Abstract
The Mahalanobis-Taguchi system (MTS) is a relatively new multi-dimensional pattern recognition technology that combines Mahalanobis distance (MD) with Taguchi's robust engineering for diagnosis and prognosis. MTS operation process generally consists of four specialised stages, namely, Mahalanobis space (MS) construction, MS validation, MS optimisation, and diagnosis and prognosis. In recent years, a significant amount of research work has been conducted on the improvement of key technologies and these four processes. Numerous applications of MTS have also been studied in various fields. There are, of course, excellent reviews of theoretical research and applied research of MTS. However, there is no systematic review that covers both theoretical and applied research comprehensively. To fill this gap, this paper reviews MTS from key technologies, four processes and application research, and provides researchers with a discussion of the current situation, upcoming challenges and possible future trends.
Disclosure statement
No potential conflict of interest was reported by the author(s).
Additional information
Notes on contributors
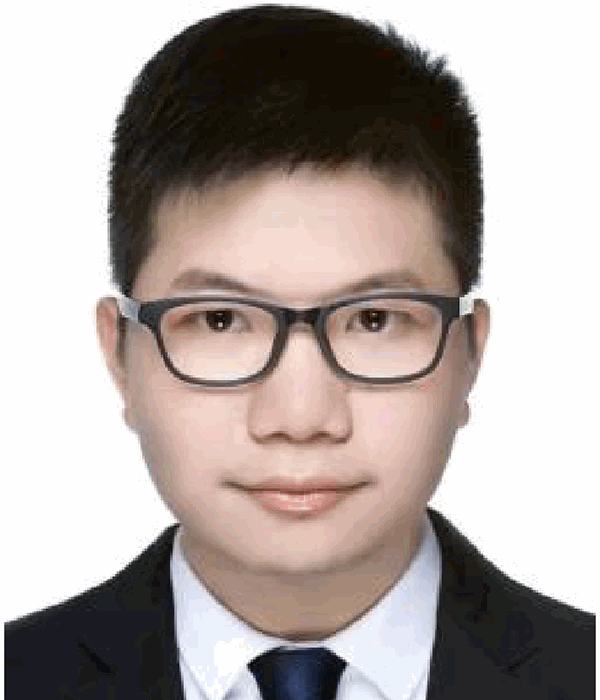
Zhaiming Peng
Zhaiming Peng received his M.S. degree in mathematics from Anhui University of Technology, in 2016. He is currently pursuing the Ph.D. degree in management at Nanjing University of Science and Technology. His current research interests include prognostics and health management (PHM), machine learning, Quality management and quality engineering, and data mining.
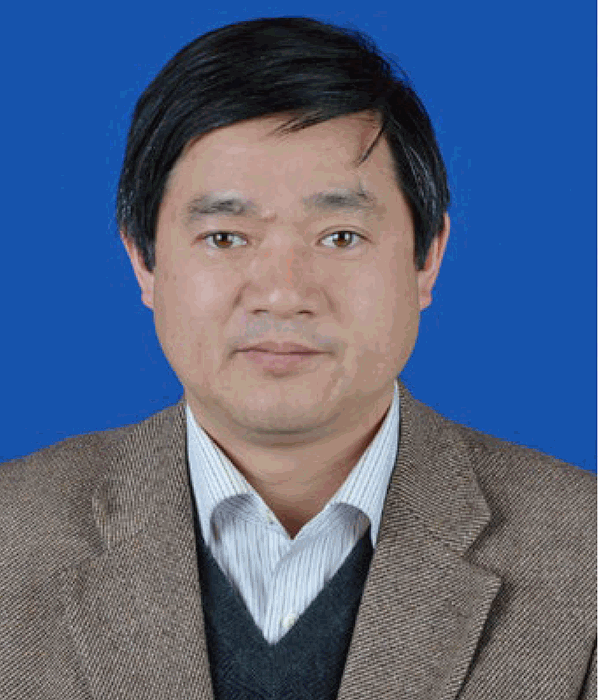
Longsheng Cheng
Longsheng Cheng received the M.S. degree from the East China Institute of Technology, China, in 1988, and the Ph.D. degree in control science and engineering from the Nanjing University of Science and Technology, China, in 1998. From 1998 to 1999, he served as a research assistant at the City University of Hong Kong. Since 2005, he has become a professor of management sciences and engineering in the School of Economics and Management, Nanjing University of Science and Technology. His current research interests include prognostics and health management (PHM), machine learning, quality engineering, and data mining.
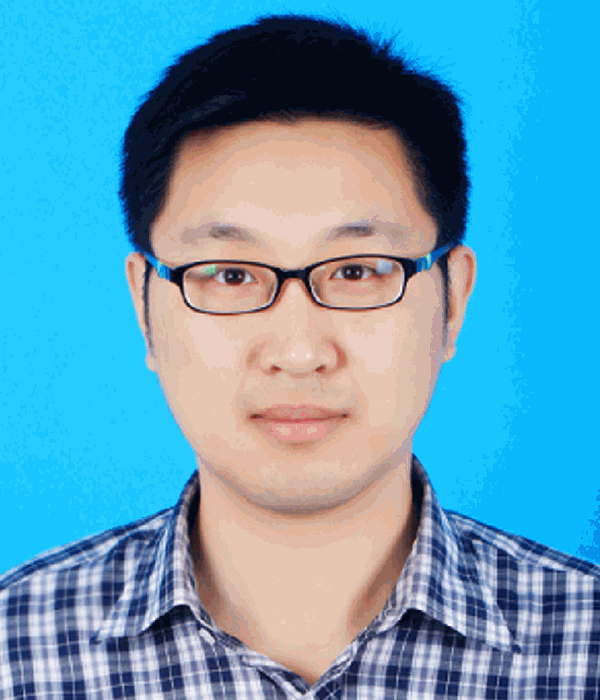
Qifeng Yao
Qifeng Yao received his M.S. degree in applied mathematics from Anhui University of Technology, in 2018. He is currently pursuing the Ph.D. degree in management at Nanjing University of Science and Technology. His current research interests include prognostics and health management (PHM), machine learning, and probability theory.
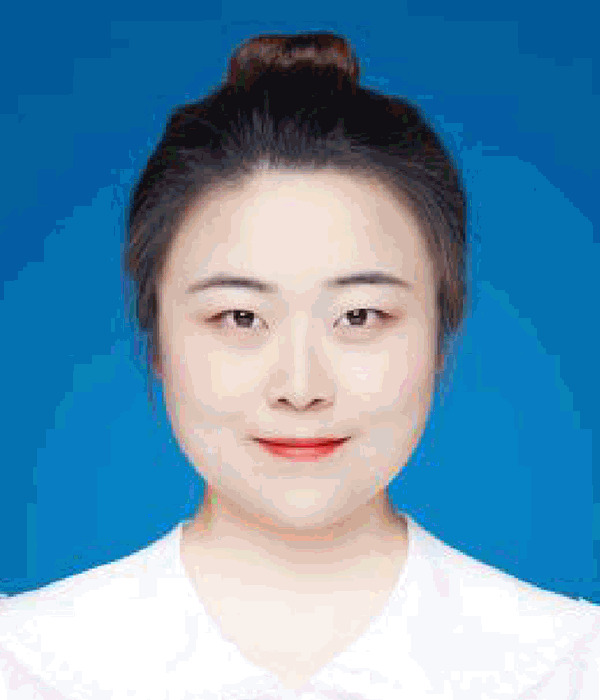
Hanting Zhou
Hanting Zhou received her master's degree in management from China Jiliang University, in 2019. She is currently pursuing the Ph.D. degree in management at Nanjing University of Science and Technology. Her current research interests include prognostics and health management (PHM), machine learning, quality management and quality engineering, and data mining.