Abstract
Recent climate change, environmental design, and ecological conservation policies require new and existing urban developments to mitigate and offset carbon dioxide emissions and for cities to become carbon neutral. Some North American models and tools are available and can be used to quantify the carbon offset function of urban trees. But, little information on urban tree carbon storage and sequestration exists from the European Southern Alps. Also, the use of these North American models in Europe has never been assessed. This study developed a protocol to quantify aboveground carbon (C) storage and sequestration using a subsample of urban trees in Bolzano, Italy, and assessed two existing and available C estimation models. Carbon storage and sequestration were estimated using city-specific dendrometrics and allometric biomass equations primarily from Europe and two other United States models; the UFORE (Urban Forest Effects Model) and the CUFR Tree Carbon Calculator (CTCC). The UFORE model carbon storage estimates were the lowest while the CUFR Tree Carbon Calculator (CTCC) C sequestration estimates were the highest. Results from this study can be used to plan, design, and manage urban forests in northern Italy to maximize C offset potential, provide ecosystem services, and for developing carbon neutral policies. Findings can also be used to predict greenhouse gas emissions from tree maintenance operations as well as estimating green waste yield from landscape maintenance activities and its use as biofuel and compost. Managers need to be aware that available models and methods can produce statistically different C storage and sequestration estimates.
Introduction
Climate change is one of the most important and pressing environmental, economic, and security issues our world faces today (Barnett Citation2003; IPCC Citation2007; Karagiannis & Soldatos Citation2010). Urban areas are steadily growing throughout the world (Grimm et al. Citation2008) and by 2030 it is expected that 60% of the world’s population will be living in cities (Rydin et al. Citation2012). Thus, as urban environments become more important as living space for humans, they are an increasing source of carbon emissions.
Several studies in North America, China, and Australia (Brack Citation2002; Nowak & Crane Citation2002; Zhao et al. Citation2010; Dobbs et al. Citation2011; Martin et al. Citation2012; Roy et al. Citation2012), and more recently in the United Kingdom and Germany (Davies et al. Citation2011; Strohbach & Haase Citation2012; Strohbach et al. Citation2012), have shown that trees in urban environments remove carbon dioxide from the atmosphere through growth and photosynthesis, and store excess carbon as biomass in roots, stems, and branches. Indirectly, urban trees also reduce building energy used for cooling through their shade and climate amelioration effects, thereby reducing CO2 emissions from decreased energy production (Akbari et al. Citation2001).
The estimation of tree carbon sequestration depends on species types and their mortality and growth characteristics as well as their overall condition (Escobedo et al. Citation2010; Lawrence et al. Citation2012). Urban tree mortality can be influenced by site and tree characteristics such as land use, natural disturbance (e.g. pests, fire, and drought), human activities and urbanization effects (Iakovoglou et al. Citation2002; Nowak et al. Citation2004; Lawrence et al. Citation2012), and stewardship (Sklar & Ames Citation1985). Similarly, tree growth is influenced by genetics, climate, soil, moisture, light, and competition (Peper & McPherson Citation1998; Bühler et al. Citation2007). These effects on tree growth and mortality are well-known in European forests, but the majority of studies of urban trees growth rates have been conducted in the United States of America (USA) (Jo & McPherson Citation1995; Iakovoglou et al. Citation2002; Lawrence et al. Citation2012; Roy et al. Citation2012). We know only a few studies on urban tree mortality and growth in northern Italy (Sanesi et al. Citation2007; Semenzato et al. Citation2011; Marziliano et al. Citation2013), but no studies providing information specific to urban tree growth rates in South Tyrol, Italy, are known to us.
Several European cities have begun to formulate CO2 mitigation policies and this is exemplified by the city of Bolzano, Italy, which decided to become carbon neutral by 2030 (Sparber et al. Citation2010). This carbon sequestration mitigation potential of urban trees is being considered a regulating ecosystem service (Escobedo et al. Citation2011) and according to the EU Biodiversity Strategy 2020, by 2014 all European member states should map and assess the state of ecosystem services in their national territory (Maes et al. Citation2012). However, with the exception of some studies in Germany and the United Kingdom (Davies et al. Citation2011; Strohbach & Haase Citation2012; Strohbach et al. Citation2012) and assessments in Spain, Switzerland, and England using the i-Tree Eco/Urban Forest Effects (UFORE) model developed in the USA (i-Tree Citation2012a) we know of no studies of carbon storage and sequestration by urban trees in Europe and particularly in South Tyrol in peer-reviewed literature.
The United States models and modeling approaches are currently the basis for tools that are become increasingly applied not only in North America and China, but in Europe as well (e.g. UFORE, i-Tree Eco, i-Tree Streets, CUFR Tree Carbon Calculator (CTCC)) (i-Tree Citation2012b). Aguaron and McPherson (Citation2012) have compared the Eco/UFORE and other North American C storage estimation models with tree data from a United States city. In addition, Escobedo et al. (Citation2013) destructively measured aboveground tree biomass and C storage for urban Quercus spp. and compared their C storage results to tree-level C storage estimates from the Eco/UFORE and CTCC models. The authors found that Eco consistently underestimated C storage by 15% and CTCC overestimated by 2%, on average. To our knowledge, the appropriateness of these models for European trees has not been assessed. According to Ferrini and Fini (Citation2011) errors of modeled carbon estimates can be substantial.
As such, C storage and sequestration methods that are developed using local or regional allometric equations and site-specific growth rates and dendrometrics should provide more reliable, consistent, and context-specific information. Therefore, the two specific objectives of this study were to: (1) estimate aboveground carbon storage and sequestration for a subsample of public trees in Bolzano using Italian and European allometric equations and local growth rates obtained from re-measurements, and (2) assess the performance of this method against carbon storage and sequestration models from the USA that are increasingly being applied in Europe. The role of biomass removal for maintenance and its related carbon emissions will also be discussed.
Material and methods
Study area
The study area was the City of Bolzano, Italy, located in the autonomous region of Trentino-Alto Adige/South Tyrol in northern Italy (46° 29′ 28″ N, 11° 21′ 15″ E). Bolzano is the capital of the province of Alto Adige/South Tyrol in the Southern Alps and its 2011 census showed a population of about 100,000 people (Comune di Bolzano Citation2012). The city of Bolzano covers an area of over 50 km2 with approximately 12,000 public urban trees (Comune di Bolzano 2010, personal communication). Multi-year climatic data (1981–2011) shows that the annual average temperature in Bolzano is 12.6°C and average annual rainfall is 701 mm (Provincia Autonoma di Bolzano Citation2012). The coldest month of the year is January with a minimum of −4.1°C, a maximum of 6.6°C, and an average of 1.2°C. The warmest month is July with a minimum of 16.3°C, a maximum of 30.3°C, and an average of 23.3°C. The extreme records range from −17°C to +40°C.
Allometric equations and carbon storage
According to the principle of allometry, a tree allometric equation relates biomass, volume, or several tree components to stem diameter at breast height (DBH), tree height, and/or other dendrometric variables (Henry et al. Citation2013).
The use of group (i.e. composite) allometric equations, or the processes used to assign both species and non-species-specific allometric equations to sampled urban trees to estimate biomass and subsequent C storage, is an internationally accepted approach (Jo & McPherson Citation1995; Strohbach & Haase Citation2012). Indeed, this approach is the basis of models such as the Eco/UFORE and CTCC (Nowak et al. Citation2008; Aguaron & McPherson Citation2012; Yoon et al. Citation2013). The majority of these allometric equations are derived primarily from non-urban, forest-grown trees that are destructively sampled (i.e. felled and weighed on site; Basuki et al. Citation2009; Yoon et al. Citation2013). However, due to local regulations, liability, and public perceptions and safety, destructive sampling is expensive and difficult in an urban environment. Although McHale et al. (Citation2009) found that some of these allometric equations for forest-grown trees yield similar biomass estimates for urban-grown trees; these allometric equations can produce very different results when applied to sites outside the region where the equations were originally developed (Zapata-Cuartas et al. Citation2012).
Therefore, since the Eco/UFORE and CTCC models use North American equations and this study was conducted in Europe, we used site-specific tree species, tree dendrometrics such as stem circumference (subsequently converted to diameter), and height data and applied mostly European-specific allometric equations derived from the literature (Appendix 1) to better approximate local urban tree C storage estimates. The equations in Appendix 1 were used specifically to calculate dry weight aboveground biomass of each measured tree and not total dry weight biomass due to the complexity in estimating the belowground portion as reported by Hutyra et al. (Citation2011) and Strohbach and Haase (Citation2012). Dry weight aboveground biomass, obtained from equations in Appendix 1 was multiplied by 0.5 to obtain C storage.
Field sampling
Our study used existing tree inventory data from the City of Bolzano, Italy (Servizio Giardineria Citation2013). As is the case for most cities, Bolzano’s urban tree inventory was developed to assess tree condition, hazards and risks, and overall public safety. Therefore, the data had to be supplemented with field measurements. We used ArcGIS (Version 10) and obtained a subsample of trees from the inventory using a stratified random sample – according to land cover classes – and selected individual trees in the inventory’s spatial database for field measurement (Piano Urbanistico Comunale Citation2012).
During June 2011, we measured the selected trees and collected data for 475 trees. Specific measurements included: tree species, total and crown base height (m), crown width in two directions (m), percent crown dieback, percent missing canopy, and crown light exposure (). Specific field methods are outlined in Nowak et al. (Citation2008). Since Bolzano’s urban tree inventory only provided girth measurements data at 1 meter above the surface (Russo Citation2013), we also re-measured tree circumference (cm) at 1 meter and additionally at 1.37 meter above the surface. The circumference was then converted to diameter (DH) and DBH by dividing by π.
Figure 1. (color online) Urban trees parameters sampled in Bolzano, N Italy: Sp = species, DH = diameter at 1 m, DBH = diameter at breast height (1.37 m), Cb = crown base height, Ht = total height, Cw = crown width, CLE = crown light exposure, PCM = percent canopy missing, D = crown dieback (Nowak et al. Citation2002, Citation2008).
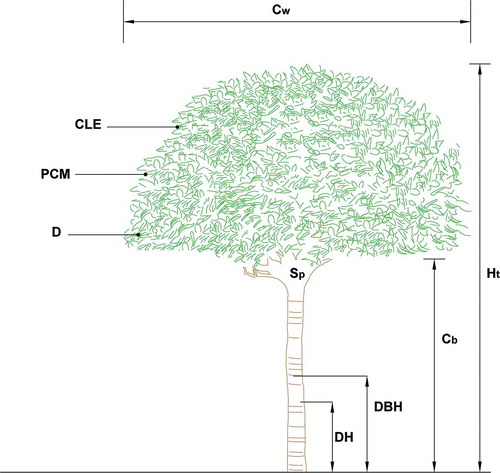
The DBH data were used in our European allometric equations, and DBH and other data were used in the UFORE model to quantify carbon storage and sequestration. The CTCC requires only information on tree species, DBH, and an overall characterization of Bolzano’s climate.
Estimated height increments and growth rates
Several allometric equations in Appendix 1 require continuous data on tree height (m) in addition to DBH. However, Bolzano’s tree inventory provided only tree height classes (Russo Citation2013). To obtain the necessary tree height increment data we used our 2011 subsample data to develop an Ordinary Least Squares predictive regression model h = f (DBH) based on the 2011 subsample’s measured tree height (h; m) and DBH (cm) to estimate the function parameters for the statistical relationship of DBH – h (; (Pretzsch Citation2009)). The model was developed using the PROC REG procedure in the Statistical Analysis Software (Version 9.2).
Table 1. Tree height (y, in meters) prediction using DBH (x, in centimeters) models for urban tree genera in Bolzano, Italy.
We then used the tree diameter–height models from to estimate height in 2011 (Hest1) and height in year 2012 (Hest2) by using measured 2011 DBH and the estimated growth rates reported in the following results section. The mean annual tree height increment () was then calculated as the difference between the estimated height at year 2011 and the estimated height at year 2012 using Equation (1):
where is the mean annual tree height increment (m/year), Hest2 is the estimated height (m) at year 2012 (m), and Hest1 is the estimated height (m) at year 2011. The tree height in 2012 (H2) was then derived using Equation (2) using the mean annual tree height increment (Hi; m/year) multiplied by the number of years (n) added to the height measured in 2011 (H1m):
Finally, diameter growth of the individual trees was calculated using diameter at 1 meter above the surface (DH), as the difference between the DH measured at the beginning and the end of a given time period (Laar & van Akça Citation2007).
Specifically in this study, the annual growth rate (AGR; cm/year) was calculated using Equation (3) (Stoffberg et al. Citation2008):
where, AGR is the annual growth rate (cm/year), DHY1 is the DH at a given year i.e. different DHs were measured during different years for different trees thus years change with different locations, DHY2 is the DH in 2011 and t is the time period (months) between measurements. To increase sample sizes for individual tree species, AGR and mean were averaged at the taxonomic order and division level (USDA Citation2013). Trees that had a DHY2 less than DHY1 were excluded from the analyses.
Carbon sequestration and biomass removals from pruning operations
Annual carbon sequestration was the estimated amount of carbon assimilated to biomass by a tree stem and branches during 1 year of growth. Thus, in this study, annual gross carbon sequestration (kg/year) was estimated as the difference of C stored between year x (2011) and year x + 1 (2012) (Liu & Li Citation2012) and was determined using an individual tree’s annual growth rate and predicted height increment as explained in the previous section.
A report on municipal waste (ISPRA Citation2012) shows that the green waste biomass from urban vegetation mowing and urban tree pruning operations in the Trentino-Alto Adige/South Tyrol region was 15,705 t in 2009. Hence, the amount of biomass waste from pruning operations can and should be accounted for when estimating net carbon sequestration effects from urban trees (Sajdak & Velazquez-Marti Citation2012). Thus, to better estimate a portion of the net annual carbon sequestration, we estimated the amount of annual biomass removals to account for maintenance-related indirect C emissions associated with Bolzano’s tree population. According to Bolzano’s Gardens Department (Personal communication, 2012), trees in parks are primarily pruned for health reasons. If there are no particular problems, the trees are not pruned and the only two trees that are subject to periodic and systematic pruning during the analysis period were Sophora japonica L., which are pruned every 2 years, and Platanus hybrida Brot., which are pruned every 7–10 years. However, the amount of biomass that is removed by tree pruning operations in Bolzano has never been measured. So, to account for maintenance-related C emissions for biomass removal, we calculated the green waste biomass removal (y) obtained from pruning Sophora japonica L. using the following linear Equation (4) derived from Sajdak and Velazquez-Marti (Citation2012) data:
where y is the dry weight biomass obtained from annual pruning operations (y; kg) and x is the DBH (cm). This assumes one single pruning intervention in 2012 for all Sophora japonica L. trees in our subsample.
UFORE and CTCC data input methods
The UFORE model is used to estimate the benefits and costs of urban trees and was developed in the late 1990s by the United States Department of Agriculture’s Forest Service to quantify urban forest structure, function, and value (Nowak & Crane Citation2000). A recent and updated user interface version is available for use and is referred to as i-Tree Eco. Using field measurements, study area characteristics, hourly annual meteorological data, and hourly annual pollution concentrations data the model quantifies several ecosystem services and disservices (Nowak et al. Citation2008).
The UFORE/i-Tree Eco model (referred to as UFORE hereafter) calculates urban forest and individual total tree (aboveground and belowground) carbon storage using forest-grown tree allometric biomass equations (Nowak Citation1994; Nowak et al. Citation2002). Dry weight biomass estimates for open-grown street trees are multiplied by a factor of 0.8 (Nowak et al. Citation2002) since these trees tend to have less aboveground biomass than predicted by these forest-derived biomass equations for trees of the same DBH (Nowak Citation1994; Nowak & Crane Citation2000). Total tree biomass estimates are then multiplied by 0.5 to obtain total stored carbon. Specific details can be found in Nowak et al. (Citation2008).
Annual gross C sequestration is also estimated by the UFORE model as the difference in estimates of changes in carbon storage between year x and year x + 1 (Nowak et al. Citation2002). Once C storage is obtained for year x, a growth rate is used for each tree to obtain a DBH and subsequent C storage at year x + 1 for the same tree. The model uses average DBH growth rates obtained from a few sites in the USA (Nowak et al. Citation2002; Lawrence et al. Citation2012). For example, for trees in forest stands, the model uses an annual growth rate of 0.38 cm/year (Smith & Shirley Citation1984; Nowak et al. Citation2002), for park-like structure the model uses 0.61 cm/year (Nowak et al. Citation2002). Average height growth is calculated based on formulas obtained from Fleming (Citation1988) as reported by Nowak et al. (Citation2002) and the specific DBH growth factor used for the tree. According to Nowak et al. (Citation2002) and Nowak et al. (Citation2008), growth rates are then adjusted based on the tree condition (i.e. no adjustment for trees in fair to excellent condition, trees in poor condition are multiplied by 0.76, critical trees by 0.42, dying trees by 0.15, and dead trees by 0). Adjustment factors are based on percent crown dieback and the assumption that less than 25% crown dieback had a limited effect on DBH growth rates. The more recent Eco version also adjusts the base growth rate based on the study area’s average annual plant growing period (i-Tree Citation2012a).
Another available model is the Center for Urban Forest Research’s (CUFR) Tree Carbon Calculator (CTCC; Urban Forest Project Reporting Protocol Citation2008) that was developed by the USDA Forest Service, Pacific Southwest Research Station. The CTCC is a Microsoft Excel spreadsheet that estimates urban tree carbon dioxide sequestration and building heating/cooling energy savings. The model estimates CO2 sequestration for individual trees located in one of the sixteen climate zones of the USA (Aguaron & McPherson Citation2012). The CTCC requires climate zone, species, and DBH or age input data to calculate individual tree CO2 sequestration (kg/tree/year), total CO2 stored (kg/tree), and aboveground biomass (dry weight) (kg/tree). Tree size and growth data were developed from samples of about 1000 urban trees and approximately 20 predominant species in each of the 16 United States reference climate zone cities (Aguaron & McPherson Citation2012). Many of the biomass equations used to derive total CO2 stored and sequestered are derived from open-grown city trees according to Aguaron and McPherson (Citation2012).
Using tree data from our Bolzano tree inventory 2011 subsample, we adapted the input variables for use in the UFORE (Version ACE 6.5) model’s complete tree inventory option based on methods outlined in Nowak et al. (Citation2002, Citation2008). We also formatted our subsample data for the use in the CTCC model. According to McPherson (Citation2010) and McPherson and Peper (Citation2012), the use of the CTCC and i-Tree Streets (formerly STRATUM) models is dependent on selecting an appropriate reference city in the United States. Therefore, Bolzano’s trees were matched to existing CTCC tree species and climates using similarities in tree taxonomy, growth forms, and overall tree structure. Specific CTCC inputs for Bolzano are presented in (Appendix 2) and are based on climate information from Bonatti (Citation2008).
Finally, to better assess our European allometric-based urban tree C storage and sequestration methods to the UFORE and CTCC models, we converted UFORE estimated total tree C estimates into aboveground C by subtracting the belowground portion using a root-to-shoot ratio of 0.26 as reported in Nowak et al. (Citation2002) and Cairns et al. (Citation1997). The CTCC model was adjusted by dividing the total biomass by 1.28 since total biomass is 1.28 times the aboveground biomass (Aguaron & McPherson Citation2012).
Model assessment
To assess the performance of the UFORE and CTCC model against our allometric equation-based approach, we tested for significant differences (P < 0.05) between these three methods using the PROC TTEST procedures in SAS version 9.2. Specifically, we used a paired t-test to test the null hypothesis that there were no differences in carbon storage and sequestration between the means from each of the three methods. Additionally, data were checked for normality using Q–Q plots and the Kolmogorov–Smirnov test. Data for the three model outputs were then fitted to a linear regression and comparison made between variables (e.g. Allometric equations vs. CTCC, Allometric equations vs. UFORE, and CTCC vs. UFORE) using a PROC GLM procedure in SAS and tested (P < 0.05) to determine whether the slope differed from 1.0.
Results
Forest structure
The mean AGR in the subsample are presented in according to taxonomic ‘division’ and ‘order’. For example, the order Fabales includes the following species: Cercis siliquastrum L., Gleditsia triacanthos L., Gymnocladus dioicus (L.) K. Koch, Robinia pseudoacacia L., Sophora japonica L., and Wisteria sinensis (Sims) DC. Overall, the order Rosales had the greatest mean AGR (1.02 cm/year) while the order Magnoliales had the lowest mean AGR (0.57 cm/year). presents the mean annual height increments in m/year and shows that Populus spp. and Salix spp. had the greatest height increments (0.63 m/year), while Tilia spp., Robinia pseudoacacia L., Gleditsia triacanthos L., and Sophora japonica L. had the lowest height increments (0.13 m/year).
Table 2. The ten most common public tree species in Bolzano, Italy, and the number sampled in 2011 (n), mean diameter at breast height (DBH) and height in meters (m). SE is standard error.
Table 3. Mean annual growth rate (AGR) of urban trees in the city of Bolzano, Italy.
Table 4. Predicted mean annual height increments (m/year) of urban tree species in the city of Bolzano, Italy.
Comparison of storage estimations
Using our allometric equation method we estimated that the total tree carbon stored in our subsample was 179.14 Mg. Meanwhile, using our field measurement data as model inputs, we estimate 140.15 Mg of C storage using the CTCC model and 134.89 Mg using the UFORE model (). The amount of carbon stored in the five most frequent tree species using the three different methods are also presented in .
Figure 2. Total carbon stored (Mg) by 475 trees in Bolzano calculated using three different methods.
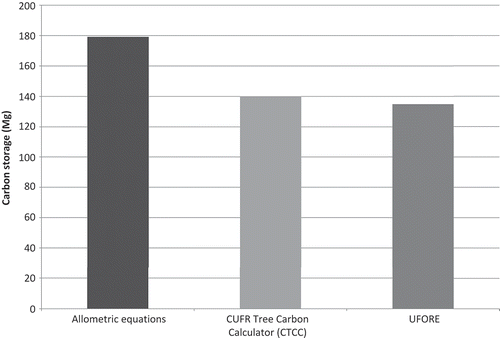
Figure 3. Average carbon storage (kg) estimates for the five most common tree species calculated using three different methods. Error bars represent ± one standard error of the mean.
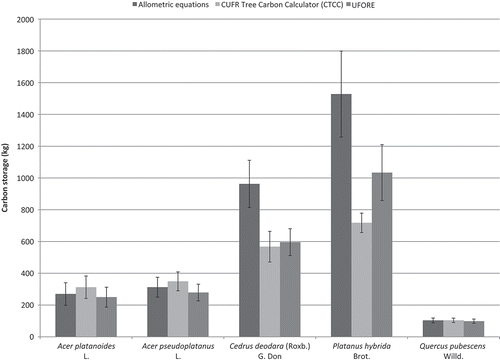
The paired t-test shows that predictions from our allometric equations are significantly higher than the CTCC (t = 4, P < 0.0001) and UFORE (t = 8.43, P < 0.0001) models. But, there was no significant difference in predictions between the CTCC and UFORE (t = −0.82, P = 0.413). Additionally, the regression slope between our allometric equations and the CTCC model was significantly different from 1 (P = 0.003), which suggests that predictions from two methods are also different. Similarly, the slope between our allometric equations, UFORE (P =< 0.0001), and CTCC and UFORE (P =< 0.0001) were also significantly different from 1 (P =< 0.0001); therefore, we can say that predictions were statistically different.
Comparison of C sequestration estimates
The total gross annual carbon sequestration for trees in our subsample was 5.73 Mg/year using the allometric equations and Bolzano’s growth rates and/or height increment predictions. However 8.27 Mg/year of annual carbon sequestration were estimated using the CTCC model and 5.82 Mg/year using the UFORE model (). The amount of carbon sequestered for the five most frequent tree species using the different methods is shown in .
Figure 4. Annual carbon sequestration (Mg/year) by 475 trees in Bolzano calculated using three different methods.
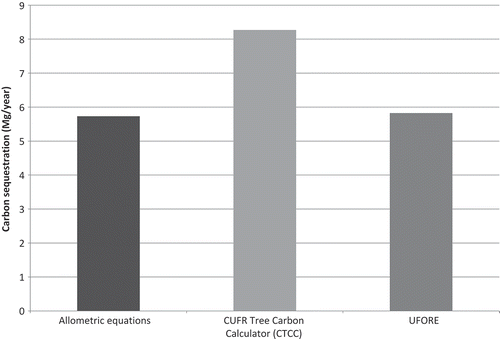
Figure 5. Average carbon sequestration (kg/year) estimates for the five most common tree species calculated using three different methods. Error bars represent ± one standard error of the mean.
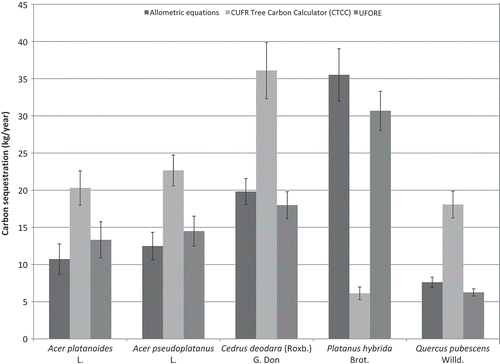
A paired t-test showed that predictions from our allometric equations were significantly lower than the CTCC model (t = −7.71, P < 0.0001). Also, there was no significant difference in estimates between the allometric equations and the UFORE model (t = −0.60, P = 0.54). However, estimates from the CTCC model were significantly higher than the UFORE model (t = 7.30, P < 0.0001) and the regression slope between the allometic equations and the CTCC model was significantly different from 1 (P < 0.0001). This suggests that the predictions from these two methods are also different. Similarly, the slope between the allometric equations and UFORE model (P =< 0.0001) and the CTCC and UFORE models (P =< 0.0001) were also significantly different from 1 (P =< 0.0001); thus model predictions are also different.
The green waste biomass from annual pruning operations of Sophora japonica L. was estimated at 678 kg/year. Assuming this biomass is burned and emitted as carbon, in the same year the trees were pruned, a potential 339 kg can be emitted per year. Since the gross annual C sequestration from trees in our subsample was 5710 kg, our net annual C sequestration (i.e. gross C sequestration minus C emitted from maintenance) is 5371 kg/year. Additionally, the 678 kg/year of dry weight biomass could be used as biofuel or as compost and thus acts as a carbon sink.
Discussion and conclusion
Our study provides a quantification of the C stored and sequestered by urban trees in an Italian city in the Southern Alps. As opposed to studies that estimate urban tree C storage and sequestration using North American models, we present an approach and protocol that primarily uses European allometric equations, city-specific measured growth rates and predicted tree height increments. In addition the study developed a protocol and compiled a list of biomass equations that can be used to estimate C storage, mean annual growth rates, and predict height increment for northern Italian urban trees at the order, division, and genera level, respectively. Finally, the study assessed the performance of two USA urban tree C models against our allometric equation approach.
Allometric equations and growth rates
The allometric equations used in our dry weight and biomass C storage estimates were developed primarily for European, forest-grown trees and were applied to 60.3% of trees in our subsample. More specifically, Italian-specific equations were applied to 51.5% of the trees in our subsample, equations from Spain and the UK were applied to 0.2% and 8.6% trees in our subsample (Bunce Citation1968; Zianis et al. Citation2005; Muukkonen & Mäkipää Citation2006; Tabacchi et al. Citation2011a, Citation2011b; Ruiz-Peinado et al. Citation2012). Due to the presence of non-native trees and lack of European-specific equations for certain species, the remaining equations were from China (4% of subsampled trees (Liu & Li Citation2012)) and North America (35.7% of subsampled trees; (Jenkins et al. Citation2003)).
Overall, our growth rates are different from those reported by Jo and McPherson (Citation1995), Iakovoglou et al. (Citation2002), and Lawrence et al. (Citation2012) for trees in the United States. The order Fagales, for example, had an AGR estimated at 0.77 cm/year which was lower than the 0.85 cm/year (average growth rates of subtropical Q. laurifolia, Q. nigra, Q. virginiana, O. virginiana) reported by Lawrence et al. (Citation2012). Also, our growth rates for hardwood trees estimated at 0.78 cm/year (Magnoliophyta) was lower than the 1.09 cm/year reported by Jo and McPherson (Citation1995), but greater for softwood trees 0.72 cm/year (Pinales) instead of 0.51 cm/year (Jo & McPherson Citation1995). Our results also differ from those reported in Strohbach et al. (Citation2012) in Leipzig, Germany, and in Bühler et al. (Citation2007) in Copenhagen, Denmark. These differences could be due to our inability to report species-specific rates and several factors such as soil and climatic conditions as well as maintenance characteristics such as irrigation and light availability.
Carbon storage and sequestration
The C storage and sequestration results from this study are difficult to assess in terms of accuracy and to compare with other studies because of the use of different estimation methodologies, climatic condition, different species composition, and urban forest structures (Jo & McPherson Citation1995; Strohbach & Haase Citation2012). Our estimates are different from those reported in . In particular, the comparison between our C estimates with the UFORE model and other European studies that have used the UFORE/i-Tree Eco model in Europe show that the average carbon storage and sequestration per tree was higher in our study than estimates reported by Wälchli (Citation2012) in Zurich in Switzerland (about 235 km from Bolzano) and Paoletti et al. (Citation2011) in Florence, Italy (about 300 km from Bolzano). The difference is likely due to Bolzano’s species composition, tree size, and health (Martin et al. Citation2012).
Table 5. Reported average per tree carbon storage and sequestration and estimation methods for case studies in Europe.
As discussed in Nowak et al. (Citation2008), the UFORE model estimates gross C sequestration using over 20 required field measurements and a series of assumptions that include non-measured root-to-shoot ratios, non-city-specific growth rates, adjustments according to tree condition, light exposure, and land use, modeled removal and decomposition rates, and no inclusion of city-specific biomass removal for maintenance-related C emissions (Escobedo et al. Citation2013; Yoon et al. Citation2013). Thus, our gross and net C sequestration estimates based on annual re-measurement data, AGRs, and predicted height increments values for Bolzano – as well as accounting for some of the observable biomass removal for maintenance-related C emissions – presents an alternative protocol based on city-specific information and fewer assumptions and parameters derived from the United States.
Model assessment
According to Jo and McPherson (Citation1995), McHale et al. (Citation2009), and Yoon et al. (Citation2013) the use of allometric biomass equations based on forest-grown trees can overestimate or underestimate urban tree biomass. For example an urban tree with the same DBH or height as a forest trees could have a different biomass due to the conditions of the urban environment relative to forest-grown trees (Jo & McPherson Citation1995).
The UFORE model reduces biomass estimates of open grown street trees by 20% based on a study of 30 street trees of nine different species in Chicago, USA (Nowak Citation1994; Yoon et al. Citation2013). However, in the case of Bolzano’s urban trees, we observed urban trees were often not open-grown, were in overall good condition, were regularly fertilized and irrigated relative to forest-grown trees. Therefore, given the uncertainty in UFORE’s assumption, we did not subtract 20% for open grown trees using our allometric equation method.
Overall, the UFORE model produced the lowest estimates (134.89 Mg) for carbon storage, and this might be because forest-based equations are used exclusively with application of the 0.8 multiplier to open-grown trees (Aguaron & McPherson Citation2012). The CUFR Tree Carbon Calculator (CTCC), however, produced an intermediate estimate of 140.15 Mg while our allometric equations produced larger estimates of 179.14 Mg. Accounting for Nowak’s (Citation1994) and Peper and McPherson (Citation1998) correction factor for open-grown urban trees, multiplying the carbon storage from our allometric equation method by a factor of 0.8 results in a carbon storage of 143.3 Mg that is more similar, yet still greater, than that estimated by the UFORE model.
The supporting evidence or verification of the application of UFORE correction factors are limited (Yoon et al. Citation2013). Yoon et al. (Citation2013) quantify the variability these correction factors can have on actual Korean urban tree biomass estimates.
The CUFR Tree Carbon Calculator (CTCC) C sequestration estimates for our subsample was the greatest at 8.27 Mg/year, while the UFORE model (5.73 Mg/year) and our equations (5.82 Mg/year) produced similar estimates. These results are similar to Escobedo et al. (Citation2013) and Aguaron and McPherson (Citation2012), who found that the UFORE model (i-Tree Eco) produced the lowest carbon storage estimate, while the CUFR Tree Carbon Calculator (CTCC) produced the largest C sequestration estimates. There are however not only statistical but also practical differences in these three methods for the calculation of C storage and sequestration as well. shows some overall strengths and weaknesses of the three carbon calculation approaches and models for Bolzano, Italy.
Table 6. Some general strengths and weaknesses for three carbon storage and sequestration estimation methods applied in Bolzano, Italy: Urban Forest Effects/Eco (UFORE), Allometric equations, and Center for Urban Forest Research Tree Carbon Calculator (CTCC).
Recommendations and applications
Further research is needed for accurate C measurements and for developing urban tree allometric equations using destructive or non-destructive approaches (Yoon et al. Citation2013). For example, it could be possible to develop urban tree equations using destructive sampling of trees removed in new developments or reconstruction sites as done by Escobedo et al. (Citation2013). The emissions of CO2 from mechanical maintenance operations can be quite variable and substantial based on the type of equipment, frequency, and intensity of maintenance activities and would require additional survey work and data collection (Strohbach et al. Citation2012), therefore we obtained only biomass removal for maintenance-related C emissions. However, this is an opportune area of future research. Further research is needed to determine the true C footprint of urban trees in Europe.
Another limitation was the calculation of the annual height and diameter increments that are not based on felled tree measurements tree cores, or re-measured height. While tree ring and stem analysis of felled trees is the most accurate method it is time-consuming and expensive, and not reasonable for urban trees. On the other hand, re-measurement of height on the same trees can have a large measurement error relative to the actual height increment (Hasenauer & Monserud Citation1997). Therefore, a modern approach to estimate urban tree heights and increments is using Light Detection and Ranging (LiDAR) data (Shrestha & Wynne Citation2012; Ramdani Citation2013). LiDAR data can be also used for estimating urban tree volume that can then be converted to biomass using specific gravity values for individual tree species (McHale et al. Citation2009).
In conclusion, our methods, findings, and model assessment can be used for integrating and assessing urban landscapes and trees into environmental design, planning, and climate-change initiatives and policies. The use of regional and European-specific biomass equations and local annual growth estimates in Italian cities can provide an alternative and arguably more reliable and transparent carbon storage and sequestration estimates than those from commonly used USA models. Findings from this study on annual growth rates, annual height increments, and model assessments can be applied to existing tree inventories and used for the development of similar protocols, model, and tools for other Italian cities or other urban areas in the Southern Alps or Europe. Similarly, green and dry weight biomass from pruning operations can be estimated and used to predict green waste yield from urban landscape maintenance activities for use as biofuel and compost, and greenhouse gas emission information from maintenance operations can also be used in green space life cycle analyses. We propose that our protocol and results from this study can be used to plan, design, and manage cities to maximize the potential of urban trees to provide ecosystem services and for developing carbon neutral policies.
Acknowledgments
We would like to thank everyone who helped during the development of this paper, in particular Dr. Ulrike Buratti (Municipality of Bolzano), Dr. Mauro Tomasi, and Prof. Giustino Tonon; this paper could not have been written without your support. The authors would like to thank the anonymous reviewers for their valuable comments and suggestions to improve the quality of the paper.
References
- Aguaron E, McPherson EG. 2012. Comparison of methods for estimating carbon dioxide storage by Sacramento’s urban forest. In: Lal R, Augustin B, editors. Carbon sequestration urban ecosyst. New York (NY): Springer; p. 43–71.
- Akbari H, Pomerantz M, Taha H. 2001. Cool surfaces and shade trees to reduce energy use and improve air quality in urban areas. Sol Energy. 70:295–310. doi:10.1016/S0038-092X(00)00089-X.
- Barnett J. 2003. Security and climate change. Glob Environ Chang. 13:7–17. doi:10.1016/S0959-3780(02)00080-8.
- Basuki TM, van Laake PE, Skidmore AK, Hussin YA. 2009. Allometric equations for estimating the above-ground biomass in tropical lowland Dipterocarp forests. For Ecol Manage. 257:1684–1694. doi:10.1016/j.foreco.2009.01.027.
- Bonatti M. 2008. Il clima di Bolzano nell’ era di Internet [Internet]. [cited 2012 Dec 10]. Available from: http://www.marcobonatti.it/nuovo/scarica/CLIMABZ_nuovo.pdf
- Brack CL. 2002. Pollution mitigation and carbon sequestration by an urban forest. Environ Pollut. 116(Suppl):S195–S200. doi:10.1016/S0269-7491(01)00251-2.
- Bühler O, Kristoffersen P, Larsen SU. 2007. Growth of street trees in Copenhagen with emphasis on the effect of different establishment concepts. Arboric Urban For. 33:330–337.
- Bunce RGH. 1968. Biomass and production of trees in a mixed deciduous woodland: I. girth and height as parameters for the estimation of tree dry weight. J Ecol. 56:759–775. doi:10.2307/2258105.
- Cairns MA, Brown S, Helmer EH, Baumgardner GA. 1997. Root biomass allocation in the world’s upland forests. Oecologia. 111:1–11. doi:10.1007/s004420050201.
- Comune di Bolzano. 2012. Censimento della popolazione 2011 [Internet]. [cited 2012 Sep 1]. Available from: http://www.comune.bolzano.it/context.jsp?ID_LINK=3980&area=154
- Crema S. 2008. Urban forestry e stima del carbonio: analisi di linee guida e calcolo in zona urbana come applicazione ed opportunità per l’ università di Padova. Padova: Università degli studi di Padova.
- Davies ZG, Edmondson JL, Heinemeyer A, Leake JR, Gaston KJ. 2011. Mapping an urban ecosystem service: quantifying above-ground carbon storage at a city-wide scale. J Appl Ecol. 48:1125–1134. doi:10.1111/j.1365-2664.2011.02021.x.
- Dobbs C, Escobedo FJ, Zipperer WC. 2011. A framework for developing urban forest ecosystem services and goods indicators. Landsc Urban Plan. 99:196–206. doi:10.1016/j.landurbplan.2010.11.004.
- Escobedo F, Chappelka A, Staudhammer C, Mayer H. 2013. Evaluating the use of i-Tree ECO in the Southeastern US. Florida Arborist. 16:1–19.
- Escobedo F, Varela S, Zhao M, Wagner JE, Zipperer W. 2010. Analyzing the efficacy of subtropical urban forests in offsetting carbon emissions from cities. Environ Sci Policy. 13:362–372. doi:10.1016/j.envsci.2010.03.009.
- Escobedo FJ, Kroeger T, Wagner JE. 2011. Urban forests and pollution mitigation: analyzing ecosystem services and disservices. Environ Pollut. 159:2078–2087. doi:10.1016/j.envpol.2011.01.010.
- Ferrini F, Fini A. 2011. Sustainable management techniques for trees in the urban areas. J Biodivers Ecol Sci. 1:1–19.
- Fleming LE. 1988. Growth estimates of street trees in central New Jersey [dissertation]. New Brunswick (NJ): Rutgers University.
- Grimm NB, Faeth SH, Golubiewski NE, Redman CL, Wu J, Bai X, Briggs JM. 2008. Global change and the ecology of cities. Science. 319:756–760. doi:10.1126/science.1150195.
- Hasenauer H, Monserud RA. 1997. Biased predictions for tree height increment models developed from smoothed ‘data’. Ecol Modell. 98:13–22. doi:10.1016/S0304-3800(96)01933-3.
- Henry M, Bombelli A, Trotta C, Alessandrini A, Birigazzi L, Sola G, Vieilledent G, Santenoise P, Longuetaud F, Valentini R. et al., 2013. GlobAllomeTree: international platform for tree allometric equations to support volume, biomass and carbon assessment. iForest – Biogeosci For. 6:326–330. doi:10.3832/ifor0901-006.
- Hutyra LR, Yoon B, Alberti M. 2011. Terrestrial carbon stocks across a gradient of urbanization: a study of the Seattle, WA region. Glob Chang Biol. 17:783–797. doi:10.1111/j.1365-2486.2010.02238.x.
- Iakovoglou V, Thompson J, Burras L. 2002. Characteristics of trees according to community population level and by land use in the U.S. midwest. J Arboric. 28:59–69.
- IPCC. 2007. Climate change 2007 – the physical science basis: working group I contribution to the fourth assessment report of the IPCC (climate change 2007). In: Solomon S, Qin D, Manning M, Chen Z, Marquis M, Averyt KB, Tignor M, Miller HL, editors. Cambridge (UK): Cambridge University Press. Available from: http://www.ipcc.ch/publications_and_data/ar4/wg1/en/contents.html
- ISPRA. 2012. Rapporto Rifiuti Urbani 2012. Roma (Italy): Istituto Superiore per la Protezione e la Ricerca Ambientale.
- i-Tree. 2012a. i-Tree reports [Internet]. [cited 2012 Nov 1]. Available from: http://www.itreetools.org/resources/reports.php
- i-Tree. 2012b. i-Tree applications [Internet]. [cited 2012 Sep 5]. Available from: http://www.itreetools.org/applications.php
- Jenkins JC, Chojnacky DC, Heath LS, Birdsey RA. 2003. National-scale biomass estimators for United States tree species. For Sci. 49:12–35.
- Jo H, McPherson GE. 1995. Carbon storage and flux in urban residential greenspace. J Environ Manage. 45:109–133. doi:10.1006/jema.1995.0062.
- Karagiannis IC, Soldatos PG. 2010. Estimation of critical CO2 values when planning the power source in water desalination: the case of the small Aegean islands. Energy Policy. 38:3891–3897. doi:10.1016/j.enpol.2010.03.009.
- Laar A, van Akça A. 2007. Forest mensuration. Dordrecht: Springer Netherlands.
- Lawrence AB, Escobedo FJ, Staudhammer CL, Zipperer W. 2012. Analyzing growth and mortality in a subtropical urban forest ecosystem. Landsc Urban Plan. 104:85–94. doi:10.1016/j.landurbplan.2011.10.004.
- Liu C, Li X. 2012. Carbon storage and sequestration by urban forests in Shenyang, China. Urban For Urban Greening. 11:121–128. doi:10.1016/j.ufug.2011.03.002.
- Maes J, Egoh B, Willemen L, Liquete C, Vihervaara P, Schägner JP, Grizzetti B, Drakou EG, La Notte A, Zulian G. et al. 2012. Mapping ecosystem services for policy support and decision making in the European Union. Ecosyst Serv. 1:31–39. doi:10.1016/j.ecoser.2012.06.004.
- Martin NA, Chappelka AH, Loewenstein EF, Keever GJ. 2012. Comparison of carbon storage, carbon sequestration, and air pollution removal by protected and maintained urban forests in Alabama, USA. Int J Biodivers Sci, Ecosyst Serv Manag. 8:265–272. doi:10.1080/21513732.2012.712550.
- Marziliano PA, Lafortezza R, Colangelo G, Davies C, Sanesi G. 2013. Structural diversity and height growth models in urban forest plantations: a case-study in northern Italy. Urban For Urban Greening. 12:246–254. doi:10.1016/j.ufug.2013.01.006.
- McHale MR, Burke IC, Lefsky MA, Peper PJ, McPherson EG. 2009. Urban forest biomass estimates: is it important to use allometric relationships developed specifically for urban trees? Urban Ecosyst. 12:95–113. doi:10.1007/s11252-009-0081-3.
- McPherson EG. 2010. Selecting reference cities for i-Tree streets. Arboric Urban For. 36:230–240.
- McPherson EG, Peper PJ. 2012. Urban tree growth modeling. Arboric Urban For. 38:172–180.
- Muukkonen P, Mäkipää R. 2006. Biomass equations for European trees: addendum. Silva Fenn. 40:763–773.
- Natural England. 2013. Green infrastructure – valuation tools assessment. Exeter (UK): Natural England. (Natural England Commissioned Report NECR126.)
- Nowak DJ. 1994. Atmospheric carbon dioxide reduction by Chicago’s urban forest. In: McPherson EG, Nowak DJ, Rowntree R, editors. Chicago’s urban for ecosyst results Chicago urban for clim proj gen tech rep NE-186. Radnor: U.S. Department of Agriculture, Forest Service, Northeastern Forest Experiment Station.
- Nowak DJ, Crane DE. 2000. The Urban Forest Effects (UFORE) model: quantifying urban forest structure and functions. In: Hansen M, Burk T, editors. Integr tools Nat Resour Invent 21st Century; Proceedings of the IUFRO Conference. St. Paul (MN): U.S. Department of Agriculture, Forest Service, North Central Research Station; p. 714–720.
- Nowak DJ, Crane DE. 2002. Carbon storage and sequestration by urban trees in the USA. Environ Pollut. 116:381–389. doi:10.1016/S0269-7491(01)00214-7.
- Nowak DJ, Crane DE, Stevens JC, Hoehn RE, Walton JT, Bond J. 2008. A ground-based method of assessing urban forest structure and ecosystem services. Arboric Urban For. 34:347–358.
- Nowak DJ, Crane DE, Stevens JC, Ibarra M. 2002. Brooklyn’s urban forest. Newtown Square (PA): Northeastern Research Station, United States Department of Agriculture, Forest Service, Borough of Brooklyn. (General Technical Report NE-290.)
- Nowak DJ, Kuroda M, Crane DE. 2004. Tree mortality rates and tree population projections in Baltimore, Maryland, USA. Urban For Urban Greening. 2:139–147. doi:10.1078/1618-8667-00030.
- Paoletti E, Bardelli T, Giovannini G, Pecchioli L. 2011. Air quality impact of an urban park over time. Procedia Environ Sci. 4:10–16. doi:10.1016/j.proenv.2011.03.002.
- Peper PJ, McPherson EG. 1998. Comparision of four foliar and woody biomass estimation methods applied to open-grown deciduous trees. J Arboric. 24:191–199.
- Piano Urbanistico Comunale. 2012. PUC – Piano Urbanistico Comunale [Internet]. [cited 2012 Dec 5]. Available from: http://www.comune.bolzano.it/urb_context02.jsp?ID_LINK=1206&page=2&area=74&id_context=8876
- Pretzsch H. 2009. Forest growth models. In: Gramm U, Eckey C, editors. For dyn growth yield. Berlin: Springer; p. 423–491.
- Provincia Autonoma di Bolzano. 2012. Servizio meteorologico della Provincia Autonoma di Bolzano, Dati storici [Internet]. [cited 2012 Dec 20]. Available from: http://www.provincia.bz.it/meteo/dati-storici.asp
- Ramdani F. 2013. Urban vegetation mapping from fused hyperspectral image and LiDAR data with application to monitor urban tree heights. J Geogr Inf Syst. 05:404–408.
- Roy S, Byrne J, Pickering C. 2012. A systematic quantitative review of urban tree benefits, costs, and assessment methods across cities in different climatic zones. Urban For Urban Greening. 11:351–363. doi:10.1016/j.ufug.2012.06.006.
- Ruiz-Peinado R, Montero G, Del Rio M. 2012. Biomass models to estimate carbon stocks for hardwood tree species. For Syst. 21:42–52.
- Russo A. 2013. Quantifying and modeling ecosystem services provided by urban greening in cities of the Southern Alps, N Italy [dissertation]. Bologna: University of Bologna.
- Rydin Y, Bleahu A, Davies M, Dávila JD, Friel S, De Grandis G, Groce N, Hallal PC, Hamilton I, Howden-Chapman P. et al. 2012. Shaping cities for health: complexity and the planning of urban environments in the 21st century. The Lancet. 379:2079–2108. doi:10.1016/S0140-6736(12)60435-8.
- Sajdak M, Velazquez-Marti B. 2012. Estimation of pruned biomass form dendrometric parameters on urban forests: case study of Sophora japonica. Renew Energy. 47:188–193. doi:10.1016/j.renene.2012.04.002.
- Sanesi G, Lafortezza R, Marziliano PA, Ragazzi A, Mariani L. 2007. Assessing the current status of urban forest resources in the context of Parco Nord, Milan, Italy. Landsc Ecol Eng. 3:187–198. doi:10.1007/s11355-007-0031-2.
- Semenzato P, Cattaneo D, Dainese M. 2011. Growth prediction for five tree species in an Italian urban forest. Urban For Urban Greening. 10:169–176. doi:10.1016/j.ufug.2011.05.001.
- Servizio Giardineria. 2013. Giardineria Comunale di Bolzano [Internet]. [cited 2013 Jan 20]. Available from: http://www.comune.bolzano.it/ambiente_context02.jsp?hostmatch=true&ID_LINK=1845&area=69
- Shrestha R, Wynne RH. 2012. Estimating biophysical parameters of individual trees in an urban environment using small footprint discrete-return imaging Lidar. Remote Sens. 4:484–508. doi:10.3390/rs4020484.
- Sklar F, Ames RG. 1985. Staying alive: street tree survival in the inner city. J Urban Aff. 7:55–66. doi:10.1111/j.1467-9906.1985.tb00077.x.
- Smith WB, Shirley SR. 1984. Diameter growth, survival, and volume estimates for trees in Indiana and Illinois. St. Paul (MN): U.S. Department of Agriculture, Forest Service, North Central Forest Experiment Station. (Res. Pap. NC-257.)
- Soares AL, Rego FC, McPherson EG, Simpson JR, Peper PJ, Xiao Q. 2011. Benefits and costs of street trees in Lisbon, Portugal. Urban For Urban Greening. 10:69–78. doi:10.1016/j.ufug.2010.12.001.
- Sparber W, Fedrizzi R, Avesani S, Exner D, Mahlknecht H. 2010. Calcolo e valutazione delle emissioni di CO2 e definizione di scenari di riduzione per la città di Bolzano. Bolzano: European Academy of Bozen/Bolzano (EURAC).
- Stoffberg GH, van Rooyen MW, van der Linde MJ, Groeneveld HT. 2008. Predicting the growth in tree height and crown size of three street tree species in the City of Tshwane, South Africa. Urban For Urban Greening. 7:259–264. doi:10.1016/j.ufug.2008.05.002.
- Strohbach MW, Arnold E, Haase D. 2012. The carbon footprint of urban green space—a life cycle approach. Landsc Urban Plan. 104:220–229. doi:10.1016/j.landurbplan.2011.10.013.
- Strohbach MW, Haase D. 2012. Above-ground carbon storage by urban trees in Leipzig, Germany: analysis of patterns in a European city. Landsc Urban Plan. 104:95–104. doi:10.1016/j.landurbplan.2011.10.001.
- Tabacchi G, Di Cosmo L, Gasparini P. 2011a. Aboveground tree volume and phytomass prediction equations for forest species in Italy. Eur J For Res. 130:911–934. doi:10.1007/s10342-011-0481-9.
- Tabacchi G, Di Cosmo L, Gasparini P, Morelli S. 2011b. Stima del volume e della fitomassa delle principali specie forestali italiane. Equazioni di previsione, tavole del volume e tavole della fitomassa arborea epigea. Trento: Consiglio per la Ricerca e la Sperimentazione in Agricoltura – Unità di Ricerca per il Monitoraggio e la Pianificazione Forestale.
- Urban Forest Project Reporting Protocol. 2008. Urban forest project reporting protocol [Internet]. [cited 2012 Nov 15];100. Available from: http://www.fs.fed.us/psw/programs/uesd/uep/UrbanForestProtocol0812081ForBoardApproval.pdf
- USDA. 2013. PLANTS classification [Internet]. [cited 2013 Nov 22]. Available from: http://plants.usda.gov/classification.html
- Wälchli G. 2012. Ökosystemdienstleistungen als ökonomische Strategie? i-Tree: ein Instrument für die Wertermittlung von Stadtbäumen Zusammenfassung [dissertation]. Wädenswil: Zürcher Hochschule für Angewandte Wissenschaften.
- Yoon TK, Park C-W, Lee SJ, Ko S, Kim KN, Son Y, Lee KH, Oh S, Lee W-K, Son Y. 2013. Allometric equations for estimating the aboveground volume of five common urban street tree species in Daegu, Korea. Urban For Urban Greening. 12:344–349. doi:10.1016/j.ufug.2013.03.006.
- Zapata-Cuartas M, Sierra CA, Alleman L. 2012. Probability distribution of allometric coefficients and Bayesian estimation of aboveground tree biomass. For Ecol Manage. 277:173–179. doi:10.1016/j.foreco.2012.04.030.
- Zhao M, Kong Z, Escobedo FJ, Gao J. 2010. Impacts of urban forests on offsetting carbon emissions from industrial energy use in Hangzhou, China. J Environ Manage. 91:807–813. doi:10.1016/j.jenvman.2009.10.010.
- Zianis D, Muukkonen P, Mäkipää R, Mencuccini M. 2005. Biomass and stem volume equations for tree species in Europe. Silvia Fenn Monogr. 4:1–63.
Appendix 1
Table S1. Sources and geographical distribution of allometric equations that were used for biomass calculation in Bolzano, Italy.
Appendix 2
Table S2. Tree species from Center for Urban Forest Research Tree Carbon Calculator (CTCC) and United States’ climate zones assigned to each species in the Bolzano, Italy inventory.