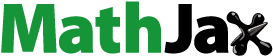
Abstract
Economic growth continues to be a crucial determinant of the achievement of socioeconomic goals. Therefore, the observed declines in economic growth and productivity levels over the last few decades have been a cause for concern, and this has reignited the discussion on the importance of intangible sources of economic growth and productivity such as intellectual property, research, technology, software and human capital. This study investigates the effect of knowledge creation through basic research (article publications) on economic growth for 130 countries from 1995 to 2020 using the Least Absolute Shrinkage and Selection Operator regression. The results of the study show that knowledge creation is positively associated with economic growth and these results are robust to various sensitivity tests. However, the impact of publications is larger for developed countries which can be explained by the large number of publications relative to emerging and developing countries. Moreover, publications in the Science, Technology, Engineering and Mathematics fields have a larger effect on economic growth. The study recommends increased investments in basic research in order to promote knowledge creation particularly in the Science, Technology, Engineering and Mathematics fields in developing and emerging economies.
1. Introduction
Economic growth continues to be a crucial determinant of development worldwide. The achievement of socioeconomic goals such as reducing inequality and enhancing living standards, cannot be a reality without growth in total production in an economy (International Monetary Fund, Citation2021). Declining economic growth and productivity levels over the last few decades have been a cause for concern, and this has been exacerbated by the COVID-19 pandemic. Global economic growth has stagnated especially during the period 2010–2019 due to the sluggish recovery from the 2008/2009 global financial crisis. The slowdown in productivity growth has been evident in mature (developed) countries and despite relatively high productivity growth in emerging countries, a decline has been recorded between 2000 and 2019. The Organisation for Economic Co-operation and Development (OECD) (Citation2013) and International Monetary Fund (Citation2021) are of the view that sources of economic growth such as productivity, innovation and adoption of latest technologies, are crucial for the world economy. The United Nations emphasised the important role of science, technology and innovation in the achievement of the 2030 sustainable development goals (SDGs) (Schlegel & Unesco, Citation2015).
Growth driven by innovation, knowledge and technological progress is highlighted by endogenous growth theories of Romer (Citation1986), Romer (Citation1990), Grossman and Helpman (Citation1991) and Aghion and Howitt (Citation1992). Furthermore, a number of studies show that innovation and knowledge creation are important drivers of economic growth (see Inglesi-Lotz & Pouris, Citation2013; Ntuli et al., Citation2015; Hatemi-J et al., Citation2016; Maradana et al., Citation2017; Pinto & Teixeira, Citation2020; Hausman, Citation2022). Hazan et al. (Citation2021) are of the view that intangible sources such as intellectual property, research, technology, software and human capital will be drivers of future long-term economic growth. The term ‘Knowledge Economy’ was coined to describe an economy where productivity, economic growth and development are determined by innovation and access to information (European Bank for Reconstruction & Development, Citation2019). One of the key pillars of knowledge-based economies is innovation which encompasses inputs such as research and development (R & D) spending, and outputs such as patents, venture capital and scientific collaborations.
The need for knowledge creation has placed role players such as government, academic institutions and industry at the forefront. According to Reichert (Citation2019), universities have an important role of knowledge creation and fostering innovation through research and education. In conducting research, universities are able to draw on different perspectives due to access to international research. The International Monetary Fund (Citation2021) suggest that innovation is driven by both applied and basic research. Academic institutions prioritise basic research which involves the creation of new knowledge which may not necessarily be directly linked to practical application. It is for this reason that R&D expenditures have traditionally been channelled to applied research by the private sector (Schlegel & Unesco, 2015). However, basic research complements applied research through the development of new knowledge and alternative approaches to the production of goods and services. Therefore, the private sector has upscaled expenditures in basic research. Lewis et al. (Citation2021) state that in two of the major innovating countries, United States and Switzerland, investment spending in basic research grew significantly between 2012 and 2017 due the realisation that basic research can be a perfect foil for applied research.
It is against this backdrop that this study investigates the effect of knowledge creation through basic research, on economic growth for a sample of developed and developing (emerging) countries. The proxies for basic research are total article publications as well as articles published in the Science, Technology, Engineering and Mathematics (STEM) fields. The other objective of the study is to compare the effect of total publications and STEM publication on economic growth. Literature suggests that STEM skills are more vital for innovation and economic growth (World Bank & Elsevier, Citation2014). Also, the study will compare the impact of publications on economic growth in developed, developing and emerging economies. This is crucial for identifying regions where impact of publications is lower, thus necessitating the implementation of policies to strengthen the link between the variables. The study’s contribution to literature is twofold. First, a large number of studies that examine the research publications-economic growth nexus employ causal analysis (Hatemi-J et al., Citation2016; Inglesi-Lotz et al., Citation2015; Inglesi-Lotz & Pouris, Citation2013; Jin, Citation2010; Ntuli et al., Citation2015; Odhiambo & Ntenga, Citation2016; Yaşgül & Güriş, Citation2016; Zaman et al., Citation2018). In most cases, causality does not reveal the magnitude of the effect of publications on economic growth, a gap that this study seeks to fill. Second, the relatively few studies that have highlighted the magnitude of the effect of publications on economic growth such as Solarin and Yen (Citation2016), Pinto and Teixeira (Citation2020), Kim and Lee (Citation2015), Agasisti and Bertoletti (Citation2020) and Bertoletti et al. (Citation2022) either do not show the disparities in the impacts of research publications on economic growth in different regions of the world or only focus on regional analysis. This study highlights the disparities in the impact of publications in developed economies, developing and emerging countries in Asia and Latin America and African countries.
The study uses panel data and employs the Least Absolute Shrinkage and Selection Operator (Lasso) regression approach initially developed by Tibshirani (Citation1996) and advanced for panel data by Belloni et al. (Citation2014); Belloni et al. (Citation2016) and Chernozhukov et al. (Citation2015). Lasso or regularized regressions are essentially machine learning techniques which manage the bias-variance trade-off of regression models by introducing a bias shrinkage mechanism and a penalty that limits the number of explanatory variables included in a model. This allows the researcher to circumvent the problem of ‘overfitting’ commonly found in traditional econometric analysis and further ensures variable selection consistency as well as higher predictive accuracy compared to conventional techniques.
The study is structured as follows: Section 2 discusses the literature review which comprises of the theoretical and empirical literature. Section 3 presents the data and methodology of the study while section four discusses the empirical results. Lastly, Section 5 concludes the study and provides recommendations.
2. Literature review
This section provides a discussion of the literature review. The theoretical literature discusses the major growth theories that emphasise the effect of knowledge and technology on economic growth. The theoretical literature is based on the neoclassical growth model of Solow (Citation1956) and Swan (Citation1956) and endogenous growth model of Romer (Citation1986, Citation1990), Lucas (Citation1988), Chen and Kee (Citation2005) and Jones (Citation2022).
2.1. Theoretical literature review
The theoretical literature on the effect of knowledge on economic growth is drawn formally from the Solow–Swan’s neoclassical growth model developed independently by Solow (Citation1956) and Swan (Citation1956). In the Solow–Swan model, economic growth is driven by capital accumulation, population and technological progress. In the neoclassical growth model, long-run economic growth is only determined by technological progress, which is assumed to be a public good available in each country. However, the exogenous nature of technological progress is a limitation of the Solow-Swan model which renders it less useful for policy analysis. In an attempt to ‘endogenise’ technological progress, Arrow (Citation1962) developed a learning-by-doing model that assumes that continuous production of goods promotes knowledge accumulation and further improvements in the production process. A major limitation of this approach is that technological progress is a by-product production and not necessarily determined by actions of individuals engaged in R & D (Romer, Citation1990).
The endogenous growth theories developed by Romer (Citation1986, Citation1990) and Lucas (Citation1988) underpin the study to a large extent. Endogenous growth models postulate that economic growth is determined by active steps taken by individuals as opposed to exogenous technological change advocated for by the neoclassical growth theory. Lucas (Citation1988) developed an endogenous growth model that depicts human capital, defined as skills such as physical and intellectual abilities possessed by individuals, as an engine for economic growth. The theory assumes that knowledge is rival and excludable, and that human capital and technological progress can have both a substitute and complimentary relationship. The major limitation of the theory is treatment of knowledge as a rival and excludable good, as assumption that was refuted by Romer (Citation1990). Furthermore, the evolution of technological advancements was largely ignored.
Initially, Romer (Citation1986) developed an endogenous growth model as an extension of Arrow (Citation1962)’s learning-by-doing model, where technological development is a ‘by-product’ of capital accumulation. The model consists of two sectors; one where goods are produced and the other knowledge is developed through capital accumulation. Romer (Citation1990) modified his endogenous growth model to take into account the technological progress developed as a result of actions taken by agents responding to market incentives. Technological change is assumed to be non-rival and also the major determinant of economic growth similar to the Solow-Swan neoclassical growth models. However, technological spillovers are introduced in the endogenous model to capture the notion that society can enjoy benefits of innovations and discoveries, and therefore knowledge has a non-excludable characteristic resembling a public good.
Romer (Citation1990)’s endogenous growth model comprises of inputs such as human capital, labour, capital and the level of technology as follows:
(1)
(1)
where
represents human capital, which is formed by formal education and on-the-job training,
is labour measured by the population size,
is knowledge/technological progress and
is a list of other inputs used in the production process. Human capital represents the rival component of knowledge compared to
which is non-rival.
Non-rival knowledge or technological progress is determined as follows:
(2)
(2)
where
is amount of human capital devoted to R & D and
current level of knowledge. Therefore, the higher the number of researchers engaged in R & D, the greater the level of knowledge produced. Romer (Citation1990)’s model consists of three sectors. First, there is the goods sector where human capital, labour and other inputs are used to produce final goods. Second, there is the research sector where human capital and the current level of knowledge contribute to new knowledge development. Third, the intermediate goods sector where output from the research sector is combined with forgone output to produce durable goods used in producing final goods. Therefore, knowledge contributes to economic growth directly through the production of final goods and indirectly through output from the research sector.
The endogenous growth model has been extended by a number of researchers. For this study, the most relevant is Chen and Kee (Citation2005)’s endogenous growth model and the semi-endogenous (SEG) growth model developed by Jones (Citation2022). Chen and Kee (Citation2005) highlighted that in the traditional endogenous growth models higher levels of human capital will promote sustained economic growth. However, these models fail to explain the high economic growth rates in East Asian Newly Industrialising Economies (NIEs) despite lower human capital levels than developed economies. Similar to the traditional endogenous growth model, Chen and Kee (Citation2005) developed an endogenous growth model where knowledge accumulation is a driver of economic development. However, the model’s point of departure is that the steady-state growth rate of output per capita is dependent on the growth rate and not the level of human capital. Jones (Citation2022) utilised the non-rival assumption of knowledge proposed by Romer (Citation1990), to develop an SEG model where output per capita is determined by the aggregate stock of ideas. Ideas are driven by the number of researchers and the existing knowledge. The model suggests that long-run economic growth is determined by growth rate of research efforts which can be interpreted as the number of publications.
2.2. Empirical literature
This section presents the empirical literature which comprises of related studies that have investigated the effect of knowledge creation through research publications, on economic growth. A summary of the studies and their findings is presented in .
Table 1. Literature review summary.
A number of studies have investigated the effect of scientific knowledge created by universities on economic growth. These studies are segregated according to their findings and as well as the techniques employed in order to uncover the gaps in literature. Studies by Kim and Lee (Citation2015), Solarin and Yen (Citation2016), Pinto and Teixeira (Citation2020), Brenner (Citation2014), Oluwatobi et al. (Citation2020), Agasisti and Bertoletti (Citation2020) and Bertoletti et al. (Citation2022) used the Generalised Method of Moments (GMM) and reached different conclusions. Solarin and Yen (Citation2016), Pinto and Teixeira (Citation2020), Brenner (Citation2014) and Bertoletti et al. (Citation2022) found evidence that research publications/output have a positive impact on economic growth through innovation. However, Pinto and Teixeira (Citation2020) showed that the impact is higher for fields such as engineering, technology, social sciences and physics. Similarly, Agasisti and Bertoletti (Citation2020) highlighted that the quality of research publications is more important in explaining regional economic development compared to the quantity. Oluwatobi et al. (Citation2020) cautioned that the effect of innovation induced by research publications is dependent on the quality of institutions. Therefore, in countries with low institutional quality, innovation has an insignificant effect on economic growth. Kim and Lee (Citation2015) found that academic publications are not major drivers of economic growth in Asia and Latin American countries. Instead, technological knowledge in the form of patents is a determinant of economic growth. A possible explanation is the lack of collaboration between academia and industry which implies that academic research remains confined to academic institutions and not contributing to innovation and the production of more sophisticated products.
Other studies that have utilised panel data analysis include Jin and Jin (Citation2014), Wang et al. (Citation2020), Anakpo and Oyenubi (Citation2022) and Hausman (Citation2022). Jin and Jin (Citation2014) found that research outputs in Science and Engineering have the largest effect on economic growth in developed countries compared to those in Business and Economics. The findings are similar to those of Pinto and Teixeira (Citation2020) to a large extent. Wang et al. (Citation2020, Anakpo and Oyenubi (Citation2022) and Hausman (Citation2022) found that university innovation has a positive effect on economic growth.
A number of studies employed causality tests (see Hatemi-J et al., Citation2016; Inglesi-Lotz et al., Citation2014, Citation2015; Jin, Citation2009; Jin, Citation2010; Kumar et al., Citation2016; Lee et al., Citation2011; Ntuli et al., Citation2015; Odhiambo & Ntenga, Citation2016; Pradhan et al., Citation2020; Yaşgül & Güriş, Citation2016; Zaman et al., Citation2018). Jin (Citation2009) found that there is bidirectional causality between research productivity and economic growth in Hong Kong, unidirectional causality from publications to economic growth in Korea and Taiwan, unidirectional causality from economic growth to publications in Japan and causal feedback for Singapore. Jin (Citation2010) also found unidirectional causality from economic growth to publications in Japan. Lee et al. (Citation2011) found that there is bidirectional causality between research output and economic growth in Asian countries and unclear causality in Western countries. There is evidence that causality runs from economic growth to research publications in most developed countries with the exception of the United States where causality is from research outputs to economic growth (see Inglesi-Lotz et al., Citation2014; Kumar et al., Citation2016; Ntuli et al., Citation2015). In line with the finding, Hatemi-J et al. (Citation2016) found that among G7 countries, research publications only have a positive effect on economic growth in the UK, while Zaman et al. (Citation2018) found causality from economic growth to publications in high income OECD countries. Odhiambo and Ntenga (Citation2016), Yaşgül and Güriş (Citation2016) and Pradhan et al. (Citation2020) found evidence of causality from research publications to economic growth in South Africa, Turkey and the Eurozone, respectively.
The long-term relationship between research publications and economic growth using the Autoregressive Distributed Lag (ARDL) model and other cointegrations tests, has been examined by the number of studies (see Inglesi-Lotz and Pouris, Citation2013; Odhiambo & Ntenga, Citation2016; Tunali, Citation2016). Inglesi-Lotz and Pouris (Citation2013) observed a long-run relationship between publications and economic growth in the sciences fields in South Africa. Odhiambo and Ntenga (Citation2016) found evidence of a long-run relationship between publications and economic growth in South Africa. Tunali (Citation2016) reported that there is a long-run relationship between scientific publications and Gross Domestic Product (GDP) per capita only in France and Sweden among 15 European countries.
Others studies used correlation analysis (see Drogovoz et al., Citation2019; Gholizadeh et al., Citation2014; Ogot & Onyango, Citation2022). Gholizadeh et al. (Citation2014) found a positive correlation between research publications and economic growth in ASEAN countries. Drogovoz et al. (Citation2019) observed that there is insignificant correlation between publications and economic growth in a sample of 95 countries. Ogot and Onyango (Citation2022) also found evidence of insignificant correlation in Kenya. Positive and significant correlation was observed when the outlying sectors of health and education were omitted.
In concluding the literature review, we highlight the contribution of our study to literature as follows: a considerable number of studies that examine the research publications and economic growth nexus utilise causal analysis. However, the establishment of causality does not unveil the extent of the impact of publications on economic growth, which is a gap that this study intends to address. Second, the limited number of studies that have shed light on the magnitude of the impact of publications on economic growth, such as Solarin and Yen (Citation2016), Pinto and Teixeira (Citation2020), Kim and Lee (Citation2015), Agasisti and Bertoletti (Citation2020) and Bertoletti et al. (Citation2022), either fail to demonstrate the disparities in the effects of research publications on economic growth across different regions of the world or solely concentrate on regional analysis. This study brings attention to the discrepancies in the impact of publications on developed economies, developing and emerging countries in Asia and Latin America, as well as African countries.
3. Data and methodology
This section presents the data to be used in the empirical analysis and also discusses the methodological approach adopted. The variables of the study are shown on .
Table 2. Data description.
The study employs the panel data approach to regression modelling. The time period of the study is 1995 to 2020 and 130 countries were selected for the analysis based on the availability of data. Panel data have advantages of time-series and cross-sectional analysis such as the ability to cater for time-invariant specific heterogeneity in models, enhancing degrees of freedom and mitigating against collinearity (Baltagi, Citation1995; Belloni et al., Citation2016). A panel data model can be specified as follows:
(3)
(3)
where
is the dependent variable,
are the explanatory variables,
are the individual-specific effects and
is the error terms with a mean of zero.
The initial empirical model including all the variables is specified as follows:
(4)
(4)
The model will also include interaction terms between the variables, quadratic terms to capture non-linear effects and individual fixed effects for the 130 countries. As such the number of potential control variables is 165.
The control variables are selected based on literature. High inflation results in macroeconomic instability and higher interest rates which hinders investments economic growth. The variable is thus expected to be negatively signed (Akinsola & Odhiambo, Citation2017; Bittencourt, Citation2012). According to the neoclassical and endogenous growth theories, capital accumulation is assumed to be an engine of economic growth (Romer, Citation1990; Solow, Citation1956). North (Citation1990) suggests that institutions are rules and regulations that guide behaviour. Institutions reduce the cost of transactions and thus promotes economic growth. FDI and trade cater for the effect of globalisation on economic growth. Salvatores (Citation2014) suggests that reducing trade barriers enhances economic growth through technological diffusion and fostering of specialisation in the production process. Therefore, the globalisation variables are expected to be positively signed.
The challenges of estimating a panel data model include the issue of cross-sectional dependence caused by common unobserved factors among the cross-sectional units (Henningsen & Henningsen, Citation2019). Cross-sectional dependence leads to inconsistent estimators which cannot be used for analysis. Panel datasets also include time-varying variables which are high dimensional in nature, thus complicating the predictive ability of estimated models and the statistical inference (Belloni et al., Citation2016). A potential remedy for high dimensional data is to employ regularised regression techniques which are an extension of linear regression models (Ahrens et al., Citation2020). Similar to linear regression, regularised regression techniques minimise the sum of squared residuals, however, a penalty is imposed on the variables to address issues such as overfitting and over estimation (Ranstam & Cook, Citation2018). A large number of independent variables can lead to situations where the number of slope parameters to be estimated exceeds the number of observations, thus increasing the variance of the least squares estimator. On the other hand, reducing the number of explanatory variables may lead to the omitted variable bias. Therefore, the regularised regression techniques are able to manage the bias-variance trade-off by introducing a bias shrinkage mechanism and a penalty that limits the number of explanatory variables included in a model (Ahrens et al., Citation2020).
A solution to high dimensional data is to estimate sparse models using regularised approaches. A sparce model is one where a subset of the explanatory variables is important for a particular model (Hastie et al., Citation2015). The Lasso operator (also known as the regularised regression) is the most popular of the regularised regression methods (Ahrens et al., Citation2020). The Lasso was developed by Tibshirani (Citation1996) and minimises the sum of the squared errors subject to a constraint on the coefficients as follows:
(5)
(5)
where
is the
norm of
, t is a tuning parameter that determines the level of regularisation
is the explained variable,
is the regressor,
and
coefficients of the model. Due to the regularisation, some of the coefficients can be constrained to zero.
The original Lasso technique is mostly appropriate for predictions and not causal or statistical inference. However, the main focus of economic studies is to estimate the causal relationship between variables. This study adopts the regularised regression approaches developed by Belloni et al. (Citation2014); Belloni et al. (Citation2016) and Chernozhukov et al. (Citation2015), that can be used for statistical inference and causal analysis. The techniques are appropriate for this study given that the main objective is to determine the causal effect of knowledge creation through research publications, on economic growth. Belloni et al. (Citation2014) developed the post-double-selection (PDS) Lasso approach for statistical inference on the treatment variable or the explanatory variable of interest. The PDS Lasso is based on the following equations:
(6)
(6)
(7)
(7)
where
is the treatment variable,
represents the control variables while
and
are both unobserved error terms.
The PDS Lasso has three stages; first, a regression of the dependent variable on the treatment variable and control variables. The important control variables that impact on the treatment variable are selected. Second, the control variables selection process is repeated in the specification where the treatment variable is used as a dependent variable. Lastly, the dependent variable is regressed on the treatment variable and the set of control variables selected in the first two steps. Chernozhukov et al. (Citation2015) developed the double-orthogolisation approach (often referred to as the CHS method) which is closely related to the PDS technique. In contrast to the third step of the PDS approach, the CHS uses the selected control variables to form ‘orthogonalised versions’ of the dependent and treatment variable.
Belloni et al. (Citation2016) developed a variant of the Lasso approach for panel data that caters for cross-sectional dependence, hence the name Cluster-Lasso. The technique also has the advantage of permitting statistical inference post the variable selection process and thus can be used in causal analysis. The Cluster-Lasso eliminates the fixed effects by demeaning the variables and estimates the slope coefficients of interest by solving the following model:
(8)
(8)
The penalty level and the covariate-specific penalty loadings
are the tuning parameters that determine the level of regularisation in the Lasso technique.
4. Empirical results
This section presents the empirical results of the study. Descriptive statistics are initially discussed to determine the properties of the data before the discussion of the regression results. The countries are demarcated into developed countries, emerging countries as well as developing countries in Africa. The delineation is based on the International Monetary Fund (Citation2022)’s classification. A full list of the countries is shown in the . Descriptive statistics presented in highlight the high GDP and number of articles in developed countries. Despite the lower and stagnant economic growth rates in developed countries over the last two decades as shown by International Monetary Fund (Citation2021), total GDP remains higher than emerging/developing economies. Notwithstanding the large number of emerging countries in the sample, the average number of articles published is on average about 20% of those in developed countries. On average, total articles published in African countries are roughly 3% of the articles in developed countries. Similar results are observed when considering publications in the STEM fields. World Bank and Elsevier (2014) showed that despite the increase in the number of publications in Sub-Saharan Africa (SSA) during the period 2003–2012, the region accounted for less than 1 percent of total global publications. Furthermore, STEM publications constitute a smaller component of total publications in SSA relative to other regions such as Asia. According to Lewis et al. (Citation2021), scientific publications from high-income countries constituted 62.9% of total publications while those of upper-middle income, lower-middle income and lower income countries were recorded at 38.1%, 11.4% and 0.9%, respectively. Furthermore, high income countries published 56% of articles in science and engineering compared to 34% and 9% for upper-middle and lower income economies, respectively, in 2018 (White, Citation2019). However, despite the lower average publications rates in upper-middle and lower income countries, the growth rate of published articles has outpaced that of high income countries (9 versus 1%) between 2008 and 2018. Due to their large numbers and high standard deviations, GDP, KNW and STEM will be used in logarithmic form in the regression models.
Table 3. Descriptive statistics.
presents scatter graphs showing the relationship between the average number of articles published and economic growth over the period 1995–2020. A positive relationship between publications and economic growth is observed for the full sample and all country groups. Publications are higher for economies with higher levels of GDP. However, this could suggest that GDP is a determinant of the number of publications. Higher levels of GDP may be linked to higher levels of research expenditure which in turn leads to more publications. Schlegel and Unesco (2015) highlight that countries such as China, USA, the EU, Republic of Korea and India constitute over 80% of total research expenditure. As such, these countries have more publications compared to countries with low research expenditure.
Figure 1. Scatter graphs.
Source: Researchers’ own computations. Note. LGDP is the logarithmic form of GDP, LART is the logarithmic form of total articles published (KNW).
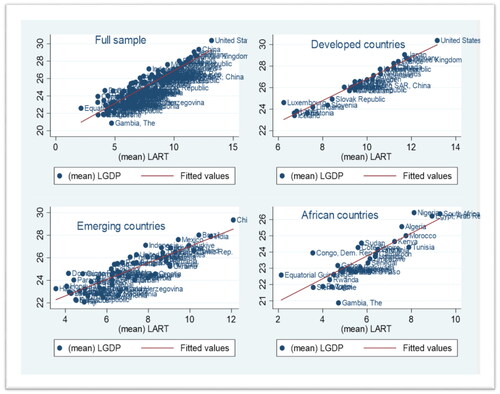
The regression results are shown in –. The study reports Belloni et al. (Citation2014)’s PDS Lasso results and Chernozhukov et al. (Citation2015)’s CHS Lasso using orthogonal variables and the Post Lasso orthogonalised variables. The three approaches allow statistical inference on the treatment variable or the explanatory variable of interest and not the control variables. The Cluster-Lasso approach and the instrumental variables (IV) Lasso are used for robustness checks. The cluster option is used to control for cross-sectional dependence that might be present in the data, while the IV lasso is used to control for potential endogeneity of the treatment variable. It is evident from the descriptive statistics that countries with higher levels of GDP (developed countries) have much higher levels of publications, possibly due to higher levels of expenditures in research. Therefore, it is possible that publications could be endogenous. The PDS and CHS Lasso models were estimated in Stata using the ‘pdslasso’ command developed by Ahrens et al. (Citation2018) while the IV Lasso models were estimated using the ‘ivlasso’ command developed by the same authors.
Table 4. PDS Lasso results: total article publications.
Table 5. PDS Lasso results: STEM publications.
Table 6. Cluster-Lasso.
Table 7. Instrumental variables Lasso.
In line with theoretical expectations, the PDS lasso results for the full sample (written as all) reveal a positive and significant relationship between economic growth and knowledge creations represented by article publications. A 1% increase in the number of publications causes an increase in economic growth in the range between 0.324% and 0.465%. Human capital, the interaction term between human capital and institutions, investment and trade were selected as control variables. Human capital has a positive effect on economic growth and the interaction term is positively signed. Investment has a positive effect on economic growth in line with the neoclassical and endogenous growth theories that emphasise capital accumulation in the growth process. A 1% point increase in investment increases economic growth by 0.70%. Contrary to expectations, trade has a negative effect on economic growth. The effect of human capital on economic growth is positive in the presence of high institutional quality. Higher levels of institutional quality encourage investments in human capital (Ouedraogo et al., Citation2022).
A positive and significant effect of publications on economic growth is observed in all the country groups included in the study. For developed economies, the coefficient ranges from 0.302 to 0.577. With regards to the control variables, trade has negative effect on economic growth similar to the full sample. Inflation is negative signed which confirms a priori expectations. High inflation is an indication of macroeconomic instability which hinders investments and economic activity. For emerging economies, the effect of publications is much lower compared to developed countries. The lower coefficient could be an indication of lower number of publications compared to the developed countries as shown in the descriptive statistics. The selected control variables, trade and human capital are negatively signed. However, there is evidence that human capital has a positive effect on economic growth in the presence of institutional quality. In African countries the impact of publications on economic growth, is lower compared to developed and emerging economies. African countries have much lower number of publications. The selected control variables are investment, human capital and the interaction between the two variables. Human capital has a positive effect on economic growth while investment is only positive in the presence of higher levels of human capital. As such, investment in human capital is of paramount importance.
The effect of STEM publications on economic growth is shown in . The coefficients are positive and significant for the entire sample and all the country groups. A 1% increase in STEM publications increases economic growth by a range between 0.347% and 0.491% for the full sample. The results suggest differential impacts between total number of publications and those in the STEM fields. The STEM publications coefficients are larger than those of total publications thus highlighting the importance of publications in the STEM fields. The control variables are largely similar to those selected when total publications were used as the treatment variable.
5. Sensitivity analysis
As mentioned above the Cluster-Lasso and the IV Lasso will be used for robustness checks. The cluster-lasso results are shown in . The results suggest that a 1% increase in publications is expected to increase economic growth by a range between 0.781% and 0.80% for the full sample. A couple of differences are observed between the PDS lasso and cluster lasso. First, despite retaining the positive and significant coefficients, the Cluster-Lasso coefficients are much larger compared to those the PDS Lasso without the clustering. Second, the Cluster-Lasso results do not show any differential affects between total number of publications and those in the STEM fields for the different country groups. However, the differences in the impact of STEM publications across country groups are evident. The selected control variables in the cluster-lasso regression (not shown on the table) are human capital and the interaction between human capital and institutions. The effect of human capital on economic growth is positive in the presence of high institutional quality. An instrumental variables model was estimated where the previous lags of publications were used as instruments. The results shown in highlight that publications retain its positive and significant coefficient, suggesting that the results are robust to any possible endogeneity.
6. Discussion and implication of the findings
The study found that knowledge creation through research publications has a positive effect on economic growth. The results are in line with those of Solarin and Yen (Citation2016), Pinto and Teixeira (Citation2020), Brenner (Citation2014) and Bertoletti et al. (Citation2022) who reported that publications have a positive effect on economic growth. The findings align with the proposition by Jones (Citation2022)’s SEG model that the greater the stock of ideas (proxied by research effort) is a crucial determinant of long-term economic growth. The study also found that STEM publications have a positive effect on economic growth. Furthermore, the impact is larger than that of overall publications which attest to their importance for economic growth. The finding supports that of Agasisti and Bertoletti (Citation2020), Pinto and Teixeira (Citation2020) and Jin and Jin (Citation2013). World Bank and Elsevier (2014) are of the view that STEM skills and knowledge are crucial for innovation and productivity.
The study has profound implications. Basic research is important for long-term economic growth similar to applied research. As shown by Lewis et al. (Citation2021), recent trends suggest that the private sector in countries such as the United States and Switzerland has increased its investment in basic research due to its complimentary relationship to applied research. The improvements in the collection of data through basic research suggests that investments in basic research should be upscaled especially in emerging and developing countries where the number of publications is lower. Furthermore, the reduction in public sector funding for basic research observed in some regions as shown by Schlegel and Unesco (2015), could be detrimental to future growth and development. STEM research has a larger effect economic growth compared to overall publications. The increase in the number of science and engineering publications observed in upper-middle and lower-middle income countries is an important development given the importance of science, engineering and technology for the achievement of SDGs.
7. Conclusion and recommendations
The objective of the study was to investigate the effect of knowledge creation through research publications, on economic growth in both developed and developing countries. The slowdown in economic growth and productivity in the world economy has been a cause for concern given the importance of the variables for the achievement of SDGs. Knowledge creation and innovation are vital for the recovery of the world economy especially after the negative effects of the COVID-19 pandemic. Research or journal publications are regarded as basic research, and have been funded mostly by the public sector, as opposed to applied research funded by the private sector. However, the private sector has upscaled its expenditure in basic research due to its complimentary relationship with applied research.
The study adopted the panel approach and employed the Lasso technique for regression analysis due to its ability to better manage the bias-variance trade-off compared to the Least Squares approach. The results of the study suggested that knowledge creation through publications is positively associated with economic growth for the different country groups in the study. The impact of publications was higher for developed countries which can be explained by the large number of publications relative to emerging and developing countries. Publications in the STEM fields have a large effect on economic growth.
A few recommendations for policy makers can be drawn from the study. First, basic research should be promoted worldwide by both the public and private sectors due to the evidence that higher numbers of publications have larger growth effects. Furthermore, the public sector should reverse any trends in the decline in investments in basic research due to its importance in promoting applied research. Second, investment in STEM fields is of paramount importance for the growth and transformation of the world economy especially for emerging and developing countries where publications are lower. A such, investment in STEM fields needs to be increases coupled with encouraging STEM education amongst the students. According to the World Bank and Elsevier (2014), the lower numbers of publications in STEM fields in SSA could be due to low quality basic education in science, mathematics and lower numbers of students enrolled in STEM qualification at tertiary institutions. Therefore, improving the quality of basic education in science and mathematics is of paramount importance in increasing the number of STEM publications in the future.
Recommendations for future research are as follows: The study has shown the importance of publications in the STEM fields for economic growth in both developed and developing countries. As such, it is recommended that future studies examine the drivers of publications in the STEM fields. This will be crucial for policy makers in their attempts to implement strategies to promote research in the STEM fields. Furthermore, the linkages between basic research, entrepreneurship and innovation should be investigated. In order to promote economic growth, basic research should be translated into innovation which in turn improves technological advancements. The translation of basic research to innovation should be strengthened especially in developing and emerging economies where the impact of publications on economic growth is lower. Strengthening the link between publications and growth could be achieved by promoting entrepreneurship and implementing tax incentives to encourage the private sector investments.
The study has a few delimitations. First, it is conducted at macro level and thus does not incorporate analysis of the individual micro units in a population. Second, panel regression was employed due to the limited time series data for some countries. Panel data studies present analysis for groups of countries and, therefore, does not show the differences between inherent between individual countries.
Disclosure statement
No conflict of interest.
Additional information
Notes on contributors
Clement Moyo
Clement Moyo is a Post Doctoral Research Fellow at Nelson Mandela University. He completed his PhD at the same university in 2018. His areas of expertise are Macroeconomics, Development Economics, Econometrics, International Economics and Industry Analysis.
Andrew Phiri
Andrew Phiri is an Associate Professor at Nelson Mandela University. His areas of expertise are Macroeconomics, Applied econometrics, Financial economics, Monetary economics and International economics.
References
- Agasisti, T., & Bertoletti, A. (2020). Higher education and economic growth: A longitudinal study of European regions 2000–2017. Socio-Economic Planning Sciences, 81, 1. https://doi.org/10.1016/j.seps.2020.100940
- Aghion, P., & Howitt, P. (1992). A model of growth through creative destruction. Econometrica, 60(2), 323–17. https://doi.org/10.2307/2951599
- Ahrens, A., Hansen, C. B., & Schaffer, M. E. (2018). Pdslasso and ivlasso: Progams for post-selection and post-regularization OLS or IV estimation and inference. Retrieved 05 July 2022, from http://ideas.repec.org/c/boc/bocode/s458459.html
- Ahrens, A., Hansen, C. B., & Schaffer, M. E. (2020). lassopack: Model selection and prediction with regularized regression in Stata. The Stata Journal: Promoting Communications on Statistics and Stata, 20(1), 176–235. https://doi.org/10.1177/1536867X20909697
- Akinsola, F. A., & Odhiambo, N. M. (2017). Inflation and economic growth: A review of the international literature. Comparative Economic Research Central and Eastern Europe, 20(3), 41–56. https://doi.org/10.1515/cer-2017-0019
- Anakpo, G., & Oyenubi, A. (2022). Technological innovation and economic growth in Southern Africa: Application of panel dynamic OLS regression. Development Southern Africa, 39(4), 543–557. https://doi.org/10.1080/0376835X.2022.2052017
- Arrow, K. J. (1962). The economic implications of learning by doing. The Review of Economic Studies, 29(3), 155–173. https://doi.org/10.2307/2295952
- Baltagi, B. H. (1995). Econometric analysis of panel data. Wiley. https://books.google.co.za/books?id=3sHZnQEACAAJ
- Belloni, A., Chernozhukov, V., & Hansen, C. (2014). Inference on treatment effects after selection among high-dimensional controls. The Review of Economic Studies, 81(2), 608–650. https://doi.org/10.1093/restud/rdt044
- Belloni, A., Chernozhukov, V., Hansen, C., & Kozbur, D. (2016). Inference in high-dimensional panel models with an application to gun control. Journal of Business & Economic Statistics, 34(4), 590–605. https://doi.org/10.1080/07350015.2015.1102733
- Bertoletti, A., Berbegal-Mirabent, J., & Agasisti, T. (2022). Higher education systems and regional economic development in Europe: A combined approach using econometric and machine learning methods. Socio-Economic Planning Sciences, 82, 101231. https://doi.org/10.1016/j.seps.2022.101231
- Bittencourt, M. (2012). Inflation and economic growth in Latin America: Some panel time-series evidence. Economic Modelling, 29(2), 333–340. https://doi.org/10.1016/j.econmod.2011.10.018
- Brenner, T. (2014). Science, innovation and national growth. Working Papers on Innovation and Space, No. 03.14, Philipps-University Marburg, Department of Geography, Marburg.
- Chen, D. H., & Kee, H. L. (2005). A model on knowledge and endogenous growth. SSRN Electronic Journal, Available at SSRN: https://doi.org/10.2139/ssrn.678901
- Chernozhukov, V., Hansen, C., & Spindler, M. (2015). Post-selection and post-regularization inference in linear models with many controls and instruments. American Economic Review, 105(5), 486–490. https://doi.org/10.1257/aer.p20151022
- Drogovoz, P., Yusufova, O., Kashevarova, N., & Shiboldenkov, V. (2019 Exploratory data analysis of national indicators referred to scientific and technological development and to economic growth [Paper presentation]. AIP Conference Proceedings.
- European Bank for Reconstruction and Development. (2019). Introducing the EBRD knowledge economy index. Retrieved 25 August 2022, from https://www.ebrd.com/news/publications/brochures/ebrd-knowledge-economy-index.html
- Gholizadeh, H., Salehi, H., Embi, M. A., Danaee, M., Motahar, S. M., Ebrahim, N. A., Tanha, F. H., & Abu Osman, N. A. (2014). Relationship among economic growth, internet usage and publication productivity: Comparison among ASEAN and world’s best countries. Modern Applied Science, 8(2), 160–170. https://doi.org/10.5539/mas.v8n2p160
- Grossman, G. M., & Helpman, E. (1991). Trade, knowledge spillovers, and growth. European Economic Review, 35(2–3), 517–526. https://doi.org/10.1016/0014-2921(91)90153-A
- Hastie, T., Tibshirani, R., & Wainwright, M. (2015). Statistical learning with sparsity. Monographs on Statistics and Applied Probability, 143, Taylor & Francis Group.
- Hatemi-J, A., Ajmi, A. N., El Montasser, G., Inglesi-Lotz, R., & Gupta, R. (2016). Research output and economic growth in G7 countries: New evidence from asymmetric panel causality testing. Applied Economics, 48(24), 2301–2308. https://doi.org/10.1080/00036846.2015.1117052
- Hausman, N. (2022). University innovation and local economic growth. The Review of Economics and Statistics, 104(4), 718–735. https://doi.org/10.1162/rest_a_01027
- Hazan, E., Smit, S., Woetzel, J., Cvetanovski, B., Krishnan, M., Gregg, B., Perrey, J., & Hjartar, K. (2021). Getting tangible about intangibles: the future of growth and productivity? Retrieved 25 August 2022, from https://www.mckinsey.com/business-functions/growth-marketing-and-sales/our-insights/getting-tangible-about-intangibles-the-future-of-growth-and-productivity
- Henningsen, A., & Henningsen, G. (2019). Chapter 12 - Analysis of panel data using R. In M. Tsionas (Ed.), Panel data econometrics (pp. 345–396). Academic Press. https://doi.org/10.1016/B978-0-12-814367-4.00012-5
- Inglesi-Lotz, R., Balcilar, M., & Gupta, R. (2014). Time-varying causality between research output and economic growth in US. Scientometrics, 100(1), 203–216. https://doi.org/10.1007/s11192-014-1257-z
- Inglesi-Lotz, R., Chang, T., & Gupta, R. (2015). Causality between research output and economic growth in BRICS. Quality & Quantity, 49(1), 167–176. https://doi.org/10.1007/s11135-013-9980-8
- Inglesi-Lotz, R., & Pouris, A. (2013). The influence of scientific research output of academics on economic growth in South Africa: An autoregressive distributed lag (ARDL) application. Scientometrics, 95(1), 129–139. https://doi.org/10.1007/s11192-012-0817-3
- International Monetary Fund. (2021). World economic outlook: Recovery during a pandemic—health concerns, supply disruptions, price pressures. International Monetary Fund.
- International Monetary Fund. (2022). World economic forum. Retrieved 29 August 2022, from https://www.imf.org/en/Publications/WEO/Issues/2022/07/26/world-economic-outlook-update-july-2022
- Jin, J. C. (2009). Economic research and economic growth: Evidence from East Asian economies. Journal of Asian Economics, 20(2), 150–155. https://doi.org/10.1016/j.asieco.2008.12.002
- Jin, J. C. (2010). Research publications, economic growth and causality: Japan’s experience. Pacific Economic Review, 15(5), 666–673. https://doi.org/10.1111/j.1468-0106.2010.00522.x
- Jin, J. C., & Jin, L. (2013). Research publications and economic growth: Evidence from cross-country regressions. Applied Economics, 45(8), 983–990. https://doi.org/10.1080/00036846.2011.613785
- Jin, J. C., & Jin, L. (2014). On the relationship between university education and economic growth: The role of professors’ publication. Education Economics, 22(6), 635–651. https://doi.org/10.1080/09645292.2012.697646
- Jones, C. I. (2022). The past and future of economic growth: A semi-endogenous perspective. Annual Review of Economics, 14(1), 125–152. https://doi.org/10.1146/annurev-economics-080521-012458
- Kim, Y. K., & Lee, K. (2015). Different impacts of scientific and technological knowledge on economic growth: Contrasting science and technology policy in East Asia and Latin America. Asian Economic Policy Review, 10(1), 43–66. https://doi.org/10.1111/aepr.12081
- Kumar, R. R., Stauvermann, P. J., & Patel, A. (2016). Exploring the link between research and economic growth: An empirical study of China and USA. Quality & Quantity, 50(3), 1073–1091. https://doi.org/10.1007/s11135-015-0191-3
- Lee, L. C., Lin, P. H., Chuang, Y. W., & Lee, Y. Y. (2011). Research output and economic productivity: A Granger causality test. Scientometrics, 89(2), 465–478. https://doi.org/10.1007/s11192-011-0476-9
- Lewis, J., Schneegans, S., & Straza, T. (2021). UNESCO science report: The race against time for smarter development (Vol. 2021). UNESCO Publishing.
- Lucas, R. E. (1988). On the mechanics of economic development. Journal of Monetary Economics, 22(1), 3–42. https://doi.org/10.1016/0304-3932(88)90168-7
- Maradana, R. P., Pradhan, R. P., Dash, S., Gaurav, K., Jayakumar, M., & Chatterjee, D. (2017). Does innovation promote economic growth? Evidence from European countries. Journal of Innovation and Entrepreneurship, 6(1), 1–23. https://doi.org/10.1186/s13731-016-0061-9
- North, D. C. (1990). Institutions, institutional change and economic performance. Cambridge University Press.
- Ntuli, H., Inglesi‐Lotz, R., Chang, T., & Pouris, A. (2015). Does research output cause economic growth or vice versa? Evidence from 34 OECD countries. Journal of the Association for Information Science and Technology, 66(8), 1709–1716. https://doi.org/10.1002/asi.23285
- Odhiambo, N. M., & Ntenga, L. (2016). Research publications and economic growth in South Africa: An empirical investigation. International Journal of Social Economics, 43(7), 662–675. https://doi.org/10.1108/IJSE-05-2014-0103
- OECD. (2013). New sources of growth: Knowledge-based capital. Retrieved 25 August 2022, from https://www.oecd.org/sti/inno/knowledge-based-capital-synthesis.pdf
- Ogot, M., & Onyango, G. M. (2022). Does universities’ research output aligned to national development goals impact economic productivity? Evidence from Kenya. Journal of Asian and African Studies, 58(6), 1005–1020. https://doi.org/10.1177/00219096221080196
- Oluwatobi, S., Olurinola, I., Alege, P., & Ogundipe, A. (2020). Knowledge-driven economic growth: The case of Sub-Saharan Africa. Contemporary Social Science, 15(1), 62–81. https://doi.org/10.1080/21582041.2018.1510135
- Ouedraogo, I., Tabi, H. N., Ondoa, H. A., & Jiya, A. N. (2022). Institutional quality and human capital development in Africa. Economic Systems, 46(1), 100937. https://doi.org/10.1016/j.ecosys.2021.100937
- Pinto, T., & Teixeira, A. A. (2020). The impact of research output on economic growth by fields of science: A dynamic panel data analysis, 1980–2016. Scientometrics, 123(2), 945–978. https://doi.org/10.1007/s11192-020-03419-3
- Pradhan, R. P., Arvin, M. B., Nair, M., & Bennett, S. E. (2020). The dynamics among entrepreneurship, innovation, and economic growth in the Eurozone countries. Journal of Policy Modeling, 42(5), 1106–1122. https://doi.org/10.1016/j.jpolmod.2020.01.004
- Ranstam, J., & Cook, J. (2018). LASSO regression. British Journal of Surgery, 105(10), 1348–1348. https://doi.org/10.1002/bjs.10895
- Reichert, S. (2019). The role of universities in regional innovation ecosystems. EUA Study, European University Association.
- Romer, P. M. (1986). Increasing returns and long-run growth. Journal of Political Economy, 94(5), 1002–1037. http://www.jstor.org/stable/1833190 https://doi.org/10.1086/261420
- Romer, P. M. (1990). Endogenous technological change. Journal of Political Economy, 98(5, Part 2), S71–S102. http://www.jstor.org/stable/2937632 https://doi.org/10.1086/261725
- Salvatore, D. (2014). International economics: trade and finance. Wiley.
- Solarin, S. A., & Yen, Y. Y. (2016). A global analysis of the impact of research output on economic growth. Scientometrics, 108(2), 855–874. https://doi.org/10.1007/s11192-016-2002-6
- Solow, R. M. (1956). A contribution to the theory of economic growth. The Quarterly Journal of Economics, 70(1), 65–94. https://doi.org/10.2307/1884513
- Swan, T. W. (1956). Economic growth and capital accumulation. Economic Record, 32(2), 334–361. https://doi.org/10.1111/j.1475-4932.1956.tb00434.x
- Tibshirani, R. (1996). Regression shrinkage and selection via the lasso. Journal of the Royal Statistical Society: Series B (Methodological), 58(1), 267–288. https://doi.org/10.1111/j.2517-6161.1996.tb02080.x
- Tunali, Ç. B. (2016). Do scientific products contribute to the level of output? Empirical evidence from the European Union Countries. Advances in Management and Applied Economics, 6(3), 59.
- Schlegel, F., & Unesco. (2015). UNESCO science report: towards 2030. UNESCO Publishing.
- Wang, X., Ren, Y., Zhang, L., & Ma, Y. (2020). The influence of technological innovation ability of universities on regional economic growth: Take coastal provinces and cities for example. Journal of Coastal Research, 103(sp1), 112–116. https://doi.org/10.2112/SI103-023.1
- White, K. (2019). Publications output: US trends and international comparisons. Science & Engineering Indicators 2020. NSB-2020-6. National Science Foundation.
- World Bank, & Elsevier. (2014). A decade of development in sub-Saharan African science, technology, engineering and mathematics research. The World Bank and Elsevier.
- Yaşgül, Y. S., & Güriş, B. (2016). Causality between research output in the field of biotechnology and economic growth in Turkey. Quality & Quantity, 50(4), 1715–1726. https://doi.org/10.1007/s11135-015-0230-0
- Zaman, K., Khan, H. U. R., Ahmad, M., & Aamir, A. (2018). Research productivity and economic growth: A policy lesson learnt from across the globe. Iranian Economic Review, 22(3), 627–641.