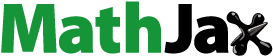
Abstract
With the rapid digital paradigm shift in educational settings, it is of utmost importance to comprehend the factors that influence the inclination of students towards utilising tablet-based learning. This study examined students’ intentions to use Tablet-based Learning (TBL) through an extended UTAUT2 model. Descriptive cross-sectional survey design was employed for the study. A questionnaire was utilised to collect data from 409 students in public senior high schools (SHSs) in the Kumasi Metropolis, Ghana. The novel variance-based structural equation modelling (PLS-SEM) was used to analyse the data. The study revealed that perceived learning opportunities, trust, effort expectancy, hedonic motivation, social influence and facilitating conditions had significant positive influence on students’ intention to use Tablet-based Learning. Also, the findings revealed that perceived learning opportunity and trust were the most external decisive factors for individual student’s intention with regards to Tablet-based Learning. Meanwhile, students’ attitude had a significant negative effect on their intention to use Tablet-based Learning. The study provides valuable insights for education policymakers and senior high school administrators regarding the essential factors that students require for effective implementation of Tablet-based learning systems.
1. Introduction
Reflections from the COVID-19 pandemic, which apparently impacted the teaching and learning process, have led to a notable rise in the number of digital learning (DL), offering diverse services and shaping the way knowledge and information are acquired. Numerous contemporary digital learning tools, such as mobile devices, smartboards, MOOCs, tablets, laptops, simulations, dynamic visualisations, and virtual laboratories, have gained significant traction within educational institutions (Haleem et al., Citation2022). This surge particularly occurred because traditional in-person education was abruptly forced to shift online in response to the COVID-19 pandemic (Bervell et al., Citation2022; Langthaler & Bazafkan, Citation2020; Shaikh & Nikooherafmaher, Citation2022).
Ghana has closely followed this global pattern. The evident importance of digital learning (DL) as the basis for inclusive education in Ghana, which has suddenly forced some institutions to shift online, is attributed to the impact of COVID-19 on traditional education (i.e., face-to-face) ([Addae et al., Citation2022]). Moreover, the history of the implementation of the internet and computer technology within Ghanaian schools can be traced back to the early 2000s with the enactment of the national ICT agenda (ICT4AD) and its corresponding legal instruments (Quaicoe & Pata, Citation2018). Additionally, the interim Strategic Plan 2018–2030 (Ministry of Education Ghana) emphasizes the essential role of utilizing ICT in educational institutions to attain the ultimate goal of providing high-quality education.
However, the essence of online learning has intensified after COVID-19 heavily disrupted traditional classroom teaching and learning (Addae et al., Citation2022). This has led to a digital paradigm shift in Ghana, with a number of digital learning platforms being introduced in schools and other academic institutions. For instance, seven out of the nine libraries in Ghana’s public universities have embarked on some sort of digitization initiative, and all the studied libraries have used the same digital asset management system (Dzandza, Citation2020). In addition, some tertiary and pre-tertiary institutions in Ghana integrated digital learning platforms into their systems to deliver live and interactive teaching (Edem-Adzovie & Jibril, Citation2022).
With the increasing importance given to digitalization nowadays, countries around the world are shifting their focus and efforts to changing the previous traditional education practice that has been ineffective. Ghana, following in the footsteps of the rest of the world, has set out to introducing a Tablet-based Learning (TBL) SHS. The Vice President of Ghana recently declared at the 60th Anniversary celebration of the Hohoe Evangelical Presbyterian Senior High School (SHS) declared the government’s plans to install textbooks and other teaching and learning resources on a digital platform for students in Senior High Schools (SHS). The Ministry of Education (MoE) has also confirmed that they are putting plans in place to procure tablets for every student currently enrolled in the SHS. Therefore, the digital paradigm shift in Ghana is likely to take the form of Tablet-based Learning (TBL) in Senior High School (SHS).
This is per the Vision of the Strategic Plan 2018–2030 (Ministry of Education[MoE], Citation2018a), which centers on the aspiration to enhance the standard of education accessible to all individuals in Ghana. The current trajectory has favorable economic implications, as prior research has established that digitalization presents significant prospects for economic advancement and tackling societal predicaments, such as poverty, inequality, and exclusion (Langthaler & Bazafkan, Citation2020). Additionally, Brossard et al. (Citation2021) assert that providing access to these digital technologies to all children has the potential to generate societies that are more inclusive and prosperous. Ahmad (Citation2016) demonstrated the facilitation of independent learning and authentic communication using technology-assisted learning (TAL), encompassing computer-assisted learning (CAL) and mobile-assisted learning (MAL).
Furthermore, a recent scholarly investigation conducted by Songkram et al. (Citation2023) highlighted the role of digital learning platforms in fostering the acquisition of skills, values, and knowledge necessary for individuals to effectively navigate the present and forthcoming digital landscape. In particular, Previous studies have demonstrated the promising potentials of tablets in particular for enhancing the learning process for students of various ages and needs (Baba-Nouri & Cerratto-Pargman, Citation2016; Yidana et al., Citation2023; Zhang & Nouri, Citation2018). For example, Nouri and Cerratto-Pargman (Citation2016) showed how tablets enhanced teaching and learning practices in Swedish schools by virtue of three affordances, including the persistence of the digital medium, the multimodality of the material, and portability and ubiquity. Nouri and Cerratto-Pargman (Citation2016) also found that tablets can enhance six themes of learning and teaching practices, including multimodal learning, assessment and formative feedback, communication, documentation, and mobile learning.
Other studies (Batsila et al., Citation2017; Furió et al., Citation2015; Hamzah et al., Citation2020) have shown how mobile-assisted learning tools enhance learning, increase motivation, and promote knowledge acquisition. Additionally, tablets are commonly utilised by students to engage in online educational activities, interacting with instructors, accessing informational resources, perusing electronic books, recording notes, and completing assignments (Songkram et al., Citation2023). Therefore, it is evident that the implementation of tablets will effectively address the digital divide among students in secondary high schools (SHS) and enhance their ability to fulfil present and future digital standards. Hence, enhancing and advocating for the utilisation of tablets among secondary school students as educational instruments holds significant importance.
However, the promising effects of using tablet computers at the senior high school (SHS) level may not be maximal. Many unsuccessful implementations can be attributed to factors such as students’ intention to use the devices. This is because the utilisation of these learning systems plays a crucial role in determining their success, whereas inadequate usage hinders the realization of their advantages, resulting in an unsuccessful innovative learning system (Sharma et al., Citation2017). As previously established, successful implementation of digital learning systems depends heavily on both instructors and students’ intention and use behaviours (Al-Adwan et al., Citation2021; Bhimasta & Suprapto, Citation2016; Sharma et al., Citation2017). Thus, the difficulty in integrating any form of technology does not originate from the technology itself but rather from the perspective of the user behavioural intentions.
These assumptions hold in Ghana within the context of SHS students’ intentions to use tablet-based learning (TBL). Although, the government of Ghana, through the Vice President, recently announced its plans to provide free tablet computers to all senior high school students to support teaching and learning, there are concerns about whether students will use tablets for this purpose. A recent study by Yidana et al. (Citation2023) maintained that understanding the intention of students to use technology tools for learning purposes has become a crucial aspect of education studies, given the erratic internet challenges and unpredictable students attitudes. Thus, the potential benefits of SHS students’ use of tablet-based learning may not be fully realised due to a significant challenge in effectively integrating new technology. It is necessary to understand the perceptions and factors that influence the use of tablet computers by SHS students.
There is a need to be aware of factors that influence behavioural intentions toward technology acceptance to achieve strategic plans of offering more flexible learning opportunities in Senior High Schools, especially given that information on this matter is still limited. Nevertheless, studies on factors influencing students’ intentions to use Tablet-based Learning (TBL) have received little attention. In Ghana, previous studies have examined students’ perspectives on the use of technology, but failed to focus on Tablet-based Learning (TBL). Arthur and Kaku (Citation2020) conducted a study to ascertain students’ perceptions of technology use in teaching economics in selected Senior High Schools in the Central Region of Ghana, but they did not focus on the use of TBL. In addition, a recent study by Yidana et al. (Citation2023) examined predictors of Economics students’ behavioural intention to use tablets in the Cape Coast Metropolis.
However, this study did not extend the UTAUT2 model and focused only on Economics students. Since, other factors such as context and subject, may influence students’ intention to use tablet-based learning, it is imperative to augment the literature on tablets use. Aheto-Domi et al. (Citation2020) also examined readiness for the use of virtualor digital learning classrooms in the Colleges of Education in Ghana, which did not specifically focus on SHS or the use of TBL. However, while the use of tablets among SHS students is limited in Ghana, an understanding of the factors influencing students’ behavioural intention to use TBL remains elusive, as reflected in theoretical and methodological examinations. From a theoretical perspective, UTAUT2 is identified as a comprehensive model that includes key psychosocial components to unravel the intentions to use any technology.
According to the literature, UTAUT2 is currently the most comprehensive research model in the Information Systems (IS) field for understanding various predictors influencing individuals to accept and make use of information technologies (Roberts et al., Citation2021; Tamilmani et al., Citation2018; Tamilmani et al., Citation2021). According to Tamilmani et al. (Citation2021), despite the introduction of UTAUT2 in 2012, it has already garnered more than 6000 citations in Google Scholar alone, spanning from the IS field and beyond, emphasizing its predictive ability to explain 74% of the variance in behavioural intentions. The use of UTAUT2 in predicting students’ behavioural intention to use Tablet-based learning is rare in the literature. For instance, Zheng and Li (Citation2020) examined students’ intention to use tablet, utilising TAM instead of UATUT2.
Fernandez and Mammen (Citation2020) explored the perceptions of students and lecturers regarding the use of tablets for learning at a rural university in the Eastern Cape state of South Africa. However, the study was not guided by UTAUT2 or any psychosocial model. Additionally, Fernandez and Mammen (Citation2020) employed descriptive analysis, instead of robust statistical tools such as structural equation modeling (SEM). Meanwhile, it is recommended to use SEM, as it can explore complex relationships among variables (Hair et al., Citation2022). Recent studies such as those by Albahri et al. (Citation2022), Hair et al. (Citation2020), and Owolabi et al. (Citation2020), have shown that SEM can determine relationships among unobserved constructs, including independent, mediator, moderator, control, and dependent variables. Also, Feng et al. (Citation2021) conducted a two model-based meta-analytic review on the determinants of technology acceptance, however, their study did not focus on UTAUT2.
Furthermore, Hamzah et al. (Citation2020) examined the significant factors that influence students’ behavioural intention to use tablets in Malaysia. This study was guided by the original UTAUT, which consists of only four variables: performance expectancy, effort expectancy, social influence, and facilitating conditions. It did not utilise UTAUT2, which extends the original UTAUT with three additional key factors: habit, hedonic motivation, and price value (Venkatesh et al., Citation2012). Although, Wang et al. (Citation2022) predicted factors that relate to the intention and actual usage of tablet computers by university students in urban and rural areas were based on the Unified Theory of Acceptance and Use of Technology (UTAUT2), this study did not focus on Senior High Schools and was conducted in China.
To elaborate further, other psychosocial factors such as trust and attitude influence individual behavioural intentions in the context of technology adoption. For instance, previous studies by Hooda et al. (Citation2022) and Zhang et al. (Citation2020) showed that trust plays a central role in users’ intention to use and the use of digital systems. Other studies (e.g., Dumford & Miller, Citation2018; Laukkanen, Citation2016; Streletskaya et al., Citation2020) have also revealed a significant relationship between attitude and the behavioural intention to use online education systems.
Moreover, while the significant role of tablet in supporting learning is widely recognised, it remains uncertain the extent to which perceived learning opportunity influence SHS students’ intention to used TBL. This is because most students who have grown up using mobile devices, including tablets, may view these tools primarily as tools for entertainment rather than for academic purposes. Therefore, perceived learning opportunity could be a key factor influencing SHS students’ decision-making in the context of TBL adoption. We argue that if an individual SHS student perceive the use of TBL to be associated with high learning opportunities, he/she is more likely to have high positive intention toward its use. Additionally, if students trust the TBL system to be consistent, they will be more willing to explore its features and maximize its benefits.
In other words, if students trust the TBL system, they are more likely to perceive it as a valuable learning tool and find it easy to navigate and interact with. Moreover, when students develop positive attitudes that foster a favourable disposition toward technology in general, they are more willing to adopt and explore digital tools and platforms such as TBL. However, it appears no study in Ghana has thoroughly considered the concept of trust, attitude, and perceived learning opportunity to outline the full spectrum of understanding students’ behavioural intention toward the use of TBL. Yidana et al. (Citation2023) employed the UTAUT2 model to examine Economics students’ behavioural intention to use tablets for learning in the Cape Coast Metropolis of Ghana. However, they did not consider trust, attitude, and perceived learning opportunity as other key socio-psychological perspectives that can affect an individual’s intention and use behaviours of the TBL. This knowledge gap highlights the importance of this study.
To elaborate further, the strength of this study is the decision to use structural equation modelling (SEM) approach as an estimation method to predict the multiple factors affecting the adoption of TBL among SHS students in Ghana. By employing the SEM approach as rigorous estimation method to help establish the multiple predictors of TBL, policymakers and educators can design targeted interventions to promote its adoption, ensuring equitable access to quality education and empower students for success in the digital age. Therefore, this study incorporates trust, attitudes, and perceived learning opportunity into the UTAUT2 model and employs the SEM approach as an estimation method to unravel the factors influencing behavioural intentions to use TBL among SHS students in the Kumasi Metropolis, Ghana.
The Kumasi Metropolis is one of the largest and most urbanized areas in Ghana with a diverse range of senior high schools. Kumasi Metropolis representation is important because TBL initiatives are more likely to be initially implemented in urban areas where internet infrastructure is better developed. Therefore, sampling SHS in the metropolis provides a strategic starting point for understanding SHS students’ intentions to embrace TBL in Ghana. While focusing on a specific region like Kumasi Metropolis, the findings of the study can still offer valuable insights into the broader Ghanaian context, especially as TBL initiatives expand across the country. As such, the findings of the study are expected to be generalised to assist policymakers in Ghana as a whole and international agencies to formulate policies and programs towards the adoption of learning technologies, including the TBL, to promote quality and inclusive education.
The subsequent section delineates the theoretical underpinnings of this study. The third section addresses the formulation of the hypotheses for the investigation. The methodologies utilised in this study are explained in the fourth section. Subsequently, the findings are presented in the fifth section, while a discussion of the results that emerged from the fieldwork is encapsulated in the sixth section. In the penultimate section, deductions are drawn and recommendations are made based on the findings of the study. The study culminates with an identification of the investigation’s constraints and propositions for future scholarly endeavours in the domain of digital learning.
2. Theoretical review
Over the past few decades, scholars have shown a significant interest in understanding the acceptance and use of technology, making it a prominent and extensively studied field. Consequently, several theoretical frameworks have been developed to explain the behaviour and determining factors behind technology usage. These frameworks include the Innovation Diffusion Theory (IDT) (Rogers, Citation1962), the Technology Acceptance Model (TAM) (Davis, Citation1989), the Theory of Reasoned Action (TRA) (Fishbein & Ajzen, Citation1975), the Social Cognitive Theory (SCT) (Bandura, Citation1989), the Theory of Planned Behaviour (TPB), the Model of PC Utilisation (MPCU) (Thompson et al., Citation1991), the Motivational Model (MM) (Davis et al., Citation1992), and the Combined TAM and TPB (Taylor & Todd, Citation1995).
Nevertheless, in 2003, Venkatesh, Morris, Davis, and Davis found that most of these frameworks possessed inadequate predictive capabilities and were constructed based on insufficient empirical evidence. They then proposed the unified theory of acceptance and use of technology (UTAUT) . UTAUT2 was later proposed by Venkatesh et al. (Citation2012) as a model for comprehending consumer behaviour when using technologies. Thus, Venkatesh et al. (Citation2012)modified some of the existing relationships in the UTAUT model by introducing new relationships, including three new constructs: hedonic motivation, price values, and habit. Apart from the fact that UTAUT2 is an extension of UTAUT, it integrates the eight established technology acceptance models mentioned above.
UTAUT2 leverages the strengths of these models and considers a broad range of factors that impact technology adoption and usage. UTAUT2 encompasses a comprehensive set of factors that influence the intention to use technology, thus providing a more thorough understanding. Hence, this study proposes a context-specific model of students’ behavioural factors towards tablet-based learning (TBL), based on the extended UTAUT2 model. The proposed model aims to uncover the factors that drive students’ intention to use the TBL system, integrating core constructs such as performance expectancy, effort expectancy, social influence, facilitating conditions, habit, hedonic motivation, and price value (Venkatesh et al., Citation2012).
These factors offer a holistic perspective on user behaviour and multiple influences on technology adoption intention (Tamilmani et al., Citation2019). More importantly, UTAUT2 acknowledges that user behaviour can vary across different contexts and user characteristics such as age, gender, and experience. Hence, these variables were considered moderating variables. UTAUT2 has demonstrated superior predictive power compared to its predecessor models. This explains the higher percentage of variance in the behavioural intention to use technology, making it a more reliable framework for understanding user behaviour (Tamilmani et al., Citation2019).
UTAUT2 has undergone rigorous empirical validation across different domains and technologies, which strengthens its credibility and generalizability. The framework has been extensively tested and refined through empirical studies, demonstrating its applicability and effectiveness in explaining technology acceptance and usage behaviours. A wide range of studies have used UTAUT2 as a framework to investigate learners’ continuance intention to use digital learning platforms, such as massive online open courses (MOOCs) (Fianu et al., Citation2018; Meet et al., Citation2022; Mohan et al., Citation2020), e-learning systems (Al-Adwan et al., Citation2021; Ansong-Gyimah, Citation2020; Osei et al., Citation2022), mobile learning (Ameri et al., Citation2020; Kumar & Bervell, Citation2019), social media (Huang, Citation2018; Tarhini et al., Citation2021), e-books (Bhimasta & Suprapto, Citation2016; Gunawan et al., Citation2019), and online courses (Amid & Din, Citation2021; Goto & Munyai, Citation2022; Kosiba et al., Citation2022).
However, studies have rarely utilised UTAUT2 to examine how its constructs affect students’ intentions to use tablet-based learning. In addition, previous studies such as Bhimasta and Suprapto (Citation2016) have established that incorporating other variables into UTAUT2 is necessary to accurately predict the factors that influence behavioural intention to adopt a tablet for learning. Therefore, the objective of this study was to develop a purpose model by extending UTAUT2 as a theoretical foundation with trust, attitude, and perceived learning opportunity to examine students’ behavioural intention to use tablets as learning tools. Consequently, the original UTAUT2 model was modified to include trust, attitude, and perceived learning opportunity.
Previous studies have demonstrated the significant roles of these factors in predicting both the intention to use and the actual use of new technologies. Trust in this context refers to students’ perceptions of the system’s reliability and trustworthiness, which are crucial factors in predicting their intention to adopt and use the system (Alalwan et al., Citation2017; Arpaci, Citation2016; Chao, Citation2019). Based on previous research conducted by Chiu et al. (Citation2017) and Hameed et al. (Citation2022), trust plays a significant role in determining the behavioural intention to use mobile learning applications. Additionally, studies have indicated that attitude, which encompasses subjective assessments, perspectives, and behavioural patterns, is a vital factor in determining students’ intention to use technology (Hussein, Citation2017, Nisa & Solekah, Citation2022; Songkram et al., Citation2023; Svenningsson et al., Citation2021).
Furthermore, we argue that perceived learning opportunity increases the intention to adopt technology. However, research regarding the impact of students’ perception of learning opportunities on their decision-making concerning the use of technology is scarce in the literature. This gap in the existing literature emphasizes the significance of the present study, as its outcomes would offer valuable insights into students’ essential requirements and the critical aspects involved in incorporating educational tools such as tablet-based learning into their academic pursuits.
In summary, trust, attitude, and perceived learning opportunity are external variables that play a role in UTAUT2 model constructs, such as performance expectancy, effort expectancy, social influence, facilitating conditions, habit, and hedonic motivation. These factors directly impact students’ behavioural intention. Additionally, the study also investigated the influence of gender as a moderator in the relationship between habit and behavioural intention, as well as hedonic motivation and behavioural intention.
However, it is worth noting that the price value, which may be defined as the costs incurred by SHS students to buy tablet devices, tools, or internet packages used for learning, was not considered. This study conceptualized price value as zero costs incurred by SHS students to buy tablet as learning tools, since the government procures them. In addition, there was no relationship between price value and user intention to use tablet-based learning tools. Figure displays the UTAUT2 model propounded by Venkatesh et al. (Citation2012). Also, Figure shows the proposed framework from the extended UTAUT2 model, as well as the initial hypothesis of the relationship between variables. The following section explains the proposed hypotheses of the research model.
3. Hypotheses framing
3.1. Behavioural intentions (dependent variable)
The motivation of individuals plays a pivotal role in determining decisions to use technology, and this defines behavioural intention. Behavioural intention refers to a person’s readiness to use a particular technology for various tasks (Moorthy et al., Citation2019). According to Davis, behavioural intention evaluates the strength of a user’s commitment to perform a specific behaviour and shows the intensity of an individual’s intention to adopt a specific behaviour. Fishbein and Ajzen (Citation1975) also posited that behavioural intention is reflected as a signal of actual behaviour. Studies have widely used this construct as an antecedent of user acceptance in various technology acceptance studies which showed that it predicts actual usage of technology (Moorthy et al., Citation2019). Behavioural intention is the outcome variable conceptualised as depending on or shaped by various psychosocial factors included in this study, including performance expectancy, effort expectancy, social influence, habit, attitude, facilitating conditions, hedonic motivation, and perceived learning opportunities.
3.2. Attitude
Attitude can be influenced by various factors that act as stimuli, including the perception of usefulness, ease of use, technology self-efficacy, subjective norms, and facilitating conditions. While not a primary component in the UTAUT2 model, its relationship with behavioural intention is supported by theoretical frameworks such as the Theory of Reasoned Action (TRA) (Fishbein & Ajzen, Citation1975) and the Theory of Planned Behaviour (TPB). In line with these theories, past studies conducted by Hussein (Citation2017), Nisa and Solekah (Citation2022), Songkram et al. (Citation2023), and Svenningsson et al. (Citation2021) have concluded that attitude exerts positive influence on behavioural intention to use any new technology. In this study, we defined attitude as SHS students’ perception of tablet-based learning, characterised by dimensions such as positive versus negative, enjoyable versus unpleasant, ease of use versus unease of use. Thus, students are likely to develop a positive attitude after experiencing that a particular technology platform is a user-friendly, easy to use which consequently fosters their continued willingness to use it. This suggests that SHS students with positive attitudes are more likely to have high behavioural intentions towards their usage than those with negative attitudes. Thus, we proposed the following hypothesis:
H1:
Attitude significantly influences SHS students’ behavioural intention to use tablet-based learning tools.
3.3. Effort expectancy
Effort expectancy in UTAUT2, also known as perceived ease of use in the TAM model, pertains to how users perceive the ease of learning and operating new technology. The concept of effort expectancy revolves around the ease with which individuals adopt technology (Arenas-Gaitan et al., Citation2015). Previous research conducted by Al-Adwan et al. (Citation2022). Hamzah et al. (Citation2020), Meet et al. (Citation2022), Twum et al. (Citation2022), Wang et al. (Citation2022) indicated a significant impact of effort expectancy on behavioural intention. Conversely, users tend to be reluctant to adopt towards new technology when it difficult to operate. From the description of effort expectancy and how it can affect students’ behavioural intentions to use technology, this study defines it as the perceived level of effortlessness that a student associates with using tablet-based learning. Thus, effort expectancy in the context of this study, is defined as the belief held by SHS students, that the tablets are user-friendly learning tools that require minimal effort to operate. Drawing inspiration from Wang et al. (Citation2022) and Yidana et al. (Citation2023) who established that there is a significant association between the students’ perception of new technology in terms of ease of use (i.e., effort expectancy) and the behavioural to use, the following hypothesis is put forward:
H2:
Effort expectancy significantly influences SHS students’ intention to use tablet-based learning tools.
3.4. Facilitating conditions
Facilitating conditions, according to research conducted by Venkatesh et al. (Citation2012), pertain to the level of trust an individual has in the organization and the existing technical infrastructure to effectively support the utilisation of a technological system. Previous research has consistently indicated a relationship between facilitating conditions and the behavioural intentions of students regarding the adoption of digital learning tools (Alshammari, Citation2021; Khrais & Alghamdi, Citation2021; Mubuke et al., Citation2017; Yidana et al., Citation2023; Youssef & Issam, Citation2022). This study operationalised facilitating conditions by evaluating the perception of students in Senior High Schools (SHSs) regarding the availability of adequate support for using tablets as learning tools. If students perceive that the necessary conditions are in place to support tablet usage, they are more likely to view tablets as valuable tools that can enhance their learning experiences. This positive perception can, in turn, influence their behavioural intentions to actively incorporate tablets into their learning routines. Hence, we hypothesised that:
H3:
Facilitating conditions significantly influences SHS students’ intention to use tablet-based learning tools.
3.5. Habit
Habit as per Venkatesh et al. (Citation2012), refers to individuals’ intention to perform actions automatically in the learning process. The habit can be defined as the extent to which an individual engages in automatic behaviour because of previous learning. This reflects the effects of previous experiences with tablet or mobile learning device usage. Previous studies conducted by Cai et al. (Citation2021), Meet et al. (Citation2022), Tamilmani et al. (Citation2019), Utomo et al. (Citation2021) have consistently demonstrated that habit exerts a positive influence on behavioural intention. In the specific context of this study, SHS students who exhibit a higher tendency to use a mobile phone for learning automatically are more likely to possess a stronger intention to engage in tablet compared to those with a lower level of automaticity. In this study, it reflects the effects of previous experiences with a tablet or a mobile learning device usage. This study suggests that habit plays a role in predicting students’ behavioural intentions to use a tablet for learning. However, gender as a moderator affects habit it may influence behavioural intention to use technology as claimed by previous studies (Ameen & Willis, Citation2019; Moon, Citation2021) like tablets for educational learning purposes. Hence, the following hypothesis is put forward:
H4a:
Habit significantly influences SHS students’ behavioural intention to use tablet-based learning tools.
H4b:
Gender moderates the direct relationship between habit and behavioural intention towards the use tablet-based learning tools.
3.6. Hedonic motivation
According to the UTAUT2 model, hedonic motivation refers to the pleasure derived from using technology (Venkatesh et al., Citation2012). In line with the motivational model (MM) developed by Davis, Bagozzi, and Warshaw (Citation1992), hedonic motivation plays a crucial role in influencing users’ acceptance of technology (Moorthy et al., Citation2019). Several studies have provided evidence of a positive connection between hedonic motivation and behavioural intention (Alalwan et al., Citation2017; Ayasrah, Citation2020; Hartelina et al., Citation2021; Moorthy et al., Citation2019; Twum et al., Citation2022; Yidana et al., Citation2023; Zacharis & Nikolopoulou, Citation2022). In the context of this study, hedonic motivation is conceptualised as the pleasure and enjoyment attached to the use of tablet-based learning from the perspective of students in SHS. This means that students will be more motivated to engage with tablet-based learning and actively participate in its use if they perceive it to be highly enjoyable and pleasurable. However, it is worth noting that specific gender characteristics can influence the relationship between hedonic motivation and behavioural intention to use tablet-based learning among SHS students. This is because the literature suggests that men and women possess diverse perceptions regarding information technologies such as mobile phones and computers, where males tend to have higher technology acceptance levels compared to females (Liu & Guo, Citation2017; Tamilmani et al., Citation2019; Tarhini et al., Citation2017). Similarly, understanding the moderating effect of gender can inform the development of tailored educational interventions and strategies to promote tablet-based learning adoption. Therefore, the following hypotheses were formed:
H5a:
Hedonic motivation significantly influences SHS students’ behavioural intention to use tablet-based learning tools.
H5b:
Gender moderates the relationship between hedonic motivation and behavioural intention towards the use tablet-based learning tools.
3.7. Perceived learning opportunity
An environment or system with many learning opportunities is likely to improve the development of skills, employability, and an individual’s knowledge (Van der Sluis & Poell, Citation2003). Learning opportunities can manifest in diverse ways, such as experiences from peers, course participation, and taking up challenging tasks. According to previous studies, perceived learning opportunities are considered an important variable because the more often individuals perceive learning opportunities in technology usage for professional development and employability, the more likely they are to undertake activities such as acquiring new knowledge and developing new skills (Boomaars et al., Citation2018; Dutton, Citation2018; Steil et al., Citation2020). Perceived learning opportunity in this study refers to the extent to which students in SHS perceive learning opportunities provided by the use of tablets of learning. We argue that perceived learning opportunities can influence students’ behavioural intention to use tablet-based learning. If they believe that this technology offers them meaningful learning experiences, they are more likely to accept and adopt it as a regular part of their educational journey. Hence, the researchers’ hypothesised that:
H6:
Perceived learning opportunities significantly influence SHS students’ behavioural intention to use tablet-based learning tools.
3.8. Performance expectancy
Performance expectancy is a key construct in the UTAUT2 that refers to the extent of advantages that are expected to be delivered to individuals through the adoption of a particular technology (Moorthy et al., Citation2019; Venkatesh et al., Citation2012). In other words, performance expectancy is how users believe that using new technology helps them improve their performance in daily activities. According to Venkatesh et al. (Citation2012, performance is the strongest predictor of new technology. Previous studies have showed that performance expectancy is the strongest predictor of students’ behavioural intention to use new technology (Amid & Din, Citation2021; Wang et al., Citation2022). This study defined performance as SHS students’ belief that using a tablet as a learning tool improves their learning outcomes. Hypothesizing the relationship between performance expectancy and behavioural intentions would provide insights into the factors that influence students’ acceptance and intentions to use tablets for learning purposes, which can inform educational strategies, instructional design, and technology integration in schools. To verify the relationship between performance expectancy and SHS students’ intentions to use tablet-based learning technology, the hypothesis was raised:
H7:
Performance expectancy significantly influences SHS students’ behavioural intention to use tablet-based learning tools.
4. Social influence
Social influence refers to the impact of other people’s perceptions and the surrounding environment on an individuals’ utilisation of new technologies. These individuals and environmental factors may include government and regional initiatives, school policies, and the opinions of friends, teachers, students, and parents. Social influence as a psychosocial construct in UTAUT2 is considered particularly important during the initial phase of accepting technology (Wang et al., Citation2022). The impact of social influence on behavioural intention has been confirmed by several previous studies including Bervell et al. (Citation2022); Leow et al. (Citation2021); Meet et al. (Citation2022); Nassar et al. (Citation2019) and Yidana et al. (Citation2023). Consequently, this study defines social influence as the adoption of tablets as learning tools by SHS students due to the influence of significant others’ perceptions and environmental factors. In other words, it is the extent to which SHS students acknowledge the expectations of important individuals, including peers, teachers, and family members, regarding their adoption of mobile learning systems. Thus, this study proposes the following hypothesis:
H8:
Social influence significantly influences SHS students’ behavioural intention to use tablet-based learning tools.
4.1. Trust
Trust is the belief that an individual has towards people (or objects) regarding their ability, reliability, and truthfulness (Roberts et al., Citation2021). Trust in the context of technology is a belief about not only the technology, but also all the stakeholders involved (e.g., the developers, managers involved in adopting new technology, leadership). According to Songkram et al. (Citation2023), attitude encompasses subjective assessments, perspectives, and behavioural patterns towards technology adoption. Although, Kabra et al. (Citation2017) revealed no significant effect of trust on the behavioural intention to use technology, others have confirmed that trust is a critical factor determining behavioural intention to use technology (Alalwan et al., Citation2017; Chao, Citation2019; Khalilzadeh et al., Citation2017). For instance, Chao (Citation2019), empirically tested a model to predict the factors affecting students’ behavioural intentions toward mobile learning (m-learning) and the results revealed that behavioural intention was significantly influenced by trust. Based on this, we proposed that SHS students’ trust levels positively influence their behavioural intention to use tablet-based learning. Based on this discussion, the following hypothesis was formulated:
H9:
Trust significantly influences SHS students’ behaviour and intention to use tablet-based learning tools.
5. Conceptual framework
In this section, we introduce the conceptual framework of the study, which is developed based on the research hypotheses formulated to guide the research. Figure shows the proposed conceptual framework of the study.
6. Methods
6.1. Research design and sampling technique
In this study, we used a quantitative methodology incorporating a cross-sectional survey (Fraenkel et al., Citation2018). This approach was selected because of its reputation in yielding dependable, accurate, and broadly applicable results (Fraenkel et al., Citation2018). A descriptive cross-sectional survey design was used to examine senior high school (SHS) students’ intentions to embrace tablet-based learning in Ghana. The decision to employ this design was guided by Dillman et al. (Citation2014) viewpoint that it is well-suited for collecting information and data regarding respondents’ attitudes and perceptions of specific phenomena. Additionally, a cross-sectional survey design was chosen for this study because the aim was to examine the existing situation on the ground without manipulating the variables involved (Saunders et al., Citation2009; Yidana & Arthur, Citation2023; Yidana et al., Citation2022, Citation2023).
The study population of comprised all students in twenty-seven public SHSs in the Kumasi Metropolis. A simple random sampling technique was used to select twenty SHSs and the SHS students. Simple random sampling is a commonly used technique for selecting a representative sample from a larger population. This involves the random selection of individuals from the population, giving each member an equal chance of being included in the sample (Ghauri et al., Citation2020). Additionally, it ensures that every member of the population has an equal chance of being selected, reducing the likelihood of bias and allowing for the generalization of findings to the entire population (Polit & Beck, Citation2017).
Furthermore, becuase of the large number of senior high school students in the Kumasi Metropolis, no sampling frame was available. The survey was distributed at twenty (20) public senior high schools (SHSs) in the Kumasi Metropolis of Ghana. The minimum sample size was determined using the G*Power statistical program. Power analysis is a crucial technique employed to determine the minimum sample size needed to evaluate a research model with a considerable number of predictors (Hair et al., Citation2016). The number of predictors was 12, after the information was computed into G*Power, the minimum sample size required for the model was 127. The result shows a minimum sample size of 127 at a 0.15 effect size (medium effect), a power of 0.8, and a 0.05 alpha (Memon et al., Citation2020). However, the sample size was increased to 500. In recent times, a plethora of academic literature has been published, with notable contributions by Memon et al. (Citation2020), Giebelhausen et al. (Citation2020), Cheah et al. (Citation2019), and Awang et al. (Citation2019), among others. These studies employed G*Power, a widely utilised software, for the sample size estimation.
6.2. Research instrument
All measurements used in this study were adapted from existing literature, and each construct was considered to be unidimensional. The respondents’ responses were measured using a five-point Likert scale. Before distributing the survey, a pre-testing phase was conducted. Five academics and two professors were invited to participate in the content validity test. Based on their feedback regarding the representativeness and clarity of constructs and questions, modifications were made to the survey. Subsequently, a pilot test was conducted immediately after the content validity test. For the pilot test, a group of 65 senior high school (SHS) students was selected, following the guidelines outlined by Baker (Citation1994). The objective of the pilot test was to evaluate the internal consistency of each construct. Baker recommended that the pilot test should include 10–20% of the total sample size anticipated for the actual study. The results revealed that the Cronbach’s Alpha values for all constructs exceeded 0.7.
Data from SHS students were collected using a questionnaire designed based on the instrument developed by Venkatesh et al. (Citation2003, Citation2012), who developed the UTAUT and UTAUT2 model. The questionnaire consisted of 40 items representing ten (10) variables. The variables were assessed using a five-point Likert scale, following relevant studies by Alalwan et al. (Citation2017), Kurt & Tingoy (Citation2017), Steil et al. (Citation2020) and Venkatesh et al. (Citation2012). The scale encompassed constructs such as attitude (four items), behavioural intentions (four items), effort expectancy (five items), facilitating conditions (four items), habit (three items), hedonic motivation (three items), performance expectancy (four items), perceived learning opportunities (four items), social influence (four items), and trust (five items).
6.3. Procedure for data collection
Before commencing data collection, a comprehensive training program was conducted for six research assistants. The training focused on ensuring a clear understanding of the scale items and proper administration of the questionnaire. Moreover, the research assistants received elaborate instructions regarding all facets of the instrument and were instructed on the principles of research ethics. Each research assistant was allocated to distinct SHSs for the purpose of data collection. The research assistants visited all the sampled schools and administered the questionnaire, allowing students 30 to 40 minutes to respond. Out of the total 500 questionnaires administered across the twenty selected senior high schools, a total of 409 completed questionnaires were collected. This yielded a questionnaire return rate of 81.8%. Following collection, each completed questionnaire was promptly reviewed to ensure absolute completeness.
6.4. Data processing and analysis
Initially, the data underwent a filtering process to eliminate irrelevant responses and was subsequently cleaned to ensure completeness. Following this, the data was coded and inputted into Statistical Product for Service Solution (SPSS) version 26 for further processing. Subsequently, the data from the SPSS software was converted into a CSV comma delimited file to facilitate its utilisation in the Smart-PLS 3 software. The data that were gathered underwent analysis through the utilisation of Partial Least Squares Structural Equation Modelling (PLS-SEM) with the aim of examining the proposed hypotheses in the research model. PLS-SEM is widely recognised as an effective approach for estimating complex models which encompass numerous relationships, latent constructs and/or indicators as well as advanced analyses. Such recognition is supported by various scholars (Akter et al., Citation2017; Hair et al., Citation2018, Citation2019; Matthews, Citation2017). Accordingly, PLS-SEM analysis was performed using Smart-PLS 3.0 software (version 3.2.9) ([Ringle et al., Citation2015]). This study adopts the two-phase data analysis methodology of Hair et al. (Citation2019). The first phase was performed to assess the measurement model by testing the internal consistency reliability, convergent validity, and discriminant validity, and the second phase was established to examine the structural model and the proposed hypotheses. Hair et al. (Citation2014) outlined a set of recommended procedures for evaluating the structural model. These procedures include the assessment of collinearity, examination of path coefficients (β), evaluation of coefficient of determination (R-square) and effect size (f-square), and assessment of predictive relevance (Q-square).
6.5. Ethical consideration
The study adhered to the necessary ethical procedures, considering the active involvement of human participants. These procedures encompassed obtaining informed consent, upholding human rights, mitigating potential risks, and upholding professional integrity, as outlined by Appiah (Citation2022). Prior to data collection, all participants were provided with comprehensive information regarding the study’s objectives. Furthermore, they were explicitly informed of their autonomy to withdraw from the study at any point. To assuage concerns, participants were reassured that the research posed no direct threats or psychological harm. Additionally, their responses were treated with utmost confidentiality, ensuring anonymity to safeguard their identities.
7. Results
7.1. Descriptive statistics
The survey results showed that more than half (n = 245, 59.9%) of the respondents were males, representing majority. While 164 (40.1%) of the respondents were females. The average age of the respondents was = 17 (
= 1.36).
7.2. Common method bias
Data collected from individuals within the same time period can be susceptible to common method bias (CMB), potentially influencing study results (Podsakoff et al., Citation2003). To address this potential bias, the study design was meticulously developed to minimize ambiguity and confusion in the survey questions. The goal was to create straightforward, concise, and easily understandable items. Additionally, measures were taken to ensure respondent privacy and encourage honest responses. An exploratory factor analysis (EFA) known as “Harman’s single-factor test” was utilised to examine all the measurement items. Consequently, the measurement items were factorized into a single factor through EFA. The analysis revealed that the items could be categorized into ten factors, with the first factor accounting for 38.59% of the total variation. This finding suggests that CMB was not a significant concern as the observed variation was below the 50% threshold recommended by Podsakoff et al. (Citation2003). Therefore, it can be concluded that CMB did not pose a problematic issue in this study.
7.3. Measurement model
Hair et al. (Citation2019) advocated for conducting four evaluations of measurement models in PLS-SEM, which encompassed examining indicator loadings, consistency reliability, convergent validity and discriminant validity.
7.4. Reflective indicator loadings
According to Hair et al. (Citation2019), the reflective indicator loadings obtained in PLS-SEM should be equal or above 0.60. After performing the calculations, it was determined that all the loadings surpassed 0.60. For instance, the highest loading, indicated by PLO, was observed in PLO3 (0.906), whereas the lowest loading was achieved by T (T5 = 0.684). A total of 40 indicators were used for the subsequent data analysis (see Table ).
Table 1. Constructs, items, IL, CA (α), rho_A, CR and AVE
7.5. Internal consistency reliability
The implementation of Internal Consistency Reliability (ICR) aims to assess result consistency across indicators. In the current approach, CA and CR were reported as evaluation metrics. ICR values should range from 0 to 1, with CA and CR exceeding 0.70 according to Hair et al. (Citation2019). Table shows the results for CA and CR. It can be observed that both the composite reliability and CA values for all constructs are satisfactory, surpassing the recommended thresholds. Specifically, AT exhibited a CA of 0.844 and a CR of 0.879, BI had a CA of 0.751 and a CR of 0.843, EE had a CA of 0.858 and a CR of 0.898, FC had a CA of 0.754 and a CR of 0.844, HB had a CA of 0.819 and a CR of 0.893, and HM had a CA of 0.795 and a CR of 0.880 (see Table ).
7.6. Convergent validity
Convergent validity, a component of construct validity, refers to the high correlation expected between tests that measure the same or similar constructs (Hair et al., Citation2019). Convergent validity is assessed by calculating the Average Variance Extracted (AVE). According to Hair et al. (Citation2022), AVE values should be 0.50 or greater (see Table ). Upon computation, all the constructs yielded AVE values that explained more than 0.5 of the variances. The AVE values for AT, BI, EE, FC, HB, HM, PE, PLO, SI and T were 0.645, 0.573, 0.639, 0.576, 0.736, 0.710, 0.726, 0.759, 0.601 and 0.591 respectively (see Table ). Table indicates that all constructs possess acceptable convergent validity. Consequently, it can be concluded that all constructs are suitable for further analyses.
Furthermore, it was observed that the item loadings corresponding to their respective underlying constructs exhibited higher magnitudes than the cross-loadings (see Appendix A). Moreover, the assessment of the cross-loadings (see Appendix A) provides evidence of convergent validity. As a result, there was no need to eliminate any of the items. Once convergent validity was established, the next step involved examining discriminant validity, which aims to determine the distinctiveness of each construct in the structural model from others (Hair et al., Citation2021). The PLS-SEM algorithm results (path coefficients and item loadings) are depicted in Figure .
7.7. Discriminant validity
Discriminant validity pertains to the extent to which a given construct is empirically distinguishable from other constructs. In this study, three distinct procedures were employed to assess the discriminant validity. To assess this, the Fornell and Larcker criterion (see Table ), the Heterotrait-Monotrait (HTMT) ratio (see Table ) and cross-loadings (see Appendix A) were employed. According to the Fornell-Larcker criterion, it is expected that the Average Variance Extracted (AVE) of a particular construct should surpass the shared variance of other constructs (Fornell & Larcker, Citation1981). As shown in Table , it is observed that the (e.g., 0.803, 0.757, 0.800, 0.759, 0.858, 0.843, 0.871, 0.852, 0.775, 0.769) for each construct surpasses the inter-construct correlations.
Table 2. Discriminant validity using Fornell-Larcker criterion
Table 3. Heterotrait-Monotrait (HTMT, <0.90) ratio for discriminant validity
In addition, Henseler et al. (Citation2015) introduced a novel method for assessing discriminant validity within the framework of partial least squares structural equation modeling. This method entails scrutinizing the Heterotrait-Monotrait (HTMT) correlation ratio, as documented by Rahi et al. (Citation2018). A HTMT coefficient that approximates 1 indicates insufficient discriminant validity. To evaluate discriminant validity using the HTMT criterion, a comparison is made between the HTMT coefficient and a pre-established threshold. If the HTMT value exceeds this threshold, it suggests an issue with discriminant validity (Hair et al., Citation2014). Henseler et al. (Citation2015) suggested using a threshold of 0.85 or 0.90 (). Table displays the outcomes of the HTMT criterion, exhibiting values that satisfy the minimum prerequisite and encompass a satisfactory spectrum in accordance with Henseler et al. (Citation2015). Consequently, no apprehension regarding discriminant validity arises.
7.8. Goodness of Fit (GOF)
The measurement model was assessed for a satisfactory goodness-of-fit (GOF); the overall model fit was evaluated by evaluating two main indices (Standardized Root Mean Square Residual [SRMR] and Normed Fit Index [NFI]). Since there are no universally accepted Goodness of Fit (GOF) statistics for PLS-SEM, Hair et al. (Citation2017) proposed the use of the SRMR as a model fit index. In this study, the SRMR value for the structural model was determined to be 0.072, which falls below the recommended threshold of 0.08 set by Hair et al. (Citation2017). Additionally, the Normed Fit Index (NFI = 0.912) exceeded the recommended threshold of 0.90, as suggested by Hair et al. (Citation2018), further supporting the conclusion of a satisfactory model fit. Based on these results, it can be inferred that the model exhibits a favourable level of fit.
7.9. Multicollinearity
To evaluate the predictive capabilities of the structural model, an analysis was conducted. However, prior to introducing the model, it is important to evaluate collinearity through the disclosure of variance inflation factor (VIF) values. The initial phase of the structural model encompassed an assessment of the existence of collinearity problems, which may arise from strong correlations among predictor constructs (Sarstedt & Mooi, Citation2019). The VIF values were scrutinized to ensure the absence of collinearity issues. Generally, VIF values that are below 5 are deemed acceptable to verify the non-existence of collinearity (Ringle et al., Citation2023). The outcomes of the collinearity are exhibited in Table .
Table 4. Multicollinearity test
Table reveals that the lowest and highest VIF values are 1.337 and 3.683 respectively, suggesting that collinearity is not a significant problem (Ringle et al., Citation2023). In the current study, the VIF values for the inner model and outer model were less than 5 (Hair et al., Citation2021).
7.10. Structural model
Structural model evaluation was used to examine the paths between the research model’s constructs in regard to the proposed hypotheses, “coefficient of determination or predictive power,” () and predictive relevance (
) ([Hair et al., Citation2019]). The subsequent phase entailed evaluating the importance of the suggested path coefficients (hypotheses) within the research model. To achieve this, a bootstrapping process consisting of 5,000 sub-samples was carried out (Hair et al., Citation2013). Figure and Table show the PLS-SEM bootstrapping results.
Table 5. Path coefficients and hypotheses testing
The results in Table show that attitude had a statistically significant negative influence on students’ behavioural intention to use tablet-based learning (AT → BI) [β = −0.144, t = 2.707, p = .007 < .05], therefore, H1 is supported. The negative path coefficient (β = −0.144) implies that a one unit increase in attitude will decrease behavioural intention by 0.144. Also, effort expectancy had a significant effect on students’ behavioural intention to use tablet-based learning (EE → BI) [β = 0.154, t = 2.474, p = .014 < .05], hence, H2 is supported. The positive path coefficient (β = 0.154) suggests that a one unit increase in effort expectancy will increase behavioural intention by 0.154.
Additionally, facilitating conditions had a statistically significant influence on students’ behavioural intention to use tablets (FC → BI) [β = 0.131, t = 2.259, p = .024 < .05], consequently, H3 is supported. The positive path coefficient (β = 0.131) means that a one unit increase in facilitating conditions will increase behavioural intention by 0.131. However, habit had no significant effect on students’ behavioural intention to use tablets (HB → BI) [β = −0.034, t = 0.626, p = .531 > .05], therefore, H4a is not supported.
Moreover, the results in Table show that hedonic motivation had a statistically significant effect on students’ behavioural intention to use tablet-based learning (HM → BI) [β = 0.187, t = 2.570, p = .010 < .05], hence, H5a is supported. The positive path coefficient (β = 0.187) implies that a one unit increase in hedonic motivation will increase behavioural intention by 0.187. In addition, perceived learning opportunities had a significant positive influence on students’ behavioural intention to embrace tablet-based learning (PLO → BI) [β = 0.156, t = 2.661, p = .008 < .05], thus, H6 is supported. The positive path coefficient (β = 0.156) suggests that a one unit increase in perceived learning opportunities will increase behavioural intention by 0.156. Performance expectancy had no significant effect on students’ behavioural intention to use tablets (PE → BI) [β = 0.021, t = 0.389, p = .697 > .05], consequently, H7 is not upheld.
Furthermore, social influence had a statistically significant influence on students’ behavioural intention to use tablet-based learning (SI → BI) [β = 0.257, t = 5.328, p < .001], hence, H8 is supported. The positive path coefficient (β = 0.257) implies that a one unit increase in social influence will increase behavioural intention by 0.257. Lastly, the results revealed that trust had a statistically significant influence on students’ behavioural intention to use tablet-based learning (T → BI) [β = 0.167, t = 3.031, p = .003 < .05], therefore, H9 is supported. The presence of a positive path coefficient (β = 0.167) indicates that if trust is increased by one unit, there will be a corresponding increase in behavioural intention by 0.167 units.
7.11. Coefficient of determination, effect size and predictive relevance
The coefficient of determination (), often denoted as R-squared, is a fundamental metric employed in evaluating the explanatory capacity of a model. It quantifies the proportion of variability in the dependent variable that can be accounted for by the independent variables. From Table , the
value (0.590) for the structural model revealed that AT, EE, FC, HM, PLO, SI and T account for 59.0% of the variance in students’ behavioural intention (BI) to use tablets. Also, the
reveals a moderate level of predictive power (Hair et al., Citation2016). This result indicates that there exists a significant proportion of variance, amounting to 41.0% in the BI construct, which can be attributed to exogenous factors not accounted for in the current model.
Table 6. Coefficient of determination, effect size and predictive relevance
Cohen’s , which assesses the magnitude of the impact of an independent variable on a dependent variable, is a pertinent metric. As per Cohen’s (Citation2013) guidelines, effect sizes of 0.02 or greater, 0.15 or greater, and 0.35 or greater reflect small, moderate, and large effect sizes, correspondingly. The results presented in Table reveal that the effect sizes of the structural model for the significant paths range from 0.016 to 0.071, indicating small effect sizes.
To evaluate the predictive significance of the model, the Stone-Geisser measure was utilised to ascertain its relevance in the study. In corroboration, Kock (Citation2021) posited that values exceeding zero are imperative in establishing predictive relevance. A blindfolding procedure was performed to calculate
estimate. Evaluation of the outcome presented in Table reveals that AT, EE, FC, HM, PLO, SI and T demonstrate a moderate level of predictive relevance, aligned with the prescribed criteria by Hair et al. (Citation2014). Table shows the results for the coefficient of determination (
), effect size (
) and predictive relevance (
).
7.12. Moderating effect
This section provides an exposition of the outcomes pertaining to the moderating effect of gender on (a) the correlation between hedonic motivation and behavioural intention and (b) the correlation between habit and behavioural intention. The moderating effect of gender is illustrated in Table .
Table 7. Moderating effect of gender
In Table , the results show that the moderating role of gender on the relationship between habit and behavioural intention is not statistically significant (Mod_G_HB → BI) [β = −0.018, t = 0.250, p = 0.802 > .05]. Hence, H4b is not supported. Additionally, gender does not moderate the positive relationship between hedonic motivation and behavioural intention (Mod_G_HM → BI) [β = 0.063, t = 0.716, p = 0.474 > .05]. Therefore, H5b is not sustained.
7.13. Importance-Performance Map Analysis (IPMA)
To augment the PLS-SEM analysis, an importance-performance map analysis (IPMA) was conducted to assess the significance and effectiveness of variables influencing behavioural intention to use tablet-based learning. By employing IPMA, variables that exhibit high importance but low performance can be identified for targeted improvement. The standardized total effects (importance) and the standardized latent variable scores (performance) are presented in Table and Figure , respectively.
Table 8. Total effects and performance Index values for behavioural intention
In relation to their respective levels of importance, the results in Table reveal that social influence (SI) emerged as the highest-ranking factor (0.257), closely followed by HM (0.187), T (0.167), PLO (0.156), EE (0.154) and finally, FC (0.131). Regarding performance, PLO exhibited the highest score (78.191), followed by AT (77.511), HM (75.117), EE (74.272), FC (73.669) T (72.526) and lastly SI (52.563). These findings highlight the significance of SHS institutions in Ghana focusing their attention on improving social influence, as it demonstrated relatively lower performance compared to other factors, despite being the most crucial aspect in fostering students’ behavioural intention to use tablets. Figure illustrates the importance-performance map within the context of our model.
8. Discussion
In accordance with quality education, which is one of the crucial elements expected to accelerate the United Nations’ sustainable development agenda set to be achieved by 2030, as well as the COVID-19 pandemic, which abruptly impacted traditional education practices, a number of digital technologies have emerged. According to a recent study by Haleem et al. (Citation2022), several new digital learning tools (e.g., smartboards, tablets, laptops, MOOCs and virtual laboratories) are now widely used in educational institutions. While scholars have shown significant interest in predicting factors driving students’ acceptance and use of tablets for learning, empirical evidence in Ghana is rare. Furthermore, despite numerous studies conducted in different settings, there is still limited understanding of students’ behavioural intention regarding tablet use, as reflected in theoretical examinations
The objective of this research was to determine the variables that influence the intentions of senior high school (SHS) students in Ghana to utilise tablet-based learning. The research model presented in this paper is unique in its integration of perceived learning opportunity, trust, and attitude into the UTAUT2 model to evaluate the determinants of students’ behavioural intentions toward tablet-based learning. In addition, we examined whether gender (i.e., male and female) moderates the relationship between habit and hedonic motivation on the behavioural intention to use TBL among SHS students. Employing the PLS-SEM for the analysis of data from a cross-sectional survey of 409 SHS students, our model demonstrated a predictive power, explaining 59% of variance in behavioural intention. Thus, seven (7) hypotheses out of the eleven (11) tested relationships were significant. The results in Table revealed that social influence emerged as the highest-ranking factor in terms of importance among the predictors, closely followed by hedonic motivation, trust, perceived learning opportunity, effort expectancy, and, finally, facilitating conditions. This suggests that creating a positive social environment that supports tablet-based learning can be crucial in encouraging students to adopt and embrace this technology. Educational institutions can leverage social influence by implementing strategies such as peer recommendations, testimonials, and showcasing success stories of students who have benefited from tablet-based learning
In addition, perceived learning opportunities received the highest score among the factors, followed by attitude, hedonic motivation, effort expectancy, facilitating conditions, trust, and social influence (refer to Table ). This indicates that students believe tablets offer a promising platform to enhance their educational experiences and achieve their learning goals. The strong positive relationship between students’ perceived learning opportunity and their behavioural intention implies that educational institutions can increase adoption and acceptance of tablet-based learning by effectively leveraging and optimizing the learning opportunities offered by tablets. However, the moderating role of gender on the relationship between habit and behavioural intention was not significant. Similarly, the moderating role of gender on the relationship between hedonic motivation and behavioural intention was found to be inconclusive.
However, the results of our analysis established significant negative effect of attitude on students’ behavioural intention toward the use of tablets. This negative effect indicates resistance, which can create barriers to successful implementation of tablet-based learning initiatives. Thus, students may be less willing to engage in technology and utilise its features. This contrasts with previous studies conducted by Hussein (Citation2017), Nisa and Solekah (Citation2022), Songkram et al. (Citation2023), and Svenningsson et al. (Citation2021), which concluded that attitude has a positive influence on behavioural intention to use new technology. Additionally, the results demonstrated a significant positive effect of effort expectancy on SHS students’ behavioural intention to use tablets. This aligns with previous research conducted by Al-Adwan et al. (Citation2022), Hamzah et al. (Citation2020), Meet et al. (Citation2022), Twum et al. (Citation2022), Wang et al. (Citation2022) and Yidana et al. (Citation2023), which found a significant impact of effort expectancy on behavioural intention. Therefore, for the future development of tablet-based learning, educational stakeholders are recommended to create an inclusive and supportive learning environment that encourages students to embrace tablets as a valuable tool for learning.
Furthermore, the results indicated a substantial impact of facilitating conditions on SHS students’ intention to embrace tablet-based learning. The significant positive relationship between facilitating conditions and the intention to use tablet-based learning signifies the key role that is required from SHS institutions. As educational stakeholders seek to increase the use of TBL systems, greater attention should be given to facilitating conditions to enhance students’ intention to use TBL. This finding is consistent with previous research conducted by Alshammari (Citation2021), Khrais and Alghamdi (Citation2021), Mubuke et al. (Citation2017), Yidana et al. (Citation2023) and Youssef and Issam (Citation2022), which revealed a positive and significant relationship between facilitating conditions and the intention to use digital learning platforms. However, this contradicts the findings of Shuhaiber (Citation2016), which indicated that facilitating conditions, specifically technical resource availability, had an insignificant impact on usage intention among students in virtual lectures
Similarly, the findings revealed that hedonic motivation has a significant and positive impact on Ghanaian SHS students’ behavioural intentions toward adopting tablet-based learning. This highlights the importance of hedonic motivation as an internal factor within the UTAUT2 model. To encourage greater adoption among SHS students, it is crucial to emphasize the enjoyable aspects of tablet-based learning, including its interactive and engaging features. These findings align with previous studies (Alalwan et al., Citation2017; Ayasrah, Citation2020; Hartelina et al., Citation2021; Moorthy et al., Citation2019; Twum et al., Citation2022; Yidana et al., Citation2023; Zacharis & Nikolopoulou, Citation2022) that found a significant positive association between hedonic motivation and behavioural intention. Therefore, if SHS students view tablets as enjoyable and pleasurable tools for digital learning, their gender does not significantly influence their decision to use them.
Likewise, the findings showed that perceived learning opportunity is significant and positively associated with behavioural intentions to use tablet-based learning. The positive effect of perceived learning opportunities implies that SHS students’ efforts to enhance their learning opportunities can lead to increased adoption and usage of the tablet-based learning platform, which has the potential to meet future digital needs. In other words, if SHS students perceive the platform as providing valuable learning opportunities, they are more likely to embrace and adopt the tablet-based learning technology. This aligns with the findings of Boomaars et al. (Citation2018), Dutton (Citation2018), and Steil et al. (Citation2020), who argued that perceived learning opportunities significantly influence individuals’ behavioural intentions to use technology. The strong positive association between perceived learning opportunities and SHS students’ behavioural intentions implies that if educational institutions can effectively leverage and optimise the learning opportunities offered by tablets, they are likely to see increased adoption and acceptance of tablet-based learning among students in the Ghanaian setting.
Furthermore, social influence as a psychosocial construct in the UTAUT2 model emerged as a fundamental determinant of SHS students’ behavioural intentions to use tablet-based learning technology. This indicates that SHS students recognise that their expectations from important individuals, including peers, teachers, and family members, have a positive impact on their adoption of digital learning systems. The influence of social influence on behavioural intention has been supported by various previous studies, including Bervell et al. (Citation2022), Leow et al. (Citation2021), Meet et al. (Citation2022), Nassar et al. (Citation2019), Wang et al. (Citation2022) and Yidana et al. (Citation2023). As a result, interventions aimed at leveraging social influence can play a crucial role in shaping students’ perceptions and behaviours regarding the use of digital learning tools. For instance, providing teachers, who are recognised as influential figures, with sufficient training and support in integrating tablet-based learning tools into their teaching practices can enhance their ability to positively influence students’ intentions to use these tools.
9. Conclusion
This study aimed to develop an integrated model that explores the individual-level factors influencing senior high school (SHS) students’ behavioural intentions towards tablet-based learning. To achieve this, we extended the widely recognised UTAUT2 model by including three additional predictor variables: attitude, perceived learning opportunity and trust. Data were collected from a sample of 409 public SHS students in the Kumasi Metropolis who had prior experience with tablet-based learning. Based on the results from the PLS-SEM analysis, the study found positive effects of factors such as trust, perceived learning opportunities, effort expectancy, facilitating conditions, hedonic motivation, and social influence on the behavioural intentions of SHS students. By understanding these factors as key drivers of tablet-based learning adoption among SHS students, educational stakeholders can improve the quality of education and facilitate the effective integration of these technologies into teaching and learning practices.
10. Implications and contributions of the study
The results of this study indicated that the use of tablet-based learning (TBL) is strongly influenced by perceived learning opportunities, trust, effort expectancy, hedonic motivation, social influence, and facilitating conditions. Specifically, the study found that perceived learning opportunities and trust were the most significant factors in determining individual students’ intention to use tablet-based learning. Conversely, students’ negative attitudes serve as a hurdle in their intention to use tablet-based learning. Overall, this study provides new insights into the factors that influence students’ adoption of learning technologies like tablets.
10.1. Practical implications
The findings of this study provide practical implications for educational institutions, such as the Ghana Education Service, as well as policymakers and educators, including headmasters and teachers regarding the key factors that need to be considered in order to promote the successful implementation of TBL. Specifically, instructors and administrators can use the study’s findings to identify the factors that affect students’ intentions to use TBL and take measures to encourage its adoption and usage in their teaching methods. Recognising the critical elements that influence individual’s intentions to use TBL and using this information to enhance learning are two ways that students can benefit from this study’s findings. For example, since perceived learning opportunities were found to be a significant determinant, efforts can be made to improve the availability and accessibility of learning resources and support systems to encourage TBL adoption.
10.2. Theoretical contributions
The study makes several contributions by utilising the UTAUT2 model and incorporating key determinants to examine the intention to use Tablet-based learning (TBL) in Ghana. By incorporating the UTAUT2 model, which includes performance expectancy, effort expectancy, social influence, facilitating conditions, habit, and hedonic motivation, the study provides a comprehensive framework for understanding the factors influencing the intention to use TBL in Ghana. Moreover, by examining attitude, trust, and perceived learning opportunities as additional determinants within the UTAUT2 model, the study identified important factors that can significantly influence students’ intention to use TBL. This identification expands the understanding of the specific factors that are crucial in the Ghanaian context and may have implications for the design and implementation of TBL initiatives. Furthermore, this study bridges the gap in the existing literature by highlighting the importance of various determinants within the UTAUT2 model for TBL adoption in Ghana. This theoretical framework allows for a holistic examination of the determinants that shape students’ attitudes and intentions toward adopting TBL.
10.3. Contribution to methodology
This study improves the field by presenting actual data on the factors impacting students’ intention to use TBL in SHS in Ghana. The study identified perceived learning opportunities, trust, effort expectancy, hedonic motivation, social influence, and facilitating conditions as significant predictors of students’ desire to employ TBL. As a result, the variables that predict students’ intention to use TBL in SHS are now known. Additionally, this study significantly contributes to literature on the use of structural equation modelling approach (SEM) to forecast the behavioural intentions toward the utilisation of TBL among SHS students in Ghana and beyond. The study’s use of SEM is a novel strategy that sheds light on the intricate connections and interactions between the variables that influence students’ intentions to use TBL. Future studies in an attempt to understand the predictors of behavioural intentions towards the use of TBL and other technology adoption in education can benefit from this methodological approach. Thus, the research methodology employed in this study can inform policies and strategies to encourage technology adoption and use.
10.4. Contribution to policy
The findings of this study contribute to policies for educational institutions (such as Ghana Education Service), policymakers, and educators (e.g., headmasters and teachers) in Ghana. As mentioned, this study found that incorporating TBL into teaching and learning practices in SHS should lead to the creation of policies to improve perceived learning opportunities, trust, effort expectancy, hedonic motivation, social influence, and facilitating conditions. Specifically, perceived learning opportunities and trust were found to be the most significant factors in determining individual students’ intention to use tablet-based learning. Negative attitudes towards TBL can reduce the positive intentions to use TBL. These regulations may also involve training initiatives to improve technological literacy, such as practical workshops, online tutorials, or mentoring. User-centered design strategies and student feedback mechanisms are additional ways to encourage a favourable view of TBL among the students. Educational institutions can guarantee effective use of TBL by implementing certain policies and procedures.
11. Limitations and suggestion for future directions
Despite the strengths of this study, certain limitations should be addressed in future studies. Over time, students’ perceptions of learning opportunities, effort expectancy, performance expectancy, habit, trust, and behavioural intentions towards tablet-based learning may change as they accumulate new knowledge and experiences. Therefore, further studies could benefit from adopting a longitudinal design, as it would provide more precise findings by tracking changes in perceptions and behavioural intentions within a specific group over an extended period. Although gender served as the moderator in this study, it is worth noting that other variables, such as experience and information and communication technology (ICT) literacy, could also potentially moderate the relationship between behavioural intentions and other factors. As a result, it is crucial for future studies to consider these variables as potential moderators.
Availability of data and materials
The corresponding author can provide access to the data that underpins the conclusions drawn in this study, upon the submission of a reasonable request.
Disclosure statement
No potential conflict of interest was reported by the author(s).
Additional information
Funding
Notes on contributors
Francis Arthur
Francis Arthur holds a Master of Philosophy degree in Economics Education. He is currently a Ph.D candidate in Economics Education in the Department of Business and Social Sciences Education (DoBSSE) at the University of Cape Coast (UCC) who is passionate about research on issues concerning teaching and learning of Economics with much interest in emerging issues such as Preservice and In-service Teachers’ Self-Efficacy, Application of Multiple Intelligences Approach, Artificial Intelligence and Emerging Technologies in Teaching and Learning.
Valentina Arkorful
Valentina Arkorful has a PhD in Educational Technology and a Master’s degree in Information Technology Education from the University of Cape Coast. Professionally, she holds a certificate in Faculty Online Teaching from Ghana Technology University and Certificate in Designing and Facilitating eLearning, Open Polytechnic University of New Zealand. Valentina does research in ICT integration in Teaching and Learning, Gender Adoption of Technologies in Teaching and Learning, E-learning and Assessment Strategies in Education using ICT. She is also a Senior lecturer at the College of Distance Education, University of Cape Coast where she facilitates courses in Technology Integration.
Iddrisu Salifu
Iddrisu Salifu is a social scientist who holds a Master's degree in Economics from the School of Economics at the University of Cape Coast (UCC), Ghana as well as a Master's degree in Integrated Coastal Zone Management (ICZM) from the World Bank's Africa Centre of Excellence in Coastal Resilience (ACECoR), UCC. Iddrisu’s research interests focus on applied microeconomics, including interdisciplinary studies centered on health, coastal, and environmental management, as well as technology adoption. Iddrisu is currently a research assistant at the Cardiometabolic Epidemiology Research Laboratory (CERL), HPER-UCC. He also teaches various courses in Economics as part-time at the Institute of Education (IoE), in the University of Cape Coast, Ghana.
References
- Addae, D., Amponsah, S., & Gborti, B. J. (2022). COVID-19 pandemic and the shift to digital learning: Experiences of students in a community college in Ghana. Community College Journal of Research and Practice, 46(1–2), 101–33.
- Aheto-Domi, B., Issah, S., & Dorleku, J. E. A. (2020). Virtual learning and readiness of tutors of colleges of education in Ghana. American Journal of Educational Research, 8(9), 653–658. https://doi.org/10.12691/education-8-9-6
- Ahmad, J. (2016). Technology assisted language learning is a silver bullet for enhancing language competence and performance: A case study. International Journal of Applied Linguistics and English Literature, 5(7), 118–131.
- Akter, S., Fosso Wamba, S., & Dewan, S. (2017). Why PLS-SEM is suitable for complex modelling? An empirical illustration in big data analytics quality. Production Planning & Control, 28(11–12), 1011–1021. https://doi.org/10.1080/09537287.2016.1267411
- Al-Adwan, A. S., Albelbisi, N. A., Hujran, O., Al-Rahmi, W. M., & Alkhalifah, A. (2021). Developing a holistic success model for sustainable e-learning: A structural equation modeling approach. Sustainability, 13(16), 9453. https://doi.org/10.3390/su13169453
- Al-Adwan, A. S., Yaseen, H., Alsoud, A., Abousweilem, F., & Al-Rahmi, W. M. (2022). Novel extension of the UTAUT model to understand continued usage intention of learning management systems: The role of learning tradition. Education and Information Technologies, 27(3), 1–27. https://doi.org/10.1007/s10639-021-10758-y
- Alalwan, A. A., Dwivedi, Y. K., & Rana, N. P. (2017). Factors influencing adoption of mobile banking by jordanian bank customers: Extending UTAUT2 with trust. International Journal of Information Management, 37(3), 99–110. https://doi.org/10.1016/j.ijinfomgt.2017.01.002
- Albahri, A. S., Alnoor, A., Zaidan, A. A., Albahri, O. S., Hameed, H., Zaidan, B. B., Peh, S. S., Zain, A. B., Siraj, S. B., Masnan, A. H. B., & Yass, A. A. (2022). Hybrid artificial neural network and structural equation modelling techniques: A survey. Complex & Intelligent Systems, 8(2), 1781–1801. https://doi.org/10.1007/s40747-021-00503-w
- Alshammari, S. (2021). Determining the factors that affect the use of virtual classrooms: A modification of the UTAUT model. Journal of Information Technology Education Research, 20, 117. https://doi.org/10.28945/4709
- Ameen, N., & Willis, R. (2019). Towards closing the gender gap in Iraq: Understanding gender differences in smartphone adoption and use. Information Technology for Development, 25(4), 660–685. https://doi.org/10.1080/02681102.2018.1454877
- Ameri, A., Khajouei, R., Ameri, A., & Jahani, Y. (2020). Acceptance of a mobile-based educational application (LabSafety) by pharmacy students: An application of the UTAUT2 model. Education and Information Technologies, 25(1), 419–435. https://doi.org/10.1007/s10639-019-09965-5
- Amid, A., & Din, R. (2021). Acceptance and use of massive open online courses: Extending UTAUT2 with personal innovativeness. Journal of Personalized Learning, 4(1), 57–66.
- Ansong-Gyimah, K. (2020). Students’ perceptions and continuous intention to use E-Learning systems: The case of Google classroom. International Journal of Emerging Technologies in Learning (iJet), 15(11), 236–244. https://doi.org/10.3991/ijet.v15i11.12683
- Appiah, K. M. (2022). A simplified model to enhance SMEs’ investment in renewable energy sources in Ghana. International Journal of Sustainable Energy Planning and Management, 35, 83–96. https://doi.org/10.54337/ijsepm.7223
- Arenas-Gaitán, J., Peral Peral, B., & Ramón Jerónimo, M. (2015). Elderly and internet banking: An application of UTAUT2. Journal of Internet Banking and Commerce, 20(1), 1–23.
- Arpaci, I. (2016). Understanding and predicting students’ intention to use mobile cloud storage services. Computers in Human Behaviour, 58, 150–157. https://doi.org/10.1016/j.chb.2015.12.067
- Arthur, F., & Kaku, D. W. (2020). The use of technology in the teaching of economics: Perspectives of economics students. GSJ, 8(8), 2906–2919. https://www.researchgate.net/profile/Francis-Arthur-8/publication/344101313_THE_USE_OF_TECHNOLOGY_IN_THE_TEACHING_OF_ECONOMICS_PERSPECTIVES_OF_ECONOMICS_STUDENTS/links/5fc9d573a6fdcc697bdb8f96/THE-USE-OF-TECHNOLOGY-IN-THE-TEACHING-OF-ECONOMICS-PERSPECTIVES-OF-ECONOMICS-STUDENTS.pdf
- Awang, H., Rahman, A. A., Sukeri, S., Hashim, N., & Rashid, N. R. N. A. (2019). Adolescentfriendly health services in primary healthcare facilities in Malaysia and its correlation with adolescent satisfaction level. International Journal of Adolescence and Youth, 25(1), 551–561. https://doi.org/10.1080/02673843.2019.1685556
- Ayasrah, F. T. M. (2020). Exploring E-Learning readiness as mediating between trust, hedonic motivation, students’ expectation, and intention to use technology in Taibah University. Journal of Education & Social Policy, 7(1), 101–109. https://doi.org/10.30845/jesp.v7n1p13
- Baker, T. L. (1994). Doing social research (2nd ed.). McGraw-Hill Inc.
- Bandura, A. (1989). Human agency in social cognitive theory. The American Psychologist, 44(9), 1175.
- Batsila, M. A., Tsihouridis, C. A., & Tsihouridis, A. H. (2017). “All for one and one for all”-creating a mobile learning net for ESP students’ needs. International Journal of Emerging Technologies in Learning, 12(4), 17. https://doi.org/10.3991/ijet.v12i04.6428
- Bervell, B. B., Kumar, J. A., Arkorful, V., Agyapong, E. M., & Osman, S. (2022). Remodelling the role of facilitating conditions for Google classroom acceptance: A revision of UTAUT2. Australasian Journal of Educational Technology, 38(1), 115–135. https://doi.org/10.14742/ajet.7178
- Bhimasta, R. A., & Suprapto, B. (2016). An empirical investigation of student adoption model toward mobile e-textbook: UTAUT2 and TTF model. In proceedings of the 2nd international conference on communication and information processing (pp. 167–173).
- Boomaars, C., Yorks, L., & Shetty, R. (2018). Employee learning motives, perceived learning opportunities and employability activities. Journal of Workplace Learning, 30(5), 335–350. https://doi.org/10.1108/JWL-01-2018-0020
- Brossard, M., Carnelli, M., Chaudron, S., DiGioia, R., Dreesen, T., Kardefelt-Winther, D. … Yameogo, J. L. (2021). Digital learning for every child: Closing the gaps for an inclusive and prosperous future. G20 Insights Policy Brief, September.
- Cai, L., Yuen, K. F., Xie, D., Fang, M., & Wang, X. (2021). Consumer’s usage of logistics technologies: Integration of habit into the unified theory of acceptance and use of technology. Technology in Society, 67, 101789. https://doi.org/10.1016/j.techsoc.2021.101789
- Chao, C. M. (2019). Factors determining the behavioural intention to use mobile learning: An application and extension of the UTAUT model. Frontiers in Psychology, 10, 1652. https://doi.org/10.3389/fpsyg.2019.01652
- Cheah, J.-H., Ting, H., Cham, T. H., & Memon, M. A. (2019). The effect of selfie promotion and celebrity endorsed advertisement on decision-making processes: A model comparison. Internet Research, 29(3), 552–577. https://doi.org/10.1108/IntR-12-2017-0530
- Chiu, J. L., Bool, N. C., & Chiu, C. L. (2017). Challenges and factors influencing initial trust and behavioural intention to use mobile banking services in the Philippines. Asia Pacific Journal of Innovation and Entrepreneurship, 11(2), 246–278. https://doi.org/10.1108/APJIE-08-2017-029
- Cohen, J. (2013). Statistical power analysis for the behavioural sciences. Academic.
- Davis, F. D. (1989). Technology acceptance model: TAM. Information Seeking Behavior and Technology Adoption.
- Davis, F. D., Bagozzi, R. P., & Warshaw, P. R. (1992). Extrinsic and intrinsic motivation to use computers in the workplace. Journal of Applied Social Psychology, 22(14), 1111–1132.
- Dillman, D. A., Smyth, J. D., & Christian, L. M. (2014). Internet, phone, mail, and mixed-mode surveys: The tailored design method. John Wiley & Sons.
- Dumford, A. D., & Miller, A. L. (2018). Online learning in higher education: Exploring advantages and disadvantages for engagement. Journal of Computing in Higher Education, 30, 452–465.
- Dutton, K. (2018). Professional development: Investigating the relationships between employee motives, learning opportunities, and activities. Development & Learning in Organizations: An International Journal, 32(5), 32–35. https://doi.org/10.1108/DLO-09-2018-127
- Dzandza, P. E. (2020). Digitizing the intellectual output of Ghanaian universities. Collection and Curation, 39(3), 69–75. https://doi.org/10.1108/CC-05-2019-0012
- Edem Adzovie, D., & Jibril, A. B. (2022). Assessment of the effects of COVID-19 pandemic on the prospects of e-learning in higher learning institutions: The mediating role of academic innovativeness and technological growth. Cogent Education, 9(1), 2041222. https://doi.org/10.1080/2331186X.2022.2041222
- Feng, G. C., Su, X., Lin, Z., He, Y., Luo, N., & Zhang, Y. (2021). Determinants of technology acceptance: Two model-based meta-analytic reviews. Journalism & Mass Communication Quarterly, 98(1), 83–104. https://doi.org/10.1177/1077699020952400
- Fernandez, S. C., & Mammen, K. J. (2020). Perceptions of selected stakeholders on the use of tablets for University teaching. TEM Journal, 9(4), 1809. https://doi.org/10.18421/TEM94-64
- Fianu, E., Blewett, C., Ampong, G. O. A., & Ofori, K. S. (2018). Factors affecting MOOC usage by students in selected Ghanaian universities. Education Sciences, 8(2), 70. https://doi.org/10.3390/educsci8020070
- Fishbein, M., & Ajzen, I. (1975). Belief, attitude, intention, and behavior: An introduction to theory and research. Don Mills.
- Fornell, C., & Larcker, D. F. (1981). Evaluating structural equation models with unobservable variables and measurement error. Journal of Marketing Research, 18(1), 39–50. https://doi.org/10.1177/002224378101800104
- Fraenkel, J. R., Wallen, N. E., & Hyun, H. H. (2018). How to design and evaluate research in education. (10th ed.). McGraw Hill.
- Furió, D., Juan, M. C., Seguí, I., & Vivó, R. (2015). Mobile learning vs. traditional classroom lessons: A comparative study. Journal of Computer Assisted Learning, 31(3), 189–201. https://doi.org/10.1111/jcal.12071
- Ghauri, P., Grønhaug, K., & Strange, R. (2020). Research methods in business studies. Cambridge University Press.
- Giebelhausen, M., Lawrence, B., & Chun, H. H. (2020). Doing good while behaving badly: Checkout charity process mechanisms. Journal of Business Ethics, 172(1), 1–17. https://doi.org/10.1007/s10551-019-04413-3
- Goto, J., & Munyai, A. (2022). The acceptance and use of online learning by law students in a South African University: An application of the UTAUT2 model. The African Journal of Information Systems, 14(1), 3.
- Gunawan, A., Muchardie, B. G., & Liawinardi, K. (2019). Millennial behavioural intention to adopt e-book using UTAUT2 model. In 2019 International Conference on Information Management and Technology (ICIMTech) (Vol. 1, pp. 98–102). IEEE.
- Hair, J. F., Jr., Howard, M. C., & Nitzl, C. (2020). Assessing measurement model quality in PLS-SEM using confirmatory composite analysis. Journal of Business Research, 109, 101–110. https://doi.org/10.1016/j.jbusres.2019.11.069
- Hair, J. F., Jr., Hult, G. T. M., Ringle, C., & Sarstedt, M. (2016). A primer on partial least squares structural equation modeling (PLS-SEM). Sage Publications.
- Hair, J. F., Hult, T., Ringle, C. M., & Sarstedt, M. (2022). A primer on partial least squares structural equation modeling (PLS-SEM) (3rd ed.). Sage.
- Hair, J., Hult, T., Ringle, C., & Sarstedt, M. (2014). A primer on partial least squares structural equation modeling (PLS-SEM). Sage Publications, Inc.
- Hair, J. F., Jr., Hult, G. T. M., Ringle, C. M., Sarstedt, M., Danks, N. P., & Ray, S. (2021). Partial least squares structural equation modeling (PLS-SEM) using R: A workbook. Springers.
- Hair, J. F., Jr., Matthews, L. M., Matthews, R. L., & Sarstedt, M. (2017). PLS-SEM or CB-SEM: Updated guidelines on which method to use. International Journal of Multivariate Data Analysis, 1(2), 107–123. https://doi.org/10.1504/IJMDA.2017.087624
- Hair, J. F., Ringle, C. M., & Sarstedt, M. (2013). Partial least squares structural equation modeling: Rigorous applications, better results and higher acceptance. Long Range Planning, 46(1–2), 1–12. https://doi.org/10.1016/j.lrp.2013.01.001
- Hair, J. F., Risher, J. J., Sarstedt, M., & Ringle, C. M. (2019). When to use and how to report the results of PLS-SEM. European Business Review, 31(1), 2–24. https://doi.org/10.1108/EBR-11-2018-0203
- Hair, J. F., Sarstedt, M., Ringle, C. M., & Gudergan, S. P. (2018). Advanced issues in partial least squares structural equations modeling (PLS-SEM). Sage.
- Haleem, A., Javaid, M., Qadri, M. A., & Suman, R. (2022). Understanding the role of digital technologies in education: A review. Sustainable Operations and Computers, 3, 275–285. https://doi.org/10.1016/j.susoc.2022.05.004
- Hameed, F., Qayyum, A., & Khan, F. A. (2022). A new trend of learning and teaching: Behavioural intention towards mobile learning. Journal of Computers in Education, 1–32. https://doi.org/10.1007/s40692-022-00252-w
- Hamzah, W. W., Yusoff, M., Ismail, I., & Yacob, A. (2020). The behavioural intentions of secondary school students to use tablet as a mobile learning device. International Journal of Interactive Mobile Technologies (iJim), 14(13), 161. https://doi.org/10.3991/ijim.v14i13.13027
- Hartelina, H., Batu, R., & Hidayanti, A. (2021). What can hedonic motivation do on decisions to use online learning services? International Journal of Data & Network Science, 5(2), 121–126. https://doi.org/10.5267/j.ijdns.2021.2.002
- Henseler, J., Ringle, C. M., & Sarstedt, M. (2015). A new criterion for assessing discriminant validity in variance-based structural equation modeling. Journal of the Academy of Marketing Science, 43, 115–135.
- Hooda, A., Gupta, P., Jeyaraj, A., Giannakis, M., & Dwivedi, Y. K. (2022). The effects of trust on behavioural intention and use behaviour within e-government contexts. International Journal of Information Management, 67, 102553. https://doi.org/10.1016/j.ijinfomgt.2022.102553
- Huang, X. (2018). Social media use by college students and teachers: An application of UTAUT2 (Doctoral dissertation, Walden University).
- Hussein, Z. (2017). Leading to intention: The role of attitude in relation to technology acceptance model in e-learning. Procedia Computer Science, 105, 159–164. https://doi.org/10.1016/j.procs.2017.01.196
- Kabra, G., Ramesh, A., Akhtar, P., & Dash, M. K. (2017). Understanding behavioural intention to use information technology: Insights from humanitarian practitioners. Telematics and Informatics, 34(7), 1250–1261.
- Khalilzadeh, J., Ozturk, A. B., & Bilgihan, A. (2017). Security-related factors in extended UTAUT model for NFC based mobile payment in the restaurant industry. Computers in Human Behavior, 70, 460–474.
- Khrais, L. T., & Alghamdi, A. M. (2021). The role of mobile application acceptance in shaping e-customer service. Future Internet, 13(3), 77. https://doi.org/10.3390/fi13030077
- Kock. (2021). WarpPLS user manual: Version 7.0. ScriptWarp Systems.
- Kosiba, J. P. B., Odoom, R., Boateng, H., Twum, K. K., & Abdul-Hamid, I. K. (2022). Examining students’ satisfaction with online learning during the COVID-19 pandemic-an extended UTAUT2 approach. Journal of Further and Higher Education, 46(7), 988–1005. https://doi.org/10.1080/0309877X.2022.2030687
- Kumar, J. A., & Bervell, B. (2019). Google classroom for mobile learning in higher education: Modelling the initial perceptions of students. Education and Information Technologies, 24(2), 1793–1817. https://doi.org/10.1007/s10639-018-09858-z
- Kurt, E. Ö., & Tingoy, Ö. (2017). The acceptance and use of a virtual learning environment in higher education: An empirical study in Turkey, and the UK. International Journal of Educational Technology in Higher Education, 14(1), 1–15.
- Langthaler, M., & Bazafkan, H. (2020). Digitalization, education and skills development in the global South: An assessment of the debate with a focus on sub-Saharan Africa (No. 28). ÖFSE Briefing Paper.
- Laukkanen, T. (2016). Consumer adoption versus rejection decisions in seemingly similar service innovations: The case of the Internet and mobile banking. Journal of Business Research, 69(7), 2432–2439.
- Leow, L. P., Phua, L. K., & Teh, S. Y. (2021). Extending the social influence factor: Behavioural intention to increase the usage of information and communication technology-enhanced student-centered teaching methods. Educational Technology Research & Development, 69(3), 1853–1879. https://doi.org/10.1007/s11423-021-10017-4
- Liu, D., & Guo, X. (2017). Exploring gender differences in acceptance of mobile computing devices among college students. Information Systems and E-Business Management, 15(1), 197–223. https://doi.org/10.1007/s10257-016-0315-x
- Matthews, L. M. (2017). Applying multi-group analysis in PLS-SEM: A step-by-step process. In H. Latan & R. Noonan (Eds.), Partial least squares structural equation modeling – basic concepts, methodological issues and applications in press. Springer International Publishing AG.
- Meet, R. K., Kala, D., & Al-Adwan, A. S. (2022). Exploring factors affecting the adoption of MOOC in generation Z using extended UTAUT2 model. Education and Information Technologies, 27(7), 10261–10283. https://doi.org/10.1007/s10639-022-11052-1
- Memon, M. A., Salleh, R., Mirza, M. Z., Cheah, J. H., Ting, H., Ahmad, M. S., & Tariq, A. (2020). Satisfaction matters: The relationships between HRM practices, work engagement and turnover intention. International Journal of Manpower, 42(1), 21–50. https://doi.org/10.1108/IJM-04-2018-0127
- Memon, M. A., Ting, H., Cheah, J. H., Thurasamy, R., Chuah, F., & Cham, T. H. (2020). Sample size for survey research: Review and recommendations. Journal of Applied Structural Equation Modeling, 4(2), 1–20. https://doi.org/10.47263/JASEM.4(2)01
- Ministry of Education. (2018a). Education strategic plan.
- Mohan, M. M., Upadhyaya, P., & Pillai, K. R. (2020). Intention and barriers to use MOOCs: An investigation among the post graduate students in India. Education and Information Technologies, 25(6), 5017–5031. https://doi.org/10.1007/s10639-020-10215-2
- Moon, S. J. (2021). Investigating beliefs, attitudes, and intentions regarding green restaurant patronage: An application of the extended theory of planned behaviour with moderating effects of gender and age. International Journal of Hospitality Management, 92, 102727. https://doi.org/10.1016/j.ijhm.2020.102727
- Moorthy, K., Yee, T. T., T’ing, L. C., & Kumaran, V. V. (2019). Habit and hedonic motivation are the strongest influences in mobile learning behaviours among higher education students in Malaysia. Australasian Journal of Educational Technology, 35(4). https://doi.org/10.14742/ajet.4432
- Mubuke, F., Kutosi Masaba, A., Ogenmungu, C., & Mayoka Kituyi, G. Examining the effect of facilitating conditions as an imperative input in enhancing the intention to use mobile learning systems in universities. 2017.
- Nassar, A. A., Othman, K., & Nizah, M. A. B. M. (2019). The impact of the social influence on ict adoption: Behavioural intention as mediator and age as moderator. The International Journal of Academic Research in Business & Social Sciences, 9(11), 963–978. https://doi.org/10.6007/IJARBSS/v9-i11/6620
- Nisa, U. K., & Solekah, N. A. (2022). The influence of TAM, social influence, security relationship toward intention to use E wallet through attitude and trust. Iqtishoduna: Jurnal Ekonomi dan Bisnis Islam, 18(1), 35–50.
- Nouri, J., & Cerratto Pargman, T. (2016). When teaching practices Meet tablets’ affordances. Insights on the materiality of learning. In K. Verbert, M. Sharples, & T. Klobučar (Eds.), Adaptive and adaptable learning. EC-TEL 2016. Lecture notes in computer science (Vol. 9891, pp. 179–192). Springer.
- Osei, H. V., Kwateng, K. O., & Boateng, K. A. (2022). Integration of personality trait, motivation and UTAUT 2 to understand e-learning adoption in the era of COVID-19 pandemic. Education and Information Technologies, 27(8), 10705–10730. https://doi.org/10.1007/s10639-022-11047-y
- Owolabi, H. O., Ayandele, J. K., & Olaoye, D. D. (2020). A Systematic Review of Structural Equation Model (SEM). Open Journal of Educational Development (ISSN: 2734-2050), 1(2), 27–39. https://doi.org/10.52417/ojed.v1i2.163
- Podsakoff, P. M., MacKenzie, S. B., Lee, J. Y., & Podsakoff, N. P. (2003). Common method biases in behavioural research: A critical review of the literature and recommended remedies. Journal of Applied Psychology, 88(5), 879–903. https://doi.org/10.1037/0021-9010.88.5.879
- Polit, D. F., & Beck, C. T. (2017). Nursing research (10th ed.). Wolters Kluwer.
- Quaicoe, J. S., & Pata, K. (2018). Basic school teachers’ perspective to digital teaching and learning in Ghana. Education and Information Technologies, 23(3), 1159–1173. https://doi.org/10.1007/s10639-017-9660-8
- Rahi, S., Ghani, M., Alnaser, F., & Ngah, A. (2018). Investigating the role of unified theory of acceptance and use of technology (UTAUT) in internet banking adoption context. Management Science Letters, 8(3), 173–186.
- Ringle, C. M., Sarstedt, M., Sinkovics, N., & Sinkovics, R. R. (2023). A perspective on using partial least squares structural equation modelling in data articles. Data in Brief, 48, 109074.
- Ringle, C. M., Wende, S., & Becker, J.-M. (2015). SmartPLS 3. SmartPLS GmbH.
- Roberts, R., Flin, R., Millar, D., & Corradi, L. (2021). Psychological factors influencing technology adoption: A case study from the oil and gas industry. Technovation, 102, 102219. https://doi.org/10.1016/j.technovation.2020.102219
- Rogers, E. M. (1962). Diffusion of innovations. Free Press.
- Sarstedt, M., & Mooi, E. (2019). A concise guide to market research. The process, data, and methods using IBM SPSS statistics (2016 Saunders, M. N., & Townsend, K. eds, 3rd ed.) Springer.
- Saunders, M., Lewis, P., & Thornhill, A. (2009). Research methods for business students (5th ed.). Pearson Education.
- Shaikh, A., & Nikooherafmaher, S. “The effects of digitalization on students’ learning experience after the rise of the COVID-19 pandemic”: A qualitative study on institutional and student behaviours because of disruptive digitalization after the rise of the COVID-19 pandemic. 2022.
- Sharma, S. K., Gaur, A., Saddikuti, V., & Rastogi, A. (2017). Structural equation model (SEM)-neural network (NN) model for predicting quality determinants of e-learning management systems. Behaviour & Information Technology, 36(10), 1053–1066. https://doi.org/10.1080/0144929X.2017.1340973
- Shuhaiber, A. (2016). How facilitating conditions impact students’ intention to use virtual lectures? An empirical evidence. AICT, 2016, 79.
- Songkram, N., Chootongchai, S., Osuwan, H., Chuppunnarat, Y., & Songkram, N. (2023). Students’ adoption towards behavioural intention of digital learning platform. Education and Information Technologies, 1–23.
- Steil, A. V., de Cuffa, D., Iwaya, G. H., & Pacheco, R. C. D. S. (2020). Perceived learning opportunities, behavioural intentions and employee retention in technology organizations. Journal of Workplace Learning, 32(2), 147–159. https://doi.org/10.1108/JWL-04-2019-0045
- Streletskaya, N. A., Bell, S. D., Kecinski, M., Li, T., Banerjee, S., Palm‐Forster, L. H., & Pannell, D. (2020). Agricultural adoption and behavioral economics: Bridging the gap. Applied Economic Perspectives and Policy, 42(1), 54–66.
- Svenningsson, J., Höst, G., Hultén, M., & Hallström, J. (2021). Students’ attitudes toward technology: Exploring the relationship among affective, cognitive and behavioral components of the attitude construct. International Journal of Technology and Design Education, 32(3), 1–21. https://doi.org/10.1007/s10798-021-09657-7
- Tamilmani, K., Rana, N. P., & Dwivedi, Y. K. (2018). Mobile application adoption predictors: Systematic review of UTAUT2 studies using weight analysis. In Challenges and Opportunities in the Digital Era: 17th IFIP WG 6.11 Conference on e-Business, e-Services, and e-Society, I3E 2018, Kuwait City, Kuwait, October 30–November 1, 2018, Proceedings 17 (pp. 1–12). Springer International Publishing.
- Tamilmani, K., Rana, N. P., & Dwivedi, Y. K. (2019). Use of ‘habit’ is not a habit in understanding individual technology adoption: A review of UTAUT2 based empirical studies. In Smart Working, Living and Organising: IFIP WG 8.6 International Conference on Transfer and Diffusion of IT, TDIT 2018, Portsmouth, UK, June 25, 2018, Proceedings, Portsmouth, UK (pp. 277–294). Springer International Publishing.
- Tamilmani, K., Rana, N. P., & Dwivedi, Y. K. (2021). Consumer acceptance and use of information technology: A meta-analytic evaluation of UTAUT2. Information Systems Frontiers, 23(4), 987–1005. https://doi.org/10.1007/s10796-020-10007-6
- Tamilmani, K., Rana, N. P., Wamba, S. F., & Dwivedi, R. (2021). The extended unified theory of acceptance and use of technology (UTAUT2): A systematic literature review and theory evaluation. International Journal of Information Management, 57, 102269.
- Tarhini, A., Alalwan, A. A., Cao, D., & Al-Qirim, N. (2021). Integrating emotional attachment, resource sharing, communication and collaboration into UTAUT2 to examine students’ behavioural intention to adopt social media networks in education. International Journal of Technology Enhanced Learning, 13(1), 1–23. https://doi.org/10.1504/IJTEL.2021.111588
- Tarhini, A., Hone, K., Liu, X., & Tarhini, T. (2017). Examining the moderating effect of individual-level cultural values on users’ acceptance of E-learning in developing countries: A structural equation modeling of an extended technology acceptance model. Interactive Learning Environments, 25(3), 306–328. https://doi.org/10.1080/10494820.2015.1122635
- Taylor, S., & Todd, P. (1995). Decomposition and crossover effects in the theory of planned behavior: A study of consumer adoption intentions. International Journal of Research in Marketing, 12(2), 137–155.
- Thompson, R. L., Higgins, C. A., & Howell, J. M. (1991). Personal computing: Toward a conceptual model of utilization, 125–143.
- Twum, K. K., Ofori, D., Keney, G., & Korang-Yeboah, B. (2022). Using the UTAUT, personal innovativeness and perceived financial cost to examine student’s intention to use E-learning. Journal of Science and Technology Policy Management, 13(3), 713–737. https://doi.org/10.1108/JSTPM-12-2020-0168
- Utomo, P., Kurniasari, F., & Purnamaningsih, P. (2021). The effects of performance expectancy, effort expectancy, facilitating condition, and habit on behaviour intention in using mobile healthcare application. International Journal of Community Service & Engagement, 2(4), 183–197. https://doi.org/10.47747/ijcse.v2i4.529
- Van der Sluis, L. E., & Poell, R. F. (2003). The impact on career development of learning opportunities and learning behaviour at work. Human Resource Development Quarterly, 14(2), 159–179. https://doi.org/10.1002/hrdq.1058
- Venkatesh, V., Thong, J. Y., & Xu, X. (2012). Consumer acceptance and use of information technology: Extending the unified theory of acceptance and use of technology. MIS Quarterly, 157–178.
- Wang, F., Wijaya, T. T., Habibi, A., & Liu, Y. (2022). Predictors influencing urban and rural Area students to use tablet computers as learning tools: Combination of UTAUT and TTF models. Sustainability, 14(21), 13965. https://doi.org/10.3390/su142113965
- Yidana, M. B., & Arthur, F. (2023). Exploring economics teachers’ efficacy beliefs in the teaching of economics. Cogent Education, 10(1), 1–21. https://doi.org/10.1080/2331186X.2023.2222652
- Yidana, M. B., Arthur, F., Ababio, B. T., & Yu, Z. (2022). Teachers’ application of multiple intelligences approach in teaching economics. Education Research International, 2022(2875555), 1–16. https://doi.org/10.1155/2022/2875555
- Yidana, M. B., Arthur, F., Kaku, D. W., & Anti Partey, P. (2023). Predictors of economics students’ behavioural intention to use tablets: A two-staged structural equation modelling-artificial neural network approach. Mobile Information Systems, 1–20. https://doi.org/10.1155/2023/9629405
- Youssef, A., & Issam, E. I. (2022). Students’ adoption of e-learning: Evidence from a Moroccan business school in the COVID-19 era. Arab Gulf Journal of Scientific Research, 40(1). https://doi.org/10.1108/AGJSR-05-2022-0052 54–78
- Zacharis, G., & Nikolopoulou, K. (2022). Factors predicting University students’ behavioral intention to use eLearning platforms in the post-pandemic normal: An UTAUT2 approach with ‘learning value’. Education and Information Technologies, 27(9), 1–18. https://doi.org/10.1007/s10639-022-11116-2
- Zhang, L., & Nouri, J. (2018). A systematic review of learning and teaching with tablets. International Association for Development of the Information Society.
- Zhang, T., Tao, D., Qu, X., Zhang, X., Zeng, J., Zhu, H., & Zhu, H. (2020). Automated vehicle acceptance in China: Social influence and initial trust are key determinants. Transportation Research Part C: Emerging Technologies, 112, 220–233. https://doi.org/10.1016/j.trc.2020.01.027
- Zheng, J., & Li, S. (2020). What drives students’ intention to use tablet computers: An extended technology acceptance model. International Journal of Educational Research, 102, 101612. https://doi.org/10.1016/j.ijer.2020.101612