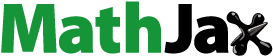
Abstract
Over the past few decades, enhancing the sustainability of concrete structures has become a worldwide necessity. This study proposes a mathematical model for predicting compressive strength (CS), aiming to further the objective of designing sustainable concretes incorporating silica-fume as a partial cementing replacement material. The article outlines the formulation, calibration, evaluation and validation of the proposed model. Various factors related to concrete mixture and age were considered in the formulation of the CS model, which employed multiple sub-models including a cement hydration model that considers cement chemical composition and hydration rate, along with other factors like aggregate packing density, capillary porosity, air pores, standard cement strength, paste-to-aggregate bond strength and presence of supplementary cementing materials. An experimental program consisting of 10 different concrete mixtures was designed to calibrate and evaluate the model. The model was then validated using databases from multiple literature sources, which consisted of 50 data points with diverse materials and mixture proportions, to test its accuracy and generalization capability. Results show that the proposed model closely matches the experimental data and has no sign of anomalies or distinct trends. The model’s coefficient of determination and standard error are 0.97 and 4.0 MPa, respectively. Moreover, model validation demonstrates high predictability and generalization capability, with a corresponding coefficient of determination and standard error of 0.93 and 4.4 MPa, respectively. Overall, this research suggests that the proposed mathematical model is a reliable tool to predict the CS of sustainable concretes that utilize silica-fume as a partial cementing replacement material.
1. Introduction
In recent decades, enhancing the sustainability of structures has evolved into a global imperative. The escalating world population and ongoing product advancements have fueled an augmented demand for cement, a pivotal constituent in concrete structures. However, cement processing carries an adverse environmental footprint, characterized by high energy inefficiency and the release of detrimental pollutants like CO2 gases, CO, NOx and SO2 (Environmental Protection Agency [EPA], 2020). The emitted CO2 ranges from 760 to 1100 kg per metric tonne of cement, contingent upon the processing method and utilized fuel. Given the construction industry’s substantial contribution to CO2 emissions and the persistent demand for cement, these emissions are anticipated to increase significantly in the near future (EPA, 2020). Environmental sustainability necessitates cutting-edge innovations to curtail CO2 emissions and energy consumption (Moutassem & Alamara, Citation2021). A viable avenue to enhance concrete structure sustainability involves partially substituting cement with supplementary cementitious materials such as Pozzolans (Seifan et al., Citation2020). ACI 234R-96 (2000) points to the potential of high-range water-reducing admixtures, enabling the incorporation of silica fume (SF) into concrete mixes as a partial cementing replacement material, yielding highly sustainable concretes renowned for their strength and durability. The advantages of utilizing SF include improved compressive and tensile strength, denser particle packing, heightened resistance to chemical aggression, enhanced durability and reduced CO2 emissions (Moutassem & Miqdadi, Citation2020; Nafees et al., Citation2022). Leveraging SF to fortify concrete structures against premature degradation enhances structural sustainability, mitigating the overall environmental impact over the lifespan of the concrete (Moutassem, Citation2020a, Citation2020b; Moutassem & Miqdadi, Citation2020).
Researchers play a crucial role in continuously improving structures for present and future generations by enabling the sustainable use of resources through appropriate design technologies. Concrete compressive strength (CS), a crucial metric in evaluating concrete mixture performance, is extensively employed by structural engineers in designing concrete structures (ACI 211.1-91, Citation1991). With the development of new, more sustainable concretes, it is necessary to improve models’ predictive capabilities to account for the mixture composition and the chemical and physical characteristics of concrete mixtures incorporating cementing replacement materials. CS of concrete mixtures depends on several design variables, such as aggregate maximum size and gradations, mixture proportions, entrapped and entrained air, and concrete placement protocol, including mixing, placing and compacting the mixture (de Larrard, Citation1999). The bond strength of the hydrated cement depends on the cement’s chemical and physical composition, degree of hydration, and aggregate types, sizes and shapes (Larrard & Belloc, Citation1997). Additionally, the aggregate strength depends on the type, maximum size and shape of the aggregate.
This study aims to create a viable approach for measuring the CS of concrete mixtures that include micro-silica as a partial substitute for cement. This article is broken into three sections that summarize the study findings. The first section looks into existing models and their weaknesses. The second section describes the mathematical formulation and development of the suggested model. The last part comprises an experimental program to fine-tune and assess the proposed model. Additionally, this section will incorporate experimental data to assess the proposed model’s ability to generalize.
2. Literature review – a brief overview
Extensive research has been conducted in literature to predict the CS of concrete. The evolution of CS models stems from a conceptual understanding of particle–cement interactions, identifying key controlling parameters. Feret (Citation1892) introduced a model postulating that concrete strength is a function of the cement-to-cement paste and air ratio; however, empirical data did not support this ratio (Popovics, Citation1985). Abrams (Citation1919) proposed replacing this ratio with the water-to-cement ratio, which better aligns with CS data. Nonetheless, Abrams’ model overlooks numerous factors such as cement type and strength, aggregate gradations, proportions, admixtures and concrete age.
Powers developed a model linking the CS of cement paste directly to the gel volume ratio to capillary porosity volume (Powers & Brownyard, Citation1960), requiring the determination of the degree of hydration, α. Tango (Citation2000) simplified Powers’ law by substituting for α, however, the model still requires calibration parameters and neglects cement and aggregate properties. Popovics (Citation1998) created a mathematical model based on Abrams’ model, incorporating some factors that influence the CS, which was later refined by empirically adding cement content parameters. Pann et al. (Citation2003) incorporated cement paste capillary porosity empirically through a statistical model.
de Larrard (Citation1999) postulated that added cement paste between two adjacent aggregates would become highly stressed and that the distance between these aggregates is the maximum paste thickness (MPT). Accordingly, a model was formulated to calculate MPT, which depends on the maximum aggregate size, volume fraction of aggregate (ϕ) and maximum packing density of aggregates (ϕ*). This effect of aggregates through MPT was incorporated into Feret’s model. Additionally, Feret’s ‘A’ coefficient was proposed to be the product of aggregate bonding strength (K) and standard cement strength at 28 d (Rc28). The model also considered the effect of age on strength empirically through fitting of strength versus time data. However, despite significant improvements, de Larrard’s model did not fundamentally account for the chemical properties of cement and the degree of cement hydration. Moreover, its predictive capability for the effect of age on strength is limited by the range of the experimental data, making it potentially unsuitable for extrapolation. Mechling et al. (Citation2009) revised de Larrard’s model, including the influence of C3S content on the strength of hydrated cement paste.
Chidiac et al. (Citation2013) later proposed a mathematical model for concrete mixtures and aggregate gradation based on packing density, presenting the most comprehensive model with the highest correlation (Moutassem & Chidiac, Citation2016). It was postulated that particles interact according to the excess paste theory, where concrete is considered a mixture of aggregate and cement paste, with excess paste dispersing and lubricating the mixture (Chidiac et al., Citation2013). A mathematical model for the average paste thickness (APT) was also developed, accounting for concrete mixtures and aggregate gradation through packing density. The proposed model by Chidiac et al. (Citation2013) consisted of two fundamental models: a cement hydration model and an APT model. It also considered the paste-to-aggregate bond strength (K) and the cement strength at 28 d (Rc28) adopted from de Larrard (Citation1999). Water-filled capillary pores and entrapped and entrained air were also included through the w/c and EA variables, respectively. Despite its comprehensiveness and accuracy, Chidiac et al.’s model does not account for micro-silica, which is crucial for producing sustainable concrete in construction.
Literature also features models accounting for SF in concrete. Pareek and Hong (Citation2020) presented a CS model using linear regression analysis with 9 calibration constants. Nafees et al. (Citation2022) developed nonlinear regression models using artificial neural networks (ANNs) to predict the mechanical properties of SF concretes. The incorporation of SF plays a vital role in producing sustainable concrete in construction. Machine learning algorithms were employed to predict CS after adding SF, promoting the use of green concrete. Chiya et al. (Citation2022) proposed various models for predicting the CS of cement pastes containing micro-silica and nano-silica, with the ANN model demonstrating the highest degree of correlation.
Several other studies were conducted for other concretes that did not utilize SF. Iftikhar et al. (Citation2023) utilized artificial intelligence to develop empirical models using gene expression programming (GEP) and multi-expression programming (MEP), to predict the CS of plastic sand paver blocks, achieving R2 values of 0.87 for GEP and 0.91 for MEP. Jaf et al. (Citation2023) conducted a study to evaluate the impact of silicon dioxide (SiO2) and calcium oxide (CaO) in fly ash on the CS of green concrete through the examination of a large set of data using four different models, concluding that the ANN model proved most effective and accurate, emphasizing the potential of machine learning for precise CS predictions. Zrar et al. (Citation2024) explored the utilization of fly ash, ground granulate blast furnace slag and waste rubber in self-compacted concrete (SCC) production, employing four models including linear regression, multi-linear regression, full quadratic and M5P-tree, with the full quadratic model identified as the most effective in predicting CS and other mechanical properties of SCC, highlighting the importance of alternative materials in sustainable construction practices.
Based on the above and the review of different models in terms of their completeness, accuracy and physical basis, it is determined that the model proposed by Moutassem and Chidiac (Citation2016) stands out as one of the most superior models. Accordingly, the proposed model in this article utilizes a similar technique and then improves on the model by incorporating microsilica parameters and then reshaping the model to make it simpler and more practical to apply while still maintaining the high degree of accuracy.
3. Developing a model for compressive strength
The proposed model for CS takes into account various factors related to the concrete mixture and age to enhance its accuracy. To achieve a comprehensive model, several sub-models will be employed. Among these, a cement hydration model will be developed, considering the chemical composition of the cement and its hydration rate at any given concrete age (t). Additional sub-models will encompass factors such as the packing density of aggregates, capillary porosity, air pores, standard cement strength, paste-to-aggregate bond strength and the presence of supplementary cementing materials. Consequently, the proposed CS model will be formulated as a function of these variables, as follows:
(1)
(1)
where
f’c (t) = Compressive strength of concrete
K = paste to aggregate bond strength constant
Rc28 = Standard cement strength at 28 d
α(t) = degree of cement hydration at time t
Va = volume proportion of confined and/or entrained air in relation to the mixture’s overall volume
w/c = water to cementing materials ratio
ϕ = packing density of aggregates
WSF = Weight fraction of SF supplementary cementing material.
3.1. Compressive strength and cement hydration
Numerous research work have explored the impact of cement hydration on the evolution of concrete CS (Mehta & Monteiro, Citation2006). The degree of cement hydration (α(t)), representing the proportion of hydrated cement relative to the original amount, is influenced by diverse factors, including cement composition and fineness, water-to-cement ratio (w/c), type and quantity of admixtures, concrete age, temperature and moisture content (Schindler & Folliard, Citation2015). Additionally, there is evidence of a bi-linear correlation between the CS of concrete and α(t), with the relationship further influenced by the w/c ratio (Beek et al., Citation1999).
Studies indicate that the initiation of cement hydration and strength development occurs when α(t) exceeds a critical degree of hydration, αcr (Rasmussen et al., Citation2002). For ordinary Portland cement, αcr can be approximated by multiplying the w/c ratio by a constant value of 0.43, as suggested by prior research (Rasmussen et al., Citation2002). Thus, the relationship between concrete CS and the degree of cement hydration, as investigated in this study, can be expressed as follows:
(2)
(2)
where
α(t) = degree of cement hydration at time t
αcr = critical degree of cement hydration
This study adopted the cement hydration prediction model Schindler and Folliard developed due to its thoroughness and strong predictability (Schindler & Folliard, Citation2015).
3.2. Effect of air pores and capillary pores on compressive strength
The amount of water in concrete plays a crucial role in determining the ratio of capillary pores to cementing materials. As the water-to-cement ratio rises, there is an increase in capillary pores, leading to a reduction in the CS of the concrete (de Larrard, Citation1999). Additionally, increasing the amount of entrapped and/or entrained air pores further reduces the CS of the concrete. In this study, Popovics’ strength relationship (Popovics & Ujhelyi, Citation2008), a modification of the Abrams model (Abrams, Citation1919), was adopted to account for these influences:
(3)
(3)
Here, (w + Va)/c represents the weight fractions of water and air relative to the cementing materials in the concrete mixture, and A is a calibration constant that depends on the test conditions and specimen shape.
3.3. Effect of bond strength and cement paste strength on compressive strength
The strength of the bond between aggregates and paste is influenced by several factors, including the size, shape and surface roughness of the aggregates. Utilizing larger aggregates leads to a reduction in specific surface area, resulting in a decrease in bond strength (Quiroga, Citation2003). Generally, rough and crushed aggregates exhibit higher bond strength compared to smooth and round aggregates (de Larrard, Citation1999; Quiroga, Citation2003). To address the impact of aggregate type on the paste-to-aggregate bond strength, de Larrard (Citation1999) suggested the use of a bonding constant, denoted as K. Additionally, de Larrard (Citation1999) took into account the standard cement strength at 28 d, Rc28, as an indicator of the cement paste strength. In this study, we followed de Larrard’s approach and incorporated both K and Rc28 into the strength prediction model, expressed as:
(4)
(4)
where
K = Constant for paste-to-aggregate bond strength
Rc28 = Standard cement strength at 28 d
3.4. Effect of packing density of aggregates on compressive strength
The packing density of aggregates in a mixture serves as an indirect indicator of porosity, taking into account various mixture parameters such as aggregate gradations and the proportions of cement and aggregates (Larrard & Belloc, Citation1997). Numerous studies have demonstrated that achieving the optimum packing density of aggregates contributes to the optimal strength of concrete by reducing porosity (Johansen & Andersen, Citation1996; Tasi et al., Citation2006; Wong & Kwan, Citation2005). Increasing the density of aggregates in the mixture enhances the concrete’s strength, which can be achieved by reducing the water-to-cement ratio (w/c) without compromising the workability of the mixture (de Larrard, Citation1999; Moutassem, Citation2010). Additionally, research indicates that strength is correlated with the ratio of the packing density of aggregates in a concrete mixture to its maximum packing density, denoted as ϕ/ϕmax (Chidiac et al., Citation2013; de Larrard, Citation1999; Moutassem, Citation2010). Studies have also shown that the packing density of aggregates not only influences strength but also influences other properties of concrete such as durability and UPV (de Larrard, Citation1999; Moutassem & Miqdadi, Citation2023).
For a given mixture, the maximum packing density of aggregates, ϕmax, is influenced by factors such as size, shape, surface texture, volume fraction of solids and the compaction method (de Larrard, Citation1999; Wong & Kwan, Citation2005). Its calculation involves employing an appropriate particle packing model, such as the Compressible Packing Model (CPM), the Modified Toufar Model (MTM) or the Theory of Particle Mixtures Model (TPM) (Moutassem, Citation2016). In this study, the MTM (Toufar et al., Citation1976) was selected due to its simplicity, high predictability and generic form relative to other models. Thus, in this study, the CS of concrete is related to the packing density ratio of aggregates in the following manner:
(5)
(5)
where
ϕ = packing density of aggregates
ϕmax = maximum packing density of aggregates
B = a calibration constant that depends on the shape of the aggregate particles.
3.5. Effect of silica fume on compressive strength
In modern construction practices, supplementary cementing materials play a pivotal role in enhancing the strength, durability and sustainability of concrete structures. Commonly utilized materials for this purpose include fly ash, granulated blast furnace slag (GGBS) and SF. While the Schindler and Folliard cement hydration model (Schindler & Folliard, Citation2015) incorporates fly ash and GGBS, it neglects the consideration of SF, a by-product derived from the production of silicon metal and ferro-silicon alloys. However, numerous studies have established that the addition of SF or micro-silica can significantly improve both the strength and durability of concrete. Hence, in this study, to account for SF as a partial replacement for cement, the following relationship is considered:
(6)
(6)
WC is the weight of cementing materials in the concrete mix (kg/m3), C is a calibration constant and WSF is the weight of SF in the concrete mix (kg/m3).
3.6. Model of compressive strength
By integrating the previously mentioned relationships and applying statistical regression, the following proposed model was developed for forecasting the CS of concrete at any point in time:
(7)
(7)
where A, B and C are calibration constants. The units of f’c and Rc28 are in MPa (SI units) or psi (US units). The equation includes dimensionless terms for the parameters K, α(t), αcr and all ratios.
4. Experimental program
In order to assess the accuracy of the proposed model in predicting concrete CS, an experimental program was conducted. This program involved the adjustment of design variables for proportioning concrete mixtures, including the water-to-cementing materials ratio, water content, air entrainment, and the quantity of SF admixture. Additionally, a high-range water reducer (HRWR) was introduced to improve the workability of the dry mixtures.
4.1. Composition of concrete mixture
shows the details of 10 different concrete mixtures proportioned according to the ACI 211 guidelines. The water-to-cement ratio (w/c) of the mixtures was either 0.4 or 0.3, and the content of the cementitious material ranged from 375 to 513 kg/m3. The percentage of cement replacement with SF in the mixtures containing SF was 15%. The table also displays the quantities of cement, coarse aggregate (CA), fine aggregates (FA) and air-entraining admixture (AEA) used in each mixture.
Table 1. Concrete mixtures composition.
4.2. Concrete materials and properties
The concrete employed in this investigation was manufactured using crushed limestone as CA and well-graded concrete sand as FA, featuring a maximum aggregate size of 19 mm. The specific gravity, absorption value and bulk density of CA were recorded as 2.68, 1.2% and 1600 kg/m3, respectively. Similarly, FA exhibited specific gravity, absorption value and bulk density values of 2.67, 2.5% and 1800 kg/m3, respectively, along with a fineness modulus of 2.82. The determination of specific gravities, absorption values and bulk densities for CA and FA followed ASTM C127 (American Society for Testing of Materials [ASTM], 2015a) and ASTM C128 (ASTM, 2015b) standards. Particle size distributions were assessed using the sieve analysis test standard (ASTM, 2014a), ensuring compliance with the required specifications.
Ordinary Portland cement Type I, adhering to ASTM C150/C150M (ASTM, 2016b) standards, was utilized, possessing a specific gravity of 3.15 and a 28-d CS (Rc28) of 40 MPa. Densified silica fume powder, in accordance with ASTM C1240 (ASTM, 2015c), was incorporated in half of the mixtures. The high-range water-reducing agent (HRWR) used, named Master-Glenium 7920, met the stipulations of ASTM C494/C494M (ASTM, 2015d), facilitating a 75–100 mm slump and ensuring workability without segregation. In certain mixtures, an AEA (Master Air AE 200) was introduced, complying with ASTM C260/C260M (ASTM, 2010).
4.3. Experimental approach
The same experimental procedures were applied to all 10 concrete mixtures. The mixing and placement followed the guidelines of ASTM C192/C192M (ASTM, 2016a). The amount of slump, which measures the consistency of the concrete, and air content were determined according to ASTM C143/C143M (ASTM, 2015e) and ASTM C231/C231M (ASTM, 2014c), respectively. Once cast and placed, the concrete specimens were sealed and cured in a standard curing room with a temperature of 23 °C and relative humidity above 95%. Three standard cylinders measuring 100 × 200 mm for each concrete mixture were made, consolidated by rodding and finished according to ASTM C192/C192M (ASTM, 2016a). The CS of the concrete was then evaluated 28 d later using the testing method described in ASTM C39/C39M (ASTM, 2016c).
5. Analysis and discussion of the experimental results
To calibrate the constants of the proposed CS model, the standard error (σ) was minimized by comparing the measured experimental values with the model’s predicted values. The standard error provided a comprehensive evaluation of the model predictions and was calculated using the equation (Montgomery & Runger, 2003):
(8)
(8)
where f’c(t)model(i) and f’c(t)exp(i) are the model and experimental CS values corresponding to mix i, respectively. The parameters n and p denote the number of test points and the number of model constants, respectively. In the assessment of the proposed model, a global measure was employed to evaluate its validity and goodness of fit. The coefficient of determination (R2) was also computed to assess the alignment of the model’s predictions with actual data. These metrics were utilized to measure the model’s capability to predict trends and compare them to those documented in the literature. The calibration constants K, A, B and C were determined through the least square error method, yielding values of 19.7, 0.032, 1.57 and 2.5, respectively. The standard error (σ) and coefficient of determination (R2) were identified as 4.0 MPa and 0.97, respectively.
displays the results of the experimental data and model predictions for each mix, along with the corresponding calculated values for the model parameters: α(t)-αcr, (w + Va)/c, ϕ/ϕmax and WSF/WC. The table also includes the model error, which is the ratio of experimental to predicted results for each mix. The mean, standard deviation and coefficient of variation for the model error are 1.002, 0.048 and 4.77, respectively. The percentage difference between predicted and measured CS values ranged from 0.7% to 6.8%. These results confirm the proposed model’s high predictability.
Table 2. Experimental vs. predicted concrete compressive strength at 28 d.
As depicted in , the proposed model exhibits strong alignment with the measured experimental data, providing additional confirmation of its capability to predict concrete CS at 28 d. The corresponding standard error and coefficient of determination are 4.03 MPa and 0.97, respectively. The robustness of the model was evaluated by comparing predicted f’c values to residual f’c values, as demonstrated in . Notably, the residuals are dispersed randomly around zero, indicating an absence of discernible patterns or outliers. This observation validates the model’s good fitness. These outcomes further reinforce the efficacy of the proposed model in accurately predicting concrete CS.
The importance of the model input parameters and their impact on the model predictions was evaluated through two methods. In the first approach, the input parameters were individually plotted against the outcome to explore potential correlations and ascertain the coefficient of determination for the best-fit relationship. It was revealed that multiple correlations existed and that the order of highest to lowest degree of correlation between model parameters and outcome were
and
respectively. For the second method, each input parameter was removed from the model and the model was recalibrated using the remaining three parameters and then the corresponding model standard error was determined. This method assisted in identifying the most influential parameters on the model prediction. It was revealed that the most influential model parameters in order of importance were
and
respectively.
Furthermore, the evaluation of the model included an examination of the trend and systematic deviation from the exact location. Both and demonstrate that the model adheres to a similar trend as the experimental data, displaying no systematic deviation. The assessment of the systematic deviation of the model from the exact location involved determining the regression coefficients in the linear regression model. As indicated in , the 95% confidence interval for the intercept encompasses zero, and the 95% confidence interval for the slope encompasses one. Additionally, a statistical analysis of the model as a whole indicated that the proposed model significantly predicts the outcome, with a p value less than 0.00001.
Table 3. Statistical analysis of model vs. experiment using linear regression.
In addition, the proposed model’s reliability was tested by validating it with experimental data from three different literature sources (ACI 234R-96, Citation2000; Hooton et al., Citation1997; Moutassem & Chidiac, Citation2016), consisting of 50 data points with diverse materials and mixture proportions. illustrates the strong correlation between the model predictions and the experimental data. The coefficient of determination and standard error were 0.93 and 4.4 MPa, respectively. These findings indicate that the proposed model has high predictability and can be applied to various concrete mixtures with great accuracy.
6. Conclusions and future research
In this study, a microstructure model for predicting the CS of sustainable concretes containing silica-fume as partial cement replacement has been formulated, calibrated, evaluated, and validated. The model formulation incorporates the following features:
The effect of cement hydration and concrete age on CS was accounted for by incorporating the cement hydration model developed by Schindler and Folliard (Citation2015) to account for cement’s chemical composition and properties.
A sub-model created by Popovics and Ujhelyi (Citation2008) was utilized to consider the impact of both air pores and capillaries.
The effect of bond strength and cement paste strength were accounted for using the relationship developed by de Larrard (Citation1999).
The effect of other mixture parameters, such as aggregate gradations and proportions of aggregates and cement, which affect the amount of porosity in the mix, was accounted for by incorporating the packing density of aggregates property into the model.
The effect of silica fume as a partial cement replacement on the CS was considered by including it in the model and utilizing a power relationship.
A thorough evaluation and validation of the model revealed the following findings:
The proposed CS model aligns well with the experimental data and shows no outliers or distinctive patterns. The coefficient of determination and standard error of the model are 0.97 and 4.0 MPa, respectively. In addition, the model error has a mean, standard deviation, and coefficient of variation of 1.02, 0.048 and 4.77, respectively.
It was identified that (w + Va)/c, ϕ/ϕmax, WSF/WC and α(t)-αcr are the most influential input parameters on the model prediction, in order of significance.
The model’s systematic deviation from the exact location was measured, and the results demonstrate that the model aligns well with the experimental data and does not show any systematic deviation. Furthermore, the statistical evaluation of the model as a whole suggests that the proposed model can significantly predict the outcome, as the p value was less than 0.00001.
An extensive literature database was used to validate the model, and the results indicate high predictability and generalization capability. The corresponding standard error and coefficient of determination are 4.4 MPa and 0.93, respectively.
The proposed method for predicting CS in sustainable concrete structures, particularly by incorporating silica-fume as a partial cementing replacement material, has significant implications for future research and application in construction materials and sustainability. First, the developed mathematical model serves as a practical tool for engineers and designers to optimize concrete mixtures, considering factors like mixture composition and age to meet both strength and sustainability requirements, potentially leading to widespread adoption of sustainable concrete practices globally. Second, by highlighting the potential of silica-fume and other supplementary cementing materials, the method encourages further research into alternative materials to enhance concrete strength and durability. Moreover, the refinement of the mathematical model opens avenues for future research in optimization, parameter fine-tuning and development of user-friendly software tools for practical implementation. Additionally, the expanded literature review offers insights into existing models and limitations, facilitating comparative studies and the development of more comprehensive predictive models. Overall, the proposed method holds promise for advancing research and application in sustainable concrete construction, contributing to the evolution of construction materials and practices.
Author contributions statement
Both authors of this article (Fayez Moutassem and Mohamad Kharseh) meet the criteria for authorship which includes substantial contributions to the conception, analysis and interpretation of data, drafting the work, reviewing it critically, and final approval of the version to be published. The first author Fayez Moutassem worked on those parts of the article related to model development, experimental program and analysis, in addition to writing approximately 70% of the paper draft. Co-author Mohamad Kharseh helped with the analysis and writing of the paper, in addition to checking the draft version and making several modifications. Fayez Moutassem and Mohamad Kharseh both agree to be accountable for the parts of the work done and in responding to questions in a professional and accurate manner.
Acknowledgments
The authors would like to thank the American University of Ras Al Khaimah for providing the resources and time needed that help shape the development of the proposed model, the analysis and the writing of the article.
Disclosure statement
On behalf of all authors, the corresponding author states that there is no conflict of interest.
Data availability statement
I agree to share the data upon reasonable request. For any inquiries regarding the data used in this study, please contact the first author ‘Fayez Moutassem’.
Additional information
Notes on contributors
Fayez Moutassem
Dr. Fayez Moutassem received his PhD in Civil Engineering from McMaster University, Canada, and his MS from the Texas A&M University, USA. Currently, he is an associate professor and the chair of the Department of Civil and Infrastructure Engineering at the American University of Ras Al Khaimah, UAE. His research interests are in concrete structures and materials including precast and prestressed members, sustainability in design and construction, green buildings, quality control, and in the properties of structural concrete utilizing special materials.
Mohamad Kharseh
Dr. Mohamad Kharseh received his PhD in renewable energy from Lulea University of Technology, Sweden, in 2011. Currently, he is an associate professor in the Mechanical and Industrial Engineering department at the American University of Ras Al Khaimah, UAE. Dr. Mohamad has a wide experience in sustainability and energy consumption systems in buildings and optimizing renewable energy projects to reduce greenhouse emissions using different approaches. Dr. Mohamad’s research resulted in several publications in peer-reviewed journals, conferences, books, and patents.
References
- Abrams, L. D. (1919). Properties of concrete (3rd ed.). Pitman Publishing Ltd.
- ACI 211.1-91. (1991). Standard practice for selecting proportions for normal, heavyweight, and mass concrete. American Concrete Institute.
- ACI 234R-96. (2000). Guide for the use of silica fume in concrete. Manual of concrete practice (pp. 1–51). American Concrete Institute.
- American Society for Testing of Materials (ASTM). (2010). Standard specification for air-entraining admixtures for concrete. ASTM C260/C260M.
- American Society for Testing of Materials (ASTM). (2014a). Standard test method for sieve analysis of fine and coarse aggregates. ASTM C136/C136M.
- American Society for Testing of Materials (ASTM). (2014b). Standard test method for air content of freshly mixed concrete by the pressure method. ASTM C231/C231M.
- American Society for Testing of Materials (ASTM). (2015a). Standard test method for relative sensity (specific gravity) and absorption of coarse aggregate. ASTM C127.
- American Society for Testing of Materials (ASTM). (2015b). Standard test method for relative density (specific gravity) and absorption of fine aggregate. ASTM C128.
- American Society for Testing of Materials (ASTM). (2015c). Standard specification for silica fume used in cementitious mixtures. ASTM C1240.
- American Society for Testing of Materials (ASTM). (2015d). Standard specification for chemical admixtures for concrete. ASTM C494/C494M.
- American Society for Testing of Materials (ASTM). (2015e). Standard test method for slump of hydraulic-cement concrete. ASTM C143/C143M.
- American Society for Testing of Materials (ASTM). (2016a). Making and curing concrete specimens in the laboratory. ASTM C192/C192M.
- American Society for Testing of Materials (ASTM). (2016b). Standard specification for Portland cement. ASTM C150/C150M.
- American Society for Testing of Materials (ASTM). (2016c). Standard test method for compressive strength of cylindrical specimens. ASTM C39/C39M.
- Beek, A., Breugel, K., & Hilhorst, M. A. (1999). Monitoring system for hardening concrete based on dielectric properties. Proceedings of the International Conference Utilizing Ready Mix Concrete and Mortar (pp. 303–312). University of Dundee.
- Chidiac, S. E., Moutassem, F., & Mahmoodzadeh, F. (2013). Compressive strength model for concrete. Magazine of Concrete Research, 65(9), 557–572. https://doi.org/10.1680/macr.12.00167
- Chiya, Y., Rahimzadeh, A. S., & Barzinjy, A. A. (2022). Systematic multiscale models to predict the compressive strength of cement paste as a function of microsilica and nanosilica contents, water/cement ratio, and curing ages. Sustainability, 14(3), 1723. https://doi.org/10.3390/su14031723
- de Larrard, F. (1999). Concrete mixture proportioning: A scientific approach. Spon Press.
- EPA (Environmental Protection Agency). (2020). Emission factor documentation for AP-42, section 11.6 Portland cement manufacturing, AP 42 - compilation of air pollutant emission factors Vol. I: Stationary point and area sources (5th ed.). Washington, DC, 1994; https://www3.epa.gov/ttn/chief/ap42/ch11/bgdocs/b11s06.pdf (accessed 21 March 2020).
- Feret, R. (1892). Sur la compacité des mortiers hydrauliques (On the compactness of hydraulic mortars). Annales Des Ponts et Chaussées, 7(4), 5–164. https://books.google.ae/books/about/Sur_la_compacit%C3%A9_des_mortiers_hydrauliq.html?id=gPmcPQAACAAJ&redir_esc=y
- Hooton, R. D., Pun, P., Kojundic, T., & Fidjestol, P. (1997). Influence of silica fume on chloride resistance of concrete. Proceedings of the PCI/FHWA International Symposium on High Performance Concrete (pp. 245–256), New Orleans, LA, USA.
- Iftikhar, B., Alih, S. C., Vafaei, M., Javed, M. F., Rehman, M. F., Abdullaev, S. S., Tamam, N., Khan, M. I., & Hassan, A. M. (2023). Predicting compressive strength of eco-friendly plastic sand paver blocks using gene expression and artificial intelligence programming. Scientific Reports, 13(1), 12149. https://doi.org/10.1038/s41598-023-39349-2
- Jaf, D. K. I., Abdulrahman, P. I., Mohammed, A. S., Kurda, R., Qaidi, S. M. A., & Asteris, P. G. (2023). Machine learning techniques and multi-scale models to evaluate the impact of silicon dioxide (SiO2) and calcium oxide (CaO) in fly ash on the compressive strength of green concrete. Construction and Building Materials, 400, 132604. https://doi.org/10.1016/j.conbuildmat.2023.132604
- Johansen, V., & Andersen, P. J. (1996). Particle packing and concrete properties", materials science of concrete II (pp. 111–147). American Ceramic Society.
- Larrard, F., & Belloc, A. (1997). The influence of aggregate on the compressive strength of normal and high-strength concrete. ACI Materials Journal, 94(5), 417–425. https://doi.org/10.14359/326
- Mechling, J. M., Lecomte, A., & Diliberto, C. (2009). Relation between cement composition and compressive strength of pure pastes. Cement and Concrete Composites, 31(4), 255–262. https://doi.org/10.1016/j.cemconcomp.2009.02.009
- Mehta, P. K., & Monteiro, P. (2006). Concrete: Microstructure, properties, and materials (3rd ed.). McGraw Hill LLC.
- Montgomery, D. C., & Runger, G. C. (2003). Applied statistics and probability for engineers (3rd ed.). John Wiley and Sons Inc.
- Moutassem, F. (2010). [Packing density links concrete mixture, rheology, and compressive strength] [PhD dissertation]. McMaster University.
- Moutassem, F. (2016). Assessment of packing density models and optimizing concrete mixtures. International Journal of Civil, Mechanical and Energy Science, 2(4), 29–36.
- Moutassem, F. (2020a). Microstructure model for predicting the sorptivity of concrete mixtures. Civil Engineering and Architecture, 8(2), 77–83. https://doi.org/10.13189/cea.2020.080205
- Moutassem, F. (2020b). Ultra-lightweight EPS concrete: Mixing procedure and predictive models for compressive strength. Civil Engineering and Architecture, 8(5), 963–972. https://doi.org/10.13189/cea.2020.080523
- Moutassem, F., & Alamara, K. (2021). Design and production of sustainable lightweight concrete precast sandwich panels for non-load bearing partition wall systems. Cogent Engineering, 8(1), 1993565. https://doi.org/10.1080/23311916.2021.1993565
- Moutassem, F., & Chidiac, S. E. (2016). Assessment of concrete compressive strength predictions methods. KSCE Journal of Civil Engineering, 20(1), 343–358. https://doi.org/10.1007/s12205-015-0722-4
- Moutassem, F., & Miqdadi, I. (2020). Sustainability-model approach for chloride permeability based on concrete mixture. Structures Journal, Elsevier Publishers, 28, 983–990. https://doi.org/10.1016/j.istruc.2020.09.041
- Moutassem, F., & Miqdadi, I. (2023). Mathematical model for predicting the ultrasonic pulse velocity of concrete. Cogent Engineering, 10(1), 2199513. https://doi.org/10.1080/23311916.2023.2199513
- Nafees, A., Amin, M. N., Khan, K., Nazir, K., Ali, M., Javed, M. F., Aslam, F., Musarat, M. A., & Vatin, N. I. (2022). Modeling of mechanical properties of silica fume-based green concrete using machine learning techniques. Polymers, 14(1), 30. https://doi.org/10.3390/polym14010030
- Pann, K. S., Yen, T., Tang, C. W., & Lin, T. D. (2003). New strength model based on water-cement ratio and capillary porosity. ACI Materials Journal, 100(4), 311–318. https://doi.org/10.14359/12669
- Pareek, K., & Hong, Y. M. (2020). Prediction of permeability and compressive strength for pervious concrete. IOP Conference Series: Materials Science and Engineering, 812(1), 012013. https://doi.org/10.1088/1757-899X/812/1/012013
- Popovics, S. (1985). New formulas for the prediction of the effect of porosity on concrete strength. ACI Materials Journal, 82(2), 136–146. https://doi.org/10.14359/10321
- Popovics, S. (1998). History of a mathematical model for strength development of Portland cement concrete. ACI Materials Journal, 95(5), 593–600. https://doi.org/10.14359/401
- Popovics, S., & Ujhelyi, J. (2008). Contribution to the concrete strength versus water-cement ratio relationship. Journal of Materials in Civil Engineering, 20(7), 459–463.) https://doi.org/10.1061/(ASCE)0899-1561(2008)20:7(459)
- Powers, T. C., & Brownyard, T. L. (1960). Studies of the physical properties of hardened Portland cement paste. Proceedings of the American Concrete Institute, 18(2), 669–712.
- Quiroga, P. (2003). [The effect of the aggregate characteristics on the performance of Portland cement concrete] [Ph.D. dissertation]. The University of Texas at Austin.
- Rasmussen, R. O., Ruiz, J. M., Rozycki, D. K., & McCullough, B. F. (2002). Constructing high-performance concrete pavements with FHWA HIPERPAV systems analysis software. Transportation Research Record: Journal of the Transportation Research Board, 1813(1), 11–20. https://doi.org/10.3141/1813-02
- Schindler, A. K., & Folliard, K. J. (2015). Heat of hydration models for cementitious materials. ACI Materials Journal, 102(1), 24–33. https://doi.org/10.14359/14246
- Seifan, M., Mendoza, S., & Berenjian, A. (2020). Mechanical properties and durability performance of fly ash based mortar containing nano and micro-silica additives. Journal of Construction and Building Materials, 252, 119121. https://doi.org/10.1016/j.conbuildmat.2020.119121
- Tango, C. E. S. (2000). Time-generalisation of Abrams’ model for high performance concrete and practical application examples. Proceedings of the International Symposium on High Performance Concrete, Hong Kong University of Science and Technology.
- Tasi, C. T., Li, S., & Hwang, C. L. (2006). The effect of aggregate gradation on engineering properties of high performance concrete. Journal of ASTM International, 3(3), 1–12. https://doi.org/10.1520/JAI13410
- Toufar, W., Born, M., & Klose, E. (1976). Contribution of optimization of components of different density in polydispersed particles systems. Freiberger Booklet A, 558, 29–24.
- Wong, H., & Kwan, A. (2005). Packing density: A key concept for mix design of high performance concrete. Proceedings of Materials Science and Technology in Engineering Conference (MaSTEC). Hong Kong.
- Zrar, Y. J., Abdulrahman, P. I., Sherwani, A. F. H., Younis, K. H., & Mohammed, A. S. (2024). Sustainable innovation in self-compacted concrete: Integrating by-products and waste rubber for green construction practices. Structures, 62, 106234. https://doi.org/10.1016/j.istruc.2024.106234