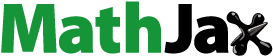
Abstract
This paper provides regional effects of land fragmentation on technical efficiency of 462 cassava farmers selected through a multitage sampling technique from South-West geopolitical zone of Nigeria. The analysis was based on cassava farmers’ 2015/2016 production season data, using the stochastic production frontier model. Mean farm holdings and plots size per farmer in the study area were 2.37 and 1.075 ha respectively. Results show that quantity of labour used, land size and quantity of planting material are influential determinants of technical efficiency of cassava farmers. The result further revealed that fragmentation index and distance between farm and farmstead are the only significant technical inefficiency variables. The study found that substantial technical inefficiency exists in cassava farming. The study recommends land reforms that directly targeted at cassava farmers in form of enhancement progamme to increase their production efficiency.
Public Interest Statement
Land is an indispensable input in agricultural production process. However, continual fragmentation of land occasion by traditional land tenure system or transfer of ownership through buying and selling may negatively affect farmers’ productivity. In this study, we investigated how reduction in land holding affects farmer’s ability to efficiently convert input to output among cassava farmers in South-West Nigeria. Results from study shows that substantial technical inefficiency exists in cassava farming in the study area. We therefore recommend that land reforms that directly targets cassava farmers in form of enhancement Progamme to increase production efficiency should be implemented in Nigeria.
1. Introduction
Cassava is the staple food for an estimated 800 million people worldwide. Grown almost exclusively by low-income, smallholder farmers, it is one of the few staple crops that can be produced efficiently on a small scale, without the need for mechanization or purchased inputs, and in marginal areas with poor soils and unpredictable rainfall. In 1999, Nigeria produced 33 million tonnes of cassava which was equivalent to 20% of global production, thus making it the world’s largest producer (Agricultural Research Council, Citation2014; Bamidele, Babatunde, & Rasheed, Citation2008). Cassava has been identified as a good staple whose cultivation if encouraged can provide the nationally required food security minimum of 2,400 calories per person per day (Food & Agricultural Organisation [FAO], Citation2000). However, land fragmentation may be a major bottleneck for improving productivity in cassava farming in Nigeria (Philip, Nkonya, Pender, & Oni, Citation2009), as found in other African countries (Bizimana, Nieuwoudt, & Ferrer, Citation2004; Musambayi, Citation2013). Land fragmentation can be defined as a situation where a farming household possesses several non-contiguous land plots, often scattered over a wide area. It implies a defective land tenure structure that often leads to major problem at various spatial scales which may hinder effective agricultural production and sustainable rural development (Demetriou, Stillwell, & See, Citation2012). Due to the traditional land tenure system, increasing population pressure, increasing urbanization and land fragmentation in the southern region of Nigeria; arable crops is cultivated in the marginal or less fertile land by smallholder farmers under traditional system of farming (Enete & Ubokudom, Citation2010; Udoh & Akpan, Citation2007).
Empirical research on the effect of land fragmentation on agricultural production efficiency at micro level is scanty in Nigeria. Available studies include Austin, Ulunma, and Sulaiman (Citation2012) who explored the link between land fragmentation and agricultural productivity in twelve communities in Umuahia-North Local Government Area in Abia State using data from 125 farm households. The results indicate negative impact of land fragmentation on agricultural productivity. Kakwagh, Aderonmu, and Ikwuba (Citation2011) investigated land fragmentation and agricultural development in Tivland of Benue State using 480 farm household across twenty-four (24) council wards. The study reveals that farmers’ land holdings in Tivland are fragmented, small in size, non-contiguous and interspersed over long walking distances. Findings from the study further shows that fragmentation of holdings has negative implications for agricultural development in the study area. Ghebru et al. (Citation2014), however assessed tenure security and demand for land tenure regularization in Nigeria. The study made use of 565 households from Kano and Ondo States. Findings from the study reveals that 48.4% of land parcels were inherited from relatives, while 42.8 and 8.6% were parcels from gift transfers and purchases, respectively. However, the dominance of access through inheritance is higher in Ondo state Nigeria than in Kano state in the Northern part and in urban areas than rural, while the dominance of access through gift is higher in Kano than in Ondo state and in rural areas than in urban areas.
Our focus in this paper is to examine the effect of land fragmentation on technical efficiency (TE), of cassava farmers. As far as we know, no research has empirically estimated the impact of land fragmentation on cassava production output in Nigeria, which justifies the need for this study. The specific objectives were to:
(i) | know the pattern of land holdings in the study area and | ||||
(ii) | investigate the determinants of technical efficiency of cassava farmers in the study area. |
2. Conceptual framework and literature review
Agricultural land fragmentation is prevalent in the world and it is commonly attributed to several factors such as inheritance laws, political system, historical antecedence and consolidation processes, and transaction costs in land markets, urban development policies, and personal valuation of land ownership (Blarel, Hazell, Place, & Quiggin, Citation1992; King & Burton, Citation1982). Land fragmentation, however, is more often believed to be one of the major problems existing in rural land management, especially in developing countries (Rusu, Citation2002; Wan & Cheng, Citation2001). Several studies have argued that the adverse effects of land fragmentation overshadow its possible benefits, primarily because it enlarges economic costs and reduces agricultural efficiency (Wan & Cheng, Citation2001). In particular, it is regarded as an obstacle to adoption of modern agricultural technologies, to construction and maintenance of rural infrastructure and thus as an obstacle to agricultural modernization. Production for commercial purposes (rather than own consumption) may only make sense if a certain scale of production is reached because commercialization comes with fixed costs of marketing (for example investment in drying equipment) and because traders require minimum amounts of product before entering into transactions.
There is some limited evidence available in the literature on the output and labour allocation effects of land fragmentation. Based on a Cobb-Douglas production function, Fleisher and Liu (Citation1992) estimated that land fragmentation led to inefficiency in agricultural production. This result was further reconfirmed by Nguyen, Cheng, and Findlay (Citation1996), who also established a positive relationship between plot size and output for major grain crops in China. While Tan, Heerink, Kruseman, and Qu (Citation2008) found that fragmented farm structures correlate with higher labour costs; it is not entirely clear why this implies that land consolidation does release rural labour, as the authors do not investigate the actual mechanisms of labour allocation any further.
2.1. Concept of land fragmentation
Land fragmentation is a phenomenon that exists when a household operates a number of owned or rented noncontiguous plots at the same time. Various factors are responsible for agricultural land fragmentation. Among the main factors that have directly or indirectly contributed to subdivision and fragmentation is the traditional system of inheritance of land (inheritance laws, which divide a family’s land among all the remaining sons, ensure that, as the population increases, not only does the size of holdings fall, but they are increasingly fragmented into small plots, scattered over a wide area (Gebeyehu, Citation1995). There are two approaches to measuring land fragmentation namely: single dimension indicators and integrated indicators. In the case of single dimension, one indicator is used to measure the extent of land fragmentation. Land fragmentation indicators like farm size, total number of plots in the farm, average plot size, distribution of plot sizes, spatial distribution of plots, and the shape of plots are commonly used in the literature (Bentley, Citation1987). Farm size is used to measure the total holding of a farm but among the remaining parameters, size and spatial distribution (i.e. distance) are often considered to be most significant (Shuhao, Citation2005). The integrated indicators capture the information from several single indicators into one index. The most popular index is the Simpson index (Blarel et al., Citation1992). The Simpson index (SI) measures the degree of land fragmentation in the following way:
where a is the area of each plot. The Simpson index is also located between 0 and 1. Contrary to the Jawanski Index (JI), a higher SI value corresponds with a higher degree of land fragmentation. The value of the Simpson index is also determined by the number of plots, average plot size and the plot size distribution. It also does not take farm size, distance and plot shape into account.
Studies in developing and developed countries indicated that land fragmentation affects the performance of farms (Tan, Heerink, Kuyvenhoven, & Qu, Citation2010), cost and benefit of the farm (Kawasaki, Citation2010), the technical efficiency of farmers (Rahman & Rahman, Citation2008) and profitability (Di Falco, Penov, Aleksiev, & van Rensburg, Citation2010).
3. Materials and methods
Data used for this study were collected during a household survey conducted in 2015 and 2016 in South-west geopolitical zone in Nigeria, covering the agricultural season of the year. The south-west zone part of Nigeria represents a geographical area covering between latitude 60 to the North and latitude 40 to the south. It is marked by longitude 40 to the West and 60 to the East and has a land area of approximately 114,271 km2 representing 12% of the country’s land mass. The total population is 27,581,992 and predominantly agrarian and more than 96% of the population is Yoruba (National Population Commission, Citation2006). The rainfall season is from March to October while dry season is from November to February. According to Obot, Chendo, Udo, and Ewona (Citation2010), Ibadan, which is the largest city in South West Nigeria and West Africa, recorded minimum, maximum and mean rainfall of 760 mm, 1,966 m and 1,321 mm respectively between the period 1978 and 2007. The vegetation pattern is central rainforest with a mean annual temperature of 26.6°C while the major types of livelihood activities in this area include farming, trading and government employment. Primary data used in this study were collected from cassava farmers using a multi-stage sampling technique. The first stage was the random selection of Osun and Ekiti States from the six States that make-up the zone. The second stage involved the random selection of two Agricultural Development Project (ADP) zones from each of the states. In the next selection, five villages were randomly selected from these ADP zones. The last stage of sampling involved the random selection of cassava farmers from these villages. Consequently, a random sample of 500 cassava farmers was selected, out of which only 462 farmers gave complete information required.
Analytical techniques employed include descriptive statistics and stochastic frontier production. Descriptive statistics: Descriptive statistics such as frequency tables, percentages means and standard deviations were used to analyze farmers’ socio-economic characteristics, land fragmentation, crop production and cropping system.
Sochastic frontier production function: A stochastic frontier production model proposed by Battese and Coelli (Citation1995) in accordance with the original models of Aigner, Lovell, and Schmidt (Citation1977); and Meeusen and van Den Broeck (Citation1977) is applied to cross-sectional data to determine the efficiency of the cassava farmers in the study area. The model was employed because it is capable of capturing measurement error and other statistical noise influencing the shape and position of the production frontier.
3.1. Model specification
This study uses the stochastic frontier production function as employed by Ajibefun (Citation2002). The stochastic frontier production function model has the advantage in that it allows simultaneous estimation of individual technical efficiency of the respondent farmers as well as determinants of technical efficiency (Battese & Coelli, Citation1995). Given our objectives we apply a Cobb-Douglas production function and the stochastic frontier is thus expressed as:
The model of the stochastic frontier production for the estimation of technical efficiency is specified as: (1)
(1)
where Y is output of the farmer 1, Xi is input, β is a vector of parameters to be estimated (including the efficiency parameter). The disturbance term ei consist of two components Vi and Ui.
Where Vi – N (O, σ2) and Ui is a one-sided error term. The two errors Vi and Ui. are assumed to be independently distributed. The term Vi. is the symmetric component and permits random variation of the production function across farms; while it also captures factors outside the control of the farmer. A one-sided component (Ui > 0) reflects technical efficiency relative to the stochastic frontier. If Ui = 0, production lies on the stochastic frontier, while if Ui > 0, production lies below the frontier and is inefficient.
The error term is assumed to follow one of three possible distributions (Bauer, Citation1990)
(i) | half-normal as U/N ( | ||||
(ii) | exponential as | ||||
(iii) | truncated normal at zero |
It follows(2)
(2)
where
In accordance with Jondrow, Lovell, Materov, and Schmidt (Citation1982), the Technical Efficiency (TE) of the individual farmer is calculated as the expected values of Vi conditional on εii = V–U that is:
Following Jondrow et al. (Citation1982), the Technical Efficiency (TE) of the individual farmer is calculated as the expected values of Vi conditional on εii = V–U that is:(3)
(3)
where E is the expectation of the farm operator, F* and f* are the values of the standard normal density and distribution functions respectively. Measures of technical efficiency (TE), technical efficiency is then calculated as:
So that 0 ≤ TE ≤ 1.(4)
(4)
The empirical model of the stochastic production frontier is specified as(5)
(5)
where Y = Output of the farmers in kg; X1 = Quantity of Labour input used in production in man-day; X2 = Farm Size in (ha); X3 = Quantity of planting material (kg); X4 = Quantity of fertilizer in (kg); X5 = Quantity of herbicides in litre; X6 = Cost of farm implement used e.g. hoe, cutlass, etc. (Naira); βi’s = Parameters to be estimated; ln’s = Natural Logarithms; Vi = The symmetric component that captures random error associated with random factor under the control of cassava farmers; Ui = The asymmetric error component represents the deviation from the frontier production (the technical inefficiency).
The inefficiency model:(6)
(6)
where Ui = Technical efficiency of the cassava farmers; Z1 = Age of farmer (years), Z2 = Sex (Male = 1, Female = 0), Z3 = Marital status (Married = 1, 0 = Otherwise), Z4 = Household size, Z5 = Years spent in school (Years); Z6 = Fragmentation Index, Z7 = Distance between plots (Kilometre), αi’s = these parameters were estimated in Frontier 4c.
4. Results and discussion
The socioeconomic characterisic of the cassava farmers is presented in Table . The result shows that majority (40%) of the farmers were in the age group of 41–50 years while about 3% were in age bracket greater than 60 years of age. An average age of farmers in the study area was 42.7 years old indicating that they are still within the active productive age. This has implication on available family labour and productivity of labour because age has a direct bearing on the availability of farm labour and the ease of adoption of new and improved agricultural practices by farmers. This result agrees with Rauf (Citation2010) that age of farmers has direct implication for labour productivity and improved farming practices.
Table 1. Socioeconomic characteristic of the cassava farmers
Most farmers (81.8%) in the study area are married. About 69.3% of the cassava farmers are male-headed households while the remaining are female. The result indicated that cassava production in the study area are dominated by male farmers. Education partly determines farmer’s stock of human capital and positively influenced the level of technical efficiency of farmers and hence; the greater is the stock of human capital, the better a farmer’s ability to organize the factors of production for maximum efficiency (Huffman, Citation1977; Lockheed, Jamison, & Lau, Citation1980; Osman, Binici, & Zulauf, Citation2009). However, majority (50.8%) of the farmers were educated to tertiary level while less than 7% had no formal education. In the case of household size of the farmersm 55.4% of cassava farmers in the study area had between 5–8 members while 22.9 and 21.7% of the farmers; had 1–4 and greater than 8 members respectively. The mean household size was high (7 members). This has implication for fragmentation of land in the area, because the tradition of the Yoruba that constitutes the majority of the respondents suggest that holdings of the farmers should be divided among the children farmer’s demise and hence will affect the techinical efficiency of the farmers.
The number of plots of land owned by cassava farmers in the study area is presented in Table . The result shows that most farmers (38.5%) had 2 plots of unequalled sizes located at different distances from each other while 20.8, 26.2 and 3.5% had 1, 3 and 5 plots of farm land respectively at different locations seperated by distance. The mean farm holdings of cassava farmers in the study area was 2.37 ± 1.01 plots per farmer while the mean plot size was 2.688 acres (1.075 ha).
Table 2. Number of plots owned and mean land size by cassava farmers in study area
The result of stochastic frontier model of cassava farmers in the study area is presented in Table . The maximum likelihood estimate of the Cobb-Douglas production function shows that the Lambda and Gamma values were 0.1391412 and 0.99999 respectively significant at 1% level. The values are significantly different from zero suggesting that the model is a good fit. The estimates show that quantity of labour used, farm size, and quantity of planting material are important inputs determining output of cassava in the study area. Quantity of labour used in the farm in manday has a negative and significant relationship with technical efficiency. Similarly, as the quantity of labour used in farm is increased by one man-day, the farmer technical efficiency will decrease by 0.13%. This shows that this resource is being overutilised and the production process is already in stage 3. The coefficient of farm size (0.0118) is negative indicating that 10% increase in area of land allocated to cassava reduced technical efficiency of farmers by 0.12%. In the case of cost of farm implement used (hoe, cutlass and bag), the coefficient is also negative and inelastic. A 10% increase in the farm implement will lead to decrease in technical efficiency of cassava farmers by 7.8%. For inefficiency variable, the significant variables are fragmentation index and distance between the plots. In case of fragmentation of land holdings, the coefficient is positive and significantly affected technical inefficiency of maize farmers in the study area. A unit increase in the fragmentation index increased technical efficiency of cassava farmers in the study area 0.15%. The positive effect of the fragmentation index on technical efficiency of farmers confims the results of Sherlund, Barrett, and Adesina (Citation2002) and Tan et al. (Citation2010) that technical effeciency is higher for farmers who cultivate more plots than few. The result disagrees with Dao (Citation2013) who claimed that there is a positive relationship between farm size and technical efficiency and a negative relationship between land fragmentation and efficiency. However, distance between plots is negative and significant. It shows that a kilometre increase in distance between farm plots increased technical efficiency by 0.04%. The result supports Sherlund et al. (Citation2002) and Tan et al. (Citation2010) that positive effect of the number of plots on technical efficiency exceeded the management effect of farmers.
Table 3. Result of stochastic frontier model of cassava farmers in the study area
The average technical efficiency score for the sample cassava farmers is shown in Table . The overall TE score for the sample was 0.81. The table shows that efficiency of the farmers ranged between 61.1 and 91.6% with a mean of 81.1%. Thus, they are all operating at high levels of efficiency given the available production technology to the farmers. The indication is that the realized output could be increased by about 19.9% by adopting the practices of the best farmer.
Table 4. The average technical efficiency score for the sample cassava farmers
4.1. Conclusion and policy recommendations
The study focuses on the effects of land fragmentation on technical efficiency of cassava farmers in south-west geopolitical zone, Nigeria. Most of the farmers were in their economically active age while only few were older than 60 years as at the time of the survey. On the average, farmers that participated in the study was 42.7 years old indicating that they are still within the active productive age. This has implication on available family labour and productivity of labour because age has a direct bearing on the technical efficiencies of the farmers since productivities tend to decline with age. The high mean household size of 7 members per household may have serious implications for fragmentation in the study area since Yoruba culture which constituted the majority of the respondents suggest that holdings of the farmers which include land should be divided among the children after the demise of farmer. The study also identified quantity of labour used in the farm, land size and cost of farm implement used as determinants of technical efficient of farmers. It further confirmed sources of inefficiency of the cassava farmers as land fragmentation index and farm distance to homestead. In light of these findings, it is consequently important to understand the determinants of technical efficiency to help inform policies on land use. The study recommends best resource allocation decision for efficient farms by farmers. Also there is a need for farmers to be sensitized on diseconomies of land fragmentation and costs that come with their continued practices.
Funding
The authors received no direct funding for this research.
Additional information
Notes on contributors
O.L. Balogun
Babatope Akinyemi is a post-doctoral research fellow in the Research Niche Area of Sustainable Agriculture and Food Security and a time-on-task lecturer in the Agricultural Economics and Extension Department, University of Fort Hare. He received his BAgric Tech Agricultural Economics and Extension (Hons) from the Federal University of Technology Akure (FUTA) in 2007, earned his MSc and PhD in Agricultural Economics from University of Ibadan and University of Fort Hare in 2012 and 2016 respectively. His most recent research has been focussing on the following; ecotourism, food security and NEET.
B.E. Akinyemi
Olubunmi Balogun is a lecturer in the Department of Agricultural Economics and Extension, School of Agriculture and Industrial Technology, Babcock University Ilishan-Remo, Ogun State Nigeria.
References
- Agricultural Research Council. (2014). Agricultural Research Council. Retrieved June 06, 2015 from http://www.arc.agric.za/arc-iic/Pages/Cassava.aspx
- Aigner, D., Lovell, C., & Schmidt, P. (1977). Formulation and estimation of stochastic frontier production function models. Journal of Econometrics, 6, 21–37.10.1016/0304-4076(77)90052-5
- Ajibefun, I. A. (2002). Analysis of policy issues in technical efficiency of small scale farmers using the stochastic frontier production function: With application to Nigerian farmers. Wageningen: Wageningen.
- Austin, O. C., Ulunma, A. C., & Sulaiman, J. (2012). Exploring the link between land fragmentation and agricultural productivity. International Journal of Agriculture and Forestry, 2(1), 30–34.10.5923/j.ijaf.20120201.05
- Bamidele, F. S., Babatunde, R., & Rasheed, A. (2008). Productivity analysis of cassava-based production systems in the Guinea Savanah: Case study of Kwara State, Nigeria. American-Eurasian Journal of Scientific Research, 3(1), 33–39.
- Battese, G., & Coelli, T. (1995). A model for technical inefficiency effects in a stochastic frontier production function for panel data. Empirical Economics, 20, 325–332.10.1007/BF01205442
- Bauer, P. W. (1990). Recent developments in the econometric estimation of frontiers. Journal of Econometrics, 46, 39–56.
- Bentley, J. (1987). Economic and ecological approach to land fragmentation: In defence of a Much Aligned phenomenon. Annual Review of Anthropology, 16, 31–67.10.1146/annurev.an.16.100187.000335
- Bizimana, C., Nieuwoudt, W., & Ferrer, S. (2004). Farm size, land fragmentation and economic efficiency in Southern Rwanda. Agrekon, 43(2), 244–262.10.1080/03031853.2004.9523648
- Blarel, B., Hazell, P., Place, F., & Quiggin, J. (1992). The economics of farm fragmentation: Evidence from Ghana and Rwanda. The World Bank Economic Review, 6, 233–254.10.1093/wber/6.2.233
- Dao, T. N. (2013). An analysis of technical efficiency of crop farms in the northern region of Vietnam (Thesis submitted in ffulfillment of the requirements for the degree of professional doctorate in business administration). Canberra: University of Canberra.
- Demetriou, D., Stillwell, J., & See, L. (2012). Landfragments: A new model for measuring land fragmentation FIG working week, knowing to manage the territory, protect the environment, evaluate the cultural heritage, Rome, Italy, 6–10 May 2012: s.n.
- Di Falco, S., Penov, I., Aleksiev, A., & van Rensburg, T. (2010). Agrobiodiversity, farm profits and land fragmentation: Evidence from Bulgaria. Land Use Policy, 27, 763–771.10.1016/j.landusepol.2009.10.007
- Enete, A., & Ubokudom, E., (2010). Economic of waterleaf production in Akwa Ibom State, Nigeria, s.l.: Field Actions Science Reports (online), 4.
- Fleisher, B., & Liu, Y. (1992). Economies of scale, plot size, human capital and productivity in Chinese agriculture. Quarterly Review of Economics and Finance, 32, 112–123.
- Food and Agricultural Organisation. (2000). Agriculture towards 2015/2030. Rome: Food and Agricultural Organisation.
- Gebeyehu, Y. (1995). Population pressure, agricultural land fragmentation and land use: A case study of dale and shashemene weredas, southern Ethiopia. Addis Ababa, s.n.
- Ghebru, H., Edeh, H., Ali, D., Deininger, K., Okumo, A., & Woldeyohannes, S. (2014). Tenure security and demand for land tenure regularization in Nigeria. Washington, DC: International Food Policy Research Institute.
- Huffman, W. E. (1977). Allocative efficiency: The role of human capital. The Quarterly Journal of Economics, 91, 59–77.10.2307/1883138
- Jondrow, J., Lovell, C., Materov, I., & Schmidt, P. (1982). On the estimation of technical inefficiency in stochastic frontier production function model. Journal of Econometrics, 19, 233–238.10.1016/0304-4076(82)90004-5
- Kakwagh, V., Aderonmu, J., & Ikwuba, A. (2011). Land fragmentation and agricultural development in tivland of Benue State, Nigeria. Current Research Journal of Social Sciences, 3(2), 54–58.
- Kawasaki, K. (2010). The costs and benefits of land fragmentation of rice farms in Japan. Australian Journal of Agricultural and Resource Economics, 54, 509–526.10.1111/j.1467-8489.2010.00509.x
- King, R., & Burton, S. (1982). Land fragmentation: “notes on a fundamental rural spatial problem”. Progress in Human Geography, 6(4), 475–494.10.1177/030913258200600401
- Lockheed, M. E., Jamison, D. T., & Lau, L. J. (1980). Farmer education and farm efficiency: A survey. Economic Development and Cultural Change, 29, 37–76.10.1086/451231
- Meeusen, W., & van Den Broeck, J. (1977). Efficiency estimation from Cobb-Douglas production functions with composed error. International Economic Review, 18, 435–444.10.2307/2525757
- Musambayi, N. J. (2013). The impact of land fragmentation/segmentation on production and food security (case study: Three major regions in Kenya). Elixir International Journal, 56, 13493–13495.
- National Population Commission. (2006). National population commission (2006): Nigerian census report.
- Nguyen, T., Cheng, E., & Findlay, C. (1996). Land fragmentation and farm productivity in China in the 1990s. China Economic Review, 7, 169–180.10.1016/S1043-951X(96)90007-3
- Obot, N. I., Chendo, M. A., Udo, S., & Ewona, I. (2010). Evaluation of rainfall trends in Nigeria for 30 years (1978–2007). International Journal of the Physical Sciences, 5(14), 2217–2222.
- Osman, K., Binici, T., & Zulauf, C. R. (2009). Assessing the efficiency of hazelnut production. African Journal of Agricultural Research, 4(8), 695–700.
- Philip, D., Nkonya, E., Pender, J., & Oni, O. A. (2009). Constraints to increasing agricultural productivity in Nigeria: A Review. Washington, DC: International Food Policy Research Institute.
- Rahman, S., & Rahman, M. (2008). Impact of land fragmentation and resource ownership on productivity and efficiency: The case of rice producers in Bangladesh. Land Use Policy, 26, 95–103.
- Rauf, M. (2010). Pattern of land use among the selected crop farmers in Osun State, Nigeria. Research Journal of Soil and Water Management, 1, 1–4.
- Rusu, M. (2002). Land fragmentation and land consolidation in Romania, Munich: Paper presented at the International CEEC Symposium, 25–28 February.
- Sherlund, S. M., Barrett, C. B., & Adesina, A. A. (2002). Smallholder technical efficiency controlling for environmental production conditions. Journal of Development Economics, 69, 85–101.10.1016/S0304-3878(02)00054-8
- Shuhao, T. (2005). Land fragmentation and rice production: A case study of small farms in Jiangxi Province, P. R. China (s.l.: Ph.D. Thesis). Wageningen University.
- Tan, S., Heerink, N., Kruseman, G., & Qu, F. (2008). Do fragmented landholdings have higher production costs? Evidence from ricefarmers in Northeastern Jianxi province, P.R. China. China Economic Review, 19, 347–358.10.1016/j.chieco.2007.07.001
- Tan, S., Heerink, N., Kuyvenhoven, A., & Qu, F. (2010). Impact of land fragmentation on rice producer’s technical efficiency in South-East China. NJAS-Wageningen Journal of Life Sciences, 57, 117–123.10.1016/j.njas.2010.02.001
- Udoh, E., & Akpan, S. (2007). Measuring technical efficiency of waterleaf (talinum triangulure) production in Akwa Ibom State, Nigeria. American Eurasian Journal of Agriculture and Environment Science, 518–522.
- Wan, G., & Cheng, E. (2001). Effects of land fragmentation and returns to scale in the Chinese farming sector. Applied Economics, 33, 183–194.10.1080/00036840121811