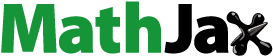
Abstract
Collective action through farmer groups has been seen to be significantly beneficial to especially smallholder farmers in sub-Saharan Africa (SSA), in terms of competitiveness, credit access, capacity building, and market access. Thus, it has been promoted as a strategy for rural development. Despite the many benefits of collective action, there is very little to no empirical evidence of its impact on the farm efficiency of rural women. This paper sought to measure the impact of collective action through farmer-based organizations (FBOs) on the technical efficiency (TE) of smallholder farmers using the stochastic frontier model and the inverse probability weighting estimator (IPW). Using representative data from 300 randomly selected smallholder cassava farmers in the Eastern region of Ghana we found that the age of the household head, years of experience in cultivation, size of the household, and the geographical location of the farm, independently have a significant influence on farmers’ decision to join FBOs. The results show that female farmers who belong to FBOs, on average, can produce about 63.5% of their potential yield while male members achieve only 57.8% of their potential yield, on average. The results further reveal that female farmers benefit the most from FBOs with their membership resulting in a 12% increase in their TE. The findings provide implications for the design of policies to improve women’s farm efficiency.
1. Introduction
Smallholder farmers are responsible for cultivating about 75 percent of the world’s agricultural land and often work in isolation (Lowder et al., Citation2016). In Ghana, the agriculture sector is largely dominated by smallholder farmers producing crops including cocoa, rice, maize, and cassava. To mitigate the challenges that come with working in isolation − and to increase profitability and productivity − these smallholders form groups. Collective action via farmer groups or, more formally, farmer-based organizations (FBOs) is deemed to be a potential remedy to market failure and high transaction costs in most developing countries (Fischer & Qaim, Citation2012). This is because when smallholder resource-poor agricultural producers work together it becomes easier to access farming inputs such as seeds, fertilizers, and agrochemicals. It also affords them better access to larger markets through reduced costs and improved bargaining power (Kersting & Wollni, Citation2012).
FBOs also serve as a medium for public extension agents to reach out to larger numbers of farmers. Nonetheless, in many developing countries, there is a shortage of agricultural extension agents. For instance, in Ghana, the ratio of extension workers to farmers is 1:2,500 farmers (Owusu-Baah, Citation2012). This number is far too many for a single agent to effectively reach. In that regard, FBOs are often seen as an effective approach to ensuring access to extension services and enhancing agricultural productivity and food security (Hussein, Citation2001; Salifu et al., Citation2012). The government of Ghana in an attempt to facilitate growth in the agriculture sector has implemented several programs including the Planting for Food and Jobs program, the Modernizing Agriculture in Ghana Program, and the Ghana Agriculture Sector Investment program (Ministry of Food and Agriculture MoFA, Citation2017).
However, Njobe (Citation2015) explain that such efforts are often limited by the already existing gender disparities in terms of participation and access. Quisumbing et al. (Citation2015) also note that most agricultural interventions are immersed in social contexts that define the work men and women do and the groups they are allowed to join, and how resources are shared. In the rural parts of most countries in SSA, the right to control, use and even own assets are highly gendered, which to a large extent reflects the gender norms that limit women’s ability to invest in relatively more profitable agricultural technologies. In many cases, farmer groups have successfully contributed to improving the social status and livelihood of women (Elbehri & Lee, Citation2011). Therefore, although FBOs are beneficial to smallholders across the sub-region (Abebaw & Haile, Citation2013; Bizikova et al., Citation2020; Verhofstadt & Maertens, Citation2015; Wossen et al., Citation2017), more pertinent questions remain unanswered. Particularly, questions regarding the effect such organizations have on the farm efficiency of rural women. Considering that women smallholder farmers make up about 50% of the agricultural labour force in Africa (Raney et al., Citation2011), and have the potential to contribute just as much as men do (Missiame et al., Citation2021), it is imperative to understand how these organizations affect their agricultural production if the wide gap between men and women is to be closed. Against this backdrop, this present study sought to answer the following question: what is the impact of farmer organizations on the technical efficiency of the farmers? Answering this question is crucial to efforts at enhancing the productivity of rural farmers, particularly women farmers, who depend solely on farming for livelihood. This study contributes to the literature on gender and agriculture in SSA and also provides a way forward for policymakers in formulating policies tailored to promoting equity through FBOs and ensuring equal access to information, credit, and other inputs.
The rest of the paper is organized as follows. Section 2 presents a brief review of the FBO and farm efficiency literature. Section 3 describes the data and the methods of analysis. The results from the estimation are presented in Section 4, followed by a discussion of the results and conclusions in Section 5. Policy recommendations are also provided in Section 5.
2. Literature review
Collective action literature reveals that a myriad of factors influences the success of collective initiatives. The factors include the size of the group, shared norms, leadership and governance structure, boundary definitions, and interdependence among the members (Shiferaw et al., Citation2009; Tefera et al., Citation2017). Along gender lines, there continue to exist disparities in group participation among male and female farmers. Studies have found that women’s participation in cooperatives is lower compared to that of men. For instance, a report by Majurin (Citation2012) on three East African countries revealed that only about 30 to 42% of cooperative members across the three countries are women. A recent study by Abdu et al. (Citation2022) reveals that the majority of male and female members in FBOs are married. The fact that most members of FBO are married could be because marriage promotes participation in social and economic activities, especially for rural women in Africa (Mwatsi et al., Citation2020). Meier zu Selhausen (Citation2016) also observed that control over agricultural land is a major factor in women’s participation in collective action in Uganda. Using the probit model, Ingutia and Sumelius (Citation2022) found that in Kenya, whereas access to credit encouraged women’s participation in farmer groups, their limited decision-making power and their inadequate access to land reduced their propensity of group participation
Besides studies on what influences FBO membership, other studies have examined the impact of membership in farmer organizations on smallholder farmers. More specifically, Chagwiza et al. (Citation2016) assessed the impact of membership on the commercialization, prices, and income, among other indicators, of dairy producers in Ethiopia and observed a tradeoff between technological transformations and better prices. Wossen et al. (Citation2017) in Nigeria examined the impact of cooperative membership on asset ownership, technology adoption, and poverty. They found that smallholders who have access to credit benefit more from cooperatives in terms of technology adoption. In another study, Verhofstadt and Maertens (Citation2015) measured the impact of cooperative membership on several agricultural performance indicators including farm revenue and income of smallholder farmers in Rwanda. The study observed a positive impact on the said farm performance but attributed the impact to the structure, and how the organization operates in terms of production activities and member remunerations.
The studies that measure the impacts of farmer organizations on the productivity of the members are scanty and with mixed findings. For instance, Abebaw and Haile (Citation2013) and Abate et al. (Citation2014), respectively, observe that membership in cooperatives heterogeneously impacts fertilizer adoption and the technical efficiency of farm households in Ethiopia. In China, Ma and Abdulai (Citation2016), employ the endogenous switching regression and observe that overall, membership in cooperatives positively impacts apple yield. They also observe that the impact on smallholder farms is much greater compared to medium and large-scale farms. Abdul-Rahaman and Abdulai (Citation2018) also attempt to measure the impact of farmer groups on farm yield and efficiency in Ghana. Using a sample selection stochastic production frontier on a sample of 412 smallholder farmers, they also observe that farmer groups positively impact yield and efficiency. Although the studies cited above all provide some insight into the impacts of farmer organizations on smallholder farmers, they fail to explicitly show whether or not these farmer groups impact male-headed and female-headed smallholder farm households differently, in terms of their production efficiency. This presents a gap in the literature that requires closing if effective policies are to be made.
This current study builds on previous works on collective action by disaggregating its impact on the production efficiency of male- and female-headed households in Ghana. This is to gain insight into the unique challenges faced by female-headed households and male-headed households to help tailor interventions and support systems to address specific needs and improve overall farm efficiency. Disaggregation of the impact of FBOs on farm efficiency can also help better understand the decision-making dynamics within the households. Such insights can also aid in identifying opportunities to empower women within the farming systems, promote their active participation in decision-making processes, and help improve overall farm efficiency by leveraging their knowledge and skills.
3. Data and methods
3.1. Data
The data used in this study was obtained from a 2019 survey of smallholder cassava farmers in the Eastern region of Ghana. A multistage sampling technique was employed in obtaining the sample for the study. In the first stage, five cassava-producing villages in the Fanteakwa District were purposively selected. The selection was based on the fact that the communities were the leading cassava farming communities in the district, according to information from the district agricultural office. The second and final stage was a simple random sampling of smallholder cassava farmers from each of the selected villages. The required sample size was calculated using the Yamane (Citation1967) formula specified:
where is the sample size,
represents the population size whiles
is the degree of precision.
The population size of the district at the time of the field exercise, according to the 2010 Population and Health Census was 121879. The required sample size for the study was, therefore, calculated as
Thus, the sample size for the study was 399 respondents. However, due to time and financial constraints, the study settled for only 300 respondents. The list of smallholder cassava farmers was obtained for each of the five cassava-producing villages to form the sampling frame. Random numbers were then assigned to each farmer on the list and the first 60 farmers were selected. If a farmer was unavailable for the study, the farmer was replaced by the next available farmer. This was done for each of the five villages. Pre-tested semi-structured questionnaires were then used to collect data on farmers’ socio-economic and farm-specific characteristics such as membership in FBOs, gender, age, education, farm size, household size, extension access, output, and input quantities, experience in cassava cultivation, and income from non-farm economic activities,
3.2. Estimation strategy
We attempt to measure the impact of collective action (as observed through farmers’ membership in FBOs) on the farm efficiency of members. To do so, we first estimate the technical efficiency of the farmers using the stochastic frontier model. This is done separately for the men and women farmers in the sample. We then measure the impact of FBO membership using the inverse probability weighting estimator (IPW).
3.2.1. The stochastic frontier model
In the model, the relationship between output and input is expressed as:
where is the scalar output of the
farmer;
is a vector of inputs used by the
farmer;
is a vector of parameters to be estimated. The term
is the error term and is composed of two components
and
. The
is the random error. It captures the factors that are outside the farmers’ control but affects the output. The other component (
is the inefficiency component and captures farmers’ managerial inefficiencies. The stochastic frontier model is, therefore, specified as:
The term is assumed to be independently and identically distributed from
(Jondrow et al., Citation1982). The term
in Equationequation (2)
(2)
(2) represents the functional form of the production technology. The functional form could be the Cobb-Douglas production function or the translog production function. In this paper, we assume the translog production function following the results of the generalized likelihood ratio test (Appendix 1). The function is generally specified as (Kymn & Hisnanick, Citation2001):
where Y is the real output, is the
factor of production and
is the
factor of production.
An advantage of the translog production function over the Cobb-Douglas functional form is that there are no a priori restrictions on the substitutability between inputs (Kymn & Hisnanick, Citation2001). We also consider two inputs namely labour (lnLabour) and cassava stem cuttings (lnSeed) for production. Accordingly, the technical efficiency (TE) of farmer is expressed as:
The TE scores obtained from Equationequation (4)(4)
(4) range between 0 and 1 with 0 implying the producer only produces 0% of its potential output given the inputs. A TE score of 1 suggests that the producer is very efficient and can achieve the potential yield given the inputs. We estimate the model separately for male-headed and female-headed smallholder farmers in our sample, regardless of their membership status in any FBO in or around the study area. We employ the sfcross routine in STATA 16 to implement Equationequation (2)
(2)
(2) and also to estimate Equationequation (4)
(4)
(4) . We assumed a truncated normal distribution for the errors in Equationequation (2)
(2)
(2) . This assumption allowed us to incorporate farmer-specific inefficiency factors in estimating the production function. However, the results for the inefficiency components are omitted to make space for discussions of other elements.
3.2.2. The inverse probability weighting estimator
In impact assessment studies with cross-sectional data, researchers often employ the propensity score matching technique to estimate the average treatment effect of the program or intervention (Abebaw & Haile, Citation2013; Mango et al., Citation2017). The propensity score measures the likelihood of exposure to treatment, given some observed covariates (Rosenbaum & Rubin, Citation1985). Matching by propensity score allows one to simultaneously control for many observed covariates by matching the treatment and the control groups based on the individual propensity scores (D’Agostino, Citation1998; Rosenbaum & Rubin, Citation1985). However, one limitation of the propensity scores matching technique in estimating the impact of a program or intervention is that the matching algorithms often omit a significant chunk of the population when the comparison group is being created. This limits the ability to generalize the results (Rosenbaum & Rubin, Citation1985).
We employed the inverse probability weighting estimator (IPW) (Cassel et al., Citation1983) to control for confounding. The IPW is a consistent and unbiased estimation technique for treatment effects in the presence of confounding outcomes due to selection bias (Curtis et al., Citation2007). One advantage of the IPW is that it is doubly robust, meaning that even in the presence of model misspecifications, the estimator is still consistent (Funk et al., Citation2011; Wooldridge, Citation2007). Another merit of the IPW is its ability to adjust for disproportional sample surveys and correct for the bias that results from missing data (Seaman & White, Citation2013; Wooldridge, Citation2007). As the name implies, IPW involves weighting the outcomes through the inverse of the probability of the individuals being assigned to treatment, given a set of covariates.
Let denote the outcome (TE) farmer
would obtain under treatment (i.e., membership of FBO), and
, the outcome farmer
would obtain under control (non-membership of FBO). Under the assumption that the data is independently and identically distributed across i, but is potentially heteroscedastic if we denote treatment by a binary variable
, then we can only observe (Busso et al., Citation2014):
The propensity score, which is the conditional probability of treatment, can then be denoted by
We estimate the effect of participation in FBO on the TE of farmer by (Handouyahia et al., Citation2013):
where and
are the number of members and non-members, respectively. The weight
is scaled up to add to 1 and is specified as (Busso et al., Citation2014):
This model was estimated using the teffects routine also available in STATA version 16. The IPW model has many empirical applications and has been employed in studies including Rahman et al. (Citation2018), Adolwa et al. (Citation2019), and Zheng and Ma (Citation2023). The variables considered in this study, their measurements, and a priori expectations are presented in Table .
Table 1. Definition and measurement of variables
Empowerment of women has a greater implication for gender equality as it promotes women’s rights and leadership and challenges stereotypes. Therefore, improving the farm efficiency of women provides opportunities for empowerment and advancement in a traditionally male-dominated sector.
4. Results and discussion
4.1. Descriptive statistics
Out of the 300 respondents who were interviewed for the study, 217 were males representing about 72% of the sample. The remaining 83 (28%) were females. Out of the 83 females, only about 25% belong to FBOs. The remaining 75% are non-members. Among the men, however, only about 34% of them are members of an FBO. Among the male sample, the majority (about 45%) have attained basic formal education. Similarly, among the female sample, the majority (49%) have attained some basic education, none of the females, however, has any tertiary education. The data also shows that more men, approximately 78%, are married compared to about 33% of women who are married.
Land is a significant resource for household agricultural production. Therefore, the nature of its ownership has a significant impact on household decision-making and, hence, production (Vu et al., Citation2021). We identified four main tenure systems in the study area, namely stool land, family land, leasehold or renting, and sharecropping. Among the male sample, the majority (36%) were practicing sharecropping. In the female sample, however, the majority (32.53%) were cultivating family lands. The last 6% of the female sample were cultivating stool lands or producing under other land tenure systems.
The data also shows that about 78% of male farmers have their farmlands located outside the administrative capital of the district, compared to about 84% of the women farmers whose farmlands are outside the district capital. The greater percentage of men (approximately 44%) had access to credit from rural banks relative to about 36% of women who accessed credit from the same kind of financial institution. On average, male farmers are older (44 years of age) and have more farming experience (11 years) relative to an average age of 42 years among women farmers and 9 years of farming experience. However, in terms of the average annual income from non-farm economic activities, women farmers earn more (approximately $121), compared to approximately $55. Also, on average, women farmers live closer to market centers (about 2.784 kilometers) while men farmers live about 5.891 kilometers away from market centers, on average. In terms of household size, both men and women farmers have household sizes of about five members, on average. These statistics are presented in Table .
Table 2. Descriptive Statistics
4.2. Technical efficiency of smallholder farmers
The results from the stochastic frontier model are presented in Table . Models 1, 2, and 3 represent results for the pooled sample (both men and women cassava farmers), women-only, and men-only samples, respectively. Under each sample, we also present the estimation results for the full sample, regardless of membership status in FBOs (pooled). We also present results for members only, and then for non-members only. At the bottom of the table is the estimated TE for each group. The inputs considered in this study are cassava stem cuttings (lnSeed) and labour (lnLabour).
Table 3. Stochastic frontier estimation of TE
In model 1 (pooled sample), labour and stems contribute significantly to total cassava yield, on average. Specifically, a 1 percent increase in the quantity of labour leads to about a 0.28% increase in yield. Similarly, a 1 percent increase in the quantity of stem cuttings results in about a 0.38% increment in yield. This result is consistent with Karani et al. (Citation2015), who also found that seed and labour significantly affect passion fruit yield in Kenya. A possible case of over-utilization of stem cuttings could be observed among the members-only sub-sample in that the coefficient of lnSeedsq is negative. The implication is that any further increase beyond the current level will cause the marginal product (MPP) of stem cuttings to fall by 0.16% for every unit increment in the number of stem cuttings. Due to limited agricultural land, most farmers in an attempt to achieve the most out of their land, end up over-populating the same piece of land. Empirical studies have found that plant density has a significant impact on cassava yield (see Onasanya et al., Citation2021).
Focusing on the women farmers only, the results show a negative yield elasticity (coefficient of lnSeed) and the MPP of stem cuttings (lnSeedsq) for women farmers who belong to FBOs. This is similar to the pattern observed in the pooled sample in terms of the number of stem cuttings planted. This is perhaps an indication of a common practice among FBO members engaged in cassava cultivation. The results from the subsample of women non-members show that stem cuttings are the largest contributor to yield with an elasticity of 0.568. In model 3, both inputs (lnLabour and lnSeed) show positive yield elasticities across all sub-samples except among the male-members subsample where the yield elasticity of stem cuttings is negative, although statistically insignificant.
We attempt to estimate the technical efficiency of the farmers. The results reveal varying technical efficiency scores across the different samples. In model 1, farmers that belong to FBOs are about 52.3% technically efficient and non-members are only able to realize about 44.7% of their potential output. In model 2, the results indicate that members of FBOs are about three times more technically efficient compared to non-members. In model 3, however, the results show average TEs of about 57.8% and 62.8% for members and non-members of FBOs, respectively. Figure presents the distribution of the estimated TE scores among the sub-samples considered in this study. A quick look at the histogram for female members suggests that about 40% of female farmers who belong to FBOs have TE scores ranging from 80–99%. For male farmers who belong to FBOs, their histogram indicates that only about 25% have TE scores in the range of 80–99%. It is obvious from these percentages that there are variations in the TE of the farmers. There is, therefore, the need to assess how much of the variations in the TEs are accounted for by membership in FBOs. The next section presents the determinants of male and female farmers’ membership in FBOs and the average impact of their memberships on their respective TEs.
4.3. Determinants of membership in FBOs
Although there are obvious variations in the TEs of the men and women farmers, there is not enough evidence to attribute the observed differences to farmers’ membership or non-membership in FBOs. In this section, we attempt to find the connection between the TE of the farmers and their membership in FBOs. First, we examined the factors influencing farmers’ decision to join an FBO or not using the binary probit model, and the results are presented in Table .
Table 4. Determinants of membership in FBOs
The results indicate that among the women sample, marital status and experience in cassava cultivation are the major factors influencing the decision to join farmer groups. Specifically, a married woman is about 74% more likely to join an FBO compared to an unmarried woman. This finding is consistent with that of Adong et al. (Citation2012), who observed that in Uganda, membership in farmer unions is most probable among farmers who are married. According to Abdu et al. (Citation2022), marriage induces women’s social and economic participation and increases their concern for household welfare. Certain patriarchal norms restrict women to caregiving in households and men to income generation. In addition to giving care to household members, it is also expected of women to help their husbands on the field (Doss, Citation1999). This may present a time constraint for women to engage in other social activities like joining farmer organizations (Anyidoho et al., Citation2016). In some cases, women may serve as representatives for their husbands in the FBO. Regardless, it is more probable for a married woman to join an FBO compared to an unmarried woman. An unmarried woman has the liberty to engage in any income-generating activity in addition to farming. In Ghana, most unmarried women engage in trading. Furthermore, in rural areas in Ghana, marriage advances the social status of women, access to information and resources, and develops their decision-making capacity, thereby empowering them (Abdu et al., Citation2022; Martey et al., Citation2014). Accordingly, they are recognized and encouraged to participate in social groups including FBOs. It is noteworthy that marriage did not matter for men’s participation in FBOs. Also, a one-year increase in the experience women have in cassava cultivation leads to about a 16.5% increase in the likelihood of them joining farmer groups.
Among the men sample, the age of the farmer, the location of the farm, income from non-farm economic activities, and the size of the household are the main determinants. The result indicates that older male farmers have a higher likelihood of joining FBOs. More specifically, the probability of a male farmer becoming a member of an FBO increases by about 3% with every year increase in his age. This finding is in line with what Chagwiza et al. (Citation2016) found among smallholder dairy farmers in Ethiopia. Etwire et al. (Citation2013) and Martey et al. (Citation2014) also note that age is a proxy of resources that capacitates men to join FBOs. The administrative capital is where most financial institutions, hospitals, and markets are located. Our results indicate that farmers whose farms and, or households are located outside the administrative capital are the least likely to join FBOs. This is similar to Fischer and Qaim (Citation2012)’s finding of the non-linear relationship between smallholder banana farmers’ cooperative membership and distance to a paved road in Kenya suggesting that up to a certain distance, farmers are more willing to join the FBOs.
However, access to non-farm activities influenced men’s participation in FBOs. Most smallholder farmers depend on non-farm economic activities for extra income to complement the income from the farm. The results from this study indicate that male farmers who are engaged in non-farm economic activities have a lower probability of joining FBOs. Such farmers are about 21% less likely to join FBOs for every 1 percent increase in income from non-farm economic activities. Intuitively, farmers who earn more outside the farm will not see the need to join an FBO, and this is consistent with the random utility theory which explains that the objective of every individual is to maximize the utility of the choices they make (Cascetta & Cascetta, Citation2009). This finding is similar to Adong et al. (Citation2012), who observed that farmers in Kampala and Central 1 sub-regions of Uganda are less likely to join farmer groups. Adong et al. attributed the low participation in FBOs to the presence of government programs and NGOs in Kampala and Central 1 sub-regions.
The results in Table also show that household size reduces the likelihood of farmers’ membership in FBOs. What it means is that male farmers who have large household sizes are less likely to join. This is intuitive because most farm households in SSA depend on household members for labour (Adama et al., Citation2018). Also, most farmers join FBOs sometimes for communal support which often comes in the form of group land preparation, planting, or harvesting (Bizikova et al., Citation2020). In the pooled sample, age, and access to credit positively influences farmers to join FBOs while the location of the farm and household size have discouraging effects.
4.4. Impact of FBO membership on TE
Table presents the average treatment effect on the TE of the members of FBOs (ATET). Also presented in Table , is the potential TE of non-members if they joined FBOs (PO-means). The results from the IPW estimation of the treatment effects show that membership in FBOs has positive impacts on the technical efficiencies of the farmers (Table ). The results also show an estimated ATET of 0.121 for women and 0.037 for men. The impact among the women sample is bigger as well as statistically significant compared to that of the male sample, which is smaller and statistically insignificant. The results imply that on average, FBOs add about 12.1% to the technical efficiency of women farmers compared to an average of about 4% to the TE of male farmers. Overall, membership in FBO contributes about 9% to the technical efficiency of the sample of farmers considered in this study. The PO-mean indicates that the women non-members would enhance their estimated average technical efficiency by about 19% if they joined FBOs. Among male non-members, their average TE would increase by about 48% if they were members. The positive impact of collective action observed in this study is in line with the findings of Abdul-Rahaman and Abdulai (Citation2018), who also observed that collective action improves the technical efficiency of paddy farmers in Ghana. It, however, contradicts Addai et al. (Citation2014) who reported that collective action has zero impact on the technical efficiency of maize farmers in Ghana.
Table 5. IPW estimation of the impact of FBO membership on TE
5. Conclusions and recommendations
Using survey data from smallholder cassava farmers in the Eastern region of Ghana, this study attempts to measure the impact of collective action on the technical efficiency of member farmers. We modeled collective action through participation in FBOs. The revelation that less than half of the farmers were members of FBOs indicates a low representation of farmers. This impedes the farmers’ socio-political action, thereby limiting their ability to access services such as agricultural extension, finance, market information, and agricultural technology for both production and processing activities (Bizikova et al., Citation2020; Minah, Citation2022). Overall, FBOs attracted older and experienced farmers as well as farmers who had access to credit. Older and more experienced farmers accumulate social capital over the years and have reaped its benefits. Consequently, they have a higher propensity to join farmer groups. On the other hand, access to credit is an indicator of resource endowment. It is expected that wealthier households are more able to afford costs such as membership dues which are associated with joining farmer groups. Across gender groups, married and more experienced women had an increased likelihood of being members of FBOs. According to Minah (Citation2022), members of FBOs enjoy access to subsidized inputs such as fertilizers and other external programs which are not accounted for in impact evaluation studies. These benefits account for enhanced farm performance. After accounting for endogeneity on the disaggregated data, the impact of FBOs on technical efficiency was only realized for women. This finding implies that enhancing women’s participation in FBOs is tantamount to achieving Sustainable Development Goals 2 and 5 of zero hunger and gender equality, respectively.
It is worth noting that although the results of this study may be affected by data limitations, they are valid and of particular relevance for smallholder farming in Ghana and, therefore, have important policy implications. The findings suggest that the training and learning opportunities and the provision of other agricultural support are more beneficial to women farmers than it is to men farmers. It provides an opportunity for women to enhance their skills and allows them to participate actively in decision-making both in the household and on the farm. Future studies may consider a cross-country analysis of the impact of these farmer organizations on farm efficiency while also taking into consideration the governance structure, objectives, and size of the group.
Disclosure statement
No potential conflict of interest was reported by the author(s).
Additional information
Funding
Notes on contributors
Arnold Missiame
Arnold Missiame holds a BA in Economics and Mathematics from the University of Cape Coast, Ghana, and an MSc in Agricultural and Applied Economics from the University of Nairobi, Kenya. His research interests are in Applied Econometrics, Economic Policy Analysis, Applied Microeconomics and Macroeconomics, Statistical Modeling, and Agricultural Productivity Analysis.
Rexford Akrong
Rexford Akrong holds a BA in Economics, an MSc in Agricultural and Applied Economics, and a Collaborative Master’s in Environmental and Natural Resource Management from the University of Cape Coast, Ghana, Universities of Nairobi, Kenya, and the University of Pretoria, South Africa. He is currently a PhD candidate at the School for Development Studies at the University of Cape Coast. His research interests are in sustainable global value chains, youth development, educational policy, and natural resource management.
Grace Darko Appiah-Kubi
Grace Darko Appiah-Kubi is a PhD in Economics candidate at the University of Cape Coast, Ghana. She holds B.A and MPhil degrees in Economics from the same University. She currently is working as a part-time course tutor with the Institute of Education at the University of Cape Coast. Her fields of interest include; Macroeconomics, Financial Economics, Agricultural Economics International Economics.
References
- Abate, G. T., Francesconi, G. N., & Getnet, K. (2014). Impact of agricultural cooperatives on smallholders’ technical efficiency: Empirical evidence from Ethiopia. Annals of Public & Cooperative Economics, 85(2), 257–15. https://doi.org/10.1111/apce.12035
- Abdul-Rahaman, A., & Abdulai, A. (2018). Do farmer groups impact on farm yield and efficiency of smallholder farmers? Evidence from rice farmers in northern Ghana. Food Policy, 81, 95–105. https://doi.org/10.1016/j.foodpol.2018.10.007
- Abdu, A., Marquis, G. S., Colecraft, E. K., Dodoo, N. D., & Grimard, F. (2022). The association of women’s participation in farmer-based organizations with female and male empowerment and its implication for nutrition-sensitive agriculture interventions in rural Ghana. Current Developments in Nutrition, 6(9), nzac121. https://doi.org/10.1093/cdn/nzac121
- Abebaw, D., & Haile, M. G. (2013). The impact of cooperatives on agricultural technology adoption: Empirical evidence from Ethiopia. Food Policy, 38, 82–91. https://doi.org/10.1016/j.foodpol.2012.10.003
- Adama, I. J., Asaleye, A. J., Oye, A. J., & Ogunjobi, J. O. (2018). Agricultural production in rural communities: Evidence from Nigeria. Journal of Environmental Management & Tourism, 9(3), 428–438. https://doi.org/10.14505//jemt.v9.3(27).04
- Addai, K. N., Owusu, V., & Danso-Abbeam, G. (2014). Effects of farmer–based-organization on the technical efficiency of maize farmers across various agro-ecological zones of Ghana. Journal of Economics & Development Studies, 2(1), 141–161.
- Adolwa, I. S., Schwarze, S., & Buerkert, A. (2019). Impacts of integrated soil fertility management on yield and household income: The case of Tamale (Ghana) and Kakamega (Kenya). Ecological Economics, 161, 186–192. https://doi.org/10.1016/j.ecolecon.2019.03.023
- Adong, A., Mwaura, F., & Okoboi, G. (2012). What factors determine membership to farmer groups in Uganda? Evidence from the Uganda census of agriculture 2008/9. Journal of Sustainable Development, 6(4), 37–55. https://doi.org/10.5539/jsd.v6n4p37
- Anyidoho, N. A., Tagoe, C. A., Adjei, M., Appiah, E., Yeboah-Banin, A. A., Crentsil, A., Oduro-Frimpong, J., Owusu, A., & Torvikey, D. (2016). Shakespeare lives in Ghana: Roles, representations, perceptions of women in contemporary Ghanaian society. ISSER final report for SLIG Project.
- Bizikova, L., Nkonya, E., Minah, M., Hanisch, M., Turaga, R. M. R., Speranza, C. I., Karthikeyan, M., Tang, L., Ghezzi-Kopel, K., Kelly, J., Celestin, A. C., & Timmers, B. (2020). A scoping review of the contributions of farmers’ organizations to smallholder agriculture. Nature Food, 1(10), 620–630. https://doi.org/10.1038/s43016-020-00164-x
- Busso, M., DiNardo, J., & McCrary, J. (2014). New evidence on the finite sample properties of propensity score reweighting and matching estimators. Review of Economics and Statistics, 96(5), 885–897. https://doi.org/10.1162/REST_a_00431
- Cascetta, E., & Cascetta, E. (2009). Random utility theory. Transportation Systems Analysis: Models and Applications, 29, 89–167. https://doi.org/10.1007/978-0-387-75857-2_3
- Cassel, C. M., Särndal, C. E., & Wretman, J. H. (1983). Some uses of statistical models in connection with the nonresponse problem. Incomplete data in sample surveys, 3, 143–160.
- Chagwiza, C., Muradian, R., & Ruben, R. (2016). Cooperative membership and dairy performance among smallholders in Ethiopia. Food Policy, 59, 165–173. https://doi.org/10.1016/j.foodpol.2016.01.008
- Curtis, L. H., Hammill, B. G., Eisenstein, E. L., Kramer, J. M., & Anstrom, K. J. (2007). Using inverse probability-weighted estimators in comparative effectiveness analyses with observational databases. Medical Care, 45(10), S103–S107. https://doi.org/10.1097/MLR.0b013e31806518ac
- D’Agostino, R. B., Jr. (1998). Propensity score methods for bias reduction in the comparison of a treatment to a non‐randomized control group. Statistics in Medicine, 17(19), 2265–2281. https://doi.org/10.1002/(SICI)1097-0258(19981015)17:19<2265:AID-SIM918>3.0.CO;2-B
- Doss, C. R. 1999. Twenty-five years of research on women farmers in Africa: Lessons and Implications for Agricultural research Institutions; with an Annotated Bibliography. CIMMYT Economics Program Paper No. 99-02. CIMMYT
- Elbehri, A., & Lee, M. (2011). The role of women producer organizations in agricultural value chains practical lessons from Africa and India. FAO.
- Etwire, P. M., Dogbe, W., Wiredu, A. N., Martey, E., Etwire, E., Owusu, R. K., & Wahaga, E. (2013). Factors influencing farmer’s participation in agricultural projects: The case of the agricultural value chain mentorship project in the Northern region of Ghana. Journal Economics Sustainable Developmental, 4(No.10), 36–43.
- Fischer, E., & Qaim, M. (2012). Linking smallholders to markets: Determinants and impacts of farmer collective action in Kenya. World Development, 40(6), 1255–1268. https://doi.org/10.1016/j.worlddev.2011.11.018
- Funk, M. J., Westreich, D., Wiesen, C., Stürmer, T., Brookhart, M. A., & Davidian, M. (2011). Doubly robust estimation of causal effects. American Journal of Epidemiology, 173(7), 761–767. https://doi.org/10.1093/aje/kwq439
- Handouyahia, A., Haddad, T., & Eaton, F. (2013). Kernel matching versus inverse probability weighting: A comparative study. International Journal of Mathematical and Computational Sciences, 7(8), 1218–1233.
- Hussein, K. (2001). Producer organizations and agricultural technology in West Africa: Institutions. Local/Global Encounters, 44(4), 61–66. https://doi.org/10.1057/palgrave.development.1110294
- Ingutia, R., & Sumelius, J. (2022). Do farmer groups improve the situation of women in agriculture in rural Kenya? International Food and Agribusiness Management Review, 25(1), 135 156. https://doi.org/10.22434/IFAMR2020.0142
- Jondrow, J., Lovell, Materov, I. S., & Materow, P. (1982). On the estimation of technical inefficiency in stochastic frontier production function model. Journal of Econometrics, 19(2–3), 233–238. https://doi.org/10.1016/0304-4076(82)90004-5
- Karani, G., Ibrahim, M., & Maina, M. (2015). Factors affecting technical efficiency of passion fruit producers in the Kenya highlands. Asian Journal of Agricultural Extension, Economics & Sociology, 5(3), 126–136. https://doi.org/10.9734/AJAEES/2015/10629
- Kersting, S., & Wollni, M. (2012). New institutional arrangements and standard adoption: Evidence from small-scale fruit and vegetable farmers in Thailand. Food Policy, 37(4), 452–462. https://doi.org/10.1016/j.foodpol.2012.04.005
- Kymn, K. O., & Hisnanick, J. J. (2001). The CES–translog production function, returns to scale and AES. Bulletin of Economic Research, 53(3), 207–214. https://doi.org/10.1111/1467-8586.00131
- Lowder, S. K., Skoet, J., & Raney, T. (2016). The number, size, and distribution of farms, smallholder farms, and family farms worldwide. World Development, 87, 16–29. https://doi.org/10.1016/j.worlddev.2015.10.041
- Ma, W., & Abdulai, A. (2016). Does cooperative membership improve household welfare? Evidence from apple farmers in China. Food Policy, 58, 94–102. https://doi.org/10.1016/j.foodpol.2015.12.002
- Majurin, E. (2012). How women fare in East African cooperatives: The case of Kenya, Tanzania and Uganda.
- Mango, N., Siziba, S., & Makate, C. (2017). The impact of adoption of conservation agriculture on smallholder farmers’ food security in semi-arid zones of southern Africa. Agriculture & Food Security, 6(1), 1–8. https://doi.org/10.1186/s40066-017-0109-5
- Martey, E., Etwire, P. M., Wiredu, A. N., & Dogbe, W. (2014). Factors influencing willingness to participate in multi-stakeholder platform by smallholder farmers in Northern Ghana: Implication for research and development. Agricultural and Food Economics, 2(1), 1–15. https://doi.org/10.1186/s40100-014-0011-4
- Meier Zu Selhausen, F. (2016). What determines women's participation in collective action? Evidence from a Western Ugandan coffee cooperative. Feminist Economics, 22(1), 130–157.
- Minah, M. (2022). What is the influence of government programs on farmer organizations and their impacts? Evidence from Zambia. Annals of Public & Cooperative Economics, 93(1), 29–53. https://doi.org/10.1111/apce.12316
- Ministry of Food and Agriculture. (2017). Planting for food and jobs strategic plan for implementation (2017-2020). http://mofa.gov.gh/site/wpcontent/uploads/2018/03/PFJ%20document%20New%20New.pdf
- Missiame, A., Irungu, P., & Nyikal, R. A. (2021). Gender-differentiated stochastic meta-frontier analysis of production technology heterogeneity among smallholder cassava farmers in Ghana. African Journal of Agricultural and Resource Economics, 16(2), 140–154. https://doi.org/10.53936/afjare.2021.16(2).10
- Mwatsi, M., Vargas, D., Porciuncula, F., Aveno, J., Vallejo, C. A., & Dollente, J. (2020). Participation in farming organizations: A case study of Lofepaco in Beni-Lubero,Republic democratic of Congo. Republic Democratic of Congo December 29, 2020.
- Njobe, B. (2015). Women and agriculture: The untapped opportunity in the wave of 671 transformation. In Paper Presented at Feeding Africa conference, 21-23 October 2015, 672 Dakar, Senegal. https://www.afdb.org/fileadmin/uploads/afdb/Documents/673 Events/DakAgri2015/Women_and_Agriculture_The_Untapped_Opportunity_in_the_Wave_of_ 674 ransformation.pdf (accessed 22 June 2022).
- Onasanya, O. O., Hauser, S., Necpalova, M., Salako, F. K., Kreye, C., Tariku, M., & Pypers, P. (2021). On-farm assessment of cassava root yield response to tillage, plant density, weed control and fertilizer application in southwestern Nigeria. Field Crops Research, 262, 108038. https://doi.org/10.1016/j.fcr.2020.108038
- Owusu-Baah, K. (2012). Ghana. In H. J. Chang (Ed.), Public Policy and Agricultural Development (pp. 137–178). Routledge.
- Quisumbing, A. R., Rubin, D., Manfre, C., Waithanji, E., Van den Bold, M., Olney, D., & Meinzen Dick, R. (2015). Gender, assets, and market-oriented agriculture: Learning from high-value crop and livestock projects in Africa and Asia. Agriculture and Human Values, 32(4), 705–725. https://doi.org/10.1007/s10460-015-9587-x
- Rahman, M. S., Norton, G. W., & Rashid, M. H. A. (2018). Economic impacts of integrated pest management on vegetables production in Bangladesh. Crop Protection, 113, 6–14. https://doi.org/10.1016/j.cropro.2018.07.004
- Raney, T., Anríquez, G., Croppenstedt, A., Gerosa, S., Lowder, S. K., Matuschke, I., & Skoet, J. (2011). The role of women in agriculture. (No. 289018). Food and Agriculture Organization of the United Nations, Agricultural Development Economics Division (ESA). https://www.fao.org/3/am307e/am307e00.pdf
- Rosenbaum, P. R., & Rubin, D. B. (1985). The bias due to incomplete matching. Biometrics, 41(1), 103–116. https://doi.org/10.2307/2530647
- Salifu, A., Rebecca, L. F., Meagan, K., & Shashidhara, K., 2012. Farmer based organizations in Ghana. IFPRI working Paper 31. International Food Policy Research Institute (IFPRI).
- Seaman, S. R., & White, I. R. (2013). Review of inverse probability weighting for dealing with missing data. Statistical Methods in Medical Research, 22(3), 278–295. https://doi.org/10.1177/0962280210395740
- Shiferaw, B., Obare, G., Muricho, G., & Silim, S. (2009). Leveraging institutions for collective action to improve markets for smallholder producers in less-favored areas. African Journal of Agricultural and Resource Economics, 3(1), 1–18.
- Tefera, D. A., Bijman, J., & Slingerland, M. A. (2017). Agricultural co‐operatives in Ethiopia: Evolution, functions and impact. Journal of International Development, 29(4), 431–453. https://doi.org/10.1002/jid.3240
- Verhofstadt, E., & Maertens, M. (2015). Can agricultural cooperatives reduce poverty? Heterogeneous impact of cooperative membership on farmers’ welfare in Rwanda. Applied Economic Perspectives and Policy, 37(1), 86–106. https://doi.org/10.1093/aepp/ppu021
- Vu, L., Rammohan, A., & Goli, S. (2021). The role of land ownership and non-farm livelihoods on household food and nutrition security in rural India. Sustainability, 13(24), 13615. https://doi.org/10.3390/su132413615
- Wooldridge, J. M. (2007). Inverse probability weighted estimation for general missing data problems. Journal of Econometrics, 141(2), 1281–1301. https://doi.org/10.1016/j.jeconom.2007.02.002
- Wossen, T., Abdoulaye, T., Alene, A., Haile, M. G., Feleke, S., Olanrewaju, A., & Manyong, V. (2017). Impacts of extension access and cooperative membership on technology adoption and household welfare. Journal of Rural Studies, 54, 223–233.
- Yamane, T. (1967). Statistics, an IntroductoryAnalysis (2nd ed.). Harper and Row.
- Zheng, H., & Ma, W. (2023). Smartphone-based information acquisition and wheat farm performance: Insights from a doubly robust IPWRA estimator. Electronic Commerce Research, 23(2), 633–658. https://doi.org/10.1007/s10660-021-09481-0