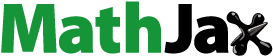
Abstract
Reflecting growing emphasis on data analysis and statistical thinking in the information age, mathematics curriculum standards in the U.S. have recently increased expectations for student learning in the domain of statistics and probability. More than 180 teachers in 36 public school districts in Florida applied for a two-week summer institute designed to increase teachers’ content and pedagogical content knowledge in statistics and probability. Individual teachers were assigned at random to a treatment or business-as-usual comparison group. The two-week institute increased teachers’ knowledge of statistics. Data analyses identified an interaction between years of teaching experience and treatment, indicating that the teachers with more than 10 years of experience had larger knowledge gains than their less-experienced peers. These results underscore the need for professional development for teachers so that they may implement policies emphasizing this branch of the mathematical sciences in the secondary mathematics curriculum. Given the observed lower baseline knowledge scores for teachers with more years of teaching experience, we posit these implications are particularly applicable to teachers who completed their own formal education more than 10 years ago.
PUBLIC INTEREST STATEMENT
We are living in the Information Age, and the role of data analysis is increasing in many facets of life. Driven by this trend, school mathematics curricula have placed greater emphasis on statistics over the last decade. This article describes a two-week summer institute designed to provide secondary-level mathematics teachers with the requisite knowledge for teaching what their students are expected to learn in statistics. Using a randomized-controlled trial evaluation design, the researchers found that the program had a positive impact on teacher knowledge, and the knowledge gains persisted for at least one year. The data also indicated that the program had a larger effect on teachers with 10 or more years of teaching experience than on teachers with less experience. The authors maintain that it is vitally important to provide teachers with opportunities to learn the material they are expected to teach—especially when the material is something they have not previously been expected to teach or learn.
1. Introduction
In recent years, a tremendous amount of data has become available in the areas of education, health, economics, and even the behaviors of individual people in their daily lives. Data analysis has become an integral part of the decision-making process for businesses and other organizations, policymakers, and individuals (Steen, Citation2001). According to the projections of the U.S. Bureau of Labor Statistics (Citation2016), jobs related to statistics are expected to grow 34% during this decade—much faster than the average expected growth rate for all occupations. Statistical thinking is not just for the workplace; it is also an essential competency “for informed citizens making everyday decisions based on data” (Franklin et al., Citation2015, p. 1).
1.1. Changes in policy and resulting expectations for secondary-level students and teachers
Reflecting changes in access to data, availability of data analysis tools, and the demand for data analysis in recent decades, expectations for teaching and learning in the domain of probability and statistics in K–12 school mathematics have substantially increased. Many state standards were influenced in the 1990s and 2000s by the National Council of Teachers of Mathematics’ Curriculum and Evaluation Standards for School Mathematics, which are widely credited for giving data analysis and probability more legitimacy in the modern K–12 school mathematics curriculum (NCTM, Citation1989, Citation2000). More recently, the adoption of the Common Core State Standards for Mathematics (CCSS-M; National Governors Association Center for Best Practices, Citation2010) places higher emphasis on statistics and probability in the middle grades than can be found in any previous U.S. curriculum standards.
There are many indicators that the demand for statistics instruction at the secondary level is steadily increasing. In Florida—the state where the current study was conducted and one of many states where the mathematics curriculum standards were heavily influenced by the CCSS-M—the proportion of content standards from the statistics and probability domain in the course description for sixth-grade mathematics increased from 10% to 17% after adoption of the CCSS-M. In seventh grade, the emphasis is even more pronounced, going from 18% of the mathematics content standards prior to adoption of the CCSS-M to 33% of the content standards in the current course description. Also in Florida, 16% of the mathematics content standards in the Algebra 1 course description are from the statistics and probability domain, an increase from 0% in the pre-CCSS-M Algebra 1 course description. The annual total number of U.S. students taking Advanced Placement (AP) statistics increased from 7,667 in 1997—the first year the exam was offered—to 215,840 in 2016 (The College Board, Citation1997, Citation2017).
Increased expectations for student learning in the domain of statistics and probability in curriculum standards and course descriptions thereby increases expectations of mathematics teachers (Bargagliotti, Citation2014). Prior to the call in the NCTM Standards (Citation1989, Citation2000) to place more emphasis on data analysis and probability in K–12 mathematics, middle-grades teachers were typically not expected to teach statistics and probability except for limited topics such as calculating indicators of central tendency, creating and interpreting graphs, and thinking about probability in terms of ratios and proportions. In the CCSS-M era, middle-grades mathematics teachers are now expected to teach principles for formulating statistical questions, convey an understanding of statistics as the study of variation, help students understand the role of sampling procedures in interpretation of data, lay the foundation for understanding statistical inference and hypothesis testing, and teach students how to design simulations to estimate probability of events (National Governors Association Center for Best Practices, Council of Chief State School Officers, Citation2010). These shifts in expectations of mathematics teachers are substantial—especially for those teachers who completed their own K–12 schooling prior to when the shift occurred.
Research findings following previous standards-based reform initiatives indicate that simply adopting new standards and related assessments is insufficient to bring intended changes in instruction and learning (Borko, Wolf, Simone, & Uchiyama, Citation2003; Fullan, Citation1985; Spillane, Reiser, & Reimer, Citation2002). Individual teachers have a major influence on how the standards are enacted in their classrooms (Ball, Citation1990; Coburn, Citation2004; Smith, Citation2000; Spillane, Citation1999; Weatherly & Lipsky, Citation1977). Teachers are in a position either to provide learning opportunities for students consistent with the goals of the standards or to respond to the changes in the standards and accountability systems with incremental, surface-level changes to their instructional practice (Chapman & Heater, Citation2010; Cohen, Citation1990; Hill, Citation2001; McLaughlin, Citation1987; Simon, Citation2013; Smith, Citation2000; Spillane et al., Citation2002; Spillane & Zeulli, Citation1999).
1.2. Importance of teachers’ subject-matter knowledge
Researchers studying standards-based reform and related changes in teachers’ instructional practice consistently argue that teacher knowledge plays a crucial role in whether the standards are successfully implemented as intended (Ball & Cohen, Citation1999; Ball, Hill, & Bass, Citation2005; Borko, Citation2004; Darling-Hammond, Wei, Andree, Richardson, & Orphanos, Citation2009; Hill & Ball, Citation2004; Putnam & Borko, Citation1997). Even in the presence of strong accountability initiatives and incentives, teachers’ practices change only when the goals and content of the reforms are understood deeply by teachers (Spillane et al., Citation2002). As Ball et al. (Citation2005) noted, “Strong standards and quality curriculum are important. But no curriculum teaches itself, and standards do not operate independently of professionals’ use of them. To implement standards and curriculum effectively, school systems depend upon the work of skilled teachers who understand the subject matter” (p. 1). Teachers’ knowledge is essential for teachers to use new instructional materials effectively, to assess students’ progress, to interpret and respond to students’ work, to make sound judgments about presentation, emphasis, and sequencing of the topics, to choose and use effective instructional tools, and to help students succeed on more challenging assessments (Ball, Lubienski, & Mewborn, Citation2001). Hill, Rowan, and Ball (Citation2005) found the strongest association between teachers’ subject-matter knowledge and student achievement when comparing teachers in the lowest 30% of the distribution with the higher-knowledge teachers.
Statistics is consistently identified as the topic in which mathematics teachers have the greatest need for learning both content and pedagogy (CBMS, Citation2001, Citation2012; Franklin et al., Citation2015; Groth, Citation2007). Despite the increased emphasis on statistics and probability in the K–12 mathematics curriculum since 1989 (National Council of Teachers of Mathematics, Citation1989, Citation2000; NGA & CCSSO, Citation2010), it is unusual for universities to offer courses designed to increase pedagogical content knowledge in statistics (i.e., knowledge specific to teaching statistics). Based on data from national surveys, the Conference Board for Mathematical Sciences (CBMS) found that very few teacher preparation programs require any statistics courses for graduation, and fewer than 1% of colleges and universities offering bachelor’s degrees or higher in statistics offer an undergraduate course designed to prepare K–12 teachers for teaching statistics (CBMS, Citation2012). Learning opportunities and other forms of support for those teachers who are presently required to teach this material (and for whom their salary and tenure are increasingly dependent upon getting results based on student achievement scores) are urgently needed. Recognizing the need at the elementary, secondary, and post-secondary levels, the American Statistical Association and the Mathematical Association of America have released several publications outlining their recommendations for learning institutions to support both teacher and student learning in statistics (ASA/MAA Joint Committee on Teaching Statistics, Citation2014; Franklin et al., Citation2007).
1.3. Content-based professional development for teachers
Many studies have found that professional development programs can help practicing teachers to improve their content knowledge and support implementation of the curriculum standards (Arbaugh & Brown, Citation2005; Bell, Wilson, Higgins, & McCoach, Citation2010; Borko, Citation2004; Cohen & Hill, Citation2000; Desimone, Citation2009; Hawley & Valli, Citation1999; Kisa & Correnti, Citation2015; Knapp, Citation2003; Moats & Foorman, Citation2003; Sykes & Darling-Hammond, Citation1999). Reviews of research on teacher PD consistently report that programs focused on specific subject-matter topics can increase student achievement (Blank & de Las Alas, Citation2009; Kennedy, Citation1998, Citation2016; Yoon, Duncan, Lee, Scarloss, & Shapley, Citation2007). More recently, several large-scale, rigorously designed evaluations of the effects of teacher PD programs on student achievement have not found evidence that the teacher PD programs result in improvements in student achievement (Garet et al., Citation2016, Citation2011; Gersten, Taylor, Keys, Rolfhus, & Newman-Gonchar, Citation2014; Jacob, Hill, & Corey, Citation2017; Jayanthi, Gersten, Taylor, Smolkowski, & Dimino, Citation2017; Yoon et al., Citation2007). A few programs that have been subjected to rigorously designed evaluation have reported a causal link between teacher PD and improved student achievement (e.g., Jacobs, Franke, Carpenter, Levi, & Battey, Citation2007; Lewis & Perry, Citation2017; Penuel, Gallagher, & Moorthy, Citation2011; Powell, Diamond, Burchinal, & Koehler, Citation2010; Schoen, LaVenia, & Tazaz, Citation2018). One design feature that the programs in this latter group have in common is that they aim to increase teacher’s subject-matter knowledge and knowledge of students while also involving teachers in creating or modifying curricular resources. We hypothesize that the combination of these two elements—especially when they are focused and integrated—may increase the likelihood that teacher PD programs will impact student learning.
1.4. Purpose of the current study
The current study reports the results of a randomized controlled trial evaluating the impact of a professional development program on teacher content and pedagogical content knowledge, the Summer 2014 Institute for Early Secondary Statistics and Probability (hereafter: Stats Institute). The PD program involved a two-week summer institute for teachers that was designed to increase teacher content and pedagogical content knowledge in the domain of probability and statistics while also supporting teachers in creating related curricular resources designed to be implemented in their classroom. The purpose of the current study was to determine the extent to which the program had the intended effect on teacher knowledge and to explore whether teacher characteristics explained variation in the effect of the program on teachers’ content knowledge. The following research questions guided the study.
What was the effect of the two-week summer Stats Institute on teachers’ knowledge in probability and statistics as measured by DTAMS at the start of the school year?
To what extent was the effect of the Stats Institute on teacher knowledge moderated by teachers’ baseline knowledge or years of teaching experience?
Did the effects on teacher knowledge on the fall 2014 posttest persist on the spring 2015 delayed-posttest?
Our aim for RQ1 was to take a confirmatory approach to determine the effect of the Stats Institute, as supported by the randomized design employed in the study. Our review of the literature suggested that teachers’ baseline knowledge in the area of statistics and probability may vary depending on where they were in their educational trajectory at the time corresponding changes in policy and resulting expectations for secondary-level students and teachers were taking place. Accordingly, we specified RQ2 to investigate the conditions under which the program was most efficacious through the inclusion of baseline knowledge and years of teaching experience as potential moderators of the PD program’s impact on teacher knowledge. Because we exploit natural variation in the sample to investigate the moderation of the effect of treatment, the results for RQ2 are interpreted as exploratory in nature. Our aim for RQ3 was to evaluate whether the findings from RQ1 and RQ2 replicate across assessment waves, constituting lasting effects of the PD program.
1.5. Description of the stats institute program
1.5.1. Stats institute development
The initial development of some components of the Stats Institute occurred from summer 2012 through summer 2013 as part of a two-year professional development program for middle grades mathematics and science teachers to learn about integrating mathematics and science through mathematical modeling and computing. In summer 2013, the workshop components were delivered as a one-week institute with a larger group of secondary-level mathematics teachers. Using a pre-post assessment design in both of the pilot programs, paired-sample t-tests suggested statistically significant increases on the content knowledge assessment for the development-year pilot sample and summer 2013 pilot sample.Footnote1 Informed by these prior experiences and their own expertise, the university professors and high-school teachers collaborated over a period of several months to plan and implement the summer 2014 version of the two-week Stats Institute, which is the subject of the present study.
1.5.2. Stats institute theory of change
The Stats Institute aimed to increase teacher content and pedagogical content knowledge as a strategy to support teachers in implementing the state mathematics standards in the statistics and probability domain at the early secondary level. The underlying theory of change in the program posits that teacher participation in the program should have a direct effect of increasing their content and pedagogical content knowledge in the domain of probability and statistics and result in teacher-created instructional resources designed to support implementation of the statistics and probability standards in their classrooms. As an indirect result, classroom instruction is expected to be affected positively by the teachers’ participation in the two-week institute. As an indirect result of changes in classroom instruction, the theory of change hypothesizes that students’ learning outcomes in the classrooms of teachers in the intervention condition will be greater than those of students in classrooms of teachers assigned to the counterfactual condition. Thus, teacher knowledge is thought to mediate instructional practice, and instructional practice, in turn, is expected to mediate student learning outcomes. The current study investigates the first link in the chain of events in this theory of change: the effect of the professional development program on teachers’ knowledge in the domain of probability and statistics.
1.5.3. Stats institute format
The program consisted of 80 h of professional development in a face-to-face, classroom-type setting. The sessions occurred over a two-week period, with 7.5 h of meetings (plus a 1-h lunch break) each day, Monday through Friday, and an additional 5-h session on the middle Saturday that was wholly dedicated to providing time for teachers to develop instructional resources to teach statistics. The 80 h of contact time comprised three main components: (1) teacher knowledge development, (2) resource development, and (3) administrative tasks.
Approximately 55 h were dedicated to the first component, which mostly consisted of activities designed to increase teachers’ knowledge related to the statistics and probability topics that students at the middle grades level are expected to learn in accordance with the CCSS-M. Five of the 55 h dedicated to component one consisted of discussion about how to interpret the state curriculum standards in statistics and probability and how to engage in formative assessment while teaching these topics.
Approximately 20 h of the 80 PD hours were dedicated to the second component (i.e., resource development). Administrative tasks, including activities such as an orientation to the agenda for the week, morning and afternoon breaks, and completion of assessment surveys comprised a total of five of the 80 h. The resource-development component primarily consisted of teachers developing lesson plans and getting feedback on the lesson plans from the institute leaders. The resource development component served several purposes. For one, it provided teachers with the opportunity to think about how to transfer what they were learning in the workshop into changes in instructional practice in their schools. In addition, it provided opportunities for the teachers to leave the institute with ready-to-use instructional materials for their classrooms. The institute leaders reviewed the lesson plans and provided feedback, which also provided opportunities for formative feedback based around what the teachers were showing they understood about the material being discussed in the institute.
The Stats Institute was conducted in three concurrent sessions of 25 to 28 participants per session. Each session had its own team of five institute leaders. One of the individuals on the team was an active university professor in the mathematical sciences, two were high school teachers with experience teaching statistics and/or data visualization strategies with technology, and two additional people reviewed the instructional resources created by teachers in the program and provided feedback to the teachers.
1.5.4. Stats institute content
The activities in the Stats Institute were organized into eight units. Table lists the topics in each of the units. Each unit was composed of three to seven individual lessons or activities that typically lasted between 90 and 120 minin length. An online component—intended to provide an introduction to the use of statistical software packages—was offered as a precursor to the two-week institute.
Table 1. Organizational structure for the content of the professional development workshops
The CCSS-M provided a guiding framework for determining the content focus and content limits. As such, the focus of the content was on a conceptual introduction rather than on proof or application of formal rules or theorems. The three developmental levels of statistical literacy (i.e., A, B, C) as described in the GAISE report (Franklin et al., Citation2007) provided a conceptual framework. As described in the GAISE report, engagement with statistical ideas at level A involves the least depth of understanding or formality from a mathematical perspective. Levels B and C involve increasingly higher levels of sophistication, depth of understanding, and formality. These proposed levels of development are based around an understanding of statistics and statistical reasoning, not the age of the learner. Participants in the institute primarily engaged in activities that would be characterized as level A in the GAISE framework, which generally matched the participants’ levels of statistical literacy, but some activities were targeted to levels B and C.
Earlier variations of the Stats Institute had been implemented with middle-grades mathematics and science teachers over the course of three years prior to the randomized trial. During implementation of the previous versions of the program, we learned that most of the middle-grades teachers had little or no experience working with software designed for data analysis such as Microsoft Excel, R, or Geogebra. Virtually all modern data analysis techniques requires the data analyst to know how to use software packages, so the first unit was designed to provide teachers with a basic introduction to using software for data-analysis purposes. This included learning how to enter data into cells, using the software to calculate numerical statistics (e.g., mean, median, mode, quartiles, and standard deviation), creating data visualization displays (e.g., scatterplots, boxplots, dotplots, and histograms), and loading add-ins that allow for linear regression and hypothesis testing. Microsoft Excel was selected not for its power or ease of use, but rather for its widespread availability in the school districts represented in the sample and its widespread use outside of school contexts. Microsoft Excel, R, and Geogebra software packages were subsequently used throughout the two-week program to support teachers in learning how to analyze data as well as how to use the software tools.
2. Method
2.1. Participants
2.1.1. Sample state representation
Participants were teachers from 35 of Florida’s 67 regular, public school districts and one university lab school. The 36 districts represent all the geographic regions of the state. Among the 180 teachers enrolled in the study, 105 represented seven urban school districts, 37 represented 12 suburban districts, and 38 represented 17 rural districts.Footnote2 Thirty of the 36 participating school districts (all but one of the urban and five of the suburban) met the state criterion for high-needs districts at that time in Florida, which was defined as 50% or greater of the students enrolled in the district being eligible for free or reduced-price lunch.
One hundred forty-six schools were represented by the sample of 180 enrolled teachers. Accordingly, there was a low incidence of clustering within schools, whereby, for 120 of the teachers, they were the only participant in their school; clusters of two, three, and four teachers represented 20 schools, four schools, and two schools, respectively. Similarly, except for the urban districts, clusters of particiants within districts were small, with 14 of the districts being represented by only one teacher each. Table presents the representation of districts and schools within the sample at assignment and each wave of analysis.
Table 2. District and school representation in the sample at assignment and each wave of analysis
2.1.2. Sample characteristics
Participants were predominantly regular classroom teachers (>80%), with approximately 10% being teachers of advanced classes (e.g., Gifted and Talented, Advanced Placement), approximately 5% being special education or intervention teachers, and several with the primary role of math coach. All participants, including the math coaches, were secondary-level mathematics teachers who reported working directly with students to teach mathematics content. Approximately one-third of the teachers in the sample taught ninth grade or higher; the others taught middle-grades students. Less than half reported having earned degrees in mathematics, mathematics education, the natural sciences, or science education. All teachers held valid state teaching certificates; nearly 90% were certified in secondary mathematics. Approximately three-fourths of the participants identified as female, two-thirds identified as White, one-third as Black, and one-sixth as Hispanic. Table presents the baseline characteristics of the analytic sample at posttest and delayed-posttest, with characteristics disaggregated by condition and for the overall sample. Figure displays the distribution of years of experience for the posttest analytic sample, disaggregated by condition and for the overall sample. Baseline characteristics of the assigned sample are provided in the supplemental materials Table S1.
Table 3. Baseline characteristics of analytic samples
2.1.3. Sample formation
We sought permission to conduct research in 67 regular school districts in Florida and three special school districts (e.g., university lab schools). Approval was obtained for 62 of the regular districts and one of the special districts. Only teachers from the 63 districts who granted (or did not require) approval to conduct research in their districts were eligible to be enrolled in the Stats Institute study. Teachers who had participated in the development-year pilot or the 2013 summer institute versions of the program were not eligible to be enrolled in the 2014 Stats Institute or the corresponding randomized controlled trial. Enrollment criteria also required teachers’ teaching assignment in the 2013–14 or 2014–15 school year to include working directly with secondary (grades 6–12) students to teach mathematics content. Following the procedures approved by the university and school districts’ institutional review boards, participating teachers were informed of the mutual expectations for the research study and actively provided their consent to participate at the time of their online application to participate in the professional development program.
2.2. Random assignment procedure
Individual teachers who applied and met the eligibility criteria were randomly assigned to the Stats Institute treatment condition or the business-as-usual control condition.Footnote3 Random assignment was conducted in an initial round, plus rolling assignment in an attempt to maintain a target of 83 institute attendees.Footnote4 The first round of assignment was conducted with 159 eligible applicants. The random assignment procedure for the first round blocked on school district in an attempt to ensure that at least some teachers from each participating district would be invited to participate in the professional-development program. For districts that were members of one of two participating consortia of small rural districts, the consortium served as the block.Footnote5
The number of participants assigned to treatment from each district or consortium was based on the proportion of number-of-institute-seats to number-of-eligible-applicants at that time. After the first round of assignment, subsequent applicants determined to be eligible for assignment were added to a waitlist. Rolling assignment was triggered by notification from treatment participants that they would be unable to attend the institute. Cumulatively, the assignment procedure resulted in 93 applicants assigned to treatment and 87 assigned to control. Detailed description of the randomization procedure is provided in the supplemental materials inclusive of Table S2. Seventy-four participants assigned to treatment attended the Stats Institute; all 74 attendees completed the full 80-h professional development program. All participants were remunerated for completing the assessments, and the treatment group participants were remunerated for attending the Stats Institute.
All participants in the sample had a non-zero probability of assignment to treatment, though the exact probability varied, based on (a) the number of teachers per block; (b) whether the larger portion was assigned to treatment or control, in instances when the block comprised an odd number of teachers; and (c) whether teachers were assigned in the initial round or during the rolling assignment phase. Treatment probabilities were documented for calculation of marginal weights for the application of inverse probability of treatment weighting within the analyses. Inverse probability of treatment weighting (IPTW) weights cases proportional to the inverse probability of assignment to treatment, weighting up for blocks where the probability of assignment to treatment fell below the average probability for the sample and weighting down for blocks that exceeded the average probability for the sample. As executed in Mplus (Muthén & Muthén, Citation1998–2015), the weights are scaled so they sum to the total number of observations. A description of the approach used for calculating the IPTW weights is provided in Appendix A.
2.3. Materials and procedures
Data collection for the Stats Institute made use of both online and paper-based questionnaires. Online questionnaires used the Qualtrics (Citation2005–2015) survey platform. Paper-based questionnaires were deployed via hyperlinked, cloud-stored files, which participants printed, completed, and returned in hardcopy via prepaid UPS shipping.
A web-based application to participate in the Stats Institute was activated at the end of January 2014, coinciding with the beginning of the recruitment phase. The pretest assessment window was early-April 2014 to mid-June 2014. The Stats Institute convened mid- through late-June 2014. The posttest assessment window was mid-August 2014 to mid-September 2014. The assessment window for the delayed posttest was mid-April 2015 to mid-May 2015.
2.4. Outcome measures
We measured teachers’ knowledge in the domain of probability and statistics using the Diagnostic Teacher Assessment of Mathematics and Science (DTAMS) middle school probability and statistics scales (Saderholm, Ronau, Brown, & Collins, Citation2010). Leveraging the parallel forms available for the DTAMS, we used a different form for each wave of assessment: pretest, posttest, and delayed-posttest.Footnote6 All three of these assessments were completed by the participants on their own time and returned to the evaluation team by mail. We then shipped the completed assessments to the DTAMS developers at the Center for Research in Mathematics and Science Teacher Development at the University of Louisville for scoring. The test scorers were not aware of the examinees’ treatment conditions. The scoring procedures for the DTAMS probability and statistics scales yield various subscale scores, but the total score was the outcome of interest for the current study. Based on a sample of 543 teachers who completed the DTAMS middle school probability and statistics scale as part of a validation study, the developers report a Cronbach’s alpha coefficient of .90 (Saderholm et al., Citation2010). Using our own sample, we calculated a Cronbach’s alpha of .82, .81, and .81 for each of the three forms used in the current study.
2.5. Analytic approach
Corresponding to the three research questions, statistical models were fit to the data to investigate (1) the effect of the two-week, summer institute on teachers’ knowledge shortly after the end of the institute, (2) moderation of the treatment effect by baseline knowledge and years of teaching experience, and (3) the persistence of effects on the delayed-posttest. Using Mplus version 7.4 (Muthén & Muthén, Citation1998–2015), models were specified using the MLR (maximum likelihood estimation with robust standard errors) estimator.
In our analyses, we adjusted for non-independence of observations within district or consortium using the Mplus TYPE = COMPLEX option, which employs a cluster-robust standard error approach as discussed by McNeish, Stapleton, and Silverman (Citation2017). Given that the cluster level was not of substantive interest to the current study, we determined the aggregated analysis of the cluster-robust standard error approach to be more suitable than the multilevel modeling approach of modeling parameters on both the within and between levels of clustering. IPTW sampling weights were employed in all models to adjust for the variation in assignment probabilities across blocks.
Our identification of baseline knowledge and years of teaching experience as potential moderators of treatment is based on issues discussed in the Introduction section. We also identified several teacher and school characteristics to potentially include in our models as covariates. In addition to baseline knowledge and years of teaching experience, which were tested as potential moderators of the treatment effect, teacher characteristics we identified as potential covariates were grade levels taught, having a college degree in mathematics or science, having a teaching certification in secondary mathematics, gender, and racial/ethnic minority. For school characteristics, we identified district urbanicity as a factor of potential importance.
We used a stepwise backward elimination approach to covariate selection. Starting with a full model containing all covariates and higher-order terms for the interaction between treatment, baseline knowledge, and years of teaching experience. We employed a .10 significance level removal criterion and did not remove lower-order terms if corresponding higher-order terms were retained. The stepwise backward elimination approach to covariate selection resulted in a decision to retain the first-order predictors of treatment, pretest, experience, and rural, and the higher-order predictor of treatment-by-experience for the analytic models. Table presents the stepwise backward elimination results and coefficient estimates for the full model and zero-order correlations on the fall posttest.
Table 4. Contrast of teachers with less than 10 years of experience with teachers with 10 or more years of experience for baseline knowledge in statistics and probability
Table 5. Treatment effects on DTAMS probability and statistics assessment at fall 2014 posttest
Table 6. Treatment effects on DTAMS probability and statistics assessment at spring 2015 delayed-posttest
Table B1. Missing data pattern across three waves of assessment.
Table B2. Stepwise backward elimination covariate selection results and coefficient estimates for the full model and zero-order correlations on the fall posttest
2.6. Missing data
At the fall 2014 posttest, data were collected for 139 participants (70 treatment and 69 control). At the spring 2015 delayed-posttest, data were collected for 84 participants (44 treatment and 40 control). Referencing the 180 randomly assigned participants, posttest measurement attrition resulted in overall and differential attrition rates of 22.8% and 4.0%, respectively. For the delayed-posttest, the overall and differential attrition rates were 53.3% and 1.3%, respectively.
Among the 139 participants who contributed posttest data, two cases were not measured at baseline. For the 84 participants who contributed delayed-posttest data, one case was not measured at baseline. Three participants did not contribute DTAMS data at any assessment wave. Table in Appendix B presents the missing data pattern for the DTAMS across the three waves of assessment. Our modeling approach estimated the means and variances for covariates, which brought the covariates into the Full Information Maximum Likelihood (FIML) model where missing data were assumed missing at random (Muthén, Muthén, & Asparouhov, Citation2016). Accordingly, our analytic models used all cases with data on the dependent variable to estimate regression coefficients.Footnote7
3. Results
3.1. Determination of model specification
To inspect the robustness of the effect of treatment across different model specifications, we used a model-building procedure that first tested the effect of treatment without any extraneous variables; second, we added pretest and rural; third, we added years of experience; and fourth, we added the interaction between treatment and years of experience. Years of experience and the treatment-by-experience moderator were added in separate steps to allow for an inspection of the average and conditional effects of the component variables. Because the treatment-by-baseline knowledge interaction was not retained in the backward elimination covariate selection procedure, we do not present the coefficients for the baseline knowledge moderator in the formal reporting of study results; however, they are provided in the supplemental materials Tables S3 and S4.
In order to get a sense for how the treatment-by-experience moderator was operating, we ran a t-test for equality of means, contrasting teachers with less than 10 years of experience with teachers with 10 or more years of experience for baseline knowledge in statistics and probability, where 10 years are the approximate mean for the sample. Table presents the t-test results.Footnote8 Inspecting within condition and for the overall sample, the pretest mean was consistently higher for teachers with fewer years of experience. Although the difference was not statistically significant at p < .05 for any of the tests, it was of a substantive magnitude for the overall sample: less than 10 years (M = 23.46, SD = 6.53) and 10 or more years (M = 22.05, SD = 6.88) years of experience; t(173) = 1.41, p = .178, Hedges’ g = 0.25. Thus, although both the treatment-by-pretest two-way interaction and the treatment-by-pretest-by-experience three-way interaction were eliminated through the covariate selection procedure, it does appear that baseline knowledge may be a factor in explaining the moderation of treatment by years of experience.
3.2. Findings at fall 2014 posttest
Table presents the coefficients for our model fitting procedure applied to the fall 2014 posttest data. Without inclusion of other covariates, treatment had an effect size of g = 0.31 and significance level of p = .07. After controlling for pretest and rural, treatment became statistically significant at the p < .01 level and remained so after adding all other covariates. The final model indicated that treatment was statistically significant (p = .001) with an effect size of g = 0.28. With the interaction term in the model and years of teaching experience centered at year 10, a gain of 0.28 standard deviations in content knowledge is interpreted as the predicted effect of treatment for teachers with 10 years of experience. The interaction term was statistically significant (p = .001) with a standardized effect of β = 0.24, indicating a linear increase of 0.24 standard deviations in the effect of treatment for each standard deviation increase in teaching experience. Table presents the coefficients for the four models fit to the posttest data.
We used Preacher, Curran, and Bauer’s (Citation2006) R-based online tool to generate plots to decompose conditional effects and identify regions of significance. Figures and graphically present the interaction between treatment and years of experience in predicting teacher knowledge at fall posttest. The plot in Figure illustrates a relatively flat slope for treatment for teachers with three years teaching experience (approximately 1 SD below the mean number of years of teaching experience), a moderate positive slope for teachers with 10 years teaching experience (the approximate mean number of years of experience), and a notably steep positive slope for teachers with 17 years teaching experience (approximately 1 SD above the mean number of years of teaching experience). The plot in Figure illustrates how the simple slope for treatment varies by years of experience. The region of significance at p < .05 is indicated by the points at which the confidence bands do not include a slope of zero, which on the posttest is teachers with nine years of experience and higher. Figure displays the distribution of years of experience for the posttest analytic sample, disaggregated by condition and for the overall sample.Footnote9
Figure 1. Plot illustrating the interaction of treatment and years of teaching experience on the immediate posttest DTAMS Total score. Estimates are based on model controlling for pretest, treatment, years of teaching experience, the interaction of treatment by experience, and rural (Table , Model 4).
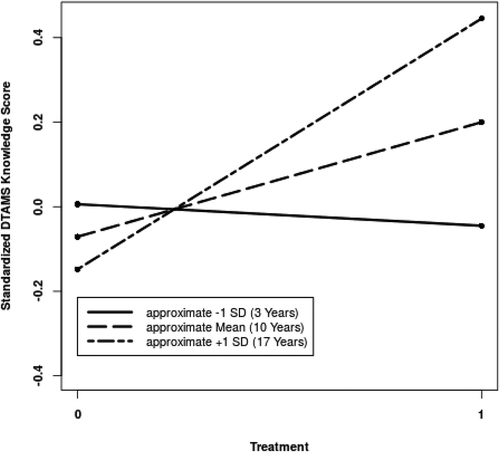
3.3. Findings at spring 2015 delayed-posttest
Table presents the coefficients for our model fitting procedure applied to the spring 2015 delayed-posttest data. We found a similar range of estimated effect sizes across time points. At fall posttest, estimates of the treatment effect ranged from 0.25 to 0.31 across models; and at spring delayed-posttest, estimates of the treatment effect ranged from 0.24 to 0.28 across models. Patterns of statistical significance were also similar at both time points, though the p-values were larger for the delayed-posttest. Without inclusion of other covariates, the size of the effect of the treatment condition on the delayed posttest was estimated to be g = 0.24 with a significance level of p = .15. After controlling for pretest and rural, the size of the effect of treatment was estimated to be approximately the same, but the p-value was less than .01 and remained less than .02 after adding all other covariates. The final model indicated that treatment was statistically significant (p = .01) with an effect size of g = 0.26. The interaction term had a significance level of p = .08 with a standardized effect size of β = 0.20, indicating a linear increase of 0.20 standard deviations in the effect of treatment for each standard deviation increase of teaching experience.
Figures and graphically present the interaction between treatment and years of experience on predicting teacher knowledge at spring delayed-posttest. Similar to results from the fall posttest data, the plots in Figures and indicate an increase in the effect of treatment associated with increased years of experience. The plotted simple slope in Figure illustrates that the region of significance at p < .05 is nine years of teaching experience and higher—the same threshold found for the fall posttest.
Figure 4. Plot illustrating the interaction of treatment and years of teaching experience on the delayed-posttest DTAMS Total score. Estimates are based on model controlling for pretest, treatment, years of teaching experience, the interaction of treatment by experience, and rural (Table , Model 4).
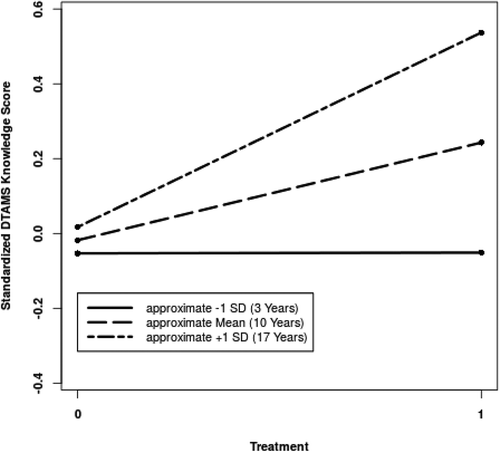
4. Discussion
The purpose of the current study was to determine whether the Stats Institute program increased teacher knowledge in statistics and probability and to explore potential moderators of the effect of the program. We remind the reader that three different, but parallel, DTAMS forms were used across the three waves of data collection. The posttest wave of data collection lagged the cessation of treatment by two months, while the delayed posttest wave lagged the cessation of treatment by 10 months, which was approximately one school year.
The immediate goal of the program was to increase teacher content knowledge, and that goal seems to have been achieved. On average, teachers’ knowledge in statistics was increased by the Stats Institute by approximately one-fourth of a standard deviation, ranging from 0.25 to 0.31 across models at posttest; and from 0.24 to 0.28 across models at delayed-posttest. The magnitude of the effect size estimates for treatment on both the posttest and delayed posttest was remarkably consistent across all models, and the introduction of covariates increases the statistical significance of the association. The stability in the effect size estimates across the two-time points provides further evidence of the effect of the program on teacher knowledge as well as evidence of validity for the use of DTAMS for its intended purpose. These results instill confidence in the conclusion that the program had a positive effect on teacher knowledge in statistics.
The discrepancy in significance levels of the estimated effects at posttest and delayed-posttest appears attributable to a loss in statistical power associated with a decrease in sample size. The estimated coefficient for the treatment effect at posttest is almost the same as the estimated coefficient for the treatment effect at delayed-posttest. The larger confidence interval for the effect of treatment on the delayed-posttest can also be observed with the comparison of the Figures and plots of the variation in the treatment slope across the range of years of teaching experience. According to standards outlined by the What Works Clearinghouse (U.S. Department of Education, Citation2017b), attrition rates for the fall posttest sample suggest a tolerable threat of bias, even under cautious assumptions. For the delayed-posttest sample, attrition rates suggest a tolerable threat of bias, but only under optimistic assumptions.Footnote10
Based on our analyses, the program seems to have been particularly effective for those teachers with 10 or more years of teaching experience. One plausible explanation for the years of experience and treatment interaction is that the teachers with 10 or more years of experience simply never had an opportunity to learn the material during their own years as a student or to otherwise pause and think deeply about statistics under the guidance of a knowledgeable statistics teacher, and the two-week institute provided that first opportunity. This conjecture is consistent with findings reported in Table , where on average, teachers with less than 10 years of experience had higher DTAMS scores at baseline than teachers with 10 or more years of experience. Given that the emphasis in the K–12 curriculum standards has been steadily growing in the past two decades, it makes sense that the teachers who completed their K–12 schooling more recently might have had more exposure to statistical ideas during their years of formal schooling than their peers who have been in the workforce longer. We expect the observation that the interaction becomes significant at about 10 years of experience is not important in an absolute sense or because it is near the sample mean years of experience. Teachers with 10 or more years of teaching experience in the year 2014 would have graduated from high school before the year 2000, which is about the time when the curriculum standards in grades K–12 mathematics began introducing higher expectations for the general population of U.S. students to learn statistics. With all other factors held constant, had we conducted the study in 2010 or 2020, rather than 2014, we might suspect that the region of significance may have started at six years or 16 years of experience, respectively.
Despite using it in the Stats Institute, we acknowledge that Microsoft’s Excel software package is not the best available software for supporting statistical thinking. There are better software packages available for this purpose. We used Excel because of its near-universal availability to teachers. Because every school district would require individual licensing agreements with software providers and security protocols for using software on district computers, and the coordination among the 36 school districts represented in the present study that would have been required to make other software available to all participating teachers was judged to be overly burdensome, we did not incorporate other commercially available software designed to support teaching and learning of statistics. Training teachers on the use of software designed for teaching statistics may serve to increase the quality of implementation of classroom instruction, which may, in turn, increase student learning.
Because some of the co-authors of the current article were also developers of the program, we would like to think that the program was brilliantly designed and executed, and we would like to think that we have particularly unique and important insight into effective instructional practices in teacher professional development in probability and statistics. While our findings show that this specific professional development program clearly has some merit, we also view the results of our study as a referendum on the general lack of support currently provided for secondary-level mathematics teachers to increase their knowledge in the domain of statistics and probability. In other words, we think that the generally inadequate knowledge in statistics and probability among the secondary-level mathematics teaching workforce combined with very little support for teacher learning in the counterfactual condition enables even a moderately well-designed and implemented program to outpace the counterfactual condition.
4.1. Limitations
There are potential threats to both internal and external validity present in this study. While the attrition rates fall within the current guidelines put forth by the What Works Clearinghouse, especially at the posttest wave of data collection, we do not know what caused some of the teachers to discontinue their participation in the study, so it is possible that the corresponding missing data could introduce bias, especially at the delayed post-test where the overall attrition rate was higher. Moreover, we do not know how the assessment conditions might have affected participants’ effort, because the teachers completed the DTAMS assessments on their own time.
Teachers who engaged in practice-as-usual with respect to professional development and statistics instruction comprised the comparison group for the present study. It is possible that the impact on teacher knowledge was primarily due to a focused amount of time thinking about the topic. Future studies are needed to compare different features of teacher learning opportunities in statistics to help develop a more precise understanding of the features of learning opportunities for statistics teachers that work better than others.
The teachers in this sample participated in the present study on their own accord. In a recent review of the impact of teacher professional-development programs, Kennedy (Citation2016) found that programs resulted in larger effect size estimates when teachers participated voluntarily than when they were required to participate by their school or district. We do not know if the results reported here would be repeated if the teachers had been required by their schools to participate.
Some of the 180 teachers in our sample reported having earned degrees in mathematics, but none of them reported having earned a degree at any level in statistics. As stated by Franklin et al. (Citation2007), “Statistics … a relatively new subject for many teachers, who have not had an opportunity to develop sound knowledge of the principles and concepts underlying the practices of data analysis that they now are called upon to teach.” (p. 5). The findings from the present study may not generalize to populations of teachers who have already had more educational opportunities in statistics.
The present study enables us to evaluate the first step in the theory of change, but it does not enable us to evaluate the implementation or impact of the program on classroom instruction or student learning. While we think teacher knowledge of the subject matter they are held accountable to teach is fundamentally important, it is widely acknowledged that simply increasing knowledge of subject matter alone is not sufficient for increasing teaching effectiveness and student achievement (PCAST, Citation2012; Shulman, Citation1986). An important longer-term goal will be to investigate whether the program has an indirect effect on student learning. While the resource-development component of the Stats Institute program may have provided teachers with some support in thinking about how to use their new knowledge to implement the standards, the professional development model may benefit from additional components that will support changes in instructional practice in order to reasonably expect the changes in teacher knowledge to impact student learning. Much more research in this area is needed.
4.2. Conclusions
Many practicing teachers have had little or no formal training in statistics, yet they are currently held accountable to teach it to children. Creating a secondary-level teaching workforce who understands the standards in the statistics and probability domain at the level expected by the current curriculum standards will require a concerted effort with long-term support for teacher learning. Raising teachers’ subject-matter knowledge to a point where they have progressed through all three levels of statistical literacy described by the GAISE report (Franklin et al., Citation2007) will most certainly require learning opportunities that are focused on specific content and considerably longer in duration than the program we implemented and evaluated in the current study. While we report a significant, positive effect of the program on teachers’ knowledge, we think there is much more for these teachers to learn in statistics before they are fully prepared to serve their important role in helping secondary-level students to build a strong foundation for understanding of statistics. Nonetheless, opportunities for teachers to learn how to teach statistics—such as the Stats Institute—represent an important, perhaps critical, part of a larger implementation strategy for attaining the goal set forth by recent standards-based initiatives in mathematics.
Supplemental Material
Download Zip (45.7 KB)Acknowledgements
The research reported here was supported by grant# 371-2353A-4CM01 to Florida State University from the Florida Department of Education through the U.S. Department of Education’s Math-Science Partnership program. The opinions expressed are those of the authors and do not represent views of the U.S. Department of Education’s Math-Science Partnership program or the Florida Department of Education.
Supplementary material
Supplemental data for this article can be accessed here.
Additional information
Funding
Notes on contributors
Robert C. Schoen
Robert C. Schoen (https://www.schoenresearch.com/), associate professor of mathematics education in the School of Teacher Education at Florida State University, is the associate director of the Florida Center for Research in Science, Technology, Engineering, and Mathematics (FCR-STEM) at Florida State University. His research is driven by the question, what will it take to improve teaching and learning for all mathematics and statistics students? The subject of the present study was the third version of a secondary-level teacher professional-development program since 2011. Extending this chain of inquiry in pursuit of answers to the driving question, Schoen continues to lead research and development involving of professional-development and curricular interventions in statistics.
Notes
1. We used the DTAMS Middle School Probability-Statistics Assessment for the development-year pilot and summer institute pilot. Using the same form pre- and post-, bootstrapped paired-sample t-tests indicated statistically significant increases on the total score for development-year (p < .001, n = 9) and the summer institute pilot (p < .001, n = 73).
2. District urbanicity designation was based on 2010 U.S. Census Bureau (Citation2012) data on population size and urban density. Urban districts were >500,000 in size with >90% density in urbanized areas. Suburban districts ranged from100,000 to 500,000 in size with >70% density in urbanized areas. Rural districts were <100,000 in size or <70% density in urbanized areas. The university lab school was assigned the same designation as the regular district in which it was located.
3. The business-as-usual condition constituted teachers participating in whatever professional development opportunities made available to them through their district or they had arranged for themselves as individuals.
4. The sample target of 83 institute attendees is derived from there being three sessions with a target minimum of 28 attendees per session. One institute seat was a wild card seat given to a non-participant; thus, (3 × 28)—1 = 83 target institute attendees who were randomly assigned to the treatment condition.
5. Seven small rural districts were members of one educational consortium, and eight small rural districts were members of another.
6. We used the DTAMS Middle School Probability-Statistics Assessment (MS PS) Version 1.3 at pretest, MS PS v3.3 at posttest, and MS PS v4.3 at delayed-pretest. Each version was created by the DTAMS developers, of which they consider to be parallel forms with equivalency correlations significant at the p < .01 level.
7. Models were constrained to base estimates only on cases with data for the dependent variable. Although FIML is capable of retaining all cases, including those missing outcome data, our finding of a tolerable level of attrition for the samples persuaded us to simply drop cases missing data for the dependent variable and retain cases regardless of missingness for covariates. The only missingness for covariates were two cases with fall posttest data and one case with spring delayed-posttest data who did not have pretest data.
8. T-tests results are based on all 175 teachers with pretest scores, regardless of whether they were observed at posttest. The t-tests do not incorporate sampling weights or cluster-robust standard errors.
9. In response to the positively skewed years of experience distribution, we re-fit the models using a natural log transformation of the experience variable. Findings did not change markedly, though the direction of change was an increase in magnitude for the treatment coefficient and decrease in its p-value. Given that the detection of treatment effects appeared robust to the scaling decision for years of experience, we determined it would be best to retain years of experience in its original metric for ease of interpretability.
10. We have no information to suggest attrition was related to the intervention. Declared reasons for withdrawing from the study included career changes to a role that did not involve secondary grades math instruction, moving to a location outside of a participating district, and personal/health related.
References
- Arbaugh, F., & Brown, C. A. (2005). Analyzing mathematical tasks: A catalyst for change. Journal of Mathematics Teacher Education, 8(6), 499–26. doi:10.1007/s10857-006-6585-3
- ASA/MAA Joint Committee on Teaching Statistics. (2014). Qualifications for teaching and introductory statistics course. Retrieved from http://www.amstat.org/asa/files/pdfs/EDU-TeachingIntroStats-Qualifications.pdf
- Ball, D. L. (1990). Reflections and deflections of policy: The case of Carol Turner. Educational Evaluation and Policy Analysis, 12(3), 247–259. doi:10.3102/01623737012003247
- Ball, D. L., & Cohen, D. K. (1999). Developing practice, developing practitioners: Toward a practice-based theory of professional education. In L. Darling-Hammond & G. Sykes (Eds.), Teaching as the learning profession (pp. 3–32). San Francisco: Jossey-Bass.
- Ball, D. L., Hill, H. C., & Bass, H. (2005). Knowing mathematics for teaching: Who knows mathematics well enough to teach third grade, and how can we decide? American Educator, 29(1), 14–17, 20–22, 43–46.
- Ball, D. L., Lubienski, S., & Mewborn, D. (2001). Research on teaching mathematics: The unsolved problem of teachers‘ mathematical knowledge. In V. Richardson (Ed.), Handbook of research on teaching (pp. 433–456). New York: Macmillan.
- Bargagliotti, A. (2014). Statistics: The new ‘it’ common-core subject. Education Week, 33(19), 20–21.
- Bell, C. A., Wilson, S. M., Higgins, T., & McCoach, D. B. (2010). Measuring the effects of professional development on teacher knowledge: The case of developing mathematical ideas. Journal for Research in Mathematics Education, 41(5), 479–512.
- Blank, R., & de Las Alas, N. (2009). Effects of teacher professional development on gains in student achievement: How metaanalysis provides scientific evidence useful to education leaders. Washington, DC: Council of Chief State School Officers.
- Borko, H. (2004). Professional development and teacher learning: Mapping the terrain. Educational Researcher, 33(8), 3–15. doi:10.3102/0013189X033008003
- Borko, H., Wolf, S., Simone, G., & Uchiyama, K. (2003). Schools in transition: Reform efforts and school capacity in Washington state. Educational Evaluation and Policy Analysis, 25, 171–203. doi:10.3102/01623737025002171
- Bureau of Labor Statistics. (2016). U.S. Department of labor, occupational outlook handbook (2016-17 ed.). Retrieved from http://www.bls.gov/ooh/math/statisticians.htm
- Chapman, O., & Heater, B. (2010). Understanding change through a high school mathematics teacher’s journey to inquiry-based teaching. Journal of Mathematics Teacher Education, 13(6), 445–458. doi:10.1007/s10857-010-9164-6
- Coburn, C. E. (2004). Beyond decoupling: Rethinking the relationship between the institutional environment and the classroom. Sociology of Education, 77(3), 211–244. doi:10.1177/003804070407700302
- Cohen, D. (1990). A revolution in one classroom: The case of Mrs. Oublier. Educational Evaluation and Policy Analysis, 12(3), 327–345. doi:10.3102/01623737012003311
- Cohen, D. K., & Hill, H. C. (2000). Instructional policy and classroom performance: The mathematics reform in California. Teachers College Record, 102(2), 294–343. doi:10.1111/tcre.2000.102.issue-2
- The College Board. (1997). 1997 advanced placement program: national summary reports. Retrieved from http://media.collegeboard.com/digitalServices/pdf/research/national_1997.pdf
- The College Board. (2017). Student score distributions. Retrieved from https://secure-media.collegeboard.org/digitalServices/pdf/research/2017/Student-Score-Distributions-2017.pdf
- Conference Board of Mathematical Sciences. (2001). The mathematical education of teachers. Providence, RI: American Mathematical Society and Mathematical Association of America.
- Conference Board of Mathematical Sciences. (2012). The mathematical education of teachers II. Providence, RI: American Mathematical Society and Mathematical Association of America.
- Darling-Hammond, L., Wei, R. C., Andree, A., Richardson, N., & Orphanos, S. (2009). Professional learning in the learning profession. Washington, DC: National Staff Development Council.
- Desimone, L. M. (2009). Improving impact studies of teachers’ professional development: Toward better conceptualizations and measures. Educational Researcher, 38(3), 181–199. doi:10.3102/0013189X08331140
- Franklin, C., Kader, G., Mewborn, D., Moreno, J., Peck, R., Perry, M., & Scheaffer, R. (2007). Guidelines for Assessment and Instruction in Statistics Education (GAISE) Report: A Pre-K−12 curriculum framework. Alexandria, VA: American Statistical Association.
- Franklin, C. A., Bargagliotti, A. E., Case, C. A., Kader, G. D., Scheaffer, R. L., & Spangler, D. A. (2015). The statistical education of teachers. Retrieved from American Statistical Association website http://www.amstat.org/education/SET/SET.pdf
- Fullan, M. (1985). Change processes and strategies at the local level. Elementary School Journal, 85, 391–421. doi:10.1086/461411
- Garet, M., Wayne, A., Stancavage, F., Taylor, J., Eaton, M., Walters, K., … Doolittle, F. (2011). Middle school mathematics professional development impact study: Findings after the second year of implementation (NCEE 2011-4024). Washington, DC: National Center for Education Evaluation and Regional Assistance, Institute of Education Sciences, U.S. Department of Education.
- Garet, M. S., Heppen, J. B., Walters, K., Parkinson, J., Smith, T. M., Song, M., … Borman, G. D. (2016). Focusing on mathematical knowledge: The impact of content-intensive teacher professional development (NCEE 2016-4010). Washington, DC: National Center for Education Evaluation and Regional Assistance, Institute of Education Sciences, U.S. Department of Education.
- Gersten, R., Taylor, M. J., Keys, T. D., Rolfhus, E., & Newman-Gonchar, R. (2014). Summary of research on the effectiveness of math professional development approaches. Tallahassee: Regional Educational Laboratory Southeast, Florida State University. Retrieved from http://ies.ed.gov/ncee/edlabs/regions/southeast/pdf/REL_2014010.pdf
- Groth, R. E. (2007). Toward a conceptualization of statistical knowledge for teaching. Journal for Research in Mathematics Education, 38(5), 427–437.
- Hawley, W. D., & Valli, L. (1999). The essentials of effective professional development: A new consensus. In L. Darling-Hammond & G. Sykes (Eds.), Teaching as the learning profession: Handbook of policy and practice (pp. 127–150). San Francisco, CA: Jossey-Bass.
- Hill, H. C. (2001). Policy is not enough: Language and the interpretation of state standards. American Educational Research Journal, 38(2), 289–318. doi:10.3102/00028312038002289
- Hill, H. C., & Ball, D. L. (2004). Learning mathematics for teaching: Results from California‘s mathematics professional development institutes. Journal for Research in Mathematics Education, 35(5), 330–351. doi:10.2307/30034819
- Hill, H. C., Rowan, B., & Ball, D. L. (2005). Effects of teachers’ mathematical knowledge for teaching on student achievement. American Educational Research Journal, 42(2), 371–406. doi:10.3102/00028312042002371
- Jacob, R., Hill, H., & Corey, D. (2017). The impact of a professional development program on teachers’ mathematical knowledge for teaching, instruction, and student achievement. Journal for Research on Educational Effectiveness, 10(2), 379–407. doi:10.1080/19345747.2016.1273411
- Jacobs, V. R., Franke, M. L., Carpenter, T. P., Levi, L., & Battey, D. (2007). Professional development focused on children‘s algebraic reasoning in elementary school. Journal for Research in Mathematics Education, 38(3), 258–288.
- Jayanthi, M., Gersten, R., Taylor, M. J., Smolkowski, K., & Dimino, J. (2017). Impact of the developing mathematical ideas professional development program on grade 4 students’ and teachers’ understanding of fractions (REL 2017–256). Washington, DC: U.S. Department of Education, Institute of Education Sciences, National Center for Education Evaluation and Regional Assistance, Regional Educational Laboratory Southeast. Retrieved from http://ies.ed.gov/ncee/edlabs.
- Kennedy, M. M. (1998). Form and substance in inservice teacher education. Madison, WI: University of Wisconsin, National Institute for Science Education. Retrieved from https://www.msu.edu/~mkennedy/publications/valuePD.html
- Kennedy, M. M. (2016). How does professional development improve teaching? Review of Educational Research, 86(4), 1–36. doi:10.3102/0034654315626800
- Kisa, Z., & Correnti, R. (2015). Examining implementation fidelity in America’s choice schools a longitudinal analysis of changes in professional development associated with changes in teacher practice. Educational Evaluation and Policy Analysis, 37(4), 437–457. doi:10.3102/0162373714557519
- Knapp, M. S. (2003). Professional development as policy pathway. Review of Research in Education, 27(1), 109–157. doi:10.3102/0091732X027001109
- Lewis, C., & Perry, R. (2017). Lesson study to scale up research-based knowledge: A randomized, controlled trial of fractions learning. Journal for Research in Mathematics Education, 48(3), 261–299. doi:10.5951/jresematheduc.48.3.0261
- McLaughlin, M. W. (1987). Learning from experience: Lessons from policy implementation. Educational Evaluation and Policy Analysis, 9(2), 171–178. doi:10.3102/01623737009002171
- McNeish, D., Stapleton, L. M., & Silverman, R. D. (2017). On the unnecessary ubiquity of hierarchical linear modeling. Psychological Methods, 22(1), 114–140. doi:10.1037/met0000078
- Moats, L. C., & Foorman, B. R. (2003). Measuring teachers content knowledge of language and reading. Annals of Dyslexia, 53, 23–45. doi:10.1007/s11881-003-0003-7
- Muthén, B. O., Muthén, L. K., & Asparouhov, T. (2016). Regression and mediation analysis using Mplus. Los Angeles, CA: Muthén & Muthén.
- Muthén, L. K., & Muthén, B. O. (1998–2015). Mplus user’s guide (7th ed.). Los Angeles, CA: Muthén & Muthén.
- National Council of Teachers of Mathematics. (1989). Curriculum and evaluation standards for school mathematics. Reston, VA: Author.
- National Council of Teachers of Mathematics. (2000). Principles and standards for school mathematics. Reston, VA: Author.
- Steen, L. A. (Ed.). (2001). Mathematics and democracy: The case for quantitative literacy. Washington, DC: National Council on Education and the Disciplines.
- National Governors Association Center for Best Practices, Council of Chief State School Officers. (2010). Common core state standards for mathematics. Washington, DC: Author.
- Penuel, W. R., Gallagher, L. P., & Moorthy, S. (2011). Preparing teachers to design sequences of instruction in earth systems science: A comparison of three professional development programs. American Educational Research Journal, 48(4), 996–1025. doi:10.3102/0002831211410864
- Powell, D. R., Diamond, K. E., Burchinal, M. R., & Koehler, M. J. (2010). Effects of an early literacy professional development intervention on head start teachers and children. Journal of Educational Psychology, 102(2), 299–312. doi:10.1037/a0017763
- Preacher, K. J., Curran, P. J., & Bauer, D. J. (2006). Computational tools for probing interactions in multiple linear regression, multilevel modeling, and latent curve analysis. Journal of Educational and Behavioral Statistics, 31(4), 437–448. doi:10.3102/10769986031004437
- President’s Council of Advisors on Science and Technology, Executive Office of the President. (2012). Engage to excel: Producing one million additional college graduates with degrees in science, technology, engineering, and mathematics. Retrieved from https://obamawhitehouse.archives.gov/sites/default/files/microsites/ostp/pcast-engage-to-excel-final_2-25-12.pdf
- Putnam, R., & Borko, H. (1997). Teacher learning: Implications of new views of cognition. In B. J. Biddle, T. L. Good, & I. F. Goodson (Eds.), The international handbook of teachers and teaching (pp. 1223–1296). Dordrecht, The Netherlands: Kluwer.
- Qualtrics. (2005–2015). Qualtrics software, version April 2014 – May 2015. Provo, UT: Author.
- Raudenbush, S. W. (2014). Random coefficient models for multi-site randomized trials with inverse probability of treatment weighting. Department of Sociology, Harris School of Public Policy, and Committee on Education University of Chicago. Retrieved from https://www.isr.umich.edu/cps/events/isqm/ITT%20manuscript%20Jan%202014%20revised%20april%2014.pdf
- Robins, J. M., Hernán, M. Á., & Brumback, B. (2000). Marginal structural models and causal inference in epidemiology. Epidemiology, 11(5), 550–560.
- Saderholm, J., Ronau, R., Brown, E. T., & Collins, G. (2010). Validation of the diagnostic teacher assessment of mathematics and science (DTAMS) instrument. School Science and Mathematics, 110(4), 180–192. doi:10.1111/ssm.2010.110.issue-4
- Schoen, R. C., LaVenia, M., & Tazaz, A. M. (2018). Effects of the first year of a three-year CGI teacher professional development program on grades 3–5 student achievement: A multisite cluster-randomized trial (Research Report No. 2018-25). Tallahassee, FL: Learning Systems Institute, Florida State University.
- Shulman, L. S. (1986). Those who understand: Knowledge growth in teaching. Educational Researcher, 15(4), 4–14. doi:10.3102/0013189X015002004
- Simon, M. A. (2013). Promoting fundamental change in mathematics teaching: A theoretical, methodological, and empirical approach to the problem. ZDM - the International Journal on Mathematics Education, 45(4), 573–582. doi:10.1007/s11858-013-0486-6
- Smith, M. S. (2000). Balancing old and new: An experienced middle school teacher’s learning in the context of mathematics instructional reform. Elementary School Journal, 100, 351–375. doi:10.1086/499646
- Spillane, J. P. (1999). External reform initiatives and teachers‘ efforts to reconstruct their practice: The mediating role of teachers‘ zones of enactment. Journal of Curriculum Studies, 31(2), 143–175. doi:10.1080/002202799183205
- Spillane, J. P., Reiser, B. J., & Reimer, T. (2002). Policy implementation and cognition: Reframing and refocusing implementation research. Review of Educational Research, 72(3), 387–431. doi:10.3102/00346543072003387
- Spillane, J. P., & Zeulli, J. S. (1999). Reform and teaching: Exploring patterns of practice in the context of national and state mathematics reforms. Educational Evaluation and Policy Analysis, 21(1), 1–27. doi:10.3102/01623737021001001
- Sykes, G., & Darling-Hammond, L. (1999). Teaching as the learning profession: Handbook of policy and practice. San Francisco: Jossey-Bass Publishers.
- U.S. Census Bureau. (2012). 2010 Census of population and housing. Washington, DC: Author.
- U.S. Department of Education, Institute of Education Sciences, What Works Clearinghouse. (2017a). What works clearinghouse: Procedures handbook (Version 4.0). Retrieved from https://ies.ed.gov/ncee/wwc/Handbooks
- U.S. Department of Education, Institute of Education Sciences, What Works Clearinghouse. (2017b). What works clearinghouse: Standards handbook (Version 4.0). Retrieved from https://ies.ed.gov/ncee/wwc/Handbooks
- Weatherly, R., & Lipsky, M. (1977). Street-level bureaucrats and institutional innovation: Implementing special-education reform. Harvard Educational Review, 47(2), 171–197. doi:10.17763/haer.47.2.v870r1v16786270x
- Yoon, K. S., Duncan, T., Lee, S. W. Y., Scarloss, B., & Shapley, K. (2007). Reviewing the evidence on how teacher professional development affects student achievement (Issues & Answers Report, REL 2007–033). Washington, DC: U.S. Department of Education, Institute of Education Sciences, National Center for Education Evaluation and Regional Assistance, Regional Educational Laboratory Southwest. doi:10.1094/PDIS-91-4-0467B
Appendix A: Inverse Probability Treatment Weights
Using the formulation presented in Equation (15) of Raudenbush (Citation2014; see also Robins, Hernán, & Brumback, Citation2000), we calculated marginal weights proportional to the inverse probability of treatment assignment:
where
is the inverse probability treatment weight (IPTW) for individual i in block j,
is a binary treatment indicator (
= 1 is assigned to treatment,
= 0 if assigned to control) for individual i in block j,
is the average probability of treatment assignment across the sample, and
is the average probability of treatment assignment for block j.
As a worked example, given the assignment of 93 participants to treatment and sample size of 180, the average probability of treatment assignment across the sample is 0.517. And given Block Z of 11 participants where 6 of whom were assigned to treatment, the average probability of assignment to treatment for Block Z would be 0.546. Accordingly,
indicates the estimated IPTW for an individual within Block Z assigned to treatment would be 0.947, and
indicates the estimated IPTW for an individual within Block Z assigned to control would be 1.063. Moreover, the oversampling for Block Z (the probability of assignment to treatment exceeded the sample average) results in the down-weighting of treatment cases in Block Z.
Conversely, for cases where the probability of assignment to treatment for a block fell below the average probability for the sample, the estimated IPTW for treatment cases would be >1 and the IPTW for the corresponding control cases would be <1, summing to n observed cases within that block.