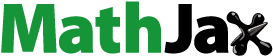
Abstract
Background: This study compared the participation of inter-provincial migrant and non-migrant South African youths in risk behaviors (RBs), and investigated the contextual and individual factors that influenced their participation in RBs.
Method: Data was from the National Income Dynamics Study (NIDS), wave 3 of 2014 which was a survey of individuals and households living in the nine provinces of South Africa. A total of 5590 young people aged 15–24 years were used for this study. Smoking of cigarettes and drinking of alcohol were used as the two outcome variables of risk behaviors. Data analysis was with univariate, bivariate, and multilevel logistic regression techniques.
Results: While 12.67% of the youths were smokers, 26.70% were drinkers. In addition, more of the non-migrants were engaged in smoking and drinking compared to migrants. With the exception of self-rated physical health and marital status, the results showed significant differences in smoking and drinking among migrants and non-migrants in other contextual and individual variables. Province of residence and living in urban areas significantly increased the odds of smoking and drinking among the migrants and non-migrants. Other contextual factors that increased the odds of drinking and smoking in the study area were poor mental health status, and good self-rated health of non-migrants (1.42; p < 0.05), and migrants (1.40; p < 0.05).
Conclusion: Intervention measures/strategies should include provision of counseling centers, improvement in the education of the youth and increased media reportage on the dangers of participation in these risk behaviors.
Public Interest Statement
Health risk behaviors (HRBs) are usually higher among the youth, and the persistence of these HRBs into adulthood can lead to morbidity and premature mortality. This study, therefore, compares the participation of migrant and non-migrant youth populations in South Africa in HRBs of smoking and drinking and estimates the factors that influence their participation in HRBs. The study showed that migrants participate more in HRBs compared to non-migrants. Rural/urban residence, self-rated physical health, mental health status age, race, employment status, and province of residence were important factors in the participation in smoking and drinking among the youth in South Africa.
Competing Interests
The authors declare that they have no competing interests.
1. Background
Migrants are usually different from non-migrants demographically, based on areas of origin and destination, and on the scale of migration and by socioeconomic characteristics such as health status and behaviors (Ajaero, Odimegwu, Chisumpa, & Obisie-Nmehielle, Citation2017; Darlington, Norman, & Gould, Citation2015). Subsequently, health status and behaviors influence the propensity and ability of individuals to migrate (Gatrell, Citation2011). In this context, the health of migrant populations is usually different from that of non-migrant populations, while the net flows of in- and out-migration have consequences on the overall health status of any area or region (Boyle, Citation2004; Norman, Boyle, & Rees, Citation2005). In addition, literature shows that the health of the migrants themselves may be affected, either negatively or positively, depending on the circumstances arising during and after the move itself (Ajaero et al., Citation2017; Darlington et al., Citation2015).
Consequently, after the initial healthy effect experienced by migrant populations (Rubalcava, Teruel, Thomas, & Goldman, Citation2008), the risks of poor health outcomes and behaviors seem to increase as the duration of migration also increases (Hesketh, Li, Jun, & Mei, Citation2007; Yang et al., Citation2009). While immigrant culture has been noted to minimize smoking and drinking risk behaviors in the United States of America (Ho & Elo, Citation2013), Newbold and Neligan (Citation2012) and Vang, Sigouin, Flenon, and Gagnon (Citation2015) found little evidence that immigrants in Canada were more likely than native-born populations to pick up risk behaviors and unhealthy habits, such as sedentary lifestyles, daily cigarette smoking, and drinking of alcohol. However, evidence from the literature indicates that internal migrants also suffer from acculturation stress just like the external migrants (Ajaero et al., Citation2017; Wan et al., Citation2011). In addition, the prevalence of cigarette smoking and alcohol drinking has been shown to be higher among internal migrant adolescents and young adults than among non-migrants in Vietnam and China (Gao, Li, Chan, Lau, & Griffiths, Citation2013).
These risk behaviors, which are associated with both migrant and non-migrant youths, result in short- and long-term negative health consequences which include various forms of cancer, cardiovascular disease and cognitive impairment (Hipple, Lando, Klein, & Winickoff, Citation2011; Morojele, Brook, & Brook, Citation2016). The risk behaviors of smoking cigarettes and drinking of alcohol have also remained preventable causes of death worldwide with about 6 million deaths attributable to cigarette smoking (Eriksen, Mackay, & Ross, Citation2012), and 3.3 million attributable to alcohol use (World Health Organization, Citation2014) each year. Consequently, engagement in these risk behaviors by the youths constitutes a major public health problem globally (Hipple et al., Citation2011; Morojele et al., Citation2016 World Health Organization, Citation2014) and in South Africa (Khan, Savahl, & Isaacs, Citation2016; Ramsoomar & Morojele, Citation2012 Seggie, Citation2012). However, there remains a dearth of research on the nature and determinants of these risk behaviors among migrant and non-migrant youths in South Africa.
In South Africa, internal migrations across provincial boundaries are mostly associated with males and young adults aged 20–39. Specifically, the youth aged 15–24 years made up 15% and 12.9% of migrant males and females, respectively, while they also constituted 9.7% and 9.8% of non-migrant males and females, respectively (Statistics South Africa, Citation2015). Also, data on inter-provincial migration stream in the 2001–2011 period showed that the provinces with the highest proportion of migrants were Gauteng (41.68%), Western Cape (14.81%) and North West (9.27%). On the other hand, the provinces with the lowest proportion of migrants were Limpopo (5.42%), Free State (4.29%), and Northern Cape (2.59%) (Statistics South Africa, Citation2015). In the 2011–2016 period, Gauteng province also had the highest net migration figure of 224,076 migrants while the figures for Eastern Cape, Limpopo, KwaZulu Natal, and Free State provinces that experienced negative net migration were Eastern Cape (36,973), Limpopo (29, 286), KwaZulu Natal (22,313), and Free State (5.018) (Statistics South Africa (StatSA), Citation2017).
Among South African youths, including those who migrated internally from one province to another, alcohol use has been associated with accidents and injury, interpersonal violence, illegal drug use, school failure, sexual risk behavior, low self-esteem, and depressive mood, especially among Black and Colored youth. (Panday, Priscilla Reddy, Ruiter, Bergström, & Hein, Citation2007; Wild, Flisher, Bhana, & Lombard, Citation2004). Cigarette smoking also remains a major cause of morbidity and mortality in South Africa (StatSA 2017), while the consequences of smoking and alcohol use in South Africa include numerous morbidities and mortality (Sitas et al., Citation2004), drug addiction (Viner et al., Citation2006), as well as epidemics of violence, tuberculosis, and HIV/AIDS (Seedat, Van Niekerk, Jewkes, Suffla, & Ratele, Citation2009; van Zyl Smit et al. Citation2010).
The 2002 National Risk Behavior Survey in South Africa showed that 29.3% of male and 17.9% of female students, in grades 9–11 have consumed five or more drinks in a row on one or more days in the past month preceding the survey (Reddy et al., Citation2003). Another report by the World Health Organization showed that South Africa has an unrecorded adult alcohol consumption prevalence of 46%, and the country ranks among the world’s highest levels of alcohol consumption with 16.6 l of pure alcohol per drinker yearly (Parry, Citation2005; Rehm et al., Citation2003). Also, the cost of alcohol consumption in South Africa in 2000/2001 constituted about 1% of the country’s gross domestic product (GDP), an equivalent of about R8.7 billion (US$ 1.4 bn.) (Parry, Myers, & Thiede, Citation2003). Recently, the South Africa Demographic and Health Survey of 2016 showed that 20.9% and 49.3% of women and men, respectively, who are aged 15–24 years drank alcohol 12 months prior to the survey while 6% of women and 30% men smoke cigarettes daily (StatSA, Citation2017).
The prevalence of these risk behaviors among young people has been attributed to anxiety, depression, and the resultant mental illness (De Matos et al., Citation2012). Mental illness, which used to be confined to industrialized societies, has become an increasing health concern in developing countries (Ajaero et al., Citation2017; Miller, Citation2006). While poor mental health status constitutes a major cause of disability in low-income countries, with depression constituting the heaviest disease burden (Lundberg et al., Citation2011), it has been noted that risky behaviors such as unsafe sex, alcohol abuse, alcohol, and tobacco smoking were responsible for over 50% of South Africa’s total burden of disease (Norman et al., Citation2007).
Factors linked to risk behaviors by the youth include individual demographic characteristics, socioeconomic status of households, parental involvement and attitudes toward alcohol drinking, self-rated physical health and mental health status. For instance, studies have shown that while higher socioeconomic status lowers the odds of smoking, it increases the odds of alcohol consumption among students and young people in Vietnam (Arunachalam & Nguyen, Citation2016). Moreover, rural youth have been found to engage more in drinking of alcohol than urban youth, while the occurrence of these risk behaviors is higher among the people aged 18–25, especially the male gender (Gale, Lenardson, Lambert, & Hartley, Citation2012).
In addition, studies have shown that based on their peculiar biological, social, environmental, economic, and cultural characteristics, health inequalities exist between various age groups, and between different geopolitical regions among migrants and non-migrants (Ajaero et al., Citation2017; Fang et al., Citation2010; Marmot & Wilkinson, Citation2009; Riva, Curtis, & Norman, Citation2011; Zatonski, Citation2007; Zatonski & Jha, Citation2000). Furthermore, literature showed that disparities in health issues, diseases, and mortality occur on a range of spatial scales over time periods, and as such, the geography of any location contributes significantly to health inequalities among migrants and non-migrants (Copeland, Kasim, & Bambra, Citation2014; Griffiths & Fitzpatrick, Citation2001; Pearce, Citation2003; Smith & Easterlow, Citation2005).
There exists a greater propensity for young males compared to young females, to consume alcohol and smoke cigarettes (Diep, Knibbe, Giang, & De Vries, Citation2013; Liem, Rahman, Emerson, Nguyen, & Zabin, Citation2012). Studies show that while lower educational status increases the likelihood of alcohol consumption (Hoang, Vung, Tien, & Plant, Citation2013), the odds of engagement in risk behaviors by young people increase with age (Myers, Citation2010). Race, ethnicity, and places of residence have also been associated with varying tendencies of risk behaviors by the youth in Western Cape Province of South Africa and in Croatia (Ewing, Venner, Hilary, & Bryan, Citation2011; Khan et al., Citation2016; Peltzer, Davids, & Njuho, Citation2011; Simetin et al., Citation2011). For instance, Ewing et al. (Citation2011) noted that white youth are more likely to consume cigarettes and alcohol compared to their black counterparts.
These risk behaviors of smoking and drinking in South Africa have been linked to about 44% of traffic injuries and homicides as well as depression, low self-esteem, or poor general health among the adult population (Fernander et al., Citation2006). Consequently, it is important to curtail these risk behaviors in South Africa, especially among young people who usually migrate from one province of the country to other provinces so as to reduce the burden of disease and ameliorate the consequent suffering of individuals and their families who are struggling with these problems.
Unfortunately, studies comparing the participation in risk behaviors by the inter-provincial migrant and non-migrant youths is lacking in the country. Also, studies on youth risk behaviors in other countries have concentrated on the individual factors of participation in these risk behaviors, thereby ignoring the influence of the contextual factors that could predispose the youth to these risk behaviors. In addition, most studies on internal migration concentrated on rural-urban migration, thereby ignoring the effects of inter-provincial migrations of youth behavior. In this context, the appraisal of the contextual and individual factors of risk behaviors of the youth in South Africa is germane in the comprehension and management of the inter-provincial migration and risk behavior linkages.
Therefore, the aim of this study is to examine the contextual and individual factors of risk behaviors among the inter-provincial migrant and non-migrant youth populations in South Africa. The research questions for this study in South Africa are; are risk behaviors by the youth dependent on their inter-provincial migration status; and what are the contextual and individual factors that influence participation in risk behaviors among the inter-provincial migrant and non-migrant youth populations?
2. Methods
2.1. Data source and study population
This study used data from the National Income Dynamics Study (NIDS), wave 4 of 2014 which was a survey of individuals and households living in the nine provinces of South Africa. For this study, a total of 6,377 youth aged 15–24 years comprising of 3,059 males and 3,318 females were used.
2.2. Outcome variables
The study made use of two dependent/outcome risk behavior variables of smoking of cigarettes and drinking of alcohol. The respondents were asked if they were involved in (i) smoking of cigarettes, and (ii) drinking of alcohol. Their responses were then grouped into 1 = yes if they were involved in any of these risk behaviors and 0 = no if they were not involved in these risk behaviors.
2.3. Exposure variables
In terms of exposure, inter-provincial migration status was categorized as 1 = migrant, and 0 = non-migrant (migrant or non-migrant). To derive the inter-provincial migration status, the variables of “place of previous residence” in the three years prior to the survey and the “place of present residence” at the time of the survey were used. Consequently, respondents whose place of previous residence was different from their place of present residence were categorized as inter-provincial migrants while respondents whose place of previous residence was the same as their place of present residence during the 2012 NIDS survey were categorized as inter-provincial non-migrants.
2.4. Individual variables
Individual-level explanatory variables used were gender (male/female), age (15–17 years/18–20 years/21+ years), education (none/primary, secondary, tertiary), marital status (never married/married), race (black African, white, Asian/Indian, Colored), employment status (unemployed/employed), self-rated physical health (good/poor), and mental health status (good/poor)
The self-rated physical health variable was derived from responses to the question that asked how the youth felt about their physical health status in the week preceding the survey. The responses were in five categories of excellent, very good, good, fair and poor health status. These responses were then recoded in two categories; “good” (comprising of excellent, very good, and good) and poor (comprising of fair and poor health) self-rated physical health.
In determining the mental health status of respondents, responses from the “Center for Epidemiological Studies Short Depression Scale” (CES-D 10), which asked 10 questions on whether certain feelings occurred; (i) rarely or none of the time, (ii) some or little of the time, (iii) occasionally or a moderate amount of time, and (iv) all of the time, within the week preceding the survey were used. Responses from these questions were then scored from “0” (rarely or none of the time) to “3” (all of the time). The exception to this scoring were questions “5” (I feel hopeful about the future) and “8” (I was happy) which were reverse coded on the Likert scale as “3” (rarely or none of the time), and “0” (all of the time). Subsequently, the responses from all the ten items in the CES-D 10 scale were added up to generate the mental health variable. Thereafter, using the scoring specification of the CES-D 10 scale, the mental health variable was divided into a dichotomous variable of mental health status of “poor” (with the value of 1) or “good” mental health status (with a value of 0). In classifying the mental health status, values of more than 10 from the mental health variable were categorized into poor mental health status while values of 0 to 10 were categorized as good mental health status. In the context of this study, the term mental health status refers to a measure of a continuum of psychological distress (symptoms of depression and anxiety) and not to examine the presence or absence of psychiatric disorders (Ajaero et al., Citation2017; Ardington & Case, Citation2010).
2.5. Contextual variables
The community-level variables used in this study were place of residence (rural/urban residence), province of residence which were categorized into the nine provinces in South Africa.
2.6. Data analysis
All analyses were carried out on two categories of the youth population, i.e., the inter-provincial migrants and non-migrants. Univariate analysis was used to describe the characteristics of the risk behavior variables, while bivariate analysis which utilized Chi-square was used to appraise the risk behavior variables relative to the control independent variables of the population of study. Finally, multilevel binary logistic regression models with a confidence level of 95% were used to determine the influence of individual-level and contextual-level explanatory independent variables on the risk behavior outcome variables.
For each of the outcome variables (smoking and drinking), there were three models. Model 1 was the empty/null model (no explanatory variable was added). It included only a random intercept and was intended only to decompose the total variance into its individual and contextual components and to identify the existence of possible contextual phenomenon for the risk behavior outcomes. Model 1 according to Anyadike (Citation2009) is as follows:
where y represents the dichotomous dependent variable of risk behavior, α is the gradient, and β0 is the intercept/constant term
Model 2 contained only individual-level explanatory variables and was used to access how the individual-level variables influenced participation in risk behaviors. According to Anyadike (Citation2009), model 2 is of the form;
where y represents the dichotomous dependent variable of risk behavior, α is the gradient, βs are the coefficients, x…..xn are the independent variables (such as age, sex, and education) and εi is the error term with confidence level of 95% and 5% allowance of error.
Finally, model 3 contained both the contextual-level and individual-level explanatory variables in order to examine their combined effects on risk behaviors and based on Anyadike (Citation2009), this model is represented with;
where y represents the dichotomous dependent variable of risk behavior, α is the gradient, βs are the coefficients, x…..xn are the individual variables (such as age, sex, and education), U8 and U9 represent the contextual variables of place of residence and the provinces, and εi is the error term with confidence level of 95% and 5% allowance of error.
The fixed effects section of the models (measures of association) contained individual-level and contextual-level factors, and the results were expressed as odds ratio (OR) at 95% confidence intervals (95% CI). All the analyses were performed using Stata version 14.0
3. Ethics approval
Ethical approval for this study was obtained from the Human Research Ethics Committee of the University of Cape Town.
4. Results
4.1. Characteristics of the study population
Males made up 47.97% of the respondents while 36.82% of the youths were aged 21 years and above. Majority of the youths (88.84%) were not employed while 81.89% had secondary education (Table ). KwaZulu-Natal province had the greatest proportion of respondent samples, while the least proportion of respondents was sampled in Free State Province.
Table 1. Characteristics of the study population
4.2. Engagement in risk behaviors
A total of 26.70% of the youths were drinkers. More of non-migrants (27.52%) compared to migrants (25.81%) were drinkers. With regard to smoking, 12.67% of the youths comprising 13.37% of non-migrants and 11.91% of migrants were smokers. In other words, non-migrants were involved in risk behaviors compared to the migrants in the country. The bivariate results of individual factors associated with risk behaviors by the youth showed significant differences in smoking among non-migrants and migrants in all the individual-level variables (Table ). For instance, 19.54% of non-migrants smoked cigarettes as against 4.49% of the female non-migrants. Also, 19.60% and 4.26% of male and female migrants, respectively, were smokers. Furthermore, youths aged 21+ years were involved in risk behaviors of smoking for both the migrants and the non-migrants. While 18.97% of non-migrants smoke cigarettes, 18.33% of migrants were involved in smoking. However, more of the migrants (31.44%) compared to 29.95% of the non-migrants who were employed smoked cigarettes. Also, more of the youths with primary education were involved in the risk behavior of smoking among the migrants and non-migrants
Table 2. Individual factors associated with smoking
With regard to drinking, the results showed significant differences in all the individual factors associated with drinking in the study area (Table ). Generally, more of the non-migrants were involved in the risk behavior of drinking compared to the migrants. For instance, more of the males were involved in drinking compared to the females. As such, the results showed that at least 30% of the migrant (30.85%) and non-migrant (31.29%) males drank alcohol. However, more of the migrants (46.25%) compared to 44.09% of the non-migrants who were employed smoked cigarettes.
Table 3. Individual factors associated with drinking
The bivariate results showed significant differences among migrants and non-migrants in all the contextual factors associated with smoking with the exception of self-rated physical health (Table ). Specifically, more urban non-migrants (17.46%) and migrants (17.38%) smoked cigarettes. In addition, more of the non-migrants (17.04%) compared to 15.66% of migrants with poor mental health status smoked cigarettes. Significant differences also existed in all the factors associated with drinking for the migrants. However, for the non-migrants, all the factors showed significant differences except self-rated physical health (Table ). While more urban non-migrants (30.64%) and migrants (30.39%) drank alcohol, more migrants (24.53%) and non-migrants (24.43%) with good self-rated physical health were involved in drinking of alcohol. Furthermore, more non-migrants (29.69%) and migrants (29.40%) with poor mental health status were involved in drinking of alcohol.
Table 4. Contextual factors associated with smoking
Table 5. Contextual factors associated with drinking
4.3. Contextual- and individual-level predictors of risk behaviors
Model 1 is the baseline model of smoking without any explanatory variables (Table ). The results show that the youth in South Africa exhibited lower odds of engaging in smoking for non-migrants (0R = 0.09; p < 0.000), and for migrants (OR = 0.07; p > 0.000). For drinking, the results showed odds ratio for non-migrants (OR = 0.07; p < 0.000), and for migrants (OR = 0.20; p < 0.000) (Table ). The ICC (ρ) obtained for smoking was 29% for non-migrants and 37% for migrants. With regard to drinking, the ICC (ρ) was 37% for non-migrants and 35% for migrants.
Table 6. Multilevel logistic results of smoking
Table 7. Multilevel logistic results of drinking
The adjustments for only individual-level factors in Model 2 still showed decreased odds of smoking among non-migrant and migrant youth in the study area. Race, being employed, self-rated physical health, and increase in age were significantly associated with increased odds of smoking among the non-migrant and migrant populations. With regard to drinking, increase in age, being employed, education, self-rated physical health, and race had increased odd for the non-migrants and the migrants.
In the final models which included both the contextual- and individual-level factors, the odds of smoking for the non-migrants (OR = 0.44; p < 0.05) and the migrants (OR = 0.10; p < 0.000) still decreased. Age, race, good self-rated health, urban residence and being employed were the variables which significantly increased the odds of smoking among the non-migrants, while increase in age, race, being employed, being a resident of urban area, and living in Free State significantly increased the odds of smoking among the migrant youth.
5. Discussion
The high values of intra-cluster correlations observed in the results emphasize the importance of contextual/community variables in the participation of the youth in risk behaviors of smoking and drinking. With regards to smoking, the effects of contextual variables were more pronounced in influencing the migrants compared to the non-migrants. Conversely, the effects of contextual variables were more influential with regard to drinking among the non-migrants as against the migrants.
Generally, the odds of smoking and drinking were higher for migrants compared to non-migrants in the study area. This agrees with earlier findings which reported that smoking and drinking were more common among internal migrants in Hanoi and China (Gao et al., Citation2013). These higher odds of risk behaviors among migrants can be explained by the fact that the migrants mix—up with people from different backgrounds with different behaviors, and the youth can easily be affected by peer pressure to engage in various anti-social behaviors. In addition, dearth of parental and cultural control which is usually the norm in urban areas make it easier for the youth to engage in deviant behaviors since there is no one to effectively monitor their activities. Our findings also support the view that males are more involved in smoking and drinking compared to females (Arunachalam & Nguyen, Citation2016; Diep et al., Citation2013; Liem et al., Citation2012; Shafey, Eriksen, Ross, & Mackay, Citation2009), and that the younger the age, the more likely it is for the youth to engage in risk behaviors (Gale et al., Citation2012; Hoang et al., Citation2013; Qian et al., Citation2010). The young people buoyed with youthful exuberance and without much experience, are more likely to try out some risk behaviors. Moreover, they have the belief that such behaviors can hardly bring them any harm, even as they may see participation in such behaviors as evidence that they are old enough to engage in adult activities. Since males are more adventurous and more prone to risk-taking, they are more likely to engage more in risk behaviors compared to their less adventurous female counterparts.
The race of the youth in South Africa is also an important predictor of engagement in risk behaviors (Ewing et al., Citation2011; Panday et al., Citation2007; Peltzer et al., Citation2011; Simetin et al., Citation2011). In this study, the Colored youth followed by the Whites were more likely to engage in smoking for the non-migrant population while for the migrant population, the Whites followed by the Colored had more odds of engaging in smoking. Furthermore, the Whites had more odds of drinking compared to other racial groups among both the migrant and non-migrant populations. The outcome of this study also agrees with other results which reported that rural youths engage more in drinking of alcohol than urban youth (Hoang et al., Citation2013).
However, our results disagree with earlier research which found that people with lower levels of education were more involved in smoking (Yang et al., Citation2008), and that depression is associated with increase in risk behaviors (Wild et al., Citation2004; Panday et al., Citation2007; De Matos et al., Citation2012). In rural areas, there may be more traditional festivities such as burials in which free alcohol in large quantities is available and this can easily predispose the rural youth to drink more since they do not need to pay for it. With regard to smoking, young people with higher education may be from families with higher socioeconomic status, and subsequently, they will be more capable to afford buying and smoking more than their counterparts in families with lower socioeconomic status.
6. Conclusion
This study had shown that while the inter-provincial migrant and non-migrant youth who live in urban areas were more involved in smoking, the inter-provincial migrant and non-migrant youth who live in rural areas were more involved in alcohol consumption. The study has also identified other contextual- and individual-level factors that predispose the youth to risk behaviors such as age, race, employment status, and perception of their mental and physical health status. Based on the findings of this study, intervention measures/strategies that will help reduce these risk behaviors should be targeted at groups of youth with a higher likelihood of engaging in these behaviors. These intervention measures should include a provision of counseling centers, improvement in the education of the youth and increased media reportage on the dangers of participation in these risk behaviors.
7. Limitations of the study
This study faced several limitations such as the paucity of data on international migrants. This could have led to a comparative analysis of the non-migrants, internal migrants, and the international migrants. Also, there was no data on either rural-urban or urban-rural migration, which could also have provided a basis for a more robust comparison. The data on internal migration also has the deficiency of not providing information on repeat or circular migration while there are cases of missing values where respondents did not answer correctly to the questions. The inclusion of these missing variables in future surveys, will go a long way in ensuring the comprehensiveness of further studies on the relationships between migration, and risk behaviors among the youth in South Africa.
Abbreviations
NIDS | = | National Income Dynamics Study |
CES-D | = | Center for Epidemiological Studies Short Depression Scale |
RB | = | Risky Behaviors |
StatSA | = | Statistics South Africa. |
Authors’ contributions
CKA conceived the study, carried out data analysis and wrote the first draft of the manuscript. COO gave critical comments on the initial draft. SM checked the analysis of the data. OOB helped in data analysis contributed to the preparation of the final manuscript. All authors read and approved the final manuscript.
Availability of data and materials
The datasets used and analyzed during the current study are available at www.nids.uct.ac.za.
Consent for publication
All authors consented to the publication of this article.
Acknowledgements
The authors gratefully acknowledge the use of the research facilities of the Demography and Population Studies Programme, University of the Witwatersrand, South Africa in conducting this study. This work is based on the research supported in part by the National Research Foundation of South Africa (Grant Number: 105931).
Additional information
Funding
Notes on contributors
Chukwuedozie K. Ajaero
Chukwuedozie K. Ajaero, PhD is a Senior Lecturer, Department of Geography, University of Nigeria Nsukka. His research interests are in migration, adolescent health, climate change and livelihoods.
C.O. Odimegwu
Clifford O. Odimegwu, PhD is a Professor and Head, Demography and Population Studies at the University of the Witwatersrand in Johannesburg, South Africa. His research interests cover the broad field of demography, sexual and reproductive health and gender issues
Sibusiso Mkwananzi
Sibusiso Mkwananzi, MD is an associate lecturer and PhD student within the Demography and Population Studies Programme, University of the Witwatersrand I Johannesburg, South Africa. Her research interests include sexual and reproductive health, youth-related studies and development.
O. Banjo
Olufunmilayo Banjo, PhD is a Lecturer, Demography and Social Statistics Department, Obafemi Awolowo University, Ile Ife, Nigeria. Her research interests include reproductive health and gender issues
References
- Ajaero, C. K., Odimegwu, C. O., Chisumpa, V., & Obisie-Nmehielle, N. (2017). The influence of internal migration on mental health status in South Africa. International Journal of Mental Health Promotion. doi:10.1080/14623730.2017.1327879
- Anyadike, R. N. C. (2009). Statistical methods for the social and environmental sciences. Ibadan, Nigeria: Spectrum Books.
- Ardington, C., & Case, A. (2010). Interactions between mental health and socioeconomic status in the South African national income dynamics study. Tydskrif Vir Studies in Ekonomie En Ekonometrie= Journal for Studies in Economics and Econometrics, 34(3), 69.
- Arunachalam, D., & Nguyen, D. Q. V. (2016). Family connectedness, school attachment, peer influence and health-compromising behaviours among young Vietnamese males. Journal of Youth Studies, 19(3), 287–304. doi:10.1080/13676261.2015.1067674
- Boyle, P. (2004). Population geography: Migration and inequalities in mortality and morbidity. Progress In Human Geography, 28, 767e776. doi:10.1191/0309132504ph518pr
- Copeland, A., Kasim, A., & Bambra, C. (2014). Grim up north or northern grit? Recessions and the English spatial health divide (1991 – 2010). Journal of Public Health, 1–6. doi:10.1093/pubmed/fdu019
- Darlington, F., Norman, P., & Gould, M. (2015). Health and internal migration. In D. P. Smith, N. Finney, K. Halfacree, & N. Walford (Eds.), Internal migration: Geographical perspective and processes (pp. 113–128). Farnham: Ashgate.
- Diep, P. B., Knibbe, R. A., Giang, K. B., & De Vries, N. (2013). Alcohol-related harm among university students in Hanoi, Vietnam. Global Health Action, 6, 1–10.
- Eriksen, M., Mackay, J., & Ross, H. (2012). The Tobacco Atlas. Fourth. Atlanta, GA: American Cancer Society; New York, NY: World Lung Foundation.
- Ewing, S. W. F., Venner, K. L., Hilary, K. M., & Bryan, A. D. (2011). Exploring racial/ethnic differences in substance use: A preliminary theory-based investigation with Juvenile justice involved youth. BMCPediatrics, 11, 71. doi:10.1186/1471-2431-11-71
- Fang, P., Dong, S., Xiao, J., Liu, C., Feng, X., & Wang, Y. (2010). Regional inequality in health and its determinants: Evidence from China. Health Policy (Amsterdam, Netherlands), 94(2010), 14–25. doi:10.1016/j.healthpol.2009.08.002
- Fernander, A. F., Flisher, A. J., King, G., Noubary, F., Lombard, C., Price, M., & Chalton, D. (2006). Gender differences in depression and smoking among youth in Cape Town, South Africa. Ethnicity and Disease, 16(1), 41.
- Gale, J. A., Lenardson, J., Lambert, D., & Hartley, D. (2012). Adolescent alcohol use: Do risk and protective factors explain rural-urban differences? Working Paper 48, Maine Rural Health Research Center, University of Southern Maine.
- Gao, Y., Li, L., Chan, E. Y. Y., Lau, J., & Griffiths, S. M. (2013). Parental migration, self-efficacy and cigarette smoking among rural adolescents in South China. PloS one, 8(3), e57569. doi:10.1371/journal.pone.0057569)
- Gatrell, A. C. (2011). Mobilities and health. Farnham: Ashgate.
- Griffiths, C., & Fitzpatrick, J. (eds). (2001). Geographic variations in health. London: The Stationery Office.
- Hesketh, T., Li, L., Jun, Y. X., & Mei, W. H. (2007). Smoking, cessation and expenditure in low income Chinese: Cross sectional survey. BMC Public Health, 7(1), 1. doi:10.1016/j.socscimed.2011.09.030
- Hipple, B., Lando, H., Klein, J., & Winickoff, J. (2011). Global teens and tobacco: A review of the globalization of the tobacco epidemic. Current Problems in Pediatric and Adolescent Health Care, 41, 216–230. doi:10.1016/j.cppeds.2011.02.010 PubMed: 21821205
- Ho, J. Y., & Elo, I. T. (2013). The contribution of smoking to black-white differences in U.S. mortality. Demography, 50, 545–568. doi:10.1007/s13524-012-0159-z
- Hoang, D. H., Vung, N. D., Tien, P. H., & Plant, M. A. (2013). Alcohol use, risk taking, leisure activities and health care use among young people in Northern Vietnam. Central Asian Journal of Global Health, 2(2), 1–16.
- Khan, G., Savahl, S., & Isaacs, S. (2016). Adolescents’ perceptions of the ‘substance use- violence nexus’: A South African perspective. Journal of Youth Studies. doi:10.1080/13676261.2016.1145635
- Liem, T. N., Rahman, Z., Emerson, M. R., Nguyen, M. H., & Zabin, L. S. (2012). Cigarette smoking and drinking behaviour of migrant adolescents and young adults in hanoi, vietnam. Journal of Adolescent Health, 50(3), S61–S67. doi:10.1016/j.jadohealth.2011.12.004
- Lundberg, P., Rukundo, G., Ashaba, S., Thorson, A., Allebeck, P., Östergren, P.-O., & Cantor-Graae, E. (2011). Poor mental health and sexual risk behaviours in Uganda: A cross-sectional population-based study. BMC Public Health, 11(1), 1. doi:10.1186/1471-2458-11-125
- Marmot, M., & Wilkinson, R. G. (eds). (2009). Social determinants of health. Oxford: Oxford University Press.
- Matos, D., Gaspar, M., Gaspar, T., Ferreira, M., Tomé, G., InêS, C., … Lucia, R. (2012). Keeping a focus on self-regulation and competence:‘ find your own style’, a school based program targeting at risk adolescents. Journal of Cognitive & Behavioral Psychotherapies, 12, 1.
- Miller, G. (2006). The unseen: Mental illness’s global toll. Science, 311(5760), 458–461. doi:10.1126/science.311.5760.458
- Morojele, N. K., Brook, J. S., & Brook, D. W. (2016). Tobacco and alcohol use among adolescents in South Africa: Shared and unshared risks. Journal of Child and Adolescent Mental Health, 28(2), 139–152. doi:10.2989/17280583.2016.1200586
- Myers, L. L. (2010). Health risk behaviours among adolescents in the rural south: A comparison of race, gender, and age. Journal of Human Behaviour in the Social Environment, 20(8), 1024–1037. doi:10.1080/10911359.2010.498675
- Newbold, K. B., & Neligan, D. (2012). Disaggregating Canadian immigrant smoking behaviour by country of birth. Social Science & Medicine, 75, 997–1005. doi:10.1016/j.socscimed.2012.05.008
- Norman, P., Boyle, P., & Rees, P. (2005). Selective migration, health and deprivation: A longitudinal analysis. Social Science & Medicine, 60, 2755e2771. doi:10.1016/j.socscimed.2004.11.008
- Norman, R., Bradshaw, D., Schneider, M., Joubert, J., Groenewald, P., Lewin, S., … Nannan, N. (2007). A comparative risk assessment for South Africa in 2000: Towards promoting health and preventing disease. South African Medical Journal, 97(8), 637–641.
- Panday, S., Priscilla Reddy, S., Ruiter, R. A. C., Bergström, E., & Hein, D. V. (2007). Determinants of smoking among adolescents in the Southern Cape-Karoo region, South Africa. Health Promotion International, 22(3), 207–217. doi:10.1093/heapro/dam018
- Parry, C. D. H. (2005). South Africa: Alcohol today. Addiction, 100(4), 426–429. doi:10.1111/j.1360-0443.2005.01015.x
- Parry, C. D. H., Myers, B., & Thiede, M. (2003). The case for an increased tax on alcohol in South Africa. South African Journal of Economics, 71(2), 265–281. doi:10.1111/j.1813-6982.2003.tb01308.x
- Pearce, J. (2003). Emerging new research in the geography of health and impairment. Health and Place, 9, 107–108. doi:10.1016/S1353-8292(03)00016-9
- Peltzer, K., Davids, A., & Njuho, P. (2011). Alcohol use and problem drinking in South Africa: Findings from a national population-based survey. African Journal of Psychiatry, 14(1), 30–37. doi:10.4314/ajpsy.v14i1.65466
- Qian, J., Cai, M., Gao, J., Tang, S., Ling, X., & Critchley, J. A. (2010). Trends in smoking and quitting in China from 1993 to 2003: National health service survey data. Bulletin of the World Health Organization, 88(10), 769–776. doi:10.2471/BLT.09.064709
- Ramsoomar, L., & Morojele, N. K. (2012). Trends in alcohol prevalence, age of initiation and association with alcohol-related harm among South African youth: Implications for policy. South African Medical Journal, 102(7), 609–612. PubMed: 22748438
- Reddy, S. P., Panday, S., Swart, D., Jinabhai, C. C., Amosun, S. L., James, S., … Kambaran, N. S. (2003). Umthenthe uhlaba usamila–the South African youth risk behaviour survey 2002. Cape Town: South African Medical Research Council; 2003.
- Rehm, J., Rehn, N., Room, R., Monteiro, M., Gmel, G., Jernigan, D., & Ulrich, F. (2003). The global distribution of average volume of alcohol consumption and patterns of drinking. European Addiction Research, 9(4), 147–156. doi:10.1159/000072221
- Riva, M., Curtis, S., & Norman, P. (2011). Residential mobility within England and urban- rural inequalities in mortality. Social Science and Medicine, 73, 1698–1706. doi:10.1016/j.socscimed.2011.09.030
- Rubalcava, L. N., Teruel, G. M., Thomas, D., & Goldman, N. (2008). The healthy migrant Effect: New findings from the Mexican family life survey. American Journal of Public Health, 98(1), 78–84. doi:10.2105/AJPH.2006.098418
- Seedat, M., Van Niekerk, A., Jewkes, R., Suffla, S., & Ratele, K. (2009). Violence and injuries in South Africa: Prioritising an Agenda for prevention. The Lancet, 374(9694), 1011–1022. doi:10.1016/S0140-6736(09)60948-X
- Seggie, J. (2012). Alcohol and South Africa’s youth. South African Medical Journal, 102(7), 587. PubMed: 22748432
- Shafey, O., Eriksen, M., Ross, H., & Mackay, J. (2009). The tobacco atlas 3rd Ed. Atlanta:: American Cancer Society.
- Simetin, I. P., Kuzman, M., Franelic, I. P., Pristas, I., Benjak, T., & Dezeljin, J. D. (2011). Inequalities in croatian pupils’ unhealthy behaviours and health outcomes: Role of school, peers and family affluence. The European Journal of Public Health, 21(1), 122–128. doi:10.1093/eurpub/ckq002
- Sitas, F., Urban, M., Bradshaw, D., Kielkowski, D., Bah, S., & Peto, R. (2004). Tobacco attributable deaths in South Africa. Tobacco Control, 13(4), 396–399. doi:10.1136/tc.2004.007682
- Smith, S. J., & Easterlow, D. (2005). The strange geography of health inequalities. Ransactions of the Institute of British Geographers NS, 30, 173–190. doi:10.1111/j.1475-5661.2005.00159.x
- Statistics South Africa. (2015). Census 2011: Migration dynamics in South Africa. Pretoria: Author.
- Statistics South Africa (StatSA). (2017). South Africa demographic and health survey 2016: Key indicators report. Pretoria: Statistics South Africa.
- van Zyl Smit, R. N., Pai, M., Yew, W. W., Leung, C. C., Zumla, A., Bateman, E. D., & Dheda, K. (2010). Global lung health: The colliding epidemics of tuberculosis, tobacco smoking, HIV and COPD. European Respiratory Journal, 35(1), 27–33. doi:10.1183/09031936.00072909
- Vang, Z., Sigouin, J., Flenon, A., & Gagnon, A. (2015). The healthy immigrant effect in Canada: A systematic review. Population Change and Lifecourse Strategic Knowledge Cluster Discussion Paper Series/Un Réseau stratégique de connaissances Changements de population et parcours de vie Document de travail: Vol. 3: Iss. 1, Article 4, Retrieved from http://ir.lib.uwo.ca/pclc/vol3/iss1/4
- Viner, R. M., Haines, M. M., Head, J. A., Bhui, K., Taylor, S., Stansfeld, S. A., … Booy, R. (2006). Variations in associations of health risk behaviors among ethnic minority early adolescents. Journal of Adolescent Health, 38(1), 55–e15. doi:10.1016/j.jadohealth.2004.09.017
- Wan, X., Shin, S. S., Wang, Q., Fisher Raymond, H., Liu, H., Ding, D., … Novotny, T. E. (2011). Smoking among young rural to urban migrant women in China: A cross-sectional survey. PloS one, 6(8), e23028. doi:10.1371/journal.pone.0023028
- Wild, L. G., Flisher, A. J., Bhana, A., & Lombard, C. (2004). Associations among adolescent risk behaviours and self-esteem in six domains. Journal of Child Psychology and Psychiatry, 45(8), 1454–1467. doi:10.1111/j.1469-7610.2004.00330.x
- World Health Organization. (2014). Global Status Report on Alcohol and Health. Geneva: WHO.
- Yang, T., Wu, J., Rockett, I. R. H., Abdullah, A. S., Beard, J., & Ye, J. (2009). Smoking patterns among Chinese rural–urban migrant workers. Public Health, 123(11), 743–749. doi:10.1016/j.puhe.2009.09.021
- Yang, T., Fuzhong, L., Yang, X., Zhenyi, W., Feng, X., Wang, Y., … Abdullah, A. S. M. (2008). Smoking patterns and sociodemographic factors associated with tobacco use among Chinese rural male residents: A descriptive analysis. BMC Public Health, 8(1), 1. doi:10.1186/1471-2458-8-248
- Zatonski, W. (2007). The east-west health gap in Europe—What are the causes? (editorial). European Journal of Public Health, 17(2), 121. doi:10.1093/eurpub/ckm006
- Zatonski, W., & Jha, P. (2000). The health transformation in eastern Europe after 1990: A second look. Retrieved from http://www.hem.home.pl/index.php?idm=58,59&cmd=1.Warsaw:Cancer Center and Institute of Oncology, 2000