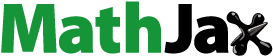
Abstract
Mining of guest’s online reviews is a new trend study that helps managers understand customers’ feeling with services they provide. The satisfaction or dissatisfaction of guest who experienced very important to manager. Hotel managers can be improve the quality of the service through reviews of guest. In this study, the authors present a solution to measure guest satisfaction about Vietnamese hotels through online reviews. Natural language processing technology and machine learning were used to analyze the satisfaction of guest through 06 criteria including: staff, food, room, sevices, location and value. With the data crawled from TripAdvisor, Vietnamese hotel reached up to 72% on average for all criteria and the most satisfactory was the staff reached 77.3%.
PUBLIC INTEREST STATEMENT
This study focus on measuring guest satisfaction from Vietnamese hotel’s online reviews. The guest who had experience with hotel’s services leave reviews on TripAdvisor, booking, hotel.com. Because of a mount of large data, natural language processing techniques are applied to mine big data from TripAdvisor. Sentiment lexicon also developed for this hotel sector to achieve more accuracy of measuring. This sentiment lexicon helps identifying and classification of reviews into positive or negative. The study also offers 06 criteria that are used to measure guest satisfaction. The test results with actual data show that guest satisfaction for these criteria is different, in which, the most satisfied criteria are staff, dissatisfaction criteria for guest is meal. This can be explained when the tastes of each countries are different.
1. Introduction
Vietnam is an attractive destination for many tourists in the world. In Vietnam, tourism industry has become one of the main contributors to the country’s GDP, and Government considered it is a key industry (http://www.vietnamtourism.gov.vn/). The number of tourists coming to Vietnam is increasing rapidly, leading to the hotel industry becoming hotter than ever. In order to easily access and compete with the international market, Vietnam’s hotel industry needs to follow the international standards. Vietnamese hotel managers also need a method to measure satisfaction of guest, and then improve quality of services to attractive more guest from many countries on the world come to Vietnam.
Online hotel business is becoming an indispensable trend of e-commerce when over 80% of guest use booking online via mobile, tablet and website. 80% of travelers looking for hotel on the website and more than 50% of them book room through websites or apps (Blomberg-Nygard & Anderson, Citation2016), (Berne-Manero et al., Citation2018). Online hotel reservations increased more than 10 times in the last decade. When booking a hotel, guest choose and book a hotel on their own, unlike when using a travel agency. The hotel offers a number of criteria according to customer interest to help them search more conveniently. Therefore, the current problem for hotels is how to measure the quality of hotels according to the criteria that guest are interested in Akhtar et al. (Citation2017), Sharma et al. (Citation2019), Boon et al. (Citation2013), Barbosa et al. (Citation2015).
Online reviews are very fast to become an important source of information for both guests and hotels. Consumer-generated content has provided an important new information medium for tourists, throughout the purchasing lifecycle, transforming the way that visitors evaluate, select and share experiences about tourism (Barreda & Bilgihan, Citation2013; Jayathilaka et al., Citation2020; Manes et al., Citation2018). In each review of guest only includes several information about hotel but it can be helpful for traveller who are looking for hotel (Chong et al., Citation2018; Mendes Filho et al., Citation2012). For hotel managers, analyzing online reviews to improve their services is needed (Blomberg-Nygard & Anderson, Citation2016; Tran et al., Citation2019). There are some online booking hotel websites as TripAdvisor, booking or Agoda allow guest to leave comments about their experience with the hotel (Berne-Manero et al., Citation2018). These reviews can impact to decision making of new traveller, especially based on the criteria that guest interested in (Mendes Filho et al., Citation2012). For example, when guest are interested in the hotel location close to the center or the station, some other criteria they often overlook. However, travellers will combine some different criteria to make a final decision.
From the manager’s view point, this information is really useful for promoting their hotel, or can consider providing services better through reviews of guest who have experienced (Barreda & Bilgihan, Citation2013). Understanding customers’ feelings is more important than everthing, therefore, managers often try to know customers’ psychology through many difference ways including questionare, survey, face to face interview. After that, analyze these data to find out which points are satisfied or dissatisfied of guest (Berezina et al., Citation2016). This task can be used excel or simple application for performing. However, for online review data, there are often a lot of reviews for each hotel in booking websites, especially the famous ones, it has several thousands comments per hotel. Many solutions were proposed with the goal of analyzing aspect what guest are interested in (Afzaal et al., Citation2016; Annisa et al., Citation2019) . In Figure below, we describe a commentary about the hotel in Hanoi, assuming that guest are only interested in some aspects such as location, room, restaurant, food, so that, they do not care about other criteria of the hotel.
This study used online reviews of guest about Vietnamese hotels to analyze the hotel experience and its relationship to guest satisfaction. Sentiment analysis method based on data set that crawled from the TripAdvisor and the sentiment weighted lexicon developed by the authors. This sentiment lexicon is built for the hospitality domain to increase accuracy than Bing Liu’s dictionary for all different domain (Hu & Liu, Citation2004; Q. Li et al., Citation2019; Nawangsari et al., Citation2019). The study also performed 02 measurement tasks, including: (1) measuring satisfaction of guest for overall Vietnamese hotels; (2) measuring satisfaction of guest on 06 criteria.
The rest of the paper is structured as follows: section 2 giving an overview of the guest satisfaction and mining guest opinion technique, the methodology will be presented in section 3, section 4 is the result, section 5 is discussion and the final is conclusion.
2. Literature review
2.1. Guest expericence and satisfaction
Guest satisfaction is argued to be the ultimate goal of every hotel business. Another definition, guest satisfaction is “the guest’s evaluation of his/her experience through interaction with various service areas”. Some studies considers satisfaction as an evaluation on which the customers have experienced with the services and customer satisfaction as an emotional response to the use of a product or service (Alrawadieh & Law, Citation2019). From the managerial point of view, evaluating guest satisfaction is the one way to understand guest’s feeling about provided services, and then will impove services better than before (Xiang et al., Citation2015). Hotels employ different tools to assess and address customer satisfactionand behavioral intentions. These tools may include placing comment cards in the guest rooms, employing service recovery techniques to address in- house service failures, distributing postdeparture guest satisfaction surveys, and introducing follow-up measures for those problems that could not be resolved in-house (Berezina et al., Citation2016).
Dong et al. (Citation2014) performed a test to measure satisfaction of guest about Chinese hotels base on factors: hotel, location, service, room, value, food and facility. They used daodao to merge dataset from TripAdvisor 100 hotels in Sanya with 24,051 reviews, with online reviews on individual hotel ranging from 2 to 1744, and averaging in 240 reviews per hotel. Berezina et al. (Citation2016) analyzed online reviews to evaluate guest satisfaction. Dataset of 2,510 hotel guests were collected from TripAdvisor.com for Sarasota, Florida. The text-mining technique was employed to understand what factors may satisfy or dissatisfy hotels customers as restaurant, club, room, furnishing, members, and sports. H. Li et al. (Citation2020) used three factors theory to measure satisfaction. Regression results indicate significant effects of both high and low performance levels of each hotel attribute on customers’ overall satisfaction—independent of hotel star rating.
ICT that has made online tourism is becoming a big revolution, Along the developing of technology and online business, guest satisfaction evaluating now is the trend of age, when technology is the useful tool to collect feedback data and give accuracy analysis results (Guo et al., Citation2017; Zhou et al., Citation2014). Online reviews helps hotel managers knows guest’ psychology who experienced and what they want about services, it also helps promote hotels. However, sometime it impact reputation of the hotel when appear negative word of mouth information (Manes et al., Citation2018). So that, summarized online reviews data and analyzed these data will help managers hold information and make decision for the next business strategy (Boon et al., Citation2013).
2.2. Guest opinion analysis
Guest online reviews for hotel services more and more over time, Travellers who plan to travel are very difficult to find and read them (Q. Li et al., Citation2019; Manes et al., Citation2018; Xiang et al., Citation2015). Many userful tools was built to mine online reviews data according hotel managers requirements (Afzaal et al., Citation2019, Citation2016; Mendes Filho et al., Citation2012). From the seen that, guest opinion and sentiment analysis field is more important than ever (Barbosa et al., Citation2015; Blomberg-Nygard & Anderson, Citation2016). Usually, guests express their views about the hotel through reviews on the website . However, at each sentence level we can also see the guest’s point of view on a certain topic, even deeper at the phrase level, we see the guest’s view on one or several criteria. Therefore, to analyze the guest’s opinion about the hotel, needs to analyze each phrase. Some of the studies in the computer science field and information technology are opinion mining or more specifically, aspect—based opinion (Afzaal et al., Citation2016; Laddha & Mukherjee, Citation2018; Marrese-Taylor et al., Citation2014; Panigrahi & Asha, Citation2018, July; Tran et al., Citation2019).
Aspect based opinion can be divided into two main tasks: Aspect extraction and aspect opinion classification (Annisa et al., Citation2019; Hu & Liu, Citation2004). While aspect extraction tries to find a set of criteria for identifying what aspect of hotel guest are interest in, aspect opinion classification classifies guest’s opinion into positive or negative (Hu & Liu, Citation2004). To mine opinion of guest, using to main approaches including: machine learning and natural language processing.
Machine learning is used to enhance classification accuracy. Machine learning based approaches employ trained data to classifier algorithm so as to learn the differentiating characteristic of the documents and to predict the polarities of the actual documents (Q. Li et al., Citation2019). The most well-known machine learning classification algorithms are Support Vector Machines (SVM), Naïve Bayes, Maximum Entropy and recently is deep learning (Martín et al., Citation2018). An other way of machine learning combined with fuzzy theory that proposed by Muhhamad (Afzaal et al., Citation2016). They presented the fuzzy aspect based opinion classification system using machine learning. The main objectives of their proposal are to extract important aspects from tourist opinions and classify each aspect opinion into positive and negative.
In the past decade, a variety of text mining techniques have been proposed and applied to tourism analysis to develop tourism value analysis models, build tourism recommendation systems, create tourist profiles, and make policies for supervising tourism markets. Q. Li et al. (Citation2019) summarize and discuss different text representation strategies, text-based NLP techniques for topic extraction, text classification, sentiment analysis, and text clustering in the context of tourism text mining, and their applications in tourist profiling, destination image analysis, market demand, etc. An approach text mining to apply in guest’ sentiment analyzing has been proposed by Guo et al. (Citation2017). The big data set includes 266,544 online reviews for 25,670 hotels located in 16 countries collected from the websites. They used latent dirichlet analysis (LDA) to extract meaning from the valuable comments provided by visitors. In the study of Thang Tran (Tran et al., Citation2019) proposed BiLSTM-CRF model to recognize name entity and LDA for topic modeling to identify the topics and their keywords of their hospitality dataset.
In this article, NLP approach used for sentiment analysis of guest who has experienced, dataset downloaded from TripAdvisor combine sentiment lexicon and statistical model to measure guest satisfaction with Vietnamese hotel
3. The methodology
3.1. Problem description
This section introduces definitions. Some of the notations are described in the following Table .
Table 1. Notations list
In the first step of this process, data is crawled from the TripAdvisor. Then, training data and developing sentiment lexicon with scoring. Testing phase is measuring the positives or negatives of reviews. Figure below describes our process.
Definition 1. Set of reviews
Reviews of guests are collected from TripAdvisor via crawling tools. This data set is used as input and analyzed for measuring.Typically, each comment downloaded on the site includes the information: title, reviewer, hotel date, review content. Assuming that each comment is denoted by r, these comments are a set with n reviews as:
Definition 2. Set of criteria
In studies often refer to the aspect (also called criteria or attribute) of the product. The problem of aspect opinion mining requires a given set of aspects. In this study, the concept of criteria and aspect are equivalent. The set of criteria represents the hotel service features guests desire. However, there are many way to representation of a concept in term of linguistic. It means that many terms have equivalent meanings. For example, “value” is the same meaning with “price”, “cost” or “food” are the same meaning with “meal” … . Therefore each criterion is usually represented by a set of equivalent words. Where the term with the most frequent occurrence in the reviews data set is considered a representative term. So, for criteria Aj has a set of term with similar meaning and Aj is the presentative of this criteria: .
Definition 3. Sentiment dictionary
Minqing Hu and Bing Liu in their study “Mining and Summarizing Customer Reviews.” have built a sentiment dictionary of about 6,800 words with positive/negative sentiment (Hu & Liu, Citation2004). Words in the dictionary have been labeled as positive or negative (in Table ).
Definition 4. Sentiment words with scoring
A set of words expressing guest’s setiment about objects and entities. This set of words was extracted from training data and matched with sentiment dictionary that developed by Bing Liu. These words were calculated scoring and shown its polarized value (postive or nagetive)
Table 2. Some sentimen words in sentiment dictionary
3.2. Calculating score of sentiment words
The task of identifying sentiment words is an important task in this article. There are already many sentiment dictionaries built in. However, sentiment dictionaries often provide a high level of accuracy when working in the field that has been trained when building dictionaries. Therefore, we have created our own sentiment dictionary for the hotel sector, and training with data that downloaded from TripAdvisor. The set includes the sentences extracted from the review set
. And
}is the value that each si is assigned as positive or negative.
In this training phase, sentiment words are calculate score by the following steps:
Step 1. Collecting online reviews data from TripAdvisor for training
Step 2. Extracting sentences from set of review . Classify its with label positive or negative
Step 3. Based on sentiment dictionary for extracting sentiment words. These sentiment words is belong both sentiment dictionary and data set.
Step 4. Caculating score of sentiment words that extracted from step 3 as follow:
;
Score of sentiment word wi is
After step 4, we built sentiment dictionary with scoring
Example 1: Extracting words and calculating score:
Given a below reviews:
“We stayed here for two nights in December. Staff are excellent. The club lounge was lovely with a view of the lake and great food. Overall though I would not rate this Intercontinental as 5 star. Despite an upgrade, our room was ‘tired’ as was much of the hotel. Definitely in need if an upgrade .”
Firstly, segment reviews into sentences:
S1 = We stayed here for two nights in December.
S2 = Staff are excellent.
S3 = The club lounge was lovely with a view of the lake and great food.
S4 = Overall though I would not rate this Intercontinental as 5 star.
S5 = Despite an upgrade, our room was “tired” as was much of the hotel.
S6 = Definitely in need if an upgrade.
Labeled sentences with negative sentences set = {s4, s5, s6}, and positive sentences set = {s1, s2, s3}.
Supposed that term “excellent” is extracted from this review is also belong to sentiment dictionary. We have
;
Sentiment score of “excellent”
Term “excellent” is positive words with scoring = 0.17
3.3. Measuring hotel quality by criteria
The set of criteria is reused from several studies (Panigrahi & Asha, Citation2018, July; Xue et al., Citation2017). Each criterion has a set of terms with the same meaning, therefore, each criterion has a representation and each representative may have seveal equivalent terms as the concept presented in the problem definiton.
Calculating polarization for criteria does not only depend on sentiment word stand in front or rear. The problem of extracting criteria becomes difficult when one sentence can contain many different criteria. The example in Figure of this article has the phrase “We found the food excellent and staff very professional.” There are two criteria mentioned are “food” and “staff”. With the criterion of “food”, excellent is sentiment word describing the views of guest positively, or as the criteria “staff”, “professional” is the opinion of guests. So that, we suggest to the following steps to identify and measure criteria:
Step 1. Based on the set of criteria in Table , extracting the criteria from online reviews data to develop a set of criteria.
Step 2. Each sentence has been separated from the review, then identify criteria. Sometimes, there are several sentences that represent the same criteria. Therefore, the value of each criterion is called = average(
where Aj is the representative of the criterion and
is the same term as Aj’ s representative criterion.
with
.
Step 3. Consider increasing or decreasing sentiment values with some words like: very, quite, extremly, great, not and so on when it appear in the sentences.
Table 3. Number of Vietnam hotel rated 4 to 5 star in 5 cities
4. Results
4.1. Dataset
In order to perform this test, we collected online review of guests from TripAdvisor for Vietnam hotels. Firstly, we filtered out the list of Vietnamese hotels that managed by the Vietnam National Administration of Tourism through the website http://vietnamhotel.org.vn to get a list of 3–5 star hotels. In the next step, we use crawling tools to collect data with these hotels. We save the data including the information: name of reviewer, title of review, content of review and date of stayed.
For Vietnam hotels rated 4 to 5 stars, we only take 5 cities including: Hanoi, Ho Chi Minh, Da Nang, Nha Trang, these hotels have been appeared in TripAdvisor as in Table below:
The number of reviews for each hotel is in the range of 1000–2000 reviews, for example for Hanoi melia hotel at the time of data collection it was 1782 reviews. The total number of reviews of all hotels after adjustment is 53,237 reviews. We use about 30% of these reviews contain 15,900 reviews to train and extract 103,823 sentences. However, after removing and cleaning the sentences, we only keep 100,000 sentences for training. Used RapidMiner (in figure ) for sentimen sentences classification, the total number of positive sentences is 62,710 and the total number of negative sentences is 37,290 sentences.
In the sentiment dictionary of M. Hu and Liu (Hu & Liu, Citation2004), there are 68,000 sentiment words, in another built with more than 70,000 sentiment words. However, in our dataset, we extracted only 120 words from sentiment words because we have removed some words with low-score value, the set of sentiment words we built mainly consists of adjectives and adverbs. The number of adjectives is 78 words, the number of adverbs is 42 words. This is more convenient in identifying and separating words, making the system run faster and more stable. The table listed some sentiment words from our sentiment lexicon.
Table 4. List of some sentiment words with scoring
The set of criteria for measuring hotel quality was reuse from previous studies (Alrawadieh & Law, Citation2019; Dong et al., Citation2014; Godnov & Redek, Citation2018; Panigrahi & Asha, Citation2018, July; Sharma et al., Citation2019; Xue et al., Citation2017). These studies demonstrate that the criteria in the table below are often of interest to guest in online reviews. These criteria include: location, room, services, staff, meal and value. These are terms that represent criteria. Details in the table below
Table 5. Criteria for measuring hotel quality
4.2. Results
4.2.1. Measuring guest satisfaction in reviews
Tested on dataset of 36,200 reviews separated from the downloaded 53,237 reviews, the comments were determined related with “satisfaction-dissatisfaction” variables and evaluated with the content analysis. In this study, we evaluate guest satisfaction—disatisfied in the 06 criteria given above for each review. To evaluate this, we draw out the sentiment words in each review and sum their scores. If the total value is> 0, guest is satisfied and vice versa.
For example Given a review below:
We had a very nice relaxing stay at Interconti Westlake Hotel. Very friendly and efficient service. A huge compliment to the lounge staff, we felt so wormly welcome. Breakfast & happy hour always a pleasure. The capuccino in the lounge is a MUST.
The extracted sentiment words include: nice, friendly, efficient, huge, wormly, pleasure. Value of the whole sentence = 2.3216533. Therefore this review is rated positive, and we judge that guest is satisfied with the hotel.
Among the sentences tested, the results for Vietnamese hotels in general were 72.7% satisfied and 27.3% were dissatisfied (Figure ), number of satisfaction reviews is 26,210 and 9,900 reviews is dissatisfaction (Table ).
Table 6. Measuring guest satisfaction in reviews
4.2.2. Measuring guest’ satisfaction by criteria
In the next, we evaluate the satisfaction—dissatisfaction of guest according to the criteria with a test set of 36,200 reviews. We had to combine sentiment word and criteria for evaluating. First, the criteria are extracted from the reviews, then looking for sentiment words that describe the criteria. For the sentiment words, we calculate automatically for left and right of the criteria to find 3 posistions. Finally, calculate the polarity for each criterion.
For example: Given review below:
We had a wonderful time here at hotel intercontinental west lake. The room was clean and the view from our balcony was pretty. Location was not that good as it’s a bit far from places of interest. Restaurant in the hotel serves good food and great service especially in MILAN, the pizza was to die for!!. The hotel lacks of activities. There’s nothing much to do rather than looking at the beautiful views from the sunset bar and the hotel balcony. That’s the only down side of the hotel. The rest was awesomely good. We will definitely come back again to this hotel.
The extracted criteria include: room, balcony, location, places, restaurant, food, service, bar
Sentiment words are extracted with the criteria and calculated from the left of the word or the right of the 3 position including: (Rom, clean); (Balconly, pretty); (location, not, good); (places, bit far, interest); (restaurant, good); (food, good); (bar, beautiful).
Based on the reviews above we see that the criteria location and places are dissatisfied.
To measure satisfaction—dissatisfaction of guest, we use the following formula:
For measuring sastifaction—dissatisfaction of guest, we used following equation:
Location and Room are the most interested criteria in 36,077 reviews. Some reviews do not mention criteria “value”, which are mostly found in negative reviews. Table is measuring of guest satisfaction and dissatisfaction with 06 criteria include: Location, Room, Services, Staff., Rate satisfaction of these criteria is illustrated in Figure .
Table 7. Measuring satisfaction—dissatisfaction of guest for Vietnamese hotel
5. Dicussion
Experience management and measuring customer satisfaction about hotels has a long history (H. Li et al., Citation2020; Neirotti et al., Citation2016). When analyze guests “ sentiment, we can draw different paintings of emotions status or understand guests” psychology more. Experience management is aimed to gain maximum benefit from limited resources. If we loss of guest satisfaction can lead to very serious consequences. Despite the cost savings and benefits in short-term, but the number of customers lost because of dissatisfaction can impact to the reputation of hotel. Many hotels encounter a mistake when trying to create an experience based on subjective thinking instead of actually collecting customer feedback and integrating those feedback into products or services improving.
Traditional methods are often based on manual collection such as questionnaires, or interviews guest who have used the service, from that given out hypothesis and assessments . However, with the trend of online business, it is difficult to collect questionnaires or interview guest directly. Following this trend, many online evaluation systems or online data collection have been developed (Berezina et al., Citation2016; Xiang et al., Citation2015), and the mining of online reviews becomes popular (Neirotti et al., Citation2016; Xu, Citation2018). Data collection and big data analysis allows for a more general overview than old method, it becomes more flexible, mining many aspects rather than framing in hypotheses or designed questions. So that, data analysis bring out more latent factors, which managers have never thought of that.
Online reviews analysis provide an overall view by the large size of sample, easy to apply new technologies and mathematical model to bring results with high accuracy. Opinion mining in many aspects is not limited by given questions, built-in scenarios or hypotheses. However, the disadvantage of this method is a lot of noise when analyzing, so before doing it, it is necessary to clean and remove noise from data. From analyzing guest experience to evaluate guest satisfaction about the hotel, and the impact of satisfaction to guest loyalty (Jayathilaka et al., Citation2020)
Due to the advantages of online reviews analysis, there are more and more studies on this field (Elnagar et al., Citation2018; Jayathilaka et al., Citation2020; H. Li et al., Citation2020) the results are shown more clearly for the overall or detailed on each aspect. To consider with Vietnamese hotels, there are now many hotels looking to promote on the online booking website, with the goal of finding ways to attract customers. When evaluating overall hotel in Vietnam, the number of positive reviews was 72.7% showing that 2/3 of the guest were satisfied with the hotels. In order to go into detail, this research has separated 06 different criteria including: location, room, services, staff, meal and value, and then, assess guest satisfaction earch each criterion. The selecting these criteria for evaluation is based on the number of mentional in dataset and previous studies. In which the highest satisfaction rate for staff is up to 77%. Meal is the lowest evaluating criterion, it can also be considered because the taste of each country is different. Room and location criteria are the two criteria that are mentioned a lot in the comments, showing the great importance in the choice of guest hotels.
6. Conclusion
Researching and applying Customer Experience Management will help to connect more with guest through regular understanding and interaction. The positive of experiences makes guest believe and ready to promoter products and services. Not only hotels have more opportunity to attract more guests, satisfied customers tend to be more loyal.
In this study, we performed two measurement tasks: Measure the satisfy the overall quality of Vietnam hotel and measure each hotel criteria. The results showed that, on the data set of Vietnamese hotels downloaded from TripAdvisor, the percentage of customers who were satisfied with the service was higher than the dissatisfied. Particularly for “price” criteria, it is only mentioned in negative reviews, so the rate of “price” dissatisfaction is higher than the satisfaction rate. In the next study, in order to help Vietnam hotels be able to accurately price when participating in the online platform, we will focus on analyzing comments and price forecasts for Vietnamese hotels on TripAdvisor.
correction
This article was originally published with errors, which have now been corrected in the online version. Please see Correction (http://dx.doi.org/10.1080/23311886.2020.1812955)
Additional information
Funding
Notes on contributors
Ha Nguyen Thi Thu
Ha Nguyen Thi Thu is a lecturer at e-commerce department, Vietnam Electric Power University, she is interested in customer behavior management on social networks, especially using mathematical models and machine learning to analyze big data for customer experience management problems.
References
- Afzaal, M., Usman, M., Fong, A. C. M., & Fong, S. (2019). Multiaspect-based opinion classification model for tourist reviews. Expert Systems, 36(2), 1–14. https://doi.org/10.1111/exsy.12371
- Afzaal, M., Usman, M., Fong, A. C. M., Fong, S., & Zhuang, Y. (2016). Fuzzy aspect based opinion classification system for mining tourist reviews. Advances in Fuzzy Systems, 2016, 1–14. https://doi.org/10.1155/2016/6965725
- Akhtar, N., Zubair, N., Kumar, A., & Ahmad, T. (2017). Aspect based sentiment oriented summarization of hotel reviews. Procedia Computer Science, 115, 563–571. https://doi.org/10.1016/j.procs.2017.09.115
- Alrawadieh, Z., & Law, R. (2019). Determinants of hotel guests’ satisfaction from the perspective of online hotel reviewers. International Journal of Culture, Tourism, and Hospitality Research, 13(1), 84–97. https://doi.org/10.1108/IJCTHR-08-2018-0104
- Annisa, R., Surjandari, I., & Zulkarnain. (2019). Opinion Mining on Mandalika Hotel Reviews Using Latent Dirichlet Allocation. Procedia Computer Science, 161, 739–746. doi: 10.1016/j.procs.2019.11.178
- Barbosa, R. R. L., Sánchez-Alonso, S., & Sicilia-Urban, M. A. (2015). Evaluating hotels rating prediction based on sentiment analysis services. Aslib Journal of Information Management, 67(4), 392–407. https://doi.org/10.1108/AJIM-01-2015-0004
- Barreda, A., & Bilgihan, A. (2013). An analysis of user-generated content for hotel experiences. Journal of Hospitality and Tourism Technology, 4(3), 263–280. https://doi.org/10.1108/JHTT-01-2013-0001
- Berezina, K., Bilgihan, A., Cobanoglu, C., & Okumus, F. (2016). Understanding satisfied and dissatisfied hotel customers: Text mining of online hotel reviews. Journal of Hospitality Marketing and Management, 25(1), 1–24. https://doi.org/10.1080/19368623.2015.983631
- Berne-Manero, C., Gómez-Campillo, M., Marzo-Navarro, M., & Pedraja-Iglesias, M. (2018). Reviewing the online tourism value chain. Administrative Sciences, 8(3), 48. https://doi.org/10.3390/admsci8030048
- Blomberg-Nygard, A., & Anderson, C. K. (2016). United nations world tourism organization study on online guest reviews and hotel classification systems: An integrated approach. Service Science, 8(2), 139–151. https://doi.org/10.1287/serv.2016.0139
- Boon, E., Bonera, M., & Bigi, A. (2013). Measuring Hotel Service Quality from Online Consumer Reviews: A Proposed Method. Information and Communication Technologies in Tourism 2014, 367–379. doi: 10.1007/978-3-319-03973-2_27
- Chong, A. Y. L., Khong, K. W., Ma, T., McCabe, S., & Wang, Y. (2018). Analyzing key influences of tourists’ acceptance of online reviews in travel decisions. Internet Research, 28(3), 564–586. https://doi.org/10.1108/IntR-05-2017-0212
- Dong, J., Li, H., & Zhang, X. (2014). Classification of customer satisfaction attributes: An application of online hotel review analysis. IFIP Advances in Information and Communication Technology, 445(2008), 238–250. https://doi.org/10.1007/978-3-662-45526-5_23
- Elnagar, A., Khalifa, Y. S., & Einea, A. (2018). Hotel arabic-reviews dataset construction for sentiment analysis applications. Studies in Computational Intelligence, 740, 35–52. https://doi.org/10.1007/978-3-319-67056-0_3
- Godnov, U., & Redek, T. (2018). Good food, clean rooms and friendly staff: Implications of user-generated content for slovenian skiing, sea and spa hotels’ management. Management (Croatia), 23(1), 29–57. https://doi.org/10.30924/mjcmi/2018.23.1.29
- Guo, Y., Barnes, S. J., & Jia, Q. (2017). Mining meaning from online ratings and reviews: Tourist satisfaction analysis using latent dirichlet allocation. Tourism Management, 59, 467–483. https://doi.org/10.1016/j.tourman.2016.09.009
- Hu, M., & Liu, B. (2004). Mining opinion features in customer reviews. In AAAI (Vol. 4, No. 4, pp. 755-760).
- Jayathilaka, R., Dharmasena, T., Rezahi, N., & Haththotuwegama, S. (2020). The impact of online reviews on inbound travellers’ decision making. Quality and Quantity, 54(3), 1005–1021. https://doi.org/10.1007/s11135-020-00971-1
- Laddha, A., & Mukherjee, A. (2018). Aspect opinion expression and rating prediction via LDA-CRF hybrid. Natural Language Engineering, 24(4), 611–639. https://doi.org/10.1017/S135132491800013X
- Li, H., Liu, Y., Tan, C. W., & Hu, F. (2020). Comprehending customer satisfaction with hotels: Data analysis of consumer-generated reviews. International Journal of Contemporary Hospitality Management, 32(5), 1713–1735. https://doi.org/10.1108/IJCHM-06-2019-0581
- Li, Q., Li, S., Zhang, S., Hu, J., & Hu, J. (2019). A Review of Text Corpus-Based Tourism Big Data Mining. Applied Sciences, 9(16), 3300. doi:10.3390/app9163300
- Liu, B. (2015). Sentiment analysis: Mining opinions, sentiments, and emotions. Sentiment Analysis: Mining Opinions, Sentiments, and Emotions, May, 1–367. https://doi.org/10.1017/CBO9781139084789
- Manes, E., Tchetchik, A., Ettinger, A., Grabner-Kräuter, S., & Terlutter, R. (2018). The role of electronic word of mouth in reducing information asymmetry: An empirical investigation of online hotel booking. Journal of Business Research, 68(September2017), 185–196. https://doi.org/10.1016/j.ijhm.2017.09.002
- Marrese-Taylor, E., Velásquez, J. D., & Bravo-Marquez, F. (2014). A novel deterministic approach for aspect-based opinion mining in tourism products reviews. Expert Systems with Applications, 41(17), 7764–7775. https://doi.org/10.1016/j.eswa.2014.05.045
- Martín, C. A., Torres, J. M., Aguilar, R. M., & Diaz, S. (2018 (2018 2018). Using Deep Learning to Predict Sentiments: Case Study in Tourism. Complexity, 2018, 1–9. doi:10.1155/2018/7408431
- Mendes Filho, L. A. M., Tan, F. B., & Mills, A. (2012). User - generated content and travel planning: An application of the theory of planned behavior. Revista Brasileira De Pesquisa Em Turismo. São Paulo, 6(3), 280–289. https://doi.org/10.7784/rbtur.v6i3.543
- Nawangsari, R. P., Kusumaningrum, R., & Wibowo, A. (2019). Word2Vec for Indonesian Sentiment Analysis towards Hotel Reviews: An Evaluation Study. Procedia Computer Science, 157, 360–366. doi:10.1016/j.procs.2019.08.178
- Neirotti, P., Raguseo, E., & Paolucci, E. (2016). Are customers’ reviews creating value in the hospitality industry? Exploring the moderating effects of market positioning. International Journal of Information Management, 36(6partA), 1133–1143. https://doi.org/10.1016/j.ijinfomgt.2016.02.010
- Panigrahi, N., & Asha, T. (2018, July). Aspect-Level Sentiment Analysis on Hotel Reviews. In Computational Intelligence in Data Mining: Proceedings of the International Conference on CIDM 2017 (Vol. 711, p. 379). Springer.
- Sharma, H., Tandon, A., Kapur, P. K., & Aggarwal, A. G. (2019). Ranking hotels using aspect ratings-based sentiment classification and interval-valued neutrosophic TOPSIS. International Journal of Systems Assurance Engineering and Management, 10(5), 973–983. https://doi.org/10.1007/s13198-019-00827-4
- Tran, T., Ba, H., & Huynh, V. N. (2019). Measuring hotel review sentiment: An aspect-based sentiment analysis approach. Lecture Notes in Computer Science (Including Subseries Lecture Notes in Artificial Intelligence and Lecture Notes in Bioinformatics), 11471(LNAI), 393–405. https://doi.org/10.1007/978-3-030-14815-7_33
- Xiang, Z., Schwartz, Z., Gerdes, J. H., & Uysal, M. (2015). What can big data and text analytics tell us about hotel guest experience and satisfaction? International Journal of Hospitality Management, 44, 120–130. https://doi.org/10.1016/j.ijhm.2014.10.013
- Xu, X. (2018). Does traveler satisfaction differ in various travel group compositions? Evidence from online reviews. International Journal of Contemporary Hospitality Management, 30(3), 1663–1685. https://doi.org/10.1108/IJCHM-03-2017-0171
- Xue, W., Li, T., & Rishe, N. (2017). Aspect identification and ratings inference for hotel reviews. World Wide Web, 20(1), 23–37. https://doi.org/10.1007/s11280-016-0398-9
- Zhou, L., Ye, S., Pearce, P. L., & Wu, M. Y. (2014). Refreshing hotel satisfaction studies by reconfiguring customer review data. International Journal of Hospitality Management, 38, 1–10. https://doi.org/10.1016/j.ijhm.2013.12.004