Abstract
The Severe Acute Respiratory Syndrome Coronavirus 2 (SARS-CoV-2) or popularly the Corona Virus Disease 2019 (COVID-19) pandemic brought significant public health challenges globally. During the crisis, mobile health (m-health) services were implemented to expedite fast, convenient and reliable dissemination of health information to the public. Meanwhile, the adoption of m-health faced high uncertainty alike other COVID-19 regulatory methods. This study examines the antecedents of m-health acceptance among Zimbabwean university students. Whilst research on m-health adoption during the pandemic is still scarce, this study becomes the first to integrate the Unified Theory of Acceptance and Use of Technology (UTAUT) and the Theory of Planned Behavior (TPB) to predict m-health adoption in a developing country. An explanatory design and a quantitative research approach were employed. Based on 271 valid responses, Structural Equation Modelling was used to estimate the proposed model. The findings revealed the positive effect of performance expectancy, effort expectancy, facilitating conditions, attitudes, behavioral norms, perceived behavioral control on behavioral intention to adopt m-health with a higher R square value (80.4%) than the original UTAUT model. The study also confirmed the positive influence of behavioral intention on m-health usage (R square = 41.5%). Health promoters were urged to design messages that stimulate positive attitudes, appeal to social groupings and improve user’s self-efficacy. M-health services should also foster use simplicity, ease of use and health value to entice m-health adoption among consumers. The study also provides an important baseline for the integration of behavioral theoretical models to enhance their predictive strength.
1. Introduction
Public health remains one of the critical concerns that continue to cripple governments and global health institutions in the quest for bio-physical sustainability. The global population embarks on a recovery trajectory as it rejuvenates from the jaws of the SARS-COV-2 outbreak, popularly known as the Corona Virus Disease 2019 or COVID-19 pandemic. The predicament of the COVID-19 pandemic has been well documented in recent public health research (Dangaiso et al., Citation2022; Dangaiso, Makudza, Jaravaza et al., Citation2023; Jaravaza et al., Citation2023; Puri et al., Citation2020; Shekhar, Citation2022). Although the epidemic curve has flattened, it follows the concerted efforts that were enforced by the World Health Organisation (WHO) through national health authorities. Vaccination, social distancing, COVID-19 screening, practicing good hygiene and wearing facemasks and gloves being the major ones (Dangaiso, Makudza, Tshuma, et al., Citation2023; Vasli et al., Citation2022).
In order to expedite public awareness and enhance access to COVID-19 information, governments adopted health education and awareness on mass communication platforms (Jaravaza et al., Citation2023). Information on COVID-19 statistics (infections, recoveries and deaths), immunization benefits, procedure and access and general health information related to COVID-19 was conveyed. Education on COVID-19 home-based care, self-care, fighting social media misinformation and general public awareness was conducted through national radio, mobile teams, television, print press, social media, and mobile phones via text messaging and applications (Pennycook et al., Citation2020; Puri et al., Citation2020). This research focuses on the antecedents of mobile health adoption in Zimbabwe during the COVID-19 pandemic. Mobile health (m-health) is defined as health intervention using mobile technologies such as mobile phones, wearable devices, personal digital assistants and tablets (Cho et al., Citation2018).
In a fast evolving digital age, much thrust has been put on transforming public health services using advanced Information Communication Technologies (ICTs) (Mathai et al., Citation2022). ICTs are defined as digitally enabled communication tools facilitate sharing or exchange of information (Said, Citation2022). The application of m-health has expanded from healthcare support (clinical decision support and electronic medical records) to health prevention, promotion, diagnosis and monitoring (Cho et al., Citation2018; Said, Citation2022). The global m-Health market size reached USD 50.7 billion in 2021 and is expected to expand at a growth rate of 11% from 2022 (Said, Citation2022). According to the Postal and Telecommunications Regulatory Authority of Zimbabwe (POTRAZ, Citation2023), the mobile penetration rate was 94.2% whilst the internet penetration rate was 65.4% by December 2022. Further, to confirm the expanding mobile communications infrastructure that supports mobile health, 98% of the active internet subscribers were on mobile (POTRAZ, Citation2023). Moreover, studies on services utilising mobile and digital servicescapes such as mobile banking (Manyanga et al., Citation2022), internet banking (Nyagadza et al., Citation2022), e-learning (Dangaiso et al., Citation2022; Dangaiso, Makudza, Jaravaza et al., Citation2023) and e-marketing (Manyanga, Citation2022) have been conducted from a Zimbabwean context.
Although COVID-19 control measures were largely communicated through national radio, social media, television and press, they faced protracted consumer resistance in most countries (Dangaiso, Makudza, Tshuma, et al., Citation2023; Jaravaza et al., Citation2023). Whilst consumer skepticism was evident in most regions (Dangaiso, Makudza, Tshuma, et al., Citation2023; Pennycook et al., Citation2020), no empirical studies have examined population response to mobile health during the COVID-19 era in most pre-emerging economies such as Zimbabwe. The purpose of this research was to examine the determinants of mobile health acceptance among Zimbabwean university students during the COVID-19 pandemic.
Extant literature concurs that human behavior is a complex construct that has seen several theoretical models expanded, integrated and re-developed by researchers with the goal of enhancing their predictive power e.g., Melzner et al. (Citation2014), Siripipatthanakul et al. (Citation2023) and Zhang et al. (Citation2020) in mobile health. Whilst these theoretical models have been constantly modified, Dwivedi et al. (Citation2019) notes that from the four exogenous factors in the Unified Theory of Acceptance and Use of Technology (UTAUT) model (Venkatesh & Davis, Citation2003), the key element missing is the ‘individual’ engaging the behavior. Across integrated models in empirical studies, the ‘technology oriented’ theories e.g., the Technology Adoption Model (TAM) (Davies, Citation1989) and UTAUT (Venkatesh & Davis, Citation2003) have focused on the key capabilities of the new technology. Meanwhile, the core ‘behavioral’ theories such as the Theory of Reasoned Action (TRA) (Ajzen & Fishbein, Citation1980) and the Theory of Planned Behavior (TPB) (Ajzen, Citation1991) measured the cognitive and socio-psychological aspects of human behavior (Cho et al., Citation2018; Dwivedi et al., Citation2019; Rahman et al., Citation2017; Siripipatthanakul et al., Citation2023). In contrast to the UTAUT, the TPB and TRA are prototypical binary theories employed to illuminate and predict human behavior from the lens of individual consciousness without any specific suggestions on marketing of technology products (Arkorful et al., Citation2022, Citation2023; Jen et al., Citation2009).
Research evidence confirms that the UTAUT and the TPB have been merged to enhance their exactitude and power of prediction on human behavior by integrating their conceptually distinct constructs. E.g., Al-Gahtani (Citation2006) in Information Technology (IT) adoption, Chuang et al. (Citation2018) in pro environmental behavior, Huang (Citation2023) in virtual reality tourism, Rahman et al. (Citation2017) in advanced driver assistance systems, Rejali et al. (Citation2023) in fully automated vehicles and Siripipatthanakul et al. (Citation2023) in telemedicine. Meanwhile, research examining m-health adoption in the COVID-19 era is still scarce especially in developing economies. Based on this evidence, this study proposes a research model that predicts m-health adoption in Zimbabwe by integrating constructs of the Unified Theory of Acceptance and Use of Technology (UTAUT) (Venkatesh & Davis, Citation2003) and the Theory of Planned Behavior (TPB) (Ajzen, Citation1991). However, only subjective norms (TPB) and social influence (UTAUT) were not used together since they are conceptually similar (Cho et al., Citation2018; Dwivedi et al., Citation2019).
The significance of this research stems from its potential to inform public health authorities, health professionals, m-health marketers and even the corporate world on the determinants of m-health adoption. This study brings evidence of the importance of technological, social and individual factors that drive acceptance of m-health. This has the potential to inform their strategy as they envisage the application of mobile messaging and applications to their intended audiences. Given the exponentially rising public health costs in developing countries, this study may further inform health authorities on key determinants of m-health acceptance; as they envisage m-health technology as a process improvement and cost management strategy in public health. Further, the study validates an integrated model using two fundamental theories that have been empirically validated in many research contexts. Given that, the study informs future researchers on the applications and limitations of the two frameworks, hence avenues for employing integrated models to deepen their understanding of consumer behavior in the domain of health technologies. The subsequent sections of this research paper focus on literature review, materials and methods, results, discussion and conclusions.
2. Literature review
2.1. Theoretical foundations and conceptualisation
This study adopts the Unified Theory of Acceptance and Use of Technology (UTAUT) (Venkatesh & Davis, Citation2003) and the Theory of Planned Behavior (TPB) (Ajzen, Citation1991). According to the UTAUT, performance expectancy, effort expectancy, facilitating conditions and social influence have an influence on behavioral intention, which subsequently affects behavior. Performance expectancy was defined as the individual perception that the use or execution of a task using a prescribed technology delivers the intended or desired level of performance (Venkatesh & Davis, Citation2003). If an individual believes that using m-health provides important benefits, that person is likely to develop an intent to adopt m-health that will facilitate m-health acceptance (Galura et al., Citation2022).
Effort expectancy was conceptualized as the belief that using a certain technology is free of effort, easy or without obstacles (Venkatesh & Davis, Citation2003). Borrowing from the original Technology Acceptance Model (TAM) Davies (Citation1989), effort expectancy resonates with ‘perceived ease of use.’ Effort expectancy refers to the user’s perception that using a technology will not encapsulate operational difficulties. Similarly, if a person believes that the use of m-health services does not imply material end user challenges, the person is likely to have an intent to adopt and subsequently adopt (Galura et al., Citation2022; Winkle et al., Citation2019).
Facilitating conditions were defined as the availability of motivating factors in the environments that stimulate the intention to adopt a technology (Venkatesh & Davis, Citation2003). If a person perceives that there are more facilitating conditions in the adoption of m-health, that person is likely to accept m-health. Social factors refer to the influence of peers, family members of simply friends and referral groups that approve or disapprove behavior (Chen & Chang, Citation2013; Galura et al., Citation2022). If a student’s peers encourage m-health usage, that person is more apt to adopt m-health usage.
According to the Theory of Planned Behavior (TPB) (Ajzen, Citation1991), individual attitudes have an influence on intention to adopt a behavior and hence behavior. Attitudes are inherent personal dispositions to like or dislike an individual, product, entity or technology (Schiffman & Kanuk, Citation1994). Whether the attitude is positive or negative, the theoretical consensus is that an attitude will affect a certain behavior (Ajzen, Citation1991, Dilotsotlhe & Akbari, Citation2021; Purwanto et al., Citation2022). A positive attitude towards m-health also implies development of intent to accept it and hence acceptance.
Secondly, subjective norms were also theorized to influence behavioral intention (Ajzen, Citation1991). Subjective norms resonate with the social factors explained in the UTAUT theory (Venkatesh & Davis, Citation2003) above. They refer to unwritten social codes of behavior that regulate group and individual behaviors. Group influences have an impact on individual choices and behaviors because humans naturally seek validation or social approval from their referral groups (Dilotsotlhe & Akbari, Citation2021; Umar et al., Citation2021). Thus, the research model adopted only subjective norms because conceptually they refer to the same concept with social factors.
Perceived behavioral control was defined as the individual belief that they are able to successfully execute a prescribed course of action (Ajzen, Citation2020). In other theories such as the Health Belief Model (Rosenstock, Citation2000), it was conceptualized as self-efficacy. If one perceives that they are more likely to execute the behavior without confronting significant challenges, they are more likely to accept the behavior (Ajzen, Citation1991, Citation2020). If a student perceives m-health to be easy to use, they are more apt to adopt it. Perceived behavioral control also accounts for post adoption efficacy within one’s self (Mathai et al., Citation2022; Purwanto et al., Citation2022). If a person lacks the conviction that they would be able to continue with their daily routines well, they are unlikely to adopt the new technology.
2.2. Development of hypotheses
Significant empirical research has reported the importance of performance expectancy in influencing user behavioral intentions with technology products (Dwivedi et al., Citation2019). Studies in the context of mobile health have also reported the positive effect of performance expectancy and behavioral intention (Alaiad et al., Citation2019; Alam et al., Citation2020; Ndayizigamiye & Maharaj, Citation2016; Siripipatthanakul et al., Citation2023). If university students believe that m-health delivers important health benefits, they are expected to adopt m-health during the COVID-19 era in Zimbabwe. Given that, the following hypothesis was formulated;
H1: Performance expectancy positively influences behavioral intention to adopt m-health by Zimbabwean university students.
The relationship between effort expectancy and behavioral intention has been found to be positive in most technology adoption studies. Potential users have a high likelihood to adopt a new technology if it is easy to use or if it is free of effort (Venkatesh & Davis, Citation2003). Rahman et al. (Citation2017) and Rejali et al. (Citation2023) reported that effort expectancy positively influenced users’ intentions to use advanced driver assistant systems and fully automated vehicles, respectively. Similarly, the positive relationship between effort expectancy and behavioral intention was evidenced in several m-health studies e.g., Alaiad et al. (Citation2019), Alam et al. (Citation2020) and Siripipatthanakul et al. (Citation2023). Given that, this study predicted that effort expectancy would positively affect students’ intentions to use m-health during COVID-19. Consequentially, the following hypothesis was proposed;
H2: Effort expectancy positively influences behavioral intentional to adopt m-health by Zimbabwean university students.
The relationship between facilitating conditions and behavioral intention has been examined in vast empirical studies; with the positive effect of facilitating conditions well supported (Alam et al., Citation2020; Ndayizigamiye & Maharaj, Citation2016; Rahman et al., Citation2017; Rejali et al., Citation2023; Siripipatthanakul et al., Citation2023). The environment in which the new technology is delivered for use should be conducive enough to entice adoption. E.g., m-health would be easier to use where mobiles phones are affordable, power is available as well as reliable internet. In the context of the COVID-19, facilitating conditions are expected to have a significant effect on students’ m-health adoption intentions. Against the foregoing, the study predicted the following hypothesis;
H3: Facilitating conditions positively influences behavioral intention to adopt m-health by Zimbabwean university students.
Based on the Theory of Planned Behavior (TPB), attitudes have a key antecedent role on behavioral intention (Ajzen, Citation1991, Citation2020). A positive attitude towards a new technology has a high propensity to motivate its adoption. Prior studies in diverse subjects have reported the positive effect of attitude on behavioral intentions (Dangaiso, Citation2023; Dilotsotlhe & Akbari, Citation2021; Purwanto et al., Citation2022). Further, researchers who extended the TPB (Ajzen, Citation1991), with the UTAUT (Venkatesh & Davis, Citation2003) and related models have also reported the positive and significant effect of attitudes on behavioral intent (Arkorful et al., Citation2022; Dwivedi et al., Citation2019; Rahman et al., Citation2017; Rejali et al., Citation2023; Siripipatthanakul et al., Citation2023). Leaning on their findings, this study formulated the following hypothesis in the context of m-health adoption during COVID-19;
H4: Attitudes positively influences m-health behavioral intention to adopt m-health by Zimbabwean university students.
Subjective norm has shown significant evidence of the strength of peer support in individual behaviors (Ajzen, Citation1991). It is the conceptual equivalent of social influence based on the UTAUT (Cho et al., Citation2018; Dwivedi et al., Citation2019). Thus, in this research, only subjective norm was adopted. Empirical research has shown that group influences have key effects on behavioral intention (Dilotsotlhe & Akbari, Citation2021; Purwanto et al., Citation2022). Researchers who have examined behavioral intention using the combined TPB-UTAUT framework have also reported a positive influence of subjective norm on behavioral intention (Al-Gahtani, Citation2006; Rejali et al., Citation2023; Siripipatthanakul et al., Citation2023). If most students in a class or a group of friends have a positive attitude towards m-health, it becomes an easier choice for other members of the class to adopt it. Therefore, in the context of mobile health adoption during COVID-19, this study predicted that;
H5: Subjective norms positively influences m-health behavioral intention to adopt m-health by Zimbabwean university students.
Perceived behavioral control was the only addition to the Theory of Reasoned Action (TRA) (Ajzen & Fishbein, Citation1980) that culminated into the TPB (Ajzen, Citation1991). Consumers self evaluate their conviction to successfully execute a behavior prior to adoption (Ajzen, Citation2020). The positive relationship between perceived behavioral control and behavioral intentions has been evident in extant health behavior literature (Arkorful et al., Citation2022; Dangaiso, Citation2023; Dangaiso, Makudza, Tshuma, et al., Citation2023; Siripipatthanakul et al., Citation2023). If potential users believe that they can easily use m-health, that triggers adoption intentions. Similarly, if students believe that they have the requisite knowledge, technical skills, mental fortitude to learn COVID-19 information on mobile devices, they are likely to develop intent to adopt it. As a result, the study hypothesized that;
H6: Perceived behavioral control positively influences m-health behavioral intention to adopt m-health by Zimbabwean university students.
Theoretically, both the TPB and UTAUT concur that behavioral intent precedes actual behavior (Ajzen, Citation1991, Citation2020; Venkatesh & Davis, Citation2003). Significant empirical research has shown the positive relationship between behavioral intent and the subsequent behavior (Alaiad et al., Citation2019; Alam et al., Citation2020; Dangaiso, Citation2023; Dilotsotlhe & Akbari, Citation2021; Siripipatthanakul et al., Citation2023). This implies that if a student intends to adopt mobile health, she or she proceeds to adopt it. Consequentially, the ultimate hypothesis of the study predicted that;
H7: Behavioral intention to adopt m-health positively influences m-health usage behavior.
illustrates the hypothesized research model.
Figure 1. Hypothesized research model.
Source: Developed from Ajzen (Citation1991) and Venkatesh and Davis (Citation2003).
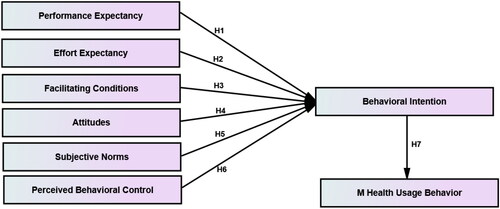
3. Materials and methods
An explanatory research design was adopted in line with the positivism research paradigm (Saunders et al., Citation2018). This research design is employed where researchers attempt to predict or explain causal relationships in a hypothesized research model. Further, the target population of the study were active students enrolled at three public universities in Zimbabwe. Young adults have been targeted by concerted health research as key stakeholders in the promotion of bio-physical sustainability (Dangaiso, Citation2023; Muposhi et al., Citation2015). Ethical approvals that granted the researcher access to research participants were obtained. More so, participants were informed of the purpose of the research, clear instructions not to provide their personal identity, right to freedom of participation and withdrawal from the study and also that no harm would be there as a result of the research.
In terms of instrumentation, the data collection questionnaire items were adopted from extant literature (Saunders et al., Citation2018). These were modified from studies that used the UTAUT and Theory of Planned Behavior to account for adoption behaviors e.g., Chen and Chang (Citation2013), Dangaiso (Citation2023), Dilotsotlhe and Akbari (Citation2021), Galura et al. (Citation2022), Purwanto et al. (Citation2022) and Siripipatthanakul et al. (Citation2023). The variables were conceptualized as performance expectancy (3 items), effort expectancy (4 items), facilitating factors (3 items), attitudes (3 items), subjective norms (4 items), perceived behavioral control (3 items), behavioral intention (3 items) and m-health usage behavior (3 items). A pretest was conducted with 8 students from a local university and the scale was also deemed appropriate by a panel of experts. These constructs attained good internal reliability scores that were all above the Cronbach alpha threshold of 0.7. Apart from the study constructs, the questionnaire had a demographic section that also addressed individual m-health awareness and use, health consciousness and self-regulation.
Data was collected when the major COVID-19 risk factors had subsided (July 2023) hence paper printed structured questionnaires were used. Adoption of an online survey was discouraged by low response rates inherent in virtual surveys (Amin, Citation2016; Herington & Weaven, Citation2009). From a population of 12,394 undergraduate students, the proportionate distribution method was employed to determine sub-samples from the three campuses. Due to challenges with obtaining a proper sampling frame, convenience sampling was employed to collect data from students who were available (Saunders et al., Citation2018). 391 questionnaires were delivered based on sample sizes used in similar studies, population size, data analysis methods, completion rates and resource constraints (Malhotra et al., Citation2017). 305 responses were returned, a response rate of 78%. Data cleaning (21) and preliminary analyses (13), eliminated 34 cases and 271 were deemed valid. Data cleaning is the process of deleting misaligned responses that reflect huge disparities from normality. 13 responses furthest from the centroid were also eliminated for outlier bias based on the Mahalanobis distance (Tabachnick & Fidell, Citation2013).
Structural Equation Modelling in SPSS AMOS was used to estimate model parameters. Based on the Maximum Likelihood estimation, the statistical results were the basis for testing research hypotheses (Anderson & Gerbin, Citation1988; Kline, Citation2023). Convergent validity was tested using Average Variance Extracted and discriminant validity was based on the comparison between the square root of the Average Variance Extracted and construct correlations (Hair et al., Citation2019). Construct reliability was also verified using Composite reliability. To evaluate the appropriateness of the measurement and structural models, absolute and incremental fit indices were used (Kline, Citation2016). The Chi square (CMIN), normed Chi square (x2/df), Root Mean Square Error of Approximation (RMSEA), Goodness of Fit Index (GFI) and the Root Mean Residual (RMR) were used as the absolute fit indices. The Normed Fit Index (NFI), Tucker-Lewis Index (TLI), Incremental Fit Index (IFI) and Comparative Fit Index (CFI) were the incremental or relative fit indices used in this study.
4. Results
4.1. Demographic profile
The sample characteristics of the participants were analysed. The major highlights were that 49.4% were females and 50.6% were males. All participants (100%) confirmed their use of a mobile device. Further, 98.9% confirmed that they received mobile health services whilst 98.2% indicated they adopted at least one m-health application during the COVID-19 pandemic. illustrates the full demographic profile for the study.
Table 1. Demographic profile.
4.2. Assessment of the measurement model
The measurement model was evaluated using a Confirmatory Factor Analysis (CFA) procedure (Anderson & Gerbin, Citation1988; Hair et al., Citation2019). It began with the assessment of unidimensionality of factor loadings. Unidimensionality examines the extent to which observed variables represent the variance in the parent construct and this is measured using factor loadings (Hair et al., Citation2019). A factor loading is a representation of the correlation between an observed variable and the latent factor (Kline, Citation2023). All observed variables had loadings above 0.5 as recommended by Hair et al. (Citation2019) except EE4 (0.485) and SN4 (0.450). Items with loadings of at least 0.5 confirm that they explain at least 50% of the variance in the parent construct (Byrne, Citation2013; Hair et al., Citation2019). Consequentially, EF4 and SN4 were dropped from further analysis.
Further, the fit between the hypothesized measurement model and the sample-implied model were examined. The results indicated that the model had very good attainment of both absolute and relative fit indices. In absolute fit indices, the normed Chi square was 3.5 whilst the Goodness of Fit (GFI) = 0.912, Root Mean Square Error of Approximation (RMSEA) = 0.06 and Root Mean Residual (RMR) = 0.06. In relative fit, the Comparative Fit Index (CFI) was 0.960 whilst the Incremental Fit Index (IFI) was 0.970, Tucker-Lewis Index (TLI) = 0.968 and the Normed Fit Index (NFI) = 0.972. According to Byrne (Citation2013), Hair et al. (Citation2019) and Schumacker and Lomax (Citation2010), the measurement model had a very good fit.
Third, validation of measurement scales was done based on their convergent validity, discriminant validity, Cronbach’s alpha and composite reliability (Hair et al., Citation2019; Kline, Citation2023). Convergent validity measures the extent to which measures of the same construct share common variance (Hair et al., Citation2020). In this study, Average Variance Extracted (AVE) was used and each construct should have at least 0.5 to confirm convergent validity (Hair et al., Citation2019). As shown in , AVE values ranged from 0.556 to 0.811, confirming that convergent validity was present.
Table 2. Psychometric properties of the measurement model.
The criteria by Fornell and Larcker (Citation1981) establishes that the square root of the Average Variance Extracted should be greater than the correlation between a construct and any other construct in the model. The requirement of discriminant validity was satisfied. Internal consistency was examined using Cronbach alpha (0.7) and these ranged from 0.756 to 0.927. Composite reliability (0.7) also confirmed the results (as recommended in Structural Equation Modelling) (Kline, Citation2016, Citation2023) and the values ranged from 0.782 to 0.928. According to Nunnally and Bernstein (Citation1994), construct reliability was attained. shows the psychometric properties of the measurement model.
4.3. Structural equation modelling and hypothesis testing
Validation of the measurement model implies that the analysis proceeds to Structural Equation Modelling (SEM) (Anderson & Gerbin, Citation1988; Schumacker & Lomax, Citation2010). SEM is an advanced estimation method that combines Confirmatory factor analysis, path analysis and regression analysis to estimate structural relationships in hypothesized models (Byrne, Citation2013; Hair et al., Citation2019; Schumacker & Lomax, Citation2010). Prior, the assumptions of data normality and multicollinearity were examined.
The skewness and kurtosis values did not surpass the −2 to +2 and −3 to +3 ranges, respectively, thus univariate normality was there (Hair et al., Citation2019). The Mardia’s coefficient (103.4) was below the p(p + 2) value (624) that is recommended, where p is the number of observed variables (p = 24) (Raycov & Malcoulides, Citation2008). Thus, multivariate normality was present. Further, the Tolerance (T) and Variance Inflation Factor (VIF) were 0.58 and 3.05 and these should be above 0.25 and not greater than 5, respectively (Hair et al., Citation2019; Tabachnick & Fidell, Citation2013). Given the results, there was no statistical evidence of the presence of multicollinearity, therefore, the examination of the structural model proceeded ().
Figure 2. The structural model.
Notes: Perf: performance; Perc: perceived; Facilita: facilitating; Beh: behavioral; Usa_Beh: usage behavior.
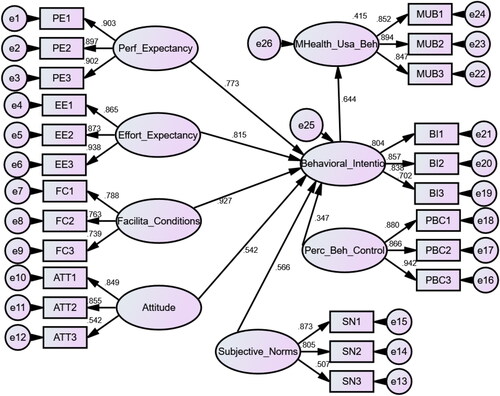
The model fit of the structural model was examined. The normed chi square was 3.8, thus within the 2-5 threshold as recommended by Hair et al. (Citation2019) and Schumacker and Lomax (Citation2010). Further, the Goodness of Fit Index (GFI) = 0.910, Root Mean Residual (RMR) = 0.06 and the Root Mean Square Error of Approximation (RMSEA) =0.07. The Comparative Fit Index (CFI) = 0.968, Incremental Fit Index = 0.950 whilst the Tucker-Lewis Index (TLI) = 0.968. The threshold of 0.9 is acceptable whilst 0.95 is perfect, thus the fit indices were very good. Given these results, the model obtained a very good fit (Byrne, Citation2013; Hair et al., Citation2019; Schumacker & Lomax, Citation2010). Subsequently, path estimates, t-statistics and p-values were examined as the basis to hypothesis testing. A t-value is the ratio between a path estimate and its standard error (Hair et al., Citation2019). At 95% confidence interval, t-statistics between −1.96 and +1.96 denote statistically significant relationships. At the same confidence interval, p-values less than 0.05 also denote statistically significant relationships (Hair et al., Citation2019; Kline, Citation2023).
The first hypothesis (H1) proposed that performance expectancy has a positive influence on behavioral intention to adopt m-health by Zimbabwean university students. The results indicated that β = 0.773, t = 18.023 and p < 0.001. Given these results, H1 received empirical support and this means that the positive student perception on the ability of m-health messages and applications to fulfill their purpose and provide invaluable COVID-19 information had a positive impact on the intent to adopt m-health.
In H2, the study had hypothesized that effort expectancy would positively influence behavioral intentions of university students to adopt m-health. The results of SEM confirm that the effect of effort expectancy on behavioral intention to adopt m-health was positive and statistically significant. This was evidenced by a beta (β) of 0.815, a t-value of 16.320 and a p-value <0.001. These results substantiate that positive perceptions of the ease of using m-health positively influenced the behavioral intentions to adopt m-health during the COVID-19 pandemic. Consequentially, H2 was supported.
Further, H3 also proposed the positive impact of facilitating conditions on university students’ intentions to accept m-health. The results indicated that facilitating or stimulating factors positively affected behavioral intentions of students to adopt m-health services (β = 0.927, t = 16.818 and p < 0.001). As a result, H3 was accepted. The presence of encouraging environmental factors such as accessibility of mobile devices, reliable electricity and accessible internet services are suggestive of the students’ behavioral intentions to adopt m-health during the COVID-19 era.
The study also predicted the positive influence of individual attitudes on the behavioral intention of university students to adopt mobile health services (H4). As shown on the SEM path diagram, a standardized estimate of 0.542, a t-value of 10.666 and a p-value <0.001 confirms that the effect was positive and statistically significant. Based on these results, H4 was supported. This implies that positive attitudes towards mobile health services influence university students’ intentions to accept m-health in the COVID-19 era.
The study had hypothesized that subjective norms would positively influence behavioral intentions of university students to adopt m-health. The results of SEM confirm that the effect of subjective norms on behavioral intention to adopt m-health was positive and statistically significant. This was evidenced by a beta (β) of 0.566, a t-value of 12.954 and a p-value <0.001. These results support that positive perceptions of peer or group approval for choosing to use m-health positively influenced students’ behavioral intentions to adopt m-health during the COVID-19 pandemic. Subsequently, H5 gained empirical support.
More so, the sixth hypothesis (H6) predicted that perceived behavioral control has a positive influence on behavioral intentions of Zimbabwean university students to adopt m-health. The results indicated that β = 0.347, t = 6.509 and p = 0.01. Informed by these results, H6 was accepted. This confirms that the positive student perception of their ability to perform important functions on the mobile health applications, retrieve invaluable COVID-19 information and comprehend m-health messages delivered to them had a positive impact on the intentions to adopt m-health. Further, the integrated model (predictors in H1–H6) explained significant variability in behavioral intentions to accept mobile health services (80.4%).
The ultimate hypothesis of this research predicted that behavioral intentions of university students to adopt m-health positively influences their m-health usage behavior, acceptance or adoption (H7). The results from SEM indicated that this relationship was positive and statistically significant. This was evidenced by a beta (β) value of 0.644, a t-statistic of 15.804 and a p-value <0.001. The results support that behavioral intention to accept m-health is a key determinant of actual m-health adoption or usage. Behavioral intention accounted for 41.5% of the variability in mobile health usage behavior or adoption. illustrates the results of hypothesis testing.
Table 3. Outcomes of hypothesis testing.
4.4. Discussion of findings
The proposed research model was estimated using Structural Equation Modelling and 7 hypotheses were examined and supported. H1 had proposed that performance expectancy would positively influence behavioral intention to adopt m-health. Given the results (β = 0.773, t = 18.023 and p < 0.001), this hypothesis was confirmed. The findings reflect that when users perceive m-health to be able to deliver the desired results, mobile users are likely to develop an intention to adopt it. The impact of performance expectancy on behavioral intention to adopt or accept new products has been confirmed in previous studies, for example, Alaiad et al. (Citation2019), Alam et al. (Citation2020), Galura et al. (Citation2022), Rejali et al. (Citation2023), and Siripipatthanakul et al. (Citation2023). Further, in the context of this research, these results encapsulate that the more university students believe in the ability of the m-health messages and applications to effectively provide valid, reliable and accurate health information about COVID-19, the more they develop intentions to accept m-health. As a result, perceived performance expectancy had a positive influence on the acceptance of m-health by university students in Zimbabwe during the COVID-19 pandemic.
H2 proposed that effort expectancy would positively impact behavioral intentions to adopt m-health among university students in Zimbabwe. The results indicate that the causal effects were positive and statistically significant (β = 0.815, t = 16.320 and p < 0.001). The findings confirm that the perceptions that m-health is easy and effort free to adopt encourage university students to develop an intention to accept m-health. More so, similar effects of effort expectancy on behavioral intention to adopt new technology have been observed in the empirical studies by Alam et al. (Citation2020), Rahman et al. (Citation2017) Chen and Chang (Citation2013) and Dwivedi et al. (Citation2019). These results could be explained by expectations by technology users that new technology should be free of technical sophistication and complexity. In vast technology acceptance studies, effort expectancy has been an important determinant of intention to adopt new products and services. In the original Technology Adoption Model (TAM), Davies (Citation1989), it was conceptualized as perceived ease of use and many researchers have confirmed its impact on adoption of new technology. Interestingly, this study adds to the evidence that the better the effort expectancy, the higher the propensity that students develop motivations to accept mobile health usage.
The study also proposed that facilitating conditions would also positively influence behavioral intention to adopt m-health in H3. The results confirm that the causal effects were positive and statistically significant (β = 0.927, t = 16.818 and p < 0.001). Facilitating conditions are factors that would motivate, encourage, or stimulate the adoption of mobile health technology. These include easy access to mobile devices, mobile network services, unlimited internet broadband services, financial resources, reliable power and availability of reliable m-health service providers or accurate health information and media. The availability of these factors positively correlated with consumer aptness to adopt m-health in university students. Prior research also confirms the positive influence of facilitating conditions on behavioral intentions to adopt new technology in diverse perspectives (Chuang et al., Citation2018; Dwivedi et al., Citation2019; Rejali et al., Citation2023; Siripipatthanakul et al., Citation2023). Availability of pull factors to motivate technology usage explains why facilitating conditions have a strong causal influence on behavioral intention to adopt mobile health among Zimbabwean university students.
In H4, the study predicted that individual attitudes have a positive effect on behavioral intention to adopt m-health among university learners. Attitudes are a well documented antecedent of intention to adopt a new behavior in behavioral studies. The results of path estimation (β = 0.542, t = 10.666 and p < 0.001) reflected that positive individual attitudes positively influenced intention to accept m-health. This implies that when students have a positive attitude towards adoption of tech savvy health platforms and services, they are more likely to develop positive emotions towards using that technology. As a result, H4 gained empirical support. The findings have been supported by the studies of Alam et al. (Citation2020) and Alaiad et al. (Citation2019) in mobile health, Dangaiso (Citation2023) in organic food consumption, Dilotsotlhe and Akbari (Citation2021) in green appliances, Purwanto et al. (Citation2022) in Islamic microfinance and Siripipatthanakul et al. (Citation2023) in telemedicine. In this context, these the findings could be attributed to the popularity and mass adoption of self-service and digital servicescapes amid enforced social distancing during the COVID-19 era. Thus, university learners were more e-ready to adopt mobile health as an important source of health information, thus a positive attitude triggered m-health usage.
The integrated model also hypothesized that subjective norms have a positive impact on behavioral intentions to adopt mobile health in H5. The results (β = 0.566, t = 12.954 and p < 0.001) confirm that group influences and the unwritten powers of social validity have a strong effect on behavioral intentions to adopt m-health among university students. Further, the results have been confirmed in prior research (e.g., Dangaiso (Citation2023), Mathai et al. (Citation2022), Siripipatthanakul et al. (Citation2023) and Vasli et al. (Citation2022). In this perspective, most university students are in the Generation Z population cohort, a dynamic yet complex demographic category with unique and well defined rules that define their relationships with the outer world. In this view, the power of positive groupthink towards the adoption of health technology explains the findings obtained in this research. Students are more apt to adopt behaviors that make them earn positive image, peer validation and social prestige in the student community.
The 6th hypothesis predicted that perceived behavioral control has a positive effect on students’ behavioral intentions to accept mobile health services. The results confirm that the relationship was positive and statistically significant (β = 0.347, t = 6.509 and p = 0.01). Confirmation of H6 entails that the ultimate predictor of behavioral intent to accept m-health was validated. This also supports that the framework was empirically sound and all predicted effects were confirmed by sample data. Further, the findings have been reported in previous research studies (Chuang et al., Citation2018; Dilotsotlhe & Akbari, Citation2021; Rejali et al., Citation2023; Siripipatthanakul et al., Citation2023). In this research, the importance of perceived self-efficacy (Dangaiso, Makudza, Tshuma, et al., Citation2023) on intention to use m-health was positively confirmed. Students in Higher Education are expected to have high self endowed abilities to execute and manage advanced technologies. High perceived behavioral control reflects on the confidence that a potential m-health user has on their ability to adopt and decode important meaning from digital health access. Altogether, the proposed determinants of behavioral intent to accept mobile health services explained a significant 80.4% variance in the criterion variable (behavioral intention to adopt m-health), confirmatory of the predictive validity of the structural model.
The ultimate hypothesis, H7, predicted that behavioral intention to adopt or accept m-health usage would have a positive effect on mobile health adoption. Without the eventual contribution to adoption of m-health, the model would not make any scientific relevance. Interestingly, the relationship between behavioral intention to accept m-health and m-health adoption or usage behavior was positive and statistically significant (β = 0.644, t = 15.804 and p < 0.001). As confirmed in a host of empirical studies, e.g., Chen and Chang (Citation2013), Dangaiso (Citation2023), Dilotsotlhe and Akbari (Citation2021), Dwivedi et al. (Citation2019), Purwanto et al. (Citation2022), and Siripipatthanakul et al. (Citation2023), behavioral intent precedes actual behavior. However, an important transition between behavioral intent and actual behavior has been reiterated in behavioral literature especially in health research (Davari & Strutton, Citation2014; Johnstone & Tan, Citation2015). This research validates the proposed model and behavioral intention managed to account for 41.5% of the variability in m-health adoption or usage behavior.
However, although the study was conducted post the major COVID-19 risk factors, potential limitations of the time period cannot be overlooked. Due to the magnitude of fatality that was associated with the pandemic, this could have influenced the students’ decisions on mobile health adoption. This has a potential impact on the generalizability of the findings generated in this research. Further, this sample was selected based on a non-probabilistic sampling method through convenience sampling. Whilst convenience sampling serves surveys where proper sampling frames are unavailable, there is a limitation on the findings on this research compared with related findings discussed in this section. Whilst the model achieved coefficient of determination from its robustness, the sampling approach presents a limitation compared to similar studies that used probability-sampling methods.
Whilst the integrated model was able to predict students’ intentions and adoption of mobile health services, this study also discusses the applications and limitations of these two theoretical models. The study provides a proxy for substantiating an argument that the models cannot independently explain human behavior in across cultures and contexts. Human behavior has shown over a range of theories that it cannot be intuitively predicted by a few variables e.g., attitudes, subjective norms and perceived behavioral control based on the TPB (Cho et al., Citation2018; Dwivedi et al., Citation2019). Although the UTAUT and TPB are products of theoretical evolution over time, these theoretical developments are evidence that these frameworks cannot independently and sufficiently measure human intentions to adopt a behavior (Moták et al., Citation2017). The theoretical integration in this study serves as an impetus for future research in developing economies to expand and integrate these behavioral frameworks with the goal of improving their predictive validity. While the TPB focuses on the cognitive and psychological aspects of human behavior, the UTAUT provides a measurement on the capabilities of the new technology in motivating consumer adoption.
Further, the applicability of these models may differ across different cultures e.g., factors such as social factors, subjective norm may reflect different results between individualistic and collectivist cultures (Leong et al., Citation2022). Individual attitudes (TPB) may have a less impact in collectivist than individualistic cultures as well. How one copes with complex behaviors (perceived behavioral control) may be subject to cultural orientation (Leong et al., Citation2022), for example individualistic cultures emphasise individual self-efficacy in complex situations while collectivism encourages group problem solving. As a result, these models can reflect different outcomes when applied to respondents who have diverse cultural origins, thus the applicability of these theories is subject to cultural orientation.
5. Conclusions, implications and limitations
5.1. Conclusions
The objective of the research was to examine the antecedents of mobile health (m-health) services among university students during the COVID-19 pandemic. Based on the findings from the integrated framework, this study concludes that performance expectancy, effort expectancy, facilitating conditions, individual attitudes, subjective norms and perceived behavioral control are important determinants of the behavioral intentions of health consumers to adopt m-health. Subsequently, behavioral intention to adopt m-health has a positive direct effect on m-health adoption or usage behavior. The power of prediction with integrated models is also brought to evidence in a health context of a developing country. The predictors had 80.4 and 41.5% variability in behavioral intention to adopt m-health and m-health usage explained, respectively. This confirms important predictive synergies between the Unified Theory of Acceptance and Use of Technology (UTAUT) (Venkatesh & Davis, Citation2003) and the Theory of Planned of Planned Behavior (TBP) (Ajzen, Citation1991) in the context of technology based health products and services.
5.2. Practical implications
The study evaluated the predictors of mobile health (m-health) acceptance among university students in the context of the COVID-19. Given the positive influences of performance expectancy, effort expectancy, facilitating conditions, attitudes, subjective norms and perceived behavioral control, the findings bear significant practical relevance to stakeholders in the health fraternity. The findings may help m-health marketers in devising their marketing strategies needed to develop rational and emotional health appeals to the targeted segments. This can take the form of developing positive attitudes towards m-health use, fostering positive group influences on m-health and improving individual self-efficacy by crafting health marketing messages that improve consumers’ perceived behavioral control.
M-health marketers may also learn from the study that health consumers perceived value on m-health adoption hinges on perceived ease of use (effort expectancy), perceived value proposition (performance expectancy) and also the availability of motivating, stimulant or encouraging factors (facilitating conditions). The study may also enlighten health authorities on the critical determinants of m-health use so that they allocate resources needed to disentangle barriers to acceptance of health technology. This research may also assist community health providers and auxiliary health organizations to be equipped with counter marketing strategies should they target mobilizing health consumers towards adoption of health services or advanced technological interventions needed to advance population longevity.
5.3. Theoretical implications
This study was grounded in two fundamental behavioral theoretical frameworks that attempt to predict human behavior, the Unified Theory of Acceptance and Use of Technology (UTAUT) and the Theory of Planned Behavior (TPB). The integrated framework predicted 80.4% variability in behavioral intention to adopt m-health and consequentially 41.5% of the variability in m-health adoption. The fundamental propositions espoused in the two theoretical frameworks were confirmed. Therefore, this study provides an empirical confirmation for the explanatory validity of the two theories in predicting consumer usage of health technologies from the context of COVID-19.
Further, the study also provides the current applications and limitations of the two theoretical models in explaining human behavior from the perspective of a developing country. Although the theories have been empirically examined, as standalone models and combined with other models in diverse research contexts, this study provides a proxy for future integration of the two theoretical frameworks in the best interest of enhancing their predictive power. This study adds to the empirical literature that employed the Unified Theory of Acceptance and Use of Technology and the Theory of Planned Behavior, albeit, in the peculiar m-health circumstances induced by the COVID-19 pandemic.
5.4. Limitations and future research directions
Although the objective of the research was accomplished, the study was not exempted from inherent research limitations. Firstly, the data was collected from university students enrolled at three public universities in Zimbabwe. This may affect the generalizability of the research findings to other health consumers outside the university setting. Future studies may investigate the antecedents of m-health usage by collecting research data from a diverse sample to enhance generalizability of results.
Secondly, the study presents a limitation on the adoption of the mono-quantitative methodology in generating the research findings. Statistical data does not capture the lived experiences of respondents in their natural setting. To enable interpretation of the findings from multiple perspectives, future studies may adopt triangulation.
Lastly, this study was a cross-sectional survey. Behavioral responses to changing health stimuli could not be captured over time. To address this shortcoming, future studies may employ longitudinal studies so that changes in public response to health technology and epidemiological circumstances are also accounted for. More so, convenience sampling provides a limitation to the generalizability of the findings. Future studies may use probabilistic methods so that findings can be compared to this research study.
Author contributions
Conception and design: PD; Collection, analysis and interpretation of the data: PD, DCJ and PM; Drafting of the paper: PD; Critical revision for intellectual content: PD, PM and DCJ; Final approval of the version to be published: PD, PM and DCJ. All authors agreed to be accountable for all aspects of the work. All listed authors meet the criteria for authorship as per the ICMJE guidelines (PD: Phillip Dangaiso; DCJ: Divaries Cosmas Jaravaza; PM: Paul Mukucha).
Acknowledgements
The authors would like to thank all participants who took part in this research. No funding was received.
Disclosure statement
No potential conflict of interest was reported by the author(s).
Data availability statement
The materials and data used in this research will be availed by the authors upon a reasonable request.
Additional information
Notes on contributors
Phillip Dangaiso
Phillip Dangaiso is a full-time Marketing lecturer and published author at Chinhoyi University of Technology (CUT), Zimbabwe.
Divaries Cosmas Jaravaza
Divaries Cosmas Jaravaza (PhD) is a full-time senior Marketing lecturer and accomplished researcher at Bindura University of Science Education (BUSE), Zimbabwe.
Paul Mukucha
Paul Mukucha (PhD) is a full-time senior Marketing lecturer and accomplished researcher at Bindura University of Science Education (BUSE), Zimbabwe.
References
- Ajzen, I. (1991). The theory of planned behavior. Organizational Behavior and Human Decision Processes, 50(2), 1–17. https://doi.org/10.1016/0749-5978(91)90020-T
- Ajzen, I. (2020). The theory of planned behavior: Frequently asked questions. Human Behavior and Emerging Technologies, 2(4), 314–324. https://doi.org/10.1002/hbe2.195
- Ajzen, I., & Fishbein, M. (1980). Understanding Attitudes And Predicting Social Behaviour. Prentice-Hall.
- Al-Gahtani, S. S. (2006). Information technology adoption, the roadmap to sustainable development : Examining three models. Saudi National Computer Conference, October 2016. https://www.researchgate.net/publication/255617584.
- Alaiad, A., Alsharo, M., & Alnsour, Y. (2019). The determinants of M-health adoption in developing countries: An empirical investigation. Applied Clinical Informatics, 10(5), 820–840. https://doi.org/10.1055/s-0039-1697906
- Alam, M. Z., Hu, W., Kaium, M. A., Hoque, M. R., & Alam, M. M. D. (2020). Understanding the determinants of mHealth apps adoption in Bangladesh: A SEM-neural network approach. Technology in Society, 61(October 2019), 101255. https://doi.org/10.1016/j.techsoc.2020.101255
- Amin, M. (2016). Internet banking service quality and its implication on e-customer satisfaction and e-customer loyalty. International Journal of Bank Marketing, 34(3), 280–306. https://doi.org/10.1108/IJBM-10-2014-0139
- Anderson, J. C., & Gerbin, D. W. (1988). Structural Equation Modelling in practise: A review and recommended two-step approach. Psychological Bulletin, 103(3), 411–423. https://doi.org/10.1037/0033-2909.103.3.411
- Arkorful, V. E., Lugu, B. K., & Shuliang, Z. (2023). Unearthing mask waste separation behavior in COVID-19 pandemic period: An empirical evidence from Ghana using an integrated theory of planned behavior and norm activation model. Current Psychology, 42(10), 8515–8530. https://doi.org/10.1007/s12144-021-02313-2
- Arkorful, V. E., Shuliang, Z., Lugu, B. K., & Jianxun, C. (2022). Consumers’ mobile health adoption intention prediction utilizing an extended version of the theory of planned behavior. ACM SIGMIS Database: The DATABASE for Advances in Information Systems, 53(2), 96–114. https://doi.org/10.1145/3533692.3533699
- Byrne, B. M. (2013). Structural equation modelling with AMOS: Basic concepts, applications and programming. Routledge Taylor and Francis.
- Chen, K., & Chang, M. (2013). User acceptance of ‘near field communication’ mobile phone service : an investigation based on the ‘unified theory of acceptance and use of technology’ model. The Service Industries Journal, 33(6), 609–623. https://doi.org/10.1080/02642069.2011.622369
- Cho, Y. M., Lee, S., Islam, S. M. S., & Kim, S. Y. (2018). Theories applied to m-health interventions for behavior change in low- and middle-income countries: A systematic review. Telemedicine Journal and e-Health, 24(10), 727–741. https://doi.org/10.1089/tmj.2017.0249
- Chuang, L. M., Chen, P. C., & Chen, Y. Y. (2018). The determinant factors of travelers’ choices for pro-environment behavioral intention-integration theory of planned behavior, Unified theory of acceptance, and use of technology 2 and sustainability values. Sustainability, 10(6), 1869. https://doi.org/10.3390/su10061869
- Dangaiso, P. (2023). Extending the Theory of Planned Behavior to predict organic food adoption behavior and perceived consumer longevity in subsistence markets: a post-peak COVID-19 perspective. Cogent Psychology, 10(1), 2258677. https://doi.org/10.1080/23311908.2023.2258677
- Dangaiso, P., Makudza, F., & Hogo, H. (2022). Modelling perceived e-learning service quality, student satisfaction and loyalty. A higher education perspective. Cogent Education, 9(1), 2145805. https://doi.org/10.1080/2331186X.20222145805
- Dangaiso, P., Makudza, F., Jaravaza, D. C., Kusvabadika, J., Makiwa, N., & Gwatinyanya, C. (2023). Evaluating the impact of quality antecedents on university students’ e-learning continuance intentions: A post COVID-19 perspective. Cogent Education, 10(1). https://doi.org/10.1080/2331186X.2023.2222654
- Dangaiso, P., Makudza, F., Tshuma, S., Hogo, H., Masona, C., Sakarombe, U., Nedure, T., Nyathi, G., Jonasi, K., Towo, T., Manhando, T., Dangaiso, P., Makudza, F., Tshuma, S., Hogo, H., Mpondwe, N., Masona, C., Sakarombe, U., Nedure, T., & Muchowe, R. (2023). Can social marketing undo the COVID-19 infodemic ? Predicting consumer preventive health behavior in the marginalized communities in Zimbabwe. Cogent Public Health, 10(1). https://doi.org/10.1080/27707571.2023.2234599
- Davari, A., & Strutton, D. (2014). Marketing mix strategies for closing the gap between green consumers’ pro-environmental beliefs and behaviors. Journal of Strategic Marketing, 22(7), 563–586. https://doi.org/10.1080/0965254X.2014.914059
- Davies, F. D. (1989). Perceived usefulness, perceived ease of use and user acceptance of information technology. MIS Quarterly, 13(4), 313–339. https://doi.org/10.2307/249008
- Dilotsotlhe, N., & Akbari, M. (2021). Factors influencing the green purchase behaviour of millennials : An emerging country perspective. Cogent Business & Management, 8(1), 1908745. https://doi.org/10.1080/23311975.2021.1908745
- Dwivedi, Y. K., Rana, N. P., Jeyaraj, A., Clement, M., & Williams, M. D. (2019). Re-examining the unified theory of acceptance and use of technology (UTAUT): Towards a revised theoretical model. Information Systems Frontiers, 21(3), 719–734. https://doi.org/10.1007/s10796-017-9774-y
- Fornell, C., & Larcker, D. (1981). Evaluating structural equation models with unobservable variables and measurement error. Journal of Marketing Research, 18(1), 39–50. https://doi.org/10.2307/3150980
- Galura, J. C., Reyes, E. G. D., Pineda, J., L. S., Galura, J. C., Reyes, E. G. D., & Pineda, J. L. S. (2022). C5-LMS design using Google classroom : User acceptance based on extended unified theory of acceptance and use of technology. Interactive Learning Environments, 31(9), 6074–6083. https://doi.org/10.1080/10494820.2022.2028852
- Hair, J., J. F., Page, M., & Brunsveld, N. (2020). Essentials of business research methods (4th ed.). Routledge.
- Hair, J. F., Black, W. C., Babin, B. J., & Anderson, R. E. (2019). Multivariate data analysis (8th ed.). Cengage.
- Herington, C., & Weaven, S. (2009). E-retailing by banks: E-service quality and its importance to customer satisfaction. European Journal of Marketing, 43(9/10), 1220–1231. https://doi.org/10.1108/03090560910976456
- Huang, Y. C. (2023). Integrated concepts of the UTAUT and TPB in virtual reality behavioral intention. Journal of Retailing and Consumer Services, 70(July 2022), 103127. https://doi.org/10.1016/j.jretconser.2022.103127
- Jaravaza, D. C., Risiro, J., & Mukucha, P. (2023). COVID-19 vaccination national radio advertising credibility assessment by rural consumers: The influence of indigenous medical knowledge systems and traditional beliefs. Cogent Public Health, 10(1). https://doi.org/10.1080/27707571.2023.2178052
- Jen, W., Lu, T., & Liu, P. (2009). An integrated analysis of technology acceptance behaviour models : Comparison of three major models. MIS Review, 15(1), 89–121.
- Johnstone, M.-L., & Tan, L. P. (2015). Exploring the gap between consumers’ green rhetoric and purchasing behaviour exploring the gap between consumer and purchasing behaviour. Journal of Business Ethics, 132(2), 311–328. https://doi.org/10.1007/s10551-014-2316-3
- Kline, R. B. (2016). Principles and practice of structural equation modelling (3rd ed.). Guilford Publication.
- Kline, R. B. (2023). Principles and practice of structural equation modelling (5th ed.). The Guilford Press.
- Leong, L. Y., Hew, T. S., Ooi, K. B., Metri, B., & Dwivedi, Y. K. (2022). Extending the theory of planned behavior in the social commerce context: A meta-analytic SEM (MASEM) approach. Information Systems Frontiers, 25(5), 1847–1879. https://doi.org/10.1007/s10796-022-10337-7
- Malhotra, N. K., Nunan, D., & Birks, D. F. (2017). Marketing research. An applied approach (5th ed.). Pearson.
- Matanhire, E., Vingirayi, I., & Manyanga, W. (2022, July). The impact of digital marketing in financial organizations. International Journal of Information, Business and Management, 13(2). https://www.researchgate.net/publication/361740407
- Manyanga, W., Ruzvidzo, P., & Chinokopota, T. (2022). Effect of the ubiquity of mobile banking on customer satisfaction in the banking sector in Zimbabwe. Cleaned International Journal of Information, Business and Management, 14(2), 2022.
- Mathai, N., McGill, T., & Toohey, D. (2022). Factors influencing consumer adoption of electronic health records. Journal of Computer Information Systems, 62(2), 267–277. https://doi.org/10.1080/08874417.2020.1802788
- Melzner, J., Heinze, J., & Fritsch, T. (2014). Mobile health applications in workplace health promotion: An integrated conceptual adoption framework. Procedia Technology, 16, 1374–1382. https://doi.org/10.1016/j.protcy.2014.10.155
- Moták, L., Neuville, E., Chambres, P., Marmoiton, F., Monéger, F., Coutarel, F., & Izaute, M. (2017). Les variables prédictives des intentions d’utilisation d’une navette autonome : aller au-delà du MAT et de la TCP ? European Review of Applied Psychology, 67(5), 269–278. https://doi.org/10.1016/j.erap.2017.06.001
- Muposhi, A., Dhurup, M., & Surujlal, J. (2015). The green dilemma: Reflections of a generation Y consumer cohort on green purchase behaviour. The Journal for Transdisciplinary Research in Southern Africa, 11(4), 31–40. https://doi.org/10.4102/td.v11i3.64
- Ndayizigamiye, P., & Maharaj, M. (2016, October 13-16). Mobile health adoption in Burundi: A UTAUT perspective. IEEE Xplore: IEEE 2016 Global Humanitarian Technology Conference, Seattle, WA. https://doi.org/10.1109/GHTC.2016.7857342
- Nunnally, J. C., & Bernstein, I. H. (1994). Psychometric theory. McGraw-Hill.
- Nyagadza, B., Mazuruse, G., Muposhi, A., Chuchu, T., Makoni, T., & Kusotera, B. (2022). Emotions’ influence on customers’ e-banking satisfaction evaluation in e-service failure and e-service recovery circumstances. Social Sciences & Humanities Open, 6(1), 100292. https://doi.org/10.1016/j.ssaho.2022.100292
- Pennycook, G., McPhetres, J., Zhang, Y., Lu, J. G., & Rand, D. G. (2020). Fighting COVID-19 misinformation on social media: Experimental evidence for a scalable accuracy-nudge intervention. Association for. Psychological Science, 31(7), 770–780. https://doi.org/10.1177/0956797620939054
- Postal and Telecommunications Regulatory Authority of Zimbabwe (POTRAZ). (2023). Postal and telecommunications regulatory authority of abridged postal and telecommunications. 0–20. Retrieved July 2, 2023, https://www.potraz.gov.zw/wp-content/uploads/2022/10/2022-Second-Quarter-Abridged-Sector-Performance-Report.pdf
- Puri, N., Coomes, E. A., Haghbayan, H., & Gunaratne, K. (2020). Social media and vaccine hesitancy: New updates for the era of COVID-19 and globalized infectious diseases. Human Vaccines & Immunotherapeutics, 16(11), 2586–2593. https://doi.org/10.1080/21645515.2020.1780846
- Purwanto, P., Abdullah, I., Ghofur, A., Abdullah, S., & Elizabeth, M. Z. (2022). Adoption of Islamic microfinance in Indonesia an empirical investigation: An extension of the theory of planned behaviour. Cogent Business & Management, 9(1). https://doi.org/10.1080/23311975.2022.2087466
- Rahman, M. M., Lesch, M. F., Horrey, W. J., & Strawderman, L. (2017). Assessing the utility of TAM, TPB, and UTAUT for advanced driver assistance systems. Accident; Analysis and Prevention, 108(June), 361–373. https://doi.org/10.1016/j.aap.2017.09.011
- Raycov, T., & Malcoulides, G. A. (2008). An introduction to applied multivariate analysis. Routledge Taylor and Francis Group.
- Rejali, S., Aghabayk, K., Esmaeli, S., & Shiwakoti, N. (2023). Comparison of technology acceptance model, theory of planned behavior, and unified theory of acceptance and use of technology to assess a priori acceptance of fully automated vehicles. Transportation Research Part A: Policy and Practice, 168(December 2022), 103565. https://doi.org/10.1016/j.tra.2022.103565
- Rosenstock, I. M. (2000). Health belief model. In A. E. Kazdin (Ed.), Encyclopedia of psychology (Vol. 4, pp. 78–80). Oxford University Press.
- Said, G. R. E. (2022). Factors affecting mHealth technology adoption in developing countries: The case of Egypt. Computers, 12(1), 9. https://doi.org/10.3390/computers12010009
- Saunders, M. N. K., Lewis, P., & Thornhill, A. (2018). Research methods for business students (8th ed.). Pearson.
- Schiffman, L. G., & Kanuk, L. L. (1994). Consumer behavior (4th ed.). Prentice-Hall.
- Schumacker, R. E., & Lomax, R. G. (2010). A beginner’s guide to structural equation modeling. Routledge.
- Shekhar, S. K. (2022). Social marketing plan to decrease the COVID-19 vaccine hesitancy among senior citizens in rural India. Sustainability, 14(13), 7561. https://doi.org/10.3390/su14137561
- Siripipatthanakul, S., Limna, P., Sriboonruang, P., & Kaewpuang, P, Manipal GlobalNxt University, Malaysia. (2023). Applying the TPB and the UTAUT models predicting intentions to use telemedicine among Thai people during the COVID-19 pandemic. International Journal of Computing Sciences Research, 7(June), 1362–1384. https://doi.org/10.25147/ijcsr.2017.001.1.107
- Tabachnick, B. G., & Fidell, L. S. (2013). Using multivariate statistics (6th ed.). Pearson.
- Umar, U., Mas’ud, A., & Matazu, S. (2021). Direct and indirect effects of customer financial condition in the acceptance of Islamic microfinance in a frontier market. Journal of Islamic Marketing, 13(9), 1940–1957. https://doi.org/10.1108/JIMA-12-2019-0267
- Vasli, P., Shekarian-Asl, Z., Zarmehrparirouy, M., & Hosseini, M. (2022). The predictors of COVID-19 preventive health behaviors among adolescents: The role of health belief model and health literacy. Journal of Public Health. https://doi.org/10.1007/s10389-022-01808-x
- Venkatesh, M., & Davis, D. (2003). User acceptance of information technology: Toward a unified view. MIS Quarterly, 27(3), 425. https://doi.org/10.2307/30036540
- Winkle, C. M., Van, Bueddefeld, J. N. H., Halpenny, E. A., Mackay, J., Winkle, C. M., Van, Bueddefeld, J. N. H., & Halpenny, E. A. (2019). The unified theory of acceptance and use of technology 2: Understanding mobile device use at festivals. Leisure Studies, 38(5), 634–650. https://doi.org/10.1080/02614367.2019.1618895
- Zhang, X., Liu, S., Wang, L., Zhang, Y., & Wang, J. (2020). Mobile health service adoption in China: Integration of theory of planned behavior, protection motivation theory and personal health differences. Online Information Review, 44(1), 1–23. https://doi.org/10.1108/OIR-11-2016-0339