Abstract
This study comprehensively reviews the key influential and intellectual aspects of machine learning in finance. The authors employ the bibliometric approach using VOSviewer software to analyse 189 academic articles from the SCOPUS database between 1988 and December 2022. Our results revealed that machine learning in the finance literature has significantly increased since 2017, indicating that the finance industry had some time to adopt newer technology. The authors find that the United States, China, and the United Kingdom were the countries that most frequently investigated this topic. It was also found that the Steven Institute of Technology (New Jersey, United States) is the most active research institute in this field. We also discovered that the application of machine learning has been adopted in crowdfunding, FinTech, forecasting, bankruptcy prediction, and computational finance. Our research is subject to several limitations. This research only utilised the SCOPUS database and was restricted to articles written in English. Our findings assist academic scholars in exploring issues related to machine learning in finance in future studies. The outcomes of the present study may also guide market participants, particularly FinTech and finance companies, on how machine learning could be used in their decision-making.
1. Introduction
Machine learning is a potent subset of the broader field of Artificial Intelligence (AI). It fundamentally involves training computer systems to comprehend, interpret, and learn from intricate patterns within data sets (Sengupta et al., Citation2020). This understanding and interpretation are not achieved through explicit programming but through the system’s ability to learn and adapt its knowledge from past data. As such, machine learning signifies a significant shift from traditional programming paradigms where specific instructions are given to machines. Instead, machine learning gives machines the autonomy to derive and apply logic in dynamic contexts.
The impact of this shift is substantial, and machine learning has been applied across an array of diverse domains. For instance, in natural language processing, machine learning is used to teach machines how to understand human language, interpret it, and even generate human-like text. This technology underpins many of today’s digital assistants and chatbots, enabling more natural and intuitive interactions between humans and machines (Sengupta et al., Citation2020). Similarly, in image recognition, machine learning algorithms are used to detect and interpret visual patterns. This has widespread applications, from diagnosing diseases through medical imaging to powering autonomous vehicles by recognising road signs and obstacles (Naseer et al., Citation2022). In pattern detection, machine learning is employed in cybersecurity and fraud, where unusual patterns can signify threats or malicious activity (Suryanarayana et al., Citation2018). Lastly, predictive analysis leverages the predictive capabilities of machine learning, enabling businesses to forecast trends, customer behaviour, and market dynamics (Dananjayan & Raj, Citation2020). Indeed, the versatility of machine learning is vast, and its potential for future applications is continually expanding.
It is interesting to obtain an overview of how machine learning has been studied and published in the context of finance. Finance is a crucial area closely associated with the economy. Over the last ten years, for example, financial technologies (FinTech) have become more prominent among businesses (Varma et al., Citation2022).
In the sphere of financial data and technology, the influence of machine learning is extensive and transformative. Machine learning algorithms have significantly influenced numerous areas of finance, from portfolio management to risk mitigation, heralding a new era of data-driven decision-making (Bao et al., Citation2017).
A crucial application of machine learning in finance is in forecasting market trends. Traditional market analysis models have encountered challenges in handling the complexity and volatility of financial markets. However, with machine learning, complex patterns within the data can be identified and used to predict future market movements with a considerably higher degree of accuracy (Kumar & Thenmozhi, Citation2006).
Evaluating investment opportunities is another area where machine learning has demonstrated its worth. Through data mining and predictive modelling, machine learning algorithms can analyse vast amounts of financial data to determine the potential return and risk associated with different investment options. These capabilities have proven invaluable for financial advisors, hedge fund managers, and individual investors, all requiring precise and timely information to make informed investment decisions (Baek & Kim, Citation2020).
Fraud detection is another domain that has been revolutionised by machine learning. Traditionally, detecting fraudulent transactions was a time-consuming and error-prone task. However, with machine learning’s pattern recognition capabilities, anomalies can be identified more quickly and accurately, protecting businesses and consumers from financial loss (Ileberi & Uzoka, Citation2022).
Additionally, the rise of FinTech further underscores the pervasive influence of machine learning in finance. Blockchain technology, underpinned by machine learning, has led to decentralised finance and cryptocurrencies, changing how we think about money and transactions (Shengelia et al., Citation2022). Similarly, smart contracts and automated trading systems powered by machine learning redefine business agreements and stock trading (Manimuthu et al., Citation2022).
Despite these significant advancements, the potential of machine learning in finance is far from exhausted. There are vast avenues for research and application yet to be explored, which will undoubtedly lead to further groundbreaking innovations. This realisation underscores the relevance and timeliness of our study, which seeks to chart the course for future research and applications of machine learning in finance.
Hence, the main aim of this study is to provide an overview of past publications related to machine learning and finance. The study’s purpose is to answer the following questions: (1) What are the key themes? (2) What is the trend of co-authorship among authors? (3) What are the keywords used?
To answer the first question, the following research objectives were formulated: to examine the growth of machine learning and finance literature (1998–2022); to study the most relevant countries by the corresponding author; to identify the ten most relevant institutions; to find the ten most relevant journals; to determine the most relevant authors; and to analyse the ten most-cited academic articles.
The co-authorship network of key authors and the ten most key authors based on co-authorship were investigated to address the second research question: What is the trend of co-authorship among authors? Finally, we examined the co-occurrence networks of keywords and the top keywords used in publications.
The three research questions were addressed using bibliometric analysis. Bibliometric analysis is a statistical analysis of books, articles, or other publications. It tracks an author or researcher’s output and impact, and calculates journal impact factors (Donthu et al., Citation2020, Citation2021). This study conducted a bibliometric analysis using the keywords “Machine Learning” and “Finance” and extracted data from the SCOPUS database. The analysis provided an overview of the publications related to the area and identified the authors who contributed to the area, their institution and country of origin, and their co-authors. An in-depth analysis was also carried out to analyse their contributions. This information will be useful for new researchers in the area as it provides a landscape of the relevant literature for those conducting such research.
2. Methodology
Machine learning plays a significant role in finance, allowing financial entities to analyse and make decisions in areas such as fraud detection, trading and investment, risk assessment, and credit scoring. As the goal of this study was to provide the broadest coverage of peer-reviewed research in machine learning and finance, we searched the literature using the SCOPUS database.
2.1. Information extraction strategies
2.1.1. Range, language, subject area, and document type
The literature search was carried out in July 2023. We compiled articles from all years between 1988 and December 2022. English-language research publications published in the SCOPUS database were chosen for this study’s review. We also selected articles and conference papers in the areas of Economics, Econometrics, and Finance.
2.1.2. Key selection strategy (search string)
The most vital step in the search process is performing the key selection strategy and refinement. Adopting a similar approach to Alshater et al. (Citation2022), we searched for articles using the following keywords: “Machine Learning” OR “Machine Learning” OR “Machine” AND “Finance” OR “Finance”. This strategy enabled us to thoroughly evaluate the literature on machine learning and finance and classify the various research streams and future research prospects. The search was conducted within the article title, abstract, and keyword fields.
The search returned 214 articles. The authors then conducted a second screening of the literature to confirm the findings of our original search. Twenty-five articles were omitted as they were not relevant, resulting in 189 articles published between 1988 and December 2022.
The flow diagram of the study is illustrated in Figure .
Figure 1. PRISMA flow chart of the study on machine learning and finance.
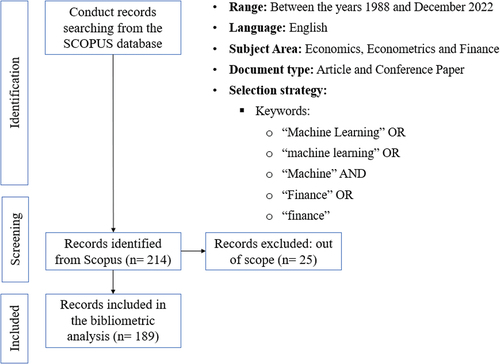
3. Method
Our study employs a bibliometric (quantitative) analysis, following several previous studies (see Alshater et al., Citation2021; Baker et al., Citation2020; Paltrinieri et al., Citation2019; Pattnaik et al., Citation2020). The bibliometric technique concentrates on two main goals: (1) performance analysis to examine the impact of research domains on the study and (2) scientific mapping tools to investigate the connections between the research domains. According to studies in the literature (Donthu et al., Citation2020, Citation2021; Verma & Gustafsson, Citation2020), applying bibliometric analysis enables researchers to discover new patterns in the performance of articles and journals, patterns of collaboration, and research components, as well as to investigate the intellectual framework of a particular field in the literature.
3.1. Analysis
Based on the research objectives, the authors addressed the following areas: (1) bibliometric analysis of the themes and trends of various research constituents (authors, countries, institutions, and journals); (2) bibliometric analysis of co-authorship; and (3) bibliometric analysis of co-words.
The social connections between authors, their affiliations, and their corresponding effects on the advancement of the study area were examined using the scientific mapping of co-authorship analysis. The method recognises the authors and their connections (Acedo et al., Citation2006; Donthu et al., Citation2021). Co-word analysis is a technique that focuses on the written text of the articles to investigate past, present, and potential links between themes (Donthu et al., Citation2021; Emich et al., Citation2020).
3.2. Software
VOSviewer software is used in this work to create and view bibliometric maps (Van Eck & Waltman, Citation2010, Citation2017). It is a well-known and popular choice for bibliometric studies (Alshater et al., Citation2021; Baker et al., Citation2020; Paltrinieri et al., Citation2019; Pattnaik et al., Citation2020). With this technique, researchers may see the dynamics and structure of previous studies on machine learning and finance challenges (Baker et al., Citation2020; Donthu et al., Citation2021).
4. Results and discussion
This section discusses the outcomes of bibliometric analysis conducted. It is divided into three sub-sections according to the research questions. Section 4.1 presents the key themes in Machine Learning and Finance Literature, while in Section 4.2 the trends of co-authorship is presented. Finally, the co-word analysis is illustrated in Section 4.3.
4.1. Key themes in the machine learning and finance literature
The general information about the input data used for the analysis is shown in Table . Our search found 189 articles related to machine learning and finance topics between the years 1988 and 2022. These involved 201 authors, 30 of whom conducted the research independently, giving an average of 0.94 documents per author. Review articles accounted for only 6% of the total. The average number of citations per document over time is 20.81, which appears to be relatively high. As a result, it is anticipated that the overall publishing and citation of issues linked to machine learning and finance will continue to grow as more researchers become interested in this area.
Table 1. General information
4.1.1. Growth of machine learning and finance literature
Figure shows the annual growth in published documents from 1988 to 2022. Although machine learning and finance studies have a history that dates to the 1930s, a literature review suggests that the dispersion of published documents was relatively low before 2014 due to the limited amount of data that was then available. There has been an increase in research on the study since 2015. The availability of massive data, improvements in processing power, and the development of complex algorithms are most likely contributing factors. The peak annual growth rate for machine learning research in finance is estimated to be 37.5% in 2022, which reflects both a rising interest in AI and a rising demand for data-driven solutions.
4.1.2. The most relevant countries by the corresponding author
The number of published documents from different countries during the same period is shown in Figure . These represent only the countries of the corresponding authors, not those of the other co-authors. According to Figure , the USA is the largest contributor to the field of machine learning, with China and the UK following in second and third place, respectively. The reason for the USA being the largest contributor is perhaps because many of the forerunners in machine learning and its application in finance (e.g. David Shaw, James Simon) were Americans; hence, the area of research was first introduced there.
Surprisingly, this study shows that research in the field has been experiencing exponential growth in developing nations like China, India, and South Korea, providing evidence of their ability to keep up with technological advancements, less restrictive data protection laws, and wide-ranging access to various types of financial data.
4.1.3. The ten most relevant institutions
Table lists the ten most productive institutions, according to the number of publications published, as well as the affiliations of the corresponding authors. The following institutions are listed along with their countries of origin: Nankai University (China), Harbin Institute of Technology (China), Malaviya National Institute of Technology (India), University of Glasgow (UK), and Delft University of Technology (Netherlands). Apart from the Stevens Institute of Technology, which is a private university, these universities are primarily public research universities.
Table 2. The ten most relevant institutions
It is interesting to note that, although the most prolific contributors related to machine learning and finance are from the USA (Figure ), only one institution from the USA is listed among the ten most relevant institutions (i.e. the Stevens Institute of Technology). The articles from the Stevens Institute of Technology were further analysed, and it was found that of its five articles, four were co-authored by G. G. Creamer, and he was the sole author for the article “Linking entity resolution and risk”. He co-authored two articles: “Leveraging social media to predict continuation and reversal in assets prices” and “Can sentiment analysis and options volume anticipate future returns?” with P. Houlihan, based at the same institute. In addition, he also co-authored a publication with H. Ghodussi, who was also affiliated with the Stevens Institute, and N. Rafizadeh, an independent researcher from the Sharif University of Technology, Iran.
Potential students, academics, and business professionals can refer to Table to discover the relevant universities with the necessary facilities and expertise in machine learning and finance research.
4.1.4. Ten most relevant journals
The most pertinent journals that publish works in machine learning are listed in Table . The journals indicated in this listing are available to authors who intend to publish work in this area. The top ten journals are published by John Wiley & Sons Ltd, Springer Netherland, MDPI AG, and KEAi Communication Co. Four of the top ten journals are owned by Elsevier and two by the Emerald Group. We also note that Elsevier publishes the journals “Knowledge-Based Systems”, “Technological Forecasting and Social Change”, “Decision Support Systems”, and “Journal of International Financial Markets, Institutions and Money”, all of which were ranked Q1 and Q2 in Scopus. These journals also have the list’s highest JH index. The advancing technological horizon has also increased interest in machine learning research in predicting publications since 2015.
Table 3. The ten most relevant journals
It is observed that many of the publications (Table ) appear in non-technology-based journals, suggesting that the articles are primarily on the application of machine learning tools to the financial environment, as the search was limited to specific relevant keywords (machine learning and finance) as well as journals limited to Economics, Econometrics, and Finance. Of the ten journals, only two are open-access (“Risks” and “Journal of Finance and Data Science”).
Further analysis illustrates that several journals (“Journal of Forecasting”, “Knowledge-Based Systems”, “Decision Support Systems”, and “Computational Economics”) accept manuscripts that are multidisciplinary and use advanced computing capabilities.
The list of the top ten sources of publications can be used as a guide for novice researchers in the area. In addition, it will help researchers find an outlet for their articles in a similar area. Thus, the study can be used as a future reference for other researchers as it provides a valuable analysis of the application of machine learning in finance, such as forecasting and investing.
4.1.5. The most relevant authors
The most relevant authors who have written about machine learning in finance are shown in Figure . Most authors wrote only three articles on average. This implies that research on machine learning is still in its infancy. Along with advances in FinTech, other fields like natural language processing, computer vision, reinforcement learning, and the study of machine learning are predicted to make substantial strides in the coming years. It has to be highlighted here that the analysis was based on the name and initials of the authors. For example, G. G. Creamer has three articles with both initials and one with only a single “G.”; hence, the latter was not included in the overall analysis as it was assumed to be a different author.
The authors analysed whether any of the ten relevant authors (Figure ) came from the top ten institutions (Table ). It was found that only M. A. M. Al Janabi and G. G. Creamer were from that list (from Tecnológico de Monterrey and the Stevens Institute of Technology, respectively).
4.1.6. The ten most-cited academic articles
The top ten academic articles on machine learning are shown in Table . Seven articles have more than 100 citations, while one has more than 200. None have fewer than 50 citations, while most of the research has between 100 and 200 citations. Except for the second-most cited article, which was published in a Q2 journal, all publications are from Q1 journals, indicating a strong association between the rating of the journal and the number of citations in this field.
Table 4. The ten most-cited academic articles
The ten academic articles with the most citations are discussed below, along with their study findings.
Dwivedi et al. (Citation2021) explored AI and its implications for the future of industry and society. According to their study, a similar potential exists for the augmentation and replacement of human tasks utilising algorithmic machine learning. This technical advancement could aid decision-making and allow for the advancement of innovation in various businesses, including banking, manufacturing, and healthcare, as well as the government and public sectors.
Ma et al. (Citation2018) investigated the anticipated default rate on credit using “multi-observation” and “multi-dimensional” data cleaning methodologies, as well as recent machine learning algorithms LighGBM and Xgboost, based on peer-to-peer transaction data gathered from Lending Club. Evidence suggests that the default risk of loans on the platform is substantially predicted by LightGBM algorithms, owing to their higher performance.
Following Ma et al. (Citation2018), Ghoddusi et al. (Citation2019) utilised machine learning algorithms to predict the default rate on credit in peer-to-peer lending. Using data from Lending Club, the authors used the “multi-observation” and “multi-dimensional” data cleaning methods, as well as the modern machine learning algorithms LightGBM and XGboost. The platform’s default risk was strongly and innovatively predicted, in which the multi-dimensional Light GBM performed the best (the loan defaults have been reduced by nearly $117 million).
Zhu et al. (Citation2019) proposed an improved hybrid ensemble ML strategy called RS-MultiBoosting that combines two classic ensemble ML algorithms, random subspace (RS) and MultiBoosting, to increase the accuracy of forecasting SMEs’ credit risk. The experimental samples were derived from data on 46 quoted SMEs and seven quoted core enterprises (CEs) on the Chinese securities market between 31 March 2014 and 31 December 2015. They were used to examine the feasibility and effectiveness of the RS-MultiBoosting approach. The forecasting result revealed that RS-MultiBoosting performs well when dealing with a small sample set. According to the SCF, the findings indicate that “traditional” characteristics, such as SMEs’ current and quick ratios, remain crucial in improving SMEs’ financing ability.
A comprehensive investigation was undertaken by Liang et al. (Citation2015) to investigate the effect of conducting filter and wrapper-based feature selection methods on financial distress prediction. The study also investigated the impact of feature selection on prediction models derived from various classification algorithms. Two bankruptcy and two credit datasets were employed. In addition, three filter and two wrapper-based feature selection strategies were investigated, along with six alternative prediction models. Over the four datasets, the experimental results revealed that there is no ideal combination of feature selection method and classification methodology. Furthermore, depending on the approaches used, feature selection does not necessarily increase prediction performance. However, on average, the study contends that using the evolutionary algorithm with logistic regression for feature selection can enhance prediction over credit and bankruptcy datasets.
Hajek and Henriques (Citation2017) aimed to investigate whether integrating certain traits generated from financial data and managerial remarks in corporate annual reports may result in a better financial fraud detection system. The study utilised a variety of machine learning methods to construct this system, including intelligent feature selection and categorisation. In terms of true positive rate (fraudulent businesses correctly labelled as fraudulent), the authors conclude that interpretable “green flag” values (indicating the absence of fraud) could be produced, thereby providing decision support to auditors during client selection or audit preparation. The study also contends that non-annual report data (analyst projections of revenues and earnings) are required to uncover fraudulent organisations.
Checchini et al. (2010) used support vector machines to detect management fraud using basic financial data. A substantial empirical data set with quantitative financial features for fraudulent and nonfraudulent public enterprises was gathered. In a holdout set, support vector machines utilising the financial kernel correctly labelled 80% of the fraudulent cases and 90.6% of the nonfraudulent cases. In addition, the authors duplicated other top fraud research studies and discovered that their method has the highest accuracy on fraudulent cases and comparable accuracy on nonfraudulent cases. The findings indicate the usage of the financial kernel in conjunction with support vector machines as an effective strategy for distinguishing between fraudulent and nonfraudulent organisations using only publicly known quantitative financial data.
Uthayakumar et al. (Citation2020) utilised an Ant Colony Optimisation (ACO)-based financial crisis prediction (FCP) model with two phases: ACO-based feature selection (ACO-FS) and ACO-based data categorisation (ACO-DC). A collection of five benchmark datasets, both qualitative and quantitative, were used to validate the proposed ACO-FCP model. The created ACO-FS approach was compared to three feature selection algorithms, including the genetic algorithm, the Particle Swarm Optimisation algorithm, and the Grey Wolf Optimisation algorithm, for feature selection design. Furthermore, classification results were compared between ACO-DC and state-of-the-art approaches. According to the experimental results, the ACO-FCP ensemble model was superior and more robust than its competitors. As a result, the study strongly suggests that the ACO-FCP model is far superior to traditional and alternative artificial intelligence methodologies.
Schebesch and Stecking (Citation2005) used a statistical model to map inputs to the labels that can determine whether a new credit applicant should be accepted or rejected. Using statistical learning theory’s support vector machines (SVM), the study developed extremely weak prior assumptions about the model structure and divided a set of labelled credit applicants into subsets of “typical” and “critical” patterns. The findings show that, even with linear classification methods, the proper class label of a common pattern is usually relatively easy to anticipate. The correct class label of a typical pattern is usually easy to predict, even with linear classification methods. Such patterns do not contain much information about the classification boundary. In the results for non-linear SVM, more “surprising” critical regions may be detected, but—owing to the relative sparseness of the data—this potential seems to be limited in credit scoring practice.
Using Latent Dirichlet Allocation machine learning models on a variety of US stock indices and equities, Atkins et al. (Citation2018) showed that information derived from news sources is better at forecasting the direction of underlying asset volatility movements or its second-order statistic than its price movement direction. The results showed that the average directional prediction accuracy for volatility was 56% when fresh information was received; however, the asset close price accuracy was only 49%. The authors conclude that volatility movements are more predictable than asset price changes when utilising financial news as machine learning input. As a result, volatility quantification may be used to improve the pricing of derivatives contracts.
We further analysed the link between top institutions, most relevant authors, and top ten citations. We noticed that the Stevens Institute of Technology has the highest number of articles and that one of their researchers (G. G. Creamer) is one of the top ten authors in the area. In addition, the article by Ghoddusi et al. (Citation2019) was listed as the third most cited article (130 citations). Both Ghoddusi and Creamer are from the Stevens Institute of Technology.
When we reviewed the top ten publications (Table ) and compared them with Table , we found that only three citations were published in the top ten. They are Atkins et al. (Citation2018) in the “Journal of Finance and Data Science” and Liang et al. (Citation2015) and Hajek and Henriques (Citation2017) in the journal “Knowledge-Based Systems”.
4.2. Trend of co-authorship among authors
In this section, our analysis aimed to identify the intellectual aspects of the machine learning research area, including the co-authorship network of key authors and the ten most key authors based on co-authorship analysis.
4.2.1. Co-authorship network visualisation of key authors
Our investigation attempts to examine how researchers in the field interact with one another (Cisneros et al., Citation2018; Donthu et al., Citation2021; Pattnaik et al., Citation2020), realising that scholarly collaborations are becoming increasingly prevalent (Acedo et al., Citation2006) and that they produce richer and more distinct understandings (Tahamtan et al., Citation2016).
Of the 201 authors, seven have the necessary minimum of three documents and no citations to provide significant results. The co-authorship network of important writers in machine learning is shown in Figure , with a total of six nodes and a total link strength of 16. The number of nodes and links translates the number of citations and the connections between each author and other researchers in the network.
Figure 5. Co-authorship network of key authors.
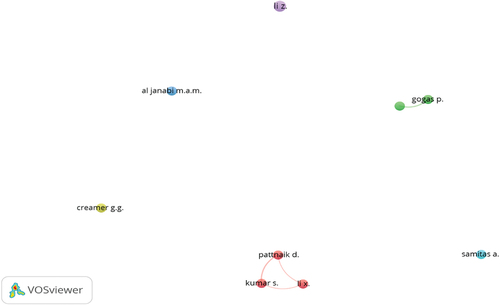
The analysis indicates that the number of citations and the connections between each author and other researchers in the network is minimal. It suggests that authors in this area are more comfortable to work with their own colleagues within their own institutions.
4.2.2. Key authors based on co-authorship analysis
Table lists the important authors based on the co-authorship analysis output and citation data. According to the data, G. G. Creamer (Stevens Institute of Technology; 140 citations) is the most well-known and prominent author of studies on machine learning in finance, followed by S. Kumar (Malaviya National Institute of Technology; 73 citations), D. Pattnaik (Woxsen University Telangana, India; 73 citations, and P. Gogas (Democritus University of Thrace; 47 citations).
Table 5. Nine most key authors based on co-authorship analysis
Further analysis was carried out to observe whether there is a link between key authors based on co-authorship analysis (Table ) and the most relevant author (Figure ). It was found that G. G. Creamer was ranked second in Figure but top in Table , illustrating that he is one of the top researchers in the area of machine learning in finance. Besides him, six authors appear in both Table and Figure : S. Kumar, D. Pattnaik, P. Gogas, T. Papadimitriou, A. Samitas, and M. A. M. Al Janabi, thus showing the consistencies in the analysis.
In addition, it was also observed that G. G. Creamer, S. Kumar, X. Li, and M. A. M. Al Janabi were affiliated with Stevens Institute of Technology, Malaviya National Institute of Technology, Nankai University, and Tecnologico de Monterrey, respectively. These institutions have been listed among the ten most relevant institutions in machine learning in finance (Table ).
4.3. Co-word analysis
4.3.1. The discussion on the co-word analysis focuses on co-occurrence network of keywords and the most frequent keywords used in publications
According to Donthu et al. (Citation2021), co-word analysis examines a publication’s real content. The current study examines the top ten keywords (Table ), the co-occurrence network of all terms (Figure ), and the map of keyword density in machine learning in finance research or classification (Figure ).
Figure 6. Co-occurrence network of keywords.
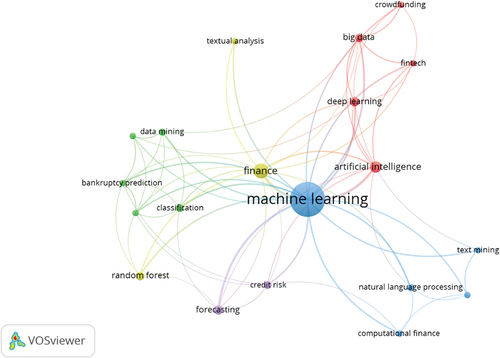
Figure 7. Keywords density visualisation map.
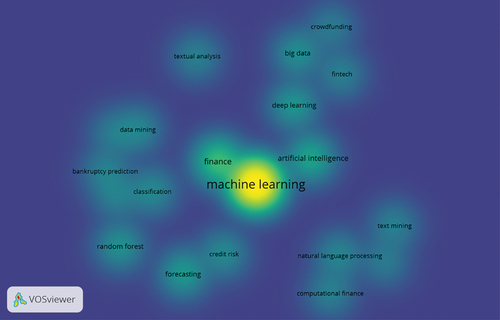
Table 6. The top 10 keywords of publications
4.3.2. Co-occurrence network of keywords
We retrieved 641 keywords from the 189 documents chosen for our study, 20 of which comply with the criterion, and the minimum occurrence of a keyword is 5. From Figure , the study identifies five clusters in this field of research.
4.3.3. Top keywords used in publications
From Table , the results indicate that the main core of keywords in the research topic were as follows: “Machine Learning” (147 occurrences and 128 total link strength); “finance” (28 occurrences and 42 total link strength); “artificial intelligence” (16 occurrences and 31 total link strength); “forecasting” (12 occurrences and 18 total link strength); “deep learning” (11 occurrences and 19 total link strength); “credit risk” (8 occurrences and 14 total link strength); “Data mining” (7 occurrences and 10 total link strength); “Natural language processing” (7 occurrences and 11 total link strength); and “computational finance” (6 occurrences and 9 total link strength).
In Figure , we plot the visual density map to visualise the keywords. The graphic helps us comprehend that each node has a distinct colour representing the density of the items, ranging from blue to green to yellow. Notably, the keywords highlighted in yellow are more prevalent and have greater weight. On the other hand, the point’s colouration tends to resemble blue with decreasing frequency and weight. According to the findings displayed in the network visualisation of keywords, we determined that the most significant keywords are “Machine Learning”, “finance”, “artificial intelligence”, “forecasting”, “deep learning”, and “credit risk”, followed by others.
Figure and Table clearly demonstrate that the main keyword used by the authors was “machine learning”, which aligns with the main keyword search used in this study. Future research in this area could benefit from this study. Instead of just using “machine learning”, researchers can use “Artificial intelligence”, “Deep learning”, and “Natural language processing”. Furthermore, the word “finance” can be used alternatively to “Forecasting”, “Credit risk”, and “Computational finance” to capture more literature pertaining to machine learning in finance. In addition, based on the keyword density visualisation map in Figure , it is suggested that future research could focus on the adoption of AI to forecast financial issues (Dwivedi et al., Citation2021).
5. Conclusion
Our paper aimed to obtain an overview of current articles on machine learning and finance published in the SCOPUS database. A total of 189 articles were extracted, reviewed and analysed.
The number of publications on the application of machine learning in the finance area is growing and there is 37.5% growth in 2022, as can be seen in Figure . As more and more researchers contribute to the field, we observed that the main themes of machine learning in the financial context can be categorised into credit risk (articles 2, 3, 4, and 9 in Table ), fraud management (articles 6 and 7) and forecasting or prediction (articles 5, 8, and 10). Nevertheless, some articles focus on the general application of machine learning in the financial sector, such as Dwivedi et al. (Citation2021), which may have contributed to it being the top-cited article in the research.
Machine learning has made significant contributions to addressing issues related to safety, security, and reliability in financial transactions. Based on articles written on fraud management (Cecchini et al., Citation2010; Hajek & Henriques, Citation2017), machine learning algorithms were able to detect odd or suspect activity. These algorithms examine transaction data, user behaviour, and historical patterns, and can detect different kinds of fraud, such as insider trading, account takeover fraud, and credit card fraud.
Authors that have written on credit risk (Ghoddusi et al., Citation2019; Ma et al., Citation2018; Schebesch & Stecking, Citation2005; Zhu et al., Citation2019) stated that machine learning is used to create more precise credit scoring models that determine a person’s or an organisation’s creditworthiness. This minimises credit risk by ensuring that loans are only made to borrowers with a higher likelihood of repaying them. Besides credit risk and fraud management, machine learning also contributed to financial crisis prediction (Uthayakumar et al., Citation2020), asset movement forecasting (Atkins et al., Citation2018), and financial distress (Liang et al., Citation2015). Therefore, it can be concluded that machine learning addresses issues related to safety, security, and reliability in financial transactions.
Machine learning algorithms can evaluate enormous amounts of data and extract insightful information to assist decision-making. Financial experts may use this information to make more informed decisions (Dwivedi et al., Citation2021). In a way, it is replacing the tasks that are normally conducted by humans, but not always accurately due to the vast amount of data involved.
Our findings also illustrate that most publications originate from the US and UK, as well as China, India, and South Korea (Figure ). There have been relatively few such studies conducted in Malaysia or its neighbouring countries, revealing a gap that researchers in Malaysia can address.
The results on the most relevant authors (Figure ), ten most-cited academic articles (Table ), and ten key authors based on co-authorship analysis (Table ), demonstrate the list of researchers who have worked on machine learning in the finance context. This list could help ensure more systematic literature reviews in the future. Supervisors may use it as a source for finding examiners. Although the Tables also revealed the number of citations, it must be used with caution as the number of citations may depend on the accessibility and visibility of the journals.
As with any other study, ours is not without limitations. The study extracted data from the SCOPUS database only, but future studies might also include other databases, such as Web of Science and Emerald.
This study focused on two main keywords: machine learning and finance. Future studies can expand the use of keywords, to include some of those illustrated in Figures and Table . The use of additional keywords would provide a wider spectrum of publications, although additional efforts would be necessary to avoid duplications.
Acknowledgments
We fully appreciate the two anonymous referees for their constructive comments.
Disclosure statement
No potential conflict of interest was reported by the author(s).
Additional information
Notes on contributors
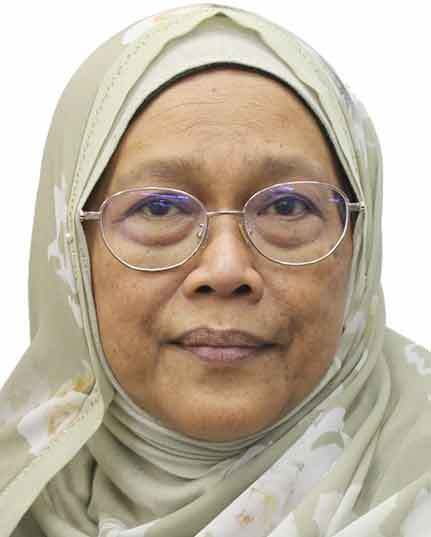
Nadisah Zakaria
Nadisah Zakaria is an accomplished scholar and educator with extensive experience in finance and business. Her dedication to research, teaching, and program development has made her a valuable asset to various institutions and organisations in Malaysia, Saudi Arabia, and the United Kingdom. Dr. Nadisah's research interests encompass corporate finance, corporate restructuring, mergers and acquisitions, as well as pricing and mispricing of assets. She has received recognition for her work, including a prestigious studentship for her Ph.D. studies. With a strong commitment to academia and a wealth of experience, Dr. Nadisah Zakaria continues to contribute significantly to the field of finance.
Ainin Sulaiman
Ainin is currently attached to the Faculty of Business, International University of Malaya-Wales. Prior to that she was with Universiti Malaya for thirty years. She has authored or co-authored over 100 refereed publications in local and international journals, book chapters, and conference proceedings, among them are Sustainability, Computers in Human Behaviour, Quality and Quantity, PLOS One, Management Decision, IEEE Access, and Government Information Quarterly. Ainin has led several research projects among them the social economic impact of the Light Emitting Diode (LED) in Malaysia. Her research interests include, Technology Adoption, Performance, ICT, Social Media, Big data, Halal, and social economic impact.
Foo Siong Min
Foo Siong Min has extensive experience in finance and corporate management. He is currently a Ph.D. candidate in Finance at Universiti Putra Malaysia, where he is researching the volatility spillover, market integration, and performance of Islamic and conventional equity markets. Previously, he worked as a Vice President at Makto Capital Management Limited in Hong Kong, where he structured, originated, and advised on transactions related to public and private equity and debt investments. He also worked as a Vice President - Research at Ping An China Securities (Hong Kong) Company Limited, where he focused on cross-border M&A, corporate finance activities, and IPOs across Asia.
Ali Feizollah
Ali Feizollah received his Bachelor of Information System (IS) from the Ajman University of Science and Technology (AUST), Ajman, UAE in 2010. He started his Master of Computer Science at the University of Malaya, Kuala Lumpur in 2011. He obtained his PhD from the same university in 2017. He was a Postdoctoral Research Fellow in the University of Malaya and currently is attached to the School of Digital Technology, Brickfields Asia College, Selangor, Malaysia. His research interests are mobile malware, intrusion detection system, sentiment analysis, and machine learning.
References
- Acedo, F. J., Barroso, C., Casanueva, C., & Galán, J. L. (2006). Co-authorship in management and organisational studies: An empirical and network analysis. Journal of Management Studies, 43(5), 957–19. https://doi.org/10.1111/j.1467-6486.2006.00625.x
- Alshater, M. M., Hassan, M. K., Khan, A., & Saba, I. (2021). Influential and intellectual structure of Islamic finance: a bibliometric review. International Journal of Islamic & Middle Eastern Finance & Management, 14(2), 339–365. https://doi.org/10.1108/IMEFM-08-2020-0419
- Alshater, M. M., Saba, I., Supriani, I., & Rabbani, M. R. (2022). FinTech in Islamic finance literature: A review. Heliyon, 8(9), e10385. https://doi.org/10.1016/j.heliyon.2022.e10385
- Atkins, A., Niranjan, M., & Gerding, E. (2018). Financial news predicts stock market volatility better than close price. The Journal of Finance and Data Science, 4(2), 120–137. https://doi.org/10.1016/j.jfds.2018.02.002
- Baek, Y., & Kim, N. (2020). Investor sentiment in the stock market and economic sectors: Evidence from textual analysis. Expert Systems with Applications, 143, 113091. https://doi.org/10.1016/j.eswa.2019.113046
- Baker, S. R., Bloom, N., Davis, S. J., Kost, K., Sammon, M. C., & Viratyosin, T. (2020). The unprecedented stock market impact of COVID-19. The Review of Corporate Finance Studies, 9(April), 622–655.
- Bao, W., Yue, J., & Rao, Y. (2017). A deep learning framework for financial time series using stacked autoencoders and long-short-term memory. PLoS One, 12(7), e0180944. https://doi.org/10.1371/journal.pone.0180944
- Cecchini, M., Aytug, H., Koehler, G. J., & Pathak, P. (2010). Detecting management fraud in public companies. Management Science, 56(7), 1146–1160. https://doi.org/10.1287/mnsc.1100.1174
- Cisneros, L., Ibanescu, M., Keen, C., Lobato-Calleros, O., & Niebla-Zatarain, J. (2018). Bibliometric study of family business succession between 1939 and 2017: Mapping and analysing authors’ networks. Scientometrics, 117(2), 919–951. https://doi.org/10.1007/s11192-018-2889-1
- Dananjayan, S., & Raj, G. M. (2020). Artificial intelligence during a pandemic: The COVID-19 example. The International Journal of Health Planning and Management, 35(5), 1260. https://doi.org/10.1002/hpm.2987
- Donthu, N., Kumar, S., Mukherjee, D., Pandey, N., Lim, W. M., & Marc, W. (2021). How to conduct a bibliometric analysis: An overview and guidelines. Journal of Business Research, 133(April), 285–296. https://doi.org/10.1016/j.jbusres.2021.04.070
- Donthu, N., Kumar, S., & Pattnaik, D. (2020). Forty-five years of journal of Business research: A bibliometric analysis. Journal of Business Research, 109(October 2019), 1–14. https://doi.org/10.1016/j.jbusres.2019.10.039
- Dwivedi, Y. K., Hughes, L., Ismagilova, E., Aarts, G., Coombs, C., Crick, T., Duan, Y., Dwivedi, R., Edwards, J., Eirug, A., Galanos, V., Ilavarasan, P. V., Janssen, M., Jones, P., Kar, A. K., Kizgin, H., Kronemann, B., Lal, B. … others. (2021). Artificial intelligence (AI): Multidisciplinary perspectives on emerging challenges, opportunities, and agenda for research, practice and policy. International Journal of Information Management, 57, 101994. https://doi.org/10.1016/j.ijinfomgt.2019.08.002
- Emich, K. J., Kumar, S., Lu, L., Norder, K., & Pandey, N. (2020). Mapping 50 years of small group research through small group research. Small Group Research, 51(6), 659–699. https://doi.org/10.1177/1046496420934541
- Ghoddusi, H., Creamer, G. G., & Rafizadeh, N. (2019). Machine learning in energy economics and finance: A review. Energy Economics, 81, 709–727. https://doi.org/10.1016/j.eneco.2019.05.006
- Hajek, P., & Henriques, R. (2017). Mining corporate annual reports for intelligent detection of financial statement fraud – a comparative study of machine learning methods. Knowledge-Based Systems, 128, 139–152. https://doi.org/10.1016/j.knosys.2017.05.001
- Ileberi, U., & Uzoka, F. M. (2022). A systematic review of machine learning techniques for credit card fraud detection. Journal of Big Data, 9(1), 1–26. https://doi.org/10.1186/s40537-022-00573-8
- Kumar, M., & Thenmozhi, M. (2006). Forecasting stock index movement: A comparison of support vector machines and random forest. Proceedings of the Indian Institute of Capital Markets 9th Capital Markets Conference Paper, India. https://doi.org/10.2139/ssrn.876544
- Liang, D., Tsai, C.-F., & Wu, H.-T. (2015). The effect of feature selection on financial distress prediction. Knowledge-Based Systems, 73, 289–297. https://doi.org/10.1016/j.knosys.2014.10.010
- Manimuthu, A., Venkatesh, V. G., Shi, Y., Sreedharan, V. R., & Koh, S. C. L. (2022). Design and development of automobile assembly model using federated artificial intelligence with smart contract. International Journal of Production Research, 60(1), 111–135. https://doi.org/10.1080/00207543.2021.1988750
- Ma, X., Sha, J., Wang, D., Yu, Y., Yang, Q., & Niu, X. (2018). Study on a prediction of P2P network loan default based on the machine learning LightGBM and XGboost algorithms according to different high dimensional data cleaning. Electronic Commerce Research and Applications, 31, 24–39. https://doi.org/10.1016/j.elerap.2018.08.002
- Naseer, I., Akram, S., Masood, T., Jaffar, A., Khan, M. A., & Mosavi, A. (2022). Performance analysis of state-of-the-art CNN architectures for LUNA16. Sensors, 22(12), 4426. https://doi.org/10.3390/s22124426
- Page, M. J., McKenzie, J. E., Bossuyt, P. M., Boutron, I., Hoffmann, T. C., & Mulrow, C. D. (2021). The PRISMA 2020 statement: An updated guideline for reporting systematic reviews. BMJ, 29(372). https://doi.org/10.1136/bmj.n71
- Paltrinieri, A., Hassan, M. K., Bahoo, S., & Khan, A. (2019). A bibliometric review of sukuk literature. International Review of Economics and Finance, 86, 897–918. https://doi.org/10.1016/j.iref.2019.04.004
- Pattnaik, D., Hassan, M. K., Kumar, S., & Paul, J. (2020). Trade credit research before and after the global financial crisis of 2008 – a bibliometric overview. Research in International Business and Finance, 54(May), 101287. https://doi.org/10.1016/j.ribaf.2020.101287
- Schebesch, K. B., & Stecking, R. (2005). Support vector machines for classifying and describing credit applicants: Detecting typical and critical regions. Journal of the Operational Research Society, 56(9), 1082–1088. https://doi.org/10.1057/palgrave.jors.2602023
- Sengupta, S., Basak, S., Saikia, P., Paul, S., Tsalavoutis, V., Atiah, F., Ravi, V., & Peters, A. (2020). A review of deep learning with special emphasis on architectures, applications and recent trends. Knowledge-Based Systems, 194, 105596. https://doi.org/10.1016/j.knosys.2020.105596
- Shengelia, N., Tsiklauri, Z., Rzepka, A., & Shengelia, R. (2022). The impact of financial technologies on digital transformation of accounting, audit and financial reporting. Economics, 105(3), 385–399. https://doi.org/10.36962/ecs105/3/2022-385
- Suryanarayana, S. V., Balaji, G. N., Rao, G. V., & others. (2018). Machine learning approaches for credit card fraud detection. International Journal of Engineering and Technology, 7(2), 917–920. https://doi.org/10.14419/ijet.v7i2.9356
- Tahamtan, I., Safipour Afshar, A., & Ahamdzadeh, K. (2016). Factors affecting number of citations: A comprehensive review of the literature. Scientometrics, 107(3), 1195–1225. https://doi.org/10.1007/s11192-016-1889-2
- Uthayakumar, J., Metawa, N., Shankar, K., & Lakshmanaprabu, S. K. (2020). Financial crisis prediction model using ant colony optimisation. International Journal of Information Management, 50, 538–556. https://doi.org/10.1016/j.ijinfomgt.2018.12.001
- Van Eck, N. J., & Waltman, L. (2010). Software survey: VOSviewer, a computer program for bibliometric mapping. Scientometrics, 84(2), 523–538. https://doi.org/10.1007/s11192-009-0146-3
- Van Eck, N. J., & Waltman, L. (2017). Citation-based clustering of publications using CitNetExplorer and VOSviewer. Scientometrics, 111(2), 1053–1070. https://doi.org/10.1007/s11192-017-2300-7
- Varma, P., Nijjer, S., Sood, K., Grima, S., & Rupeika-Apoga, R. (2022). Thematic analysis of financial technology (Fintech) influence on the banking industry. Risks, 10(10), 186. https://doi.org/10.3390/risks10100186
- Verma, S., & Gustafsson, A. (2020). Investigating the emerging COVID-19 research trends in the field of business and management: A bibliometric analysis approach. Journal of Business Research, 118(June), 253–261. https://doi.org/10.1016/j.jbusres.2020.06.057
- Zhu, Y., Zhou, L., Xie, C., Wang, G.-J., & Nguyen, T. V. (2019). Forecasting SMEs’ credit risk in supply chain finance with an enhanced hybrid ensemble machine learning approach. International Journal of Production Economics, 211, 22–33. https://doi.org/10.1016/j.ijpe.2019.01.032