Abstract
Follicular Lymphomas (FL) and diffuse large B cell lymphomas (DLBCL) must evolve some immune escape strategy to develop from lymphoid organs, but their immune evasion pathways remain poorly characterized. We investigated this issue by transcriptome data mining and immunohistochemistry (IHC) of FL and DLBCL lymphoma biopsies. A set of genes involved in cancer immune-evasion pathways (Immune Escape Gene Set, IEGS) was defined and the distribution of the expression levels of these genes was compared in FL, DLBCL and normal B cell transcriptomes downloaded from the GEO database. The whole IEGS was significantly upregulated in all the lymphoma samples but not in B cells or other control tissues, as shown by the overexpression of the PD-1, PD-L1, PD-L2 and LAG3 genes. Tissue microarray immunostainings for PD-1, PD-L1, PD-L2 and LAG3 proteins on additional biopsies from 27 FL and 27 DLBCL patients confirmed the expression of these proteins. The immune infiltrates were more abundant in FL than DLBCL samples, and the microenvironment of FL comprised higher rates of PD-1+ lymphocytes. Further, DLBCL tumor cells comprised a higher proportion of PD-1+, PD-L1+, PD-L2+ and LAG3+ lymphoma cells than the FL tumor cells, confirming that DLBCL mount immune escape strategies distinct from FL. In addition, some cases of DLBCL had tumor cells co-expressing both PD-1, PD-L1 and PD-L2. Among the DLBCLs, the activated B cell (ABC) subtype comprised more PD-L1+ and PD-L2+ lymphoma cells than the GC subtype. Thus, we infer that FL and DLBCL evolved several pathways of immune escape.
Abbreviations:
- ABC subtype DLBCL, activated B cell origin of DLBCL
- DLBCL, diffuse large B cell lymphoma
- ECDF, empirical cumulative distribution function
- FFPE, formalin-fixed paraffin-embedded
- FL, follicular lymphoma
- FLIPI, Follicular Lymphoma International Prognostic Index
- GC subtype DLBCL, germinal center B cell origin of DLBCL
- GELF, Groupe d'Etude des Lymphomes Folliculaires
- HLA, human leucocyte antigen; IHC, immunohistochemistry
- KS, Kolmogorov–Smirnov
- NA, Not available
- R-CHOP, rituximab cyclophosphamide, doxorubicin, vincristine and prednisone
- TAMs, tumor associated macrophage; TMA, tissue microarray
Introduction
In order to develop within immunocompetent hosts, it is imperative that tumors evolve an immune escape strategy.Citation1-3 This Darwinian selection may operate not only through emergence of somatic mutations which protect against immunity, but also by upregulation of genes mediating immune escape. Indeed, genetic instability stands on a passive and random basis while regulation of gene expression represents an active evolution strategy. Different mechanisms leading to immune-evasion have been identified, and include the impairment of immune infiltration through endothelial defects, the inhibition or blockade of immune activation by galectin 3 or CTLA4 ligands,Citation4-7 metabolic depletion by indoleamine dioxygenaseCitation8-10 or arginase, the secretion of suppressive mediators such as PGE2, TGFβ, IL10 and adenosine,Citation11 the local recruitment of immunosuppressive cells such as Tregs, TAMs or myeloid-derived suppressor cells (MDSC), and the impairment of functional responses from pre-activated lymphocytes through apoptotic depletion by FasL, PD-L1 or PD-L2, as well as exhaustion or anergy, to name but a few. Among these different options, tumor cells particularly need to develop protection against T-cell-mediated immune responses, and indeed some aggressive B cell lymphomas frequently delete HLA class I or β2-microglobulin genes accordingly.Citation12 Inhibitory ligands and receptors that regulate T cell effector functions in normal tissues are expressed by non-transformed cells to ensure self-tolerance, but as a consequence, the upregulated expression of such immune checkpoint proteins efficiently allows the immune-evasion of tumor cells.Citation2,13
Programmed death-1 (PD-1, CD279) is an immune-suppressive molecule that is upregulated in a large proportion of tumor-infiltrating lymphocytes (TILs) in many different tumor types,Citation14 but it is also upregulated in other immune cells. Intracellular PD-1 signaling is activated upon PD-1 binding to its ligands PD-L1 (B7-H1, CD274) or PDL2 (PDCD1LG2, CD273), which induce a reduction in the T cell activation cascade.Citation15,16 PD-L1 is expressed by many human solid cancers, by certain B cell lymphomas such as primary mediastinal B cell lymphomas and Hodgkin's disease, and by myeloid cells in the tumor microenvironment.Citation17-22 The other PD-1 ligand is PD-L2, which is highly upregulated in cells from certain B cell lymphomas such as primary mediastinal B cell lymphomas and Hodgkin's disease.Citation19,23 Thus, by expressing PD-1 ligands on the tumor cell surface and engaging PD-1-positive infiltrating lymphocytes, tumors utilizing the PD-1 pathway can therefore evade an immune response. Accordingly, a blockade of the PD-1/PD-L1 interaction has led to good clinical responses in several but not all cancer types, and the heterogeneity observed in the cellular expression of PD-1/PD-L1 may underlie these varied responses.Citation24-26
Lymphocyte activation gene 3 (LAG3; also known as CD223) is an immunosuppressive molecule highly expressed on Tregs.Citation27 It is activated upon binding to MHC class II molecules, inducing the inhibition of T lymphocyte activity and eventually their anergy. It has been shown that LAG3 is upregulated in some epithelial cancers but it is also expressed in tumor-infiltrating macrophages and dendritic cells.Citation28 Hence, there are many means by which tumors may escape immune recognition and destruction.
Mutations and deletions of genes involved in immune responses have been characterized for FL and DLBCL but the changes in gene expression adopted by these lymphomas for the purpose of immune escape have remained poorly characterized so far. To investigate this issue, we analyzed mRNA co-expression levels of 54 immune escape genes in samples of FL, DLBCL and normal B cell controls using the NCBI's GEO database, and found a significant enrichment of these genes in most of the lymphomas tested. IHC analysis of the PD-1, PD-L1, PD-L2 and LAG3 markers in an independent cohort of biopsies from FL and DLBCL tumors confirmed the co-expression of these immune checkpoint proteins by both the lymphoma cells and the cells in their immune microenvironment. Our study reveals that most FL and DLBCL lymphomas have evolved a variety of methods for immune escape that involve several different pathways.
Results
FL and DLBCL upregulate the expression of immune escape genes
By collating the literature, we were able to define a non-exhaustive set of 54 genes involved in different pathways of cancer immune escape (Immune Escape Gene Set, IEGS) (). We analyzed the distribution of these genes in transcriptomes from lymphoma samples. First, we ranked the IEGS genes from each transcriptome in decreasing order of expression, and then analyzed the distribution of these ranks by plotting and testing their empirical cumulative distribution function (ECDF). For a subset extracted from a random permutation (null hypothesis), the ECDF approaches the identity function, and the plot is close to the diagonal. Hence, genes in the IEGS are revealed to be collectively upregulated when the ECDF plot is above the diagonal or downregulated when below the diagonal. We also needed to compare two ECDF's, and test whether they were significantly different, without reference to the identity function. For statistical procedures, a homogeneous choice was made for both cases: either the one-sample Kolmogorov–Smirnov (KS) test for the first case (comparison of one ECDF with the identity function) or the two-sample KS test for the second case (comparison of two ECDFs). We then downloaded a series of transcriptomes from 38 samples of FL and 20 samples of the corresponding normal B cell controls from the NCBI GEO dataset GSE12195.Citation29 The mean of transcriptomes from each group of samples (control B cells, FL) was calculated as arithmetic mean of each gene expression value. In a preliminary control test, a set of 54 randomly chosen genes was defined and its distribution was tested as above for the mean of control B cell group and the mean of FL cell group (blue and red, respectively, ). There was no significant difference between these two ECDFs, indicating no upregulation for a random gene set in these two groups. The same KS test involving the same samples as above was then applied to the IEGS instead of the random gene set. In contrast to the previous result, the expression of the IEGS was significantly upregulated in the FL group when compared to the control B cell group (p = 0.0075, ). The IEGS genes that were significantly upregulated in FL versus normal B cells were: TIMP1, CCL2, TIGIT, CTLA4, IDO1, VEGFA, CD163, CSF1, IL6ST, LGALS3, MRC1, LGALS1, ICOS, IL10, PDCD1, SOCS3, CCL22, MCL1, CD274(PDCD1LG1), JAK2, HAVCR2, LAG3, IDO2, PDCD1LG2, PTGS2 and GDF15 (p values from 5.10−16 to 2.10−2).
Figure 1. ECDFs of the (normalized) orders of immune escape genes in transcriptomes from FL and DLBCL biopsies. Each plot represents the appearance order of the IEGS genes in transcriptomes from the specified cell type (depicted as ECDF). The ECDFs of a random gene set show similar random distributions in transcriptomes from normal B cell controls (blue: mean from n = 20 samples) or FL cells (red: mean from n = 38 samples) (A), unlike the ECDFs of the IEGS which evidence a significantly up-regulated expression in the FL samples (red) compared to the normal B cell controls (blue) (B). The distribution of a random gene set is uniform and not different in transcriptomes from normal B cells (blue) and DLBCL cells (red)(C), while that of IEGS shows significant upregulation in the transcriptomes from DLBCL samples when compared to the normal B cell controls (red curve: mean from n = 73 DLBCL samples) from the same study (D) (p values for lymphoma vs. normal group comparisons) (data for A–D comes from the NCBI GEO dataset GSE12195Citation29). The ECDF plots for control human tissues show no upregulation of the IEGS (E) in lymph nodes, tonsils, spleen, skin, kidney, pancreas, colon and breast (means from n = 3–9 samples, p values for tissue vs. diagonal), (NCBI GEO data set GSE7307Citation31). ECDFs of the IEGS from individual samples (F) of normal B cell controls (n = 20), FL biopsies (n = 38), DLBCL biopsies (n = 73) (GEO dataset GSE12195Citation29), multiple myeloma (MM, n = 12) (NCBI GEO data set GSE6691Citation33), and chronic lymphocytic leukemia (CLL, n = 188) (NCBI GEO dataset GSE31048Citation32).
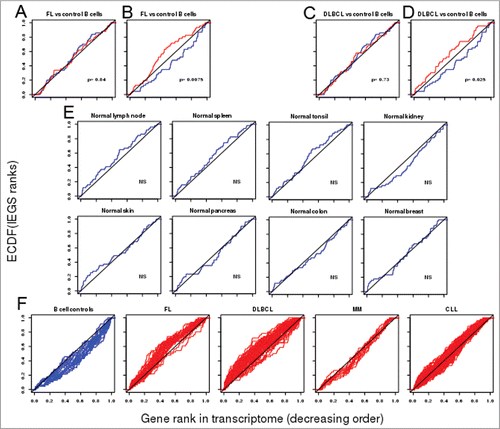
Table 1. List of 54 genes of IEGS
Similar results were obtained when we likewise tested 73 samples of DLBCL and 20 normal B cell controls, both downloaded from the same GEO data set. The random gene set was uniformly distributed along both groups of transcriptomes (), whereas the IEGS distribution was not uniform for the DLBCLs and revealed significant upregulation in the DLBCL group relative to the B cell control group (p = 0.025) (). The IEGS genes significantly upregulated in DLBCL vs. normal B cells were, in decreasing order of magnitude: TIMP1, LGALS3, CCL2, IL6ST, LGALS1, CD163, IDO1, VEGFA, CSF1, IL10, LAG3, MRC1, CTLA4, CD274 (PDCD1LG1), TIGIT, SOCS3, PDCD1LG2, PVR, HGF, GDF15, IDO2, HAVCR2, MSR1, JAK2, LAIR1, CCL22, MCL1, PDCD1, and PIM1 (p values from 5.10−12 to 3.10−2).
The above results evidencing significant upregulation of IEGS in FL and DLBCL samples from the Compagno study's cohortCitation29 were confirmed when testing as above another cohort of FL samples (p = 0.032) and DLBCL (p = 0.032).Citation30 Since both groups of lymphomas showed a significant upregulation of the IEGS, we wondered whether this gene set was also enriched in unrelated samples of lymphoid and non-lymphoid control tissues. Transcriptomes from normal human tissues were downloaded from the NCBI GEO dataset GSE7307Citation31 and the IEGS was analyzed as above. The uniform distribution of the IEGS ECDF indicated that the IESG genes were not upregulated in normal lymph nodes, tonsils, spleen, colon, breast, kidney skin and pancreas ().
We then asked whether the pattern of IEGS upregulation was homogeneous among the individual FL and DLBCL samples. The individual IEGS ECDF plots of all the normal B cell samples were below the diagonal, indicating that none of these had upregulated expression of the IEGS. In contrast, the same type of analysis indicated that most of the individual FL and DLBCL samples had upregulated expression. Outliers were scarce in these two lymphoma cohorts, indicating that the upregulation of immune escape genes is a common occurrence in these diseases (). Analysis of an independent DLBCL cohort from The Cancer Genome Atlas confirmed that 78% of individual DLBCL samples had upregulated mRNA expression of genes from IEGS. The analysis of additional cohorts from other cancers evidenced an individual variation of IEGS expression among patients with chronic lymphocytic leukemia (CLL) (NCBI GEO data set GSE31048Citation32), and no significant upregulation of the IEGS in samples from multiple myeloma patients (NCBI GEO dataset GSE6691Citation33) (). In contrast to FL and DLBCL, the most upregulated IEGS genes from other cancers comprised CTLA4, TIGIT, KIR2DL1, KIR2DL2, KIR2DL3, BTLA and IL4 in CLL, and FOXP3, TIMP1, COL17A1 PVR, PDCD1, IDO2 and VEGFA in lung carcinomas (not shown).
In summary, the upregulation of 26–30 IEGS genes in FL and DLBCL is likely to reflect selection of multiple mechanisms comprising the CTLA4 and PD-1/PD-L1–2 inhibitory axes, the LAG3 and TIM3/galectins exhaustion axes, the production of the immunosuppressive molecules IDO, IL10, GDF15 and CSF1, and the recruitment of immunosuppressive cells by CCL2, CCL22 and CSF1, among others. We thus speculate that the gene and protein expression patterns of FL and DLBCL indicates these malignancies evolved a combination of several immune escape pathways.
Distribution of the PD-1, PD-L1, PD-L2 and LAG3 proteins in biopsies of FL and DLBCL tumors
In the above analyses, the mRNA expression of most IEGS genes was significantly upregulated. For example among these genes, PDCD1, encoding PD-1, was up-regulated 1.4-fold in FL samples relative to controls (p < 10−9) and 1.1-fold in DLBCL relative to controls (p < 0.05); PDCD1-LG1, encoding PD-L1/CD274, was upregulated 1.3-fold in FL samples relative to controls (p < 10−3) and 1.7-fold in DLBCL relative to controls (p < 10−7); PDCD1-LG2, encoding PD-L2, was upregulated 1.5-fold in FL samples relative to controls (p < 10−10) and 2.5-fold in DLBCL relative to controls (p < 10−11); and the LAG3 gene was upregulated 2.1-fold in FL samples relative to controls (p < 10−8) and 3.4-fold in DLBCL relative to controls (p < 10−13). This slight-but-significant upregulation could result from the increased expression of these immune checkpoints by malignant cells as well as by immune cells from the lymphoma samples.
To formally validate the actual presence of these immune checkpoints in FL and DLBCL samples in an independent cohort, we analyzed the expression of the PD-1, PD-L1, PD-L2 and LAG3 proteins using specific antibodies and IHC analysis of tissue microarrays (TMA) comprising 54 biopsy samples obtained from 27 DLBCL and 27 FL patients recently diagnosed at Institut Universitaire du Cancer-Oncopole de Toulouse (). FL samples were divided into two groups according to the WHO classificationCitation34: those graded 1–2 FL (85% of FL samples) and those graded 3 FL (15% of FL samples). The two main subtypes of DLBCL, namely GC and ABC (26% and 74% of the DLBCL cases, respectively) were classified using the Hans algorithm.Citation35 Expression of the PD-1, PD-L1, PD-L2 and LAG3 proteins was visually inspected on each of the tumor cells from the 27 DLBCL and 27 FL samples and was quantified in terms of percentage of stained cells and intensity of staining (scored 1+, 2+, 3+). In FL samples, tumors harbored a predominantly follicular pattern, and the neoplastic follicles were composed of small- to medium-sized centrocyte-like cells associated with a variable number of large centroblasts (). None of the FL cases expressed PD-1 (), PD-L1 () or LAG3 () on the lymphoma cells themselves. However, most FL samples contained a rich immune infiltrate of PD-1+ cells, as illustrated in . These cells were mostly located in the inter-follicular areas (Fig. 2C). A few cases expressed PD-1+ diffusely with PD-1+ T cells inside follicles (). Likewise, the FL samples contained PD-L1+ (), PD-L2+ () or LAG3+ () immune cell infiltrates which were mostly located in the inter-follicular areas. We also noticed that some FL samples comprised some rare tumor cells which weakly expressed PD-L2 ().\raster="rgFigKONI_A_1026530_F0002_B"
Figure 2. IHC of immune escape markers expressed by FL. (A and B) Classical H&E stainings of FL biopsies (A: FL grades 1–2, ×100, B: FL grade 3, ×100) reveal small and atypical lymphoid cells with a round nucleus and an irregular membrane. (C–H) Representative IHC of FL samples stained for: PD-1 (C: ×100, D: ×200); PD-L1 (E: ×100, F: ×40), PD-L2 (G: ×100), and LAG3 (H: ×100).
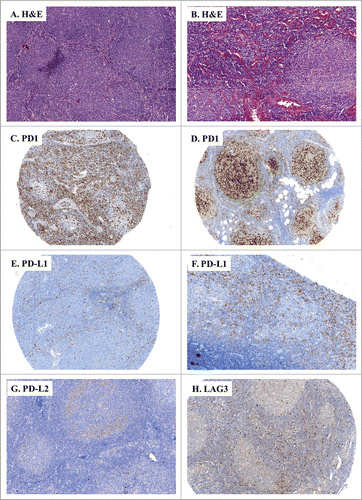
Table 2. Clinical characteristics of FL and DLBCL patients
In the DLBCL tumor biopsies, we observed a different pattern of expression for PD-1, PD-L1, PD-L2 and LAG3. Indeed, DLBCL harbored a diffuse proliferation of large lymphoid cells admixed with variable numbers of inflammatory cells (). These biopsies comprised tumor cells which expressed PD-1 (), PD-L1 (), PD-L2 () and LAG3 (). In addition, their microenvironment also comprised a variable proportion of PD-1+, PD-L1+, PD-L2+ and LAG3+ immune cell infiltrates.
Figure 3. IHC of immune escape markers expressed by DLBCL. (A and B) Classical H&E stainings of DLBCL biopsies (A: ×200, B: ×100) reveal large-sized and atypical lymphoid cells harbouring a round nucleus and an irregular membrane. (C–H) Representative IHC of DLBCL samples stained for: PD-1 (C: ×100, D: ×200); PD-L1 (E: ×100, F: ×150), PD-L2 (G: ×100), and LAG3 (H: ×100).
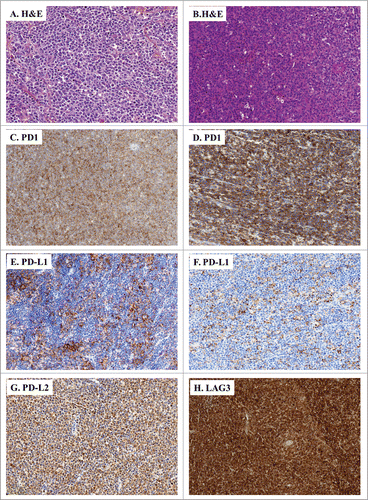
Thus, in line with the previous data-mining results, these IHC analyses validate the conclusion that both FL and DLBCL tumors express immune escape checkpoint genes. Furthermore, IHC revealed that whereas FLs carry such markers almost exclusively through their immune microenvironment, the DLBCLs harbor these checkpoints not only on their microenvironment but also and even mostly on the tumor cells themselves (see below).
Quantitative analysis of PD-1, PD-L1, PD-L2 and LAG3 protein expression in FL and DLBCL tumor cells and their respective immune infiltrates
In the above visual examinations of the FL and DLBCL samples, we quantified the proportion of tumor cells that were positively stained and scored the intensity of their PD-1+, PD-L1+, PD-L2+ and LAG3+ stainings ().
Figure 4. Rates of lymphoma cells expressing immune escape markers in DLBCL and FL biopsies. (A–D) Shown are the rates of tumor cells found positive for the specified IHC stainings upon visual examination of the FL (n = 27) and DLBCL (n = 27) samples: PD-1+ (A), PD-L1+ (B), PD-L2+ (C), LAG3+ (D). * indicates significant differences between groups (p = 0.01; p = 0.0003; p = 0.0002; p = 0.003, respectively), Wilcoxon–Mann–Whitney tests.
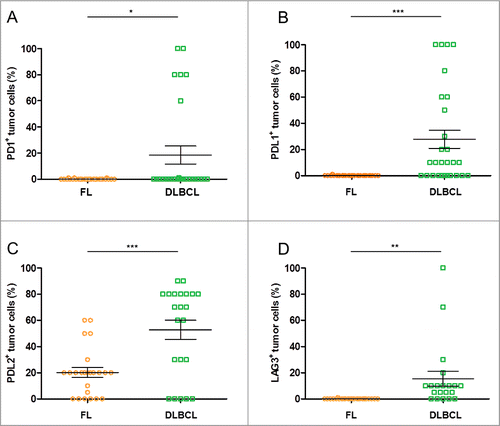
As depicted above, FL tumor cells did not express PD-1, PD-L1 and LAG3. Only the PD-L2 protein was detected in 75% of the FL samples, in which it was expressed on average by 27% of tumor cells (range: 5–60%) with a staining intensity score of 1+.
In DLBCL, tumor cells expressed these immune checkpoint proteins at different levels. We found that 22% of the DLBCL cases expressed PD-1, and in these cases it was expressed on average by 80% (range: 60–100%) of the tumor cells with a staining intensity score of 3+. We also observed that 61% of DLBCL cases were PD-L1+, and this was expressed on average by 46% of tumor cells (range: 10–100%) with a staining intensity score of 1+. Further, 81% of the DLBCL cases expressed PD-L2, which was expressed on average by 68% of tumor cells (range: 30–90%) with a staining intensity score of 2+. Finally, 85% of the DLBCL cases were LAG3+, which was expressed on average by 20% of tumor cells (range: 5–100%) with a staining intensity score of 1+.
We then analyzed immune infiltrates in the non-Hodgkin's lymphomas (NHL) samples comparatively to those of samples of tonsil controls. In most FLs, the immune infiltrates expressed PD-L1, PD-L2 and LAG3 at normal levels (i.e., in 5 to 10% of cells), while PD-1 was notably overexpressed (80% of FL cases were stained with an intensity score of 3+) and more intensely stained than in extra-follicular area of tonsils. This staining was also more frequent and more intense than in DLBCL samples (25% of immune cells in FL vs. 10% of immune cells in DLBCL) (). Besides, the immune infiltrates in DLBCL were mostly similar to FL in terms of PD-L1, PD-L2 and LAG3 expression, with nearly 10% of positive immune cells in both lymphomas. Of note, the rate of PD-L1+ immune cells was significantly higher in DLBCL than in FL and in control samples ().
Figure 5. Rates of infiltrated immune cells expressing immune escape markers in control samples and DLBCL and FL biopsies. (A–D) Shown are the rates of immune cell infiltrates found to be positive for the specified stainings upon visual examination of the FL (n = 27), DLBCL (n = 27) and control tonsil samples (n = 9): PD-1+ (A), PD-L1+ (B), PD-L2+ (C), LAG3+ (D). * indicates significant differences between groups (p < 0.0001; p = 0.02; p = 0.03; p = 0.1355, respectively), Wilcoxon–Mann–Whitney tests.
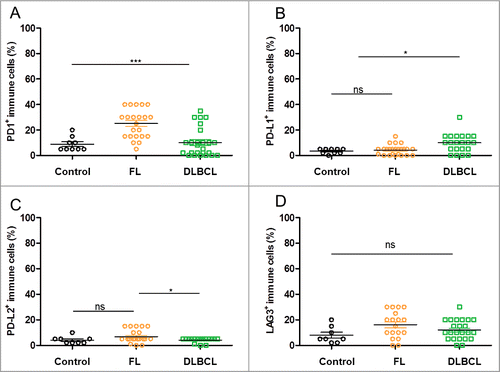
These figures indicate that the proportion of cancer cells expressing immune checkpoint genes was significantly higher in DLBCL than in FL, while their immune infiltrates were similar in both cases except for a higher PD-1 expression in FL lymphocytes. These conclusions validate the above transcriptome data-mining results and extend them by delineating the cellular nature of their respective expression patterns.
Differential expressions of PD-1, PD-L1, PD-L2 and LAG3 in ABC vs. GCB subtypes of DLBCL
We next compared the expression of PD-1, PD-L1, PD-L2 and LAG3 between the ABC and GC subtypes of DLBCL samples. As shown in , ABC and germinal center B cell origin (GCB) DLBCL were roughly similar in terms of PD-1 and LAG3 expression. Of note, the aforementioned few cases of PD-1+ DLBCL cells were present in both groups.
Figure 6. Proportion of tumor cells expressing immune escape markers in ABC- and GC-type DLBCL. (A–D) Shown are the rates of tumor cells found to be positive for the specified stainings upon visual examination of the ABC (n = 20) and GC (n = 7) DLBCL samples: PD-1+ (A), PD-L1+ (B), PD-L2+ (C), LAG3+ (D). * indicates significant differences between groups (p = 0.7; p = 0.04; p = 0.04; p = 0.29, respectively), Wilcoxon–Mann–Whitney tests. (E) Boxplot for normalized mRNA expression of the PD-1 gene PDCD1, the PD-L1 gene CD274, the PD-L2 gene PDCD1LG2, and the LAG3 gene in published transcriptomes from GC and ABC subtypes of DLBCL samples,Citation29 p values for ABC vs. GC obtained from Wilcoxon–Mann–Whitney tests.
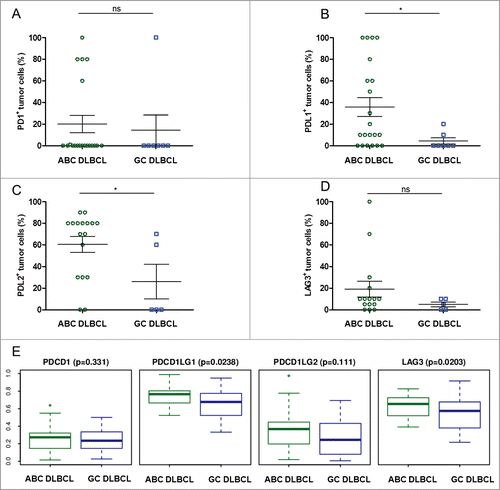
In contrast, the mean rates of PD-L1+ and PD-L2+ lymphoma cells were significantly higher in the ABC subtype (p = 0.04 for each marker) (). This trend for PD-L1 expression was in agreement with a previous study of DLBCL.Citation36 We found that the percentage of PD-L1+ tumor cells per sample was 8-fold higher in ABC DLBCL than in GCB DLBCL: there were on average 36% PD-L1+ tumor cells in the ABC DLBCL samples versus only 4% in GC DLBCL samples. In addition, half the PD-L1+ stained cells from the ABC DLBCL cases had a staining intensity rated 1+, while only 15% of PD-L1+ cells from the GCB DLBCL samples had this intensity. Likewise, the percentage of PD-L2+ tumor cells per sample in ABC DLBCL was double that of GCB DLBCL, with an average of 60% PD-L1+ tumors cells in the ABC DLBCL samples vs. 26% in the GCB DLBCL. However, both subtypes of DLBCL had the same pattern of staining for PD-L2, with two-thirds of the PD-L2+ tumor cells showing 1+ staining intensities.
We finally compared mRNA expression levels of the PD-1 gene PDCD1, the PD-L1 gene PDCD1LG1, the PD-L2 gene PDCD1LG2, and the LAG3 gene in samples of both subgroups of DLBCL taken from the same independent cohort used for the transcriptome data sets. These data confirmed the IHC results, namely that the ABC DLBCL subgroup significantly overexpresses the PD-L1 gene relative to the GCB DLBCL subgroup ().
Discussion
The present combination of transcriptome data-mining and IHC analysis has revealed that both DLBCL and FL tumor cells as well as their infiltrating immune cells upregulate several immune checkpoint genes and critical proteins in a distinct pattern of several immune escape strategies. The expression of genes involved in pathways of cancer immune escape has been already explored in solid tumors.Citation1–3 Here, the data mining of a large series of tumor samples from lymphoma patients evidenced the upregulation of 26–30 genes involved in different mechanisms of immune escape. This conclusion is based on the analysis of the expression of a non-exhaustive group comprising only 54 genes, regardless of additional genes that could be relevant for immune escape and the additional contribution of mutational and epigenetic events. Here, we found that the overexpression of 25 immune escape genes was shared by both FL and DLBCL. These genes are involved in mechanisms as varied as the CTLA4 and PD-1/PD-L1–2 T cell inhibitory axes, the LAG3 and TIM3/galectin T cell exhaustion axes, the production of the immunosuppressive molecules IDO, IL10, GDF15 and CSF1, and the recruitment of immunosuppressive cells by CCL2, CCL22 and CSF1, among others.
We speculate that this expression pattern might result from immune selection in the lymphoma niche, which imposed to evolve several immune escape pathways. Multiple immune escape pathways were recently proposed to take place in solid tumors.Citation37 However, their occurrence in FL and DLBCL had not been reported previously, and their combination might differ from those evolved by other cancers.Citation38
Indeed, transcriptomes from biopsies do not identify which cells in these samples are expressing the immune checkpoint molecules. Therefore, our IHC study not only confirmed the expression of immune-evasion at the protein level, but also specified their respective distribution pattern, whether in tumor cells themselves or in their immune infiltrates. However, IHC on formalin-fixed paraffin-embedded (FFPE) slides is poorly suited to detecting extracellular proteins like secreted cytokines and it does not detect small metabolites like the immunosuppressive PGE2, adenosine or the indoleamine dioxygenase (IDO) product N-formyl-kynurenine. Therefore, combining these two approaches represented a better strategy for a more exhaustive exploration of immune-evasion pathways in lymphomas.
Our study suggests that FL and DLBCL evolve several patterns of immune escape. In these malignancies, the expression of PD-L1 by the tumor cells of DLBCL and by the immune infiltrates of both is consistent with recent reports in various human cancers. The proportion of PD-L1+ DLBCL cases (found in 61% of cases) is higher than in other solid tumor types, in which only 30% of melanoma cases and 25–36% of non-small cell lung cancer cases, respectively, were positive for PD-L1.Citation39,40 Moreover, the ABC subtype of DLBCL prominently expressed not only PD-L1,Citation36,41,42 but also PD-L2. This ABC DLBCL subtype is associated with an inferior survival prognosis compared to the GCB subtype.Citation43 Although over-activation of NFκB and BCR represent major cell-intrinsic determinants of the ABC DLBCL aggressiveness, we propose that by mediating immune escape, the co-expression of PD-L1 and PD-L2 contributes further to giving ABC the worst prognosis of the DLBCL subtypes.Citation44 Although the modest size of the cohort depicted here did not allow us to investigate correlations with clinical data, further studies from our group are currently ongoing to evaluate the impact of immune escape checkpoints on patients' survival. Indeed, recent studies have indicated that PD-L1 expressed on tumor cells not only protects them from antitumoral immunity,Citation2,24,25,45 but also enhances the tumorigenicity in vivo.Citation46 The co-expression of both PD-1 and PD-L1 we detected on some DLBCL tumor cells might unveil a positive autocrine feedback loop of unknown significance in these tumors. Likewise, we found that DLBCL tumor cells express other immune-evasion proteins such as LAG3, a marker involved in inducing lymphocyte anergy. Thus, in DLBCL, the high proportion of cases with immune checkpoint-expressing tumor cells suggests that evolving immune escape pathways is mandatory for this disease. In contrast to DLBCL, most FL cases lacked tumor cells expressing LAG3, PD-1 and its ligands but these markers were instead harbored by the immune microenvironment.Citation36,47 Such immune infiltrates were frequently and strongly stained for PD-1 (reported inCitation48-52 and this study). Most FL-infiltrating lymphocytes which expressed PD-1 had lost cytokine responsiveness and had impaired antitumor immunity.Citation50 Therefore, the PD-1/PD-L1 immunosuppression axis is an important component of the immune escape armament of FL, as was recently demonstrated by the therapeutic benefits of PD-1 blockade in phase 2 FL trials.Citation53 The PD-1/PD-L1,2 pathway is of special concern in Hodgkin's lymphoma as well, where 9p24 chromosomal alterations result in JAK-STAT activation and subsequent overproduction of the ligands PD-L1 and /PD-L2.Citation19-21 As recently demonstrated by trials of anti-PD-1 in Hodgkin's lymphomas,Citation54 this prominence extends beyond of NHL up to non-hematopoietic tumors such as melanoma and lung carcinomas.Citation55,56
In conclusion, we report here that FL and DLBCL evolved several immune escape pathways, suggesting that escape to antitumor immunity was critical in these aggressive lymphomas. Accordingly, NHL tumor cells expressed more of the immune checkpoint genes and proteins on DLBCL than FL. The overexpression of PD-L1 depicted here by DLBCL tumor cells extended previous reports of soluble PD-L1 in circulating blood which is associated with poor outcome for DLBCL patients.Citation57 In accordance with these earlier studies, the PD-1/PD-L1 axis was almost confined to the ABC subtype of DLBCL which presents the worst prognosis of the DLBCL subtypes.
Indeed, the landscape of somatic mutations such as HLA-I deletions and activation of neoantigens and endogenous retroviruses is a major driver to the multiplicity of immuno-escape pathways in human cancer.Citation29,37 We postulate that together with genetic instability, this multiple gene expression strategy reflects a strong and sustained immune pressure on lymphomas in line with the cancer immunoediting concept.Citation58 Since NHL cells emerge and grow despite permanent exposure to distinct mechanisms of surveillance by various immune effectors in their lymphoid tissues, we suggest that the several escape pathways of NHLs reflect their tumor's age, aggressiveness and the local stringency of immune pressure.
Clearly, much remains to be done to determine to what extent immune checkpoints impact on lymphoma patients survival. However, our report provides a rationale for clinical trials investigating multi-targeted combinations of immune checkpoint inhibitors.
Materials and Methods
Patients
Twenty-seven FL and 27 DLBCL patients were diagnosed in the Department of Hematology, CHU Toulouse, France, between 2009 and 2013, according to the World Health Organization 2008 classificationCitation34 (). The median age of the FL cohort was 63 yr (range: 26 to 85 years) and the male/female ratio was 16/11. The median age of the DLBCL cohort was 65.5 yr (range: 40 to 84 years) and the male/female ratio was 23/5. According to Ann Arbor staging, 27 patients (50%) were in the advanced stages (stages III–IV). Institutional ethical approval from INSERM and informed consent were obtained in compliance with the Helsinki protocol.
TMA and IHC staining
We constructed a TMA) from nine control tonsil samples, 27 DLBCL and 27 FL tissues using duplicate 2 mm cores from FFPE blocks. IHC was then performed on 3 µm TMA sections using routine protocols on a fully-automated platform (Ventana Benchmark XT).
The following antibodies were used: CD20 (1:250; DAKO GA604 clone L26), CD10 (1:100; Novocastra-Leica microsystems ORG-8941 clone 56C6), BCL6 (1:30; DAKO GA625 clone PG-B6p), MUM1 (1:25; DAKO GA644 clone MUM1p), BCL2 (pre-diluted; Ventana Medical Sytems, clone SP66 790–4604), PD-1 (1:100; AbCam ab52587 clone NAT-105), PD-L1 (1:60; Roche-Ventana, clone SP142, kind gift from Fabien Soldevilla, Roche-Ventana), PD-L2 (1:600; AbDSerotec AHP1704 polyclonal) LAG3 (1:200; Novus Biologicals NBP1–85781 polyclonal) and Ki67 (1:100; DAKO GA626 clone MIB-1). GC B and non-GC B phenotypes were defined using the decision tree established by Hans and colleagues with the indicated cut-offs.Citation35 Protein expression by IHC was independently scored by two pathologists (C.L., C.F.). The inter-rater agreements for the percentage of cells positive for immune checkpoint molecules estimated by Cohen kappa coefficient (κ) were substantial (κ = 0.71; p < 0.0001) according to Landis and Koch guidelines.Citation59
Statistical analysis
KS tests for IEGS distribution analysis
This analysis of transcriptomes from human FL and DLBCL biopsies as well as B cell controls was based on relevant datasets downloaded from NCBI GEO (http://www.ncbi.nlm.nih.gov/gds). Since several IEGS genes were missing from some of these data sets however, we focused our work to the most recent and exhaustive dataset GSE12195.Citation29 All sets of data from this study were used, except the five cell lines which were excluded because cultured in vitro. The data set was annotated (mapping from probe-ids to gene symbols) using the R/Bioconductor package hgu133plus2.db.Citation60 Then, the duplicate gene symbols were removed, by keeping the row with the maximal interquartile range (reduction). Thus, a matrix of 16,845 rows for genes and 136 columns for samples was obtained. It comprised 20 normal B cell controls, 38 FL and 73 DLBCL. For each of these three groups the row means were computed, producing three vectors of length 16,845 which were then ranked in decreasing order.
We defined the immune escape gene set (IEGS) as a non-exhaustive list of 54 immune escape genes () as already reported elsewhere for different sets of genes.Citation38 This list of genes was selected from a literature (Pubmed)-based search using the keywords: immunosuppressive cytokines, immunosuppressive mechanisms, chemokines, tumor-supportive myeloid genes, immunosuppressive myeloid and monocyte-related genes, immunosuppressive signaling pathway genes, synthesis of immunosuppressive metabolite, lymphocyte exhaustion marker, lymphocyte inhibitory receptor genes, Tregs genes, tumor immune escape, tumor immune evasion, tumor immune suppression. This search yielded the 54 relevant gene symbols which were selected for the IEGS (). As a control, we also defined a random gene set composed of 54 randomly chosen genes.
The positions of the IEGS and the random gene set in the three vectors, once divided by the total number of genes, were calculated and their respective ECDFwere plotted () using Rscript for auxiliary functions depicted below. The curves corresponding to the samples of normalized orders computed from healthy cells appear in blue and those from cancer cells appear in red. For both the random gene set and the IEGS gene set, a two-sample KS test was applied to evaluate if the two samples of their normalized orders in the vector from healthy cells and from cancer cells were significantly different or not. As a control, we simulated 1,000 sets of 54 randomly chosen genes, tested them in the control B cells and NHL samples, applied the two-sample KS test and obtained 1,000 p values. The p values so obtained were checked to be uniform as they should be for random gene sets. We also considered the possibility that non-relevant genes such as olfactory receptors randomly reaching the random set might be upregulated in the NHL samples. There were 114 olfactory receptor genes (i.e., gene symbols ORnXm) out of the 21,229 analyzed genes in GSE12195). Since such genes are expected to represent <1 of any random set of 54 genes, they might not suffice to impact the over-expression the whole random set. Actually, only eight genes out of these 114 were upregulated (p < 0.05, fc >1), while 54 were downregulated and 52 unchanged in FL relative to control B cells. In the DLBCL samples, 24 were upregulated (p <0.05, fc >1), 20 were downregulated and 35 were unchanged relative to the control cells. So non-relevant genes such as the ORnXm cannot significantly impact on upregulation of the random sets in the NHL samples.
The above-depicted procedure was applied to the GEO dataset GSE7307,Citation31 where the samples corresponding to normal colon, breast, kidney, skin, pancreas, lymph nodes, tonsils and spleens were considered separately. Here, the orders of the IEGS genes in the five ranked lists, normalized accordingly, were computed. The one-sample KS test was applied to test whether the IEGS genes are randomly distributed throughout the ranked vector or primarily found at the top of it in each of the five cases. Normal B controls (blue) and lymphoma samples (red) are shown in ; a two-sample KS test was applied to compare these groups. For normal samples of lymph nodes, tonsils, spleen, skin, kidney, pancreas, colon and breast (), the random distribution of ECDF of IEGS was tested by one-sample KS test. The same procedure was applied to other samples of NHLCitation30 : ABC and GC samples of DLBCL from the study GSE12195,Citation29 MM samples of the study GSE6691Citation33 and CLL samples of the study GSE31048Citation32 (ECDFs shown in red, ).
Rscript for auxiliary functions of KS test for IEGS distribution analysis downloaded of GEO data set, treatments of annotations and reduction were done using functions that have been encoded as R script in61.
Additional R scripts used for the present study are as follows:
#————————————–#
# Auxiliary functions
colline <- “black” # color for lines
cexa <- 1.1 # axes size
cexm <- 1.3 # title size
lty <- 2 # line type
lth <- 1.5 # line thickness
#v <- scan(“IEGS.txt”,what=“char”)
order.matching <- function(v1,v2){
# returns the indices of those entries of v1 found in v2,
# v1 and v2 being any two vectors of charater chains
#
return(which(v1 %in% v2))
} # end function order.matching
empcdf <- function(l1,l2,v,title){
# Takes two named vectors of numeric l1 and l2
# and a vector of character v. The (normalized)
# positions of v in the two ranked lists are computed
# and their respective ECDF's are plotted.
#
vs1 <- sort(l1,decreasing=TRUE)
gene.list1 <- names(vs1)
ma1 <- order.matching(gene.list1,v)
vs2 <- sort(l2,decreasing=TRUE)
gene.list2 <- names(vs2)
ma2 <- order.matching(gene.list2,v)
ngenes <- length(gene.list1)
ma1 <- ma1/ngenes
ma2 <- ma2/ngenes
sfun1 <- stepfun(ma1,c(0,seq(1/length(ma1),1,1/length(ma1))), f = 0)
sfun2 <- stepfun(ma2,c(0,seq(1/length(ma2),1,1/length(ma2))), f = 0)
plot(sfun1,verticals = TRUE,do.points=FALSE,xlim=c(0,1),
ylim=c(0,1),main=title,
xlab=“”,
ylab=“”,col=4,xaxt=“n”,yaxt=“n”)
lines(sfun2,verticals = TRUE,do.points=FALSE,col=2)
abline(0,1)
} # end function empcdf
empcdf2 <- function(M,v,title,colour ){
# Takes an expression matrix M. Each sample is
# ranked in decreasing order of expression values and
# then the orders of the genes inside v in the ranked
# list are computed. ECDF's for all samples are plotted.
#
nc <- dim(M)[2]
for (j in 1:nc){
l <- M[,j]
vs <- sort(l,decreasing=TRUE)
gene.list <- names(vs)
ma <- order.matching(gene.list,v)
ngenes <- length(gene.list)
ma <- ma/ngenes
sfun <- stepfun(ma,c(0,seq(1/length(ma),1,1/length(ma))), f = 0)
if (j==1){
plot(sfun,verticals = TRUE,do.points=FALSE,xlim=c(0,1),
ylim=c(0,1),main=title,xlab=“”,ylab=“”,col=colour,xaxt=“n”,yaxt=“n”)
}else{
lines(sfun,verticals = TRUE,do.points=FALSE,xlim=c(0,1),
ylim=c(0,1),col=colour)
}
}
abline(0,1)
} # end function empcdf
KSt <- function(lh,lc,v,alt){
# Takes two named vectors of numeric lh and lc
# and a vector of character v. The (normalized)
# positions of v in the two ranked lists are computed. The
# two-sample KS test with alternative alt is applied.
# Returns the corresponding p-value.
#
lhs <- sort(lh,decreasing=TRUE)
gene.list1 <- names(lhs)
ma1 <- order.matching(gene.list1,v)
ngenes <- length(gene.list1)
ma1 <- ma1/ngenes
lcs <- sort(lc,decreasing=TRUE)
gene.list2 <- names(lcs)
ma2 <- order.matching(gene.list2,v)
ma2 <- ma2/ngenes
pv <- ks.test(ma2,ma1,alternative=alt)$p.value
return(pv)
} # end function KSt
Comparison of IHC immune checkpoint expression
Wilcoxon–Mann–Whitney tests with α = 5% were used to compare the control samples, FL and DLBCL groups, as indicated in , and the ABC-type versus GC-type DLBCL groups, as indicated in .
Comparison of gene expression in ABC and GC subtypes of DLBCL
The GEO dataset GSE12195Citation29 contained 73 DLBCL samples with 31 ABC DLBCL samples and 34 GC DLBCL samples. In each of the two subtype matrices, column values were replaced by ranks and further normalized, by dividing by the total number of rows. The normalized ranks of the genes PDCD1, CD274, PDCD1LG2 and LAG3 in the transcriptomes from GC and ABC subtypes of DLBCL samples are shown as boxplots, and their ranks compared by using the Wilcoxon–Mann–Whitney test ().
Disclosure of Potential Conflicts of Interest
No potential conflicts of interest were disclosed.
Authors' Contributions
CL, KC, and PG performed experiments and analyzed data; CL, MT, and CF analyzed the data; CL, BY, AB, and JJF designed experiments; BY, CL, and JJF supervised the study and wrote the manuscript. AB is an employee of the Laboratoires Pierre Fabre.
Acknowledgments
We are grateful to Laurence Jalabert, Gabrielle Perez and François Xavier Frenois for the TMA and whole-imaging (Plateformes d'immunohistochimie et d'imagerie, département de Pathologie du Pr Brousset, IUCT Oncopole, France). We acknowledge Fabien Soldevilla (Roche-Ventana, France) for kind gifts of anti PD-L1 antibodies and Pierre Ferre (Institut de Recherche Pierre Fabre, France). We thank Kelly Thornber for English editing of the text.
Funding
This work was supported in part by institutional grants from the Institut National de la Santé et de la Recherche Médicale (INSERM), the Université Toulouse III: Paul Sabatier, the Center National de la Recherche Scientifique (CNRS), the Laboratoire d'Excellence Toulouse Cancer (TOUCAN) (contract ANR11-LABX), the Program Hospitalo-Universitaire en Cancérologie CAPTOR (contract ANR11-PHUC0001)) and the Institut Carnot Lymphome (CALYM).
References
- Kim R, Emi M, Tanabe K. Cancer immunoediting from immune surveillance to immune escape. Immunology 2007; 121:1-14; PMID:17386080; http://dx.doi.org/10.1111/j.1365-2567.2007.02587.x
- Pardoll DM. The blockade of immune checkpoints in cancer immunotherapy. Nat Rev Cancer 2012; 12:252-64; PMID:22437870; http://dx.doi.org/10.1038/nrc3239
- Gajewski TF, Schreiber H, Fu YX. Innate and adaptive immune cells in the tumor microenvironment. Nat Immunol 2013; 14:1014-22; PMID:24048123; http://dx.doi.org/10.1038/ni.2703
- Leach DR, Krummel MF, Allison JP. Enhancement of antitumor immunity by CTLA-4 blockade. Science 1996; 271:1734-6; PMID:8596936; http://dx.doi.org/10.1126/science.271.5256.1734
- Phan GQ, Yang JC, Sherry RM, Hwu P, Topalian SL, Schwartzentruber DJ, Restifo NP, Haworth LR, Seipp CA, Freezer LJ et al. Cancer regression and autoimmunity induced by cytotoxic T lymphocyte-associated antigen 4 blockade in patients with metastatic melanoma. Proc Natl Acad Sci USA 2003; 100:8372-7; PMID:12826605; http://dx.doi.org/10.1073/pnas.1533209100
- Hodi FS, Mihm MC, Soiffer RJ, Haluska FG, Butler M, Seiden MV, Davis T, Henry-Spires R, MacRae S, Willman A et al. Biologic activity of cytotoxic T lymphocyte-associated antigen 4 antibody blockade in previously vaccinated metastatic melanoma and ovarian carcinoma patients. Proc Natl Acad Sci USA 2003; 100:4712-7; PMID:12682289; http://dx.doi.org/10.1073/pnas.0830997100
- Hodi FS, O'Day SJ, McDermott DF, Weber RW, Sosman JA, Haanen JB, Gonzalez R, Robert C, Schadendorf D, Hassel JC et al. Improved survival with ipilimumab in patients with metastatic melanoma. N Engl J Med 2010; 363:711-23; PMID:20525992; http://dx.doi.org/10.1056/NEJMoa1003466
- Ninomiya S, Hara T, Tsurumi H, Hoshi M, Kanemura N, Goto N, Kasahara S, Shimizu M, Ito H, Saito K et al. Indoleamine 2,3-dioxygenase in tumor tissue indicates prognosis in patients with diffuse large B-cell lymphoma treated with R-CHOP. Ann Hematol 2011; 90:409-16; PMID:20938662; http://dx.doi.org/10.1007/s00277-010-1093-z
- Löb S, Königsrainer A, Rammensee HG, Opelz G, Terness P. Inhibitors of indoleamine-2,3-dioxygenase for cancer therapy: can we see the wood for the trees? Nat Rev Cancer 2009; 9:445-52; PMID:19461669; http://dx.doi.org/10.1038/nrc2639
- Munn DH, Mellor AL. Indoleamine 2,3-dioxygenase and tumor-induced tolerance. J Clin Invest 2007; 117:1147-54; PMID:17476344; http://dx.doi.org/10.1172/JCI31178
- Rodríguez PC, Ochoa AC. Arginine regulation by myeloid derived suppressor cells and tolerance in cancer: mechanisms and therapeutic perspectives. Immunol Rev 2008; 222:180-91; PMID:18364002; http://dx.doi.org/10.1111/j.1600-065X.2008.00608.x
- Challa-Malladi M, Lieu YK, Califano O, Holmes AB, Bhagat G, Murty VV, Dominguez-Sola D, Pasqualucci L, Dalla-Favera R. Combined genetic inactivation of β2-Microglobulin and CD58 reveals frequent escape from immune recognition in diffuse large B cell lymphoma. Cancer Cell 2011; 20:728-40; PMID:22137796; http://dx.doi.org/10.1016/j.ccr.2011.11.006
- Schietinger A, Greenberg PD. Tolerance and exhaustion: defining mechanisms of T cell dysfunction. Trends Immunol 2014; 35:51-60; PMID:24210163
- Keir ME, Butte MJ, Freeman GJ, Sharpe AH. PD-1 and its ligands in tolerance and immunity. Annu Rev Immunol 2008; 26:677-704; PMID:18173375; http://dx.doi.org/10.1146/annurev.immunol.26.021607.090331
- Nishimura H, Nose M, Hiai H, Minato N, Honjo T. Development of lupus-like autoimmune diseases by disruption of the PD-1 gene encoding an ITIM motif-carrying immunoreceptor. Immunity 1999; 11:141-51; PMID:10485649; http://dx.doi.org/10.1016/S1074-7613(00)80089-8
- Freeman GJ, Long AJ, Iwai Y, Bourque K, Chernova T, Nishimura H, Fitz LJ, Malenkovich N, Okazaki T, Byrne MC et al. Engagement of the PD-1 immunoinhibitory receptor by a novel B7 family member leads to negative regulation of lymphocyte activation. J Exp Med 2000; 192:1027-34; PMID:11015443; http://dx.doi.org/10.1084/jem.192.7.1027
- Dong H, Strome SE, Salomao DR, Tamura H, Hirano F, Flies DB, Roche PC, Lu J, Zhu G, Tamada K et al. Tumor-associated B7-H1 promotes T-cell apoptosis: a potential mechanism of immune evasion. Nat Med 2002; 8:793-800; PMID:12091876; http://dx.doi.org/10.1038/nm0902-1039c
- Keir ME, Liang SC, Guleria I, Latchman YE, Qipo A, Albacker LA, Koulmanda M, Freeman GJ, Sayegh MH, Sharpe AH et al. Tissue expression of PD-L1 mediates peripheral T cell tolerance. J Exp Med 2006; 203:883-95; PMID:16606670; http://dx.doi.org/10.1084/jem.20051776
- Steidl C, Shah SP, Woolcock BW, Rui L, Kawahara M, Farinha P, Johnson NA, Zhao Y, Telenius A, Neriah SB et al. MHC class II transactivator CIITA is a recurrent gene fusion partner in lymphoid cancers. Nature 2011; 471:377-81; PMID:21368758; http://dx.doi.org/10.1038/nature09754
- Green MR, Monti S, Rodig SJ, Juszczynski P, Currie T, O'Donnell E, Chapuy B, Takeyama K, Neuberg D, Golub TR et al. Integrative analysis reveals selective 9p24.1 amplification, increased PD-1 ligand expression, and further induction via JAK2 in nodular sclerosing Hodgkin lymphoma and primary mediastinal large B-cell lymphoma. Blood 2010; 116:3268-77; PMID:20628145; http://dx.doi.org/10.1182/blood-2010-05-282780
- Green MR, Rodig S, Juszczynski P, Ouyang J, Sinha P, O'Donnell E, Neuberg D, Shipp MA. Constitutive AP-1 activity and EBV infection induce PD-L1 in Hodgkin lymphomas and posttransplant lymphoproliferative disorders: implications for targeted therapy. Clin Cancer Res 2012; 18:1611-8; PMID:22271878; http://dx.doi.org/10.1158/1078-0432.CCR-11-1942
- Noman MZ, Desantis G, Janji B, Hasmim M, Karray S, Dessen P, Bronte V, Chouaib S. PD-L1 is a novel direct target of HIF-1α, and its blockade under hypoxia enhanced MDSC-mediated T cell activation. J Exp Med 2014; 211:781-90; PMID:24778419; http://dx.doi.org/10.1084/jem.20131916
- Rosenwald A, Wright G, Chan WC, Connors JM, Campo E, Fisher RI, Gascoyne RD, Muller-Hermelink HK, Smeland EB, Giltnane JM et al. The use of molecular profiling to predict survival after chemotherapy for diffuse large-B-cell lymphoma. N Engl J Med 2002; 346:1937-47; PMID:12075054; http://dx.doi.org/10.1056/NEJMoa012914
- Andersen MH. The targeting of immunosuppressive mechanisms in hematological malignancies. Leukemia 2014; 28:1784-92; PMID:24691076; http://dx.doi.org/10.1038/leu.2014.108
- Blank CU. The perspective of immunotherapy: new molecules and new mechanisms of action in immune modulation. Curr Opin Oncol 2014; 26:204-14; PMID:24424272; http://dx.doi.org/10.1097/CCO.0000000000000054
- Ansell SM, Lesokhin AM, Borrello I, Halwani A, Scott EC, Gutierrez M, Hurt EM, Zhao H, Averett L, Yang L et al. PD-1 blockade with nivolumab in relapsed or refractory hodgkin's lymphoma. N Engl J Med 2015; 372:311-9. PMID: 25482239; http://dx.doi.org/10.1056/NEJM oa1411087
- Huang CT, Workman CJ, Flies D, Pan X, Marson AL, Zhou G, Hipkiss EL, Ravi S, Kowalski J, Levitsky HI et al. Role of LAG-3 in regulatory T cells. Immunity 2004; 21:503-13; PMID:15485628; http://dx.doi.org/10.1016/j.immuni.2004.08.010
- Woo SR, Turnis ME, Goldberg MV, Bankoti J, Selby M, Nirschl CJ, Bettini ML, Gravano DM, Vogel P, Liu CL et al. Immune inhibitory molecules LAG-3 and PD-1 synergistically regulate T-cell function to promote tumoral immune escape. Cancer Res 2012; 72:917-27; PMID:22186141; http://dx.doi.org/10.1158/0008-5472.CAN-11-1620
- Compagno M, Lim WK, Grunn A, Nandula SV, Brahmachary M, Shen Q, Bertoni F, Ponzoni M, Scandurra M, Califano A et al. Mutations of multiple genes cause deregulation of NF-kappaB in diffuse large B-cell lymphoma. Nature 2009; 459:717-21; PMID:19412164; http://dx.doi.org/10.1038/nature07968
- Brune V, Tiacci E, Pfeil I, Döring C, Eckerle S, van Noesel CJ, Klapper W, Falini B, von Heydebreck A, Metzler D et al. Origin and pathogenesis of nodular lymphocyte-predominant Hodgkin lymphoma as revealed by global gene expression analysis. J Exp Med 2008; 205:2251-68; PMID:18794340; http://dx.doi.org/10.1084/jem.20080809
- Roth RB. Human body index - transcriptional profiling. In: http://www.ncbi.nlm.nih.gov/geo/query/acc.cgi?acc=GSE7307NG, ed., 2007.
- Wang L, Shalek AK, Lawrence M, Ding R, Gaublomme JT, Pochet N, Stojanov P, Sougnez C, Shukla SA, Stevenson KE et al. Somatic mutation as a mechanism of Wnt/β-catenin pathway activation in CLL. Blood 2014; 124:1089-98; PMID:24778153; http://dx.doi.org/10.1182/blood-2014-01-552067
- Gutiérrez NC, Ocio EM, de Las Rivas J, Maiso P, Delgado M, Fermiñán E, Arcos MJ, Sánchez ML, Hernández JM, San Miguel JF. Gene expression profiling of B lymphocytes and plasma cells from Waldenström's macroglobulinemia: comparison with expression patterns of the same cell counterparts from chronic lymphocytic leukemia, multiple myeloma and normal individuals. Leukemia 2007; 21:541-9; PMID:17252022; http://dx.doi.org/10.1038/sj.leu.2404520
- Swerdlow SH, Campo E, Harris NL, Jaffe ES, Pileri SA, Stein H, Thiele J, Vardiman JW. WHO Classification of Tumours of Haematopoietic and Lymphoid Tissues, Fourth Edition. In: IARC Press ed., 2008; 439 p
- Hans CP, Weisenburger DD, Greiner TC, Gascoyne RD, Delabie J, Ott G, Müller-Hermelink HK, Campo E, Braziel RM, Jaffe ES et al. Confirmation of the molecular classification of diffuse large B-cell lymphoma by immunohistochemistry using a tissue microarray. Blood 2004; 103:275-82; PMID:14504078; http://dx.doi.org/10.1182/blood-2003-05-1545
- Andorsky DJ, Yamada RE, Said J, Pinkus GS, Betting DJ, Timmerman JM. Programmed death ligand 1 is expressed by non-hodgkin lymphomas and inhibits the activity of tumor-associated T cells. Clin Cancer Res 2011; 17:4232-44; PMID:21540239; http://dx.doi.org/10.1158/1078-0432.CCR-10-2660
- Rooney MS, Shukla SA, Wu CJ, Getz G, Hacohen N. Molecular and genetic properties of tumors associated with local immune cytolytic activity. Cell 2015; 160:48-61; PMID:25594174; http://dx.doi.org/10.1016/j.cell.2014.12.033
- Doucette T, Rao G, Rao A, Shen L, Aldape K, Wei J, Dziurzynski K, Gilbert M, Heimberger AB. Immune heterogeneity of glioblastoma subtypes: extrapolation from the cancer genome atlas. Cancer Immunol Res 2013; 1:112-22; PMID:24409449; http://dx.doi.org/10.1158/2326-6066.CIR-13-0028
- Velcheti V, Schalper KA, Carvajal DE, Anagnostou VK, Syrigos KN, Sznol M, Herbst RS, Gettinger SN, Chen L, Rimm DL. Programmed death ligand-1 expression in non-small cell lung cancer. Lab Invest 2014; 94:107-16; PMID:24217091; http://dx.doi.org/10.1038/labinvest.2013.130
- Weber JS, Kudchadkar RR, Yu B, Gallenstein D, Horak CE, Inzunza HD, Zhao X, Martinez AJ, Wang W, Gibney G et al. Safety, efficacy, and biomarkers of nivolumab with vaccine in ipilimumab-refractory or -naive melanoma. J Clin Oncol 2013; 31:4311-8; PMID:24145345; http://dx.doi.org/10.1200/JCO.2013.51.4802
- Chen BJ, Chapuy B, Ouyang J, Sun HH, Roemer MG, Xu ML, Yu H, Fletcher CD, Freeman GJ, Shipp MA et al. PD-L1 expression is characteristic of a subset of aggressive B-cell lymphomas and virus-associated malignancies. Clin Cancer Res 2013; 19:3462-73; PMID:23674495; http://dx.doi.org/10.1158/1078-0432.CCR-13-0855
- Berghoff AS, Ricken G, Widhalm G, Rajky O, Hainfellner JA, Birner P, Raderer M, Preusser M. PD1 (CD279) and PD-L1 (CD274, B7H1) expression in primary central nervous system lymphomas (PCNSL). Clin Neuropathol 2014; 33:42-9; PMID:24359606; http://dx.doi.org/10.5414/NP300698
- Alizadeh AA, Eisen MB, Davis RE, Ma C, Lossos IS, Rosenwald A, Boldrick JC, Sabet H, Tran T, Yu X et al. Distinct types of diffuse large B-cell lymphoma identified by gene expression profiling. Nature 2000; 403:503-11; PMID:10676951; http://dx.doi.org/10.1038/35000501
- Davis RE, Brown KD, Siebenlist U, Staudt LM. Constitutive nuclear factor kappaB activity is required for survival of activated B cell-like diffuse large B cell lymphoma cells. J Exp Med 2001; 194:1861-74; PMID:11748286; http://dx.doi.org/10.1084/jem.194.12.1861
- Brahmer JR, Pardoll DM. Immune checkpoint inhibitors: making immunotherapy a reality for the treatment of lung cancer. Cancer Immunol Res 2013; 1:85-91; PMID:24777499; http://dx.doi.org/10.1158/2326-6066.CIR-13-0078
- Iwai Y, Ishida M, Tanaka Y, Okazaki T, Honjo T, Minato N. Involvement of PD-L1 on tumor cells in the escape from host immune system and tumor immunotherapy by PD-L1 blockade. Proc Natl Acad Sci USA 2002; 99:12293-7; PMID:12218188; http://dx.doi.org/10.1073/pnas.192461099
- Brown JA, Dorfman DM, Ma FR, Sullivan EL, Munoz O, Wood CR, Greenfield EA, Freeman GJ. Blockade of programmed death-1 ligands on dendritic cells enhances T cell activation and cytokine production. J Immunol 2003; 170:1257-66; PMID:12538684; http://dx.doi.org/10.4049/jimmunol.170.3.1257
- Wahlin BE, Aggarwal M, Montes-Moreno S, Gonzalez LF, Roncador G, Sanchez-Verde L, Christensson B, Sander B, Kimby E. A unifying microenvironment model in follicular lymphoma: outcome is predicted by programmed death-1–positive, regulatory, cytotoxic, and helper T cells and macrophages. Clin Cancer Res 2010; 16:637-50; PMID:20068089; http://dx.doi.org/10.1158/1078-0432.CCR-09-2487
- Amé-Thomas P, Le Priol J, Yssel H, Caron G, Pangault C, Jean R, Martin N, Marafioti T, Gaulard P, Lamy T et al. Characterization of intratumoral follicular helper T cells in follicular lymphoma: role in the survival of malignant B cells. Leukemia 2012; 26:1053-63; PMID:22015774; http://dx.doi.org/10.1038/leu.2011.301
- Myklebust JH, Irish JM, Brody J, Czerwinski DK, Houot R, Kohrt HE, Timmerman J, Said J, Green MR, Delabie J et al. High PD-1 expression and suppressed cytokine signaling distinguish T cells infiltrating follicular lymphoma tumors from peripheral T cells. Blood 2013; 121:1367-76; PMID:23297127; http://dx.doi.org/10.1182/blood-2012-04-421826
- Carreras J, Lopez-Guillermo A, Roncador G, Villamor N, Colomo L, Martinez A, Hamoudi R, Howat WJ, Montserrat E, Campo E. High numbers of tumor-infiltrating programmed cell death 1-positive regulatory lymphocytes are associated with improved overall survival in follicular lymphoma. J Clin Oncol 2009; 27:1470-6; PMID:19224853; http://dx.doi.org/10.1200/JCO.2008.18.0513
- Farinha P, Al-Tourah A, Gill K, Klasa R, Connors JM, Gascoyne RD. The architectural pattern of FOXP3-positive T cells in follicular lymphoma is an independent predictor of survival and histologic transformation. Blood 2010; 115:289-95; PMID:19901260; http://dx.doi.org/10.1182/blood-2009-07-235598
- Westin JR, Chu F, Zhang M, Fayad LE, Kwak LW, Fowler N, Romaguera J, Hagemeister F, Fanale M, Samaniego F et al. Safety and activity of PD1 blockade by pidilizumab in combination with rituximab in patients with relapsed follicular lymphoma: a single group, open-label, phase 2 trial. Lancet Oncol 2014; 15:69-77; PMID:24332512; http://dx.doi.org/10.1016/S1470-2045(13)70551-5
- Ansell SM, Lesokhin AM, Borrello I, Halwani A, Scott EC, Gutierrez M, Schuster SJ, Millenson MM, Cattry D, Freeman GJ et al. PD-1 blockade with nivolumab in relapsed or refractory Hodgkin's lymphoma. N Engl J Med 2015; 372:311-9; PMID:25482239; http://dx.doi.org/10.1056/NEJMoa1411087
- Gettinger SN, Horn L, Gandhi L, Spigel DR, Antonia SJ, Rizvi NA, Powderly JD, Heist RS, Carvajal RD, Jackman DM, et al. Overall Survival and Long-Term Safety of Nivolumab (Anti-Programmed Death 1Antibody, BMS-936558, ONO-4538) in Patients With Previously Treated Advanced Non-Small-Cell Lung Cancer. J Clin Oncol. 2015 Apr 20. pii: JCO.2014.58.3708. [Epub ahead of print]. PMID:25897158
- Topalian SL, Sznol M, McDermott DF, Kluger HM, Carvajal RD, Sharfman WH, Brahmer JR, Lawrence DP, Atkins MB, Powderly JD et al. Survival, durable tumor remission, and long-term safety in patients with advanced melanoma receiving nivolumab. J Clin Oncol 2014; 32:1020-30; PMID:24590637; http://dx.doi.org/10.1200/JCO.2013.53.0105
- Rossille D, Gressier M, Damotte D, Maucort-Boulch D, Pangault C, Semana G, Le Gouill S, Haioun C, Tarte K, Lamy T et al. High level of soluble programmed cell death ligand 1 in blood impacts overall survival in aggressive diffuse large B-Cell lymphoma: results from a French multicenter clinical trial. Leukemia 2014; 28:2367-75; PMID:24732592; http://dx.doi.org/10.1038/leu.2014.137
- Shankaran V, Ikeda H, Bruce AT, White JM, Swanson PE, Old LJ, Schreiber RD. IFNgamma and lymphocytes prevent primary tumour development and shape tumour immunogenicity. Nature 2001; 410:1107-11; PMID:11323675; http://dx.doi.org/10.1038/35074122
- Landis JR, Koch GG. The measurement of observer agreement for categorical data. Biometrics 1977; 33:159-74; PMID:843571; http://dx.doi.org/10.2307/2529310
- Carlson M. hgu133plus2.db: affymetrix human genome U133 plus 2.0 array annotation data (chip hgu133plus2). R Package Version 2 14.0
- Ycart B, Charmpi K, Rousseaux S, Fournié JJ (2014) Large Scale Statistical Analysis of GEO Datasets. Gene Technology 3: 113. doi:10.4172/2329-6682.1000113 ISSN:2329-6682 GNT, an open-access journal.