EC, Des Jarlais D, Strathdee S, Wiessing L, Hickman M. Is the HCV-HIV co-infection prevalence amongst injecting drug users a marker for the level of sexual and injection related HIV transmission? Drug Alcohol Depend 2013; 132:172-81; PMID:23453261; http://dx.doi.org/10.1016/j.drugalcdep.2013.01.020
- Martin TC, Martin NK, Hickman M, Vickerman P, Page EE, Everett R, Gazzard BG, Nelson M. Hepatitis C virus reinfection incidence and treatment outcome among HIV-positive MSM. Aids 2013; 27:2551-7; PMID:23736152; http://dx.doi.org/10.1097/QAD.0b013e32836381cc
- Bansal S, Grenfell BT, Meyers LA. When individual behaviour matters: homogeneous and network models in epidemiology. J Royal Society Interface 2007; 4:879-91; http://dx.doi.org/10.1098/rsif.2007.1100
- Ferguson NM, Garnett GP. More realistic models of sexually transmitted disease transmission dynamics: sexual partnership networks, pair models, and moment closure. Sex Transm Dis 2000; 27:600-9; PMID:11099075; http://dx.doi.org/10.1097/00007435-200011000-00008
- Meyers L. Contact network epidemiology: Bond percolation applied to infectious disease prediction and control. Bulletin American Mathematical Society 2007; 44:63-86; http://dx.doi.org/10.1090/S0273-0979-06-01148-7
- Rahmandad H, Sterman J. Heterogeneity and network structure in the dynamics of diffusion: Comparing agent-based and differential equation models. Management Science 2008; 54:998-1014; http://dx.doi.org/10.1287/mnsc.1070.0787