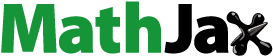
Abstract
The healthcare supply chain begins with the production of medical products. Different stages of supply chain flow may have their own objectives. The healthcare supply chain management process can be inefficient and fragmented because supply chain goals are not always matched within the medical requirements. To choose a certain product, healthcare organizations must consider a variety of demands and perspectives. The MRI team contributes a wealth of knowledge to the essential task of improving our clients’ supply chain and logistics performance. MRI is considered the most efficient medical device for the acquisition and treatment of medical imaging. Medical devices and manufacturing are classified as crucial factors for the supply chain in radiology. The objective of this paper is to present an approach that consists of modeling in 3D a segment of a stenosing aorta with a parallel treatment in order to determine the cost and the time of treatment for the reporting, which can be considered a promoter element to optimize the course of supply chain from manufacture to medical industry. Acceleration has sought to reduce the imaging speed in parallel architecture convergence for cardiac MRI. The image computation time is comparatively long owing to the iterative reconstruction process of 3D models. The aim of this paper is to suggest a CPU-GPU parallel architecture based on multicore to increase the speed of mesh generation in a 3D model of a stenosis aorta. A retrospective cardiac MRI scan with 74 series and 3057 images for a 10-year-old patient with congenital valve and valvular aortic stenosis on close MRI and coarctation (operated and dilated) in the sense of shone syndrome. The 3D mesh model was generated in Standard Tessellation Language (STL), as well as the libraries used to operate with Pymesh and Panda, and the time spent in tracing, decomposing, and finalizing the mesh crucially depends on the number of nuclei used in the parallel processing and the mesh quality chosen. A parallel processing based on four processors are required for the 3D shape refinement.To improve the efficiency of image processing algorithms and medical applications acquired in real-time analysis and control, a hybrid architecture (GPU/GPU) was proposed. The response time of parallel processing based on the CPU-GPU architecture used at the mesh level to achieve a 3D model is critically dependent on the number of kernels required.
Public Interest Statement
Virtual centralization of supply chains, supply utilization management strategies, usage of 3D modeling in medical imaging, analytics, workflow overview, and other emerging are considering trends to reduce costs in healthcare supply chain operations. The adoption of these strategies can provide Medical Industry with cost-effective healthcare solutions.
1. Introduction and literature
1.1. Healthcare supply chain management
The resources required to deliver goods or services to consumers are referred to as supply chains. Managing the supply chain in healthcare is generally complicated and fragmented. Obtaining resources, managing supplies, and delivering goods and services to doctors and patients are all part of the healthcare supply chain management (Lee et al., Citation2011). Physical items and information regarding medical products and services typically pass through a variety of independent parties, including manufacturers, insurance companies, hospitals, providers, group buying groups, and various regulatory bodies, in order to complete the process. Radiologists frequently further down the chain of events that lead the patients to receive a particular imaging scan. The notions of accountable care and value-based imaging will be clarified by examining the whole imaging value chain(Pinna et al., Citation2015) Continuous quality improvement that will enable better clinical outcomes at lower costs. Accountable care entails a keen focus on quality, outcomes, and costs across the entire care continuum, and continuous quality improvement that will enable better clinical outcomes at lower costs (Samuel et al., Citation2010). The MRI team brings a lot of experience to the critical role of enhancing the supply chain and logistics performance of our clients. The most efficient medical equipment for the acquisition and treatment of medical imaging is magnetic resonance imaging (MRI). Medical equipment and manufacturing are among the most important aspects of the supply chain in radiology, as indicated in .
A simulation-based optimization method for identifying important factors for sustainability strategies (Sayyadi & Awasthi, Citation2018). Innovative value-added manufacturing in sustainable development and how an integrated information system can be designed and implemented to support collaboration has been described (Hao et al., Citation2018). With the goal of inventory system management, the optimal lot-sizing of replenishments has a cumulative influence on practical economic production quantity (EPQ) models (Gharaei, Shekarabi et al., Citation2020) with generalized cross decomposition under the separability approach (Gharaei et al., Citation2021). Evaluating and selecting a suitable sustainable supplier is a critical choice that is crucial to the effective management of a sustainable supply chain that consists of a single producer, distributor, and retailer(Shah et al., Citation2020). This research(Rabbani et al., Citation2019) presents a unique interval-valued fuzzy group decision model for evaluating supplier sustainability performance in sustainable supply chain management. This study describes a continuous approximation approach for constructing a model that can solve any issue with any size data collection. The piecewise nonlinear issue was solved using a nonlinear optimization method. The solution techniques and the impacts of the factors on decisions and costs were demonstrated through numerical simulations(Tsao, Citation2015). A supplier, manufacturer, wholesaler, numerous retailers, and collector are all part of a five-level supply chain (SC) are built and optimized. As a result, a multi-stage closed-loop supply chain (CLSC) was created in accordance with green manufacturing principles and a quality control (QC) policy(Amjadian & Gharaei, Citation2021). Evaluating sustainable mobility projects is difficult. The challenge is complicated by the unique setting, the participation of many stakeholders’ aims, confusion regarding the evaluation method and criteria, and a lack of quantitative data(Awasthi & Omrani, Citation2019). The presence of many correlated, constantly changing constituents in this system, as well as their dependency and feedback, adds to the complexity of the problem. System dynamics simulations were used to create the data for the policies. The assessment criteria and alternatives were ranked using the ANP (Sayyadi & Awasthi, Citation2020). The optimal lot-sizing strategy in supply chain management is critical for firms using SC management. SC inventory expenses may be controlled and managed using a good lot-sizing policy (Dubey et al., Citation2015). Many systems are necessary to complete a sequence of tasks with little more than a few minutes between any two assignments. During the breaks, maintenance action is performed on components to enhance the likelihood of the system successfully completing the following mission (Duan et al., Citation2018): Many systems are necessary to complete a sequence of tasks with little more than a few minutes between any two assignments. During the breaks, maintenance action is performed on components to enhance the likelihood of the system successfully completing the following mission(Kazemi et al., Citation2018): A multi-product, multi-buyer supply chain governed by penalty, green, and quality control regulations, and a vendor-managed inventory with a consignment stock agreement. In an integrated multiproduct restricted supply chain, the best batch-sizing policy was found(Gharaei et al., Citation2019). To deal with the challenge of unpredicted parameters, a hybrid robust possibilistic method for a sustainable supply chain location-allocation network design was proposed, as well as a unique approach to uncertainty with possibilistic programming (Rabbani et al., Citation2020). In the manufacturing setting, the impact of reduced ordering costs and improved process quality was described with the development of a model of a stochastic supply chain with imperfect manufacturing and a manageable defect rate(Sarkar & Giri, Citation2020). The centralized model serves as a reference point, while the non-coordinated approach is investigated using a wholesale price-only contract. A repurchase contract increases the overall projected profit and shows that the chain can be coordinated through this contract, despite the fact that the chain is subject to interruption(Giri & Bardhan, Citation2014). Real-world stochastic constraints, such as space constraints, procurement costs, ordering, and combined economic lot-size limitations, can improve the model’s applicability to SCs in the real world. Both the combined economic lot-sizing strategy and the optimal period duration must be determined such that the SC’s overall inventory cost is minimized while stochastic restrictions are met(Gharaei, Karimi et al., Citation2020), and under demand uncertainty, the supply chain coordination problem comprises one producer and several suppliers with quality differences. The manufacturer is unaware of the quantity of defective components acquired from suppliers, whereas each supplier may calculate the standard deviation of defective products (Yin et al., Citation2016). It is observed that learning in production has a significant positive effect on the optimal decisions of the closed-loop supply chain(Giri & Masanta, Citation2020). The goal of the one-machine scheduling issue is to find the optimum sequence for a collection of jobs in a manufacturing system that has only one machine(Gharaei et al., Citation2015).
1.2. Managerial insights for Supply chain: towards CPU-GPU Parallel Architecture for MRI processing
1.2.1. CPU-GPU parallel architecture
As healthcare organizations seek to improve operational efficiency and reduce costs, performance monitoring of healthcare supply chain management (SCM) has become increasingly essential. Healthcare supply chain management is unique because each stakeholder has its own interests to protect. The objectives of the various phases of supply chain flow may differ. Providers may prefer to use a specific product because that is what they have been trained to use, while hospital executives want to acquire the most cost-effective, high-quality products available. In order to keep the quality, the main contribution is to describe an approach to improve the perfremance of MRIs by proposing an advanced methodology of medical imaging treatment based on CFD solvers, 3D modeling with a parallel CPU-GPU architecture. The advancement of high-performance computing systems and the majority of simulations conducted on these platforms have resulted in advances on one level while exposing limitations in existing CFD programs on another. As a result, hardware-aware design and optimizations are essential for fully using contemporary computer resources in our proposed SCM, as shown in .
According to the CFD theorem, having finer, and hence more frequent, elements make a more reliable prediction of outcomes at the expense of additional computation time. Increasing computational complexity by integrating specific turbulence equations and other search-relevant variables may have a significant impact on the processing time and code capacity. Therefore, CFD has only recently been embraced as a research solution to laboratory experiments and pure statistical equations, although the latter is only applicable to smaller problems. Computing power has progressed to the point that it is now possible to simulate very controversial needs on a personal computer in a fair period of time. Techniques such as multiple processing limit the computation much further. CFD has a number of significant benefits over theoretical fluid dynamics surveys (EFDs). The first advantage is in architecture and production. Sampling flow data at several locations requires the creation of a cell data sampler or multiple data samplers equipped to cover the area of interest (aorta model in our case). The greatest power of CFD simulations is based on experimental techniques and theorems. Experimental studies may have large-scale values such as strain on a comparatively large test section, velocity profile or average velocity at a point of interest, and particle paths. However, obtaining correct outcomes for the entire model can be costly, time-consuming, and sometimes impractical.(Chen., Citation2006).
Numerous real-time modeling and real-time computing applications have been created to take advantage of CUDA in the medical industry. AxRecon is a diagnostic medical sight recovery system that enables CUDA to remove constraints using MRI.Footnote1 ELEKS assists medical devices in reducing patient diagnosis time by speeding MRI post-treatment software with CUD. AGSnrc works with CUDA for acceleration and archived gear ratios of up to 20x—40x. The Aetina M3N970M-MN is a 4D ultrasound system that employs CUDA nuclei to achieve sophisticated 3D visualization of ultrasound data using the most recent phase-shift imaging technology (Lippuner & Elbakri, Citation2011). The modern medical industry generates a vast volume of data, which is then processed using sophisticated algorithms. Medical imaging modalities with MRI usually produce 2D, 3D, and 4D volumes for diagnosis and surgical preparation processes.(Piccialli et al., Citation2013) (Xu et al., Citation2018) (Eklund et al., Citation2013) (Bergen et al., Citation2015). These considerations involve the use of a high-performance computing device with a massive computational capacity and hardware setup (Deserno et al., Citation2013). Any of the most recent GPU calculations on these medical image analyses can be found in (Kalaiselvi et al., Citation2017).
This procedure requires parallel processing with a CPU and GPU at the mesh generation level and resolution of the Navier–Stokes equation. Compute Unified Device Architecture2 (CUDA) is a framework released in 2007 to target Nvidia GPU, as co-processors for general-purpose calculations. CUDA designates a processor architecture, a software model, and an API.CUDA is specifically designed for NVIDIA GPUs, whereas Open-CL is designed to work on a variety of architectures, including graphics processors, processors, and digital signal processors (DSPs). These technologies allow the specified functions of a C program to run on the stream processors of the graphics processor. This allows C programs to take advantage of the GPU’s ability to run on large arrays in parallel, while using the processor when appropriate(Rabbani et al., Citation2019).
CUDA software has two phases that run in a host (CPU) or a computer (GPU). The host code has no data parallelism. The device code implements phases with a high degree of data parallelism. The NVIDIA C (NVCC) compiler is used by CUDA programs to distinguish between the two phases during the compilation process. Our sight to programming model is summarized in .
The GPU-CUDA hardware consists of three major components that work together to fully utilize the GPU’s computational power. The CUDA architecture is built up of grids, blocks, and connecting wires, as shown in . CUDA can handle a large number of concurrent threads. Threads are organized into blocks, and the blocks are organized into threads. The execution is independent of bodies of the same rank in these three architectural hierarchy stages. A grid is a set of thread blocks that can run independently of each other. Each block has a unique block identifier and is arranged as a 3D array of threads (blockIdx). The kernel function executes threads, and each thread has a special thread identifier (thread ID) (Tsao, Citation2015).
The GPU device has multiple memories for executing the threads. depicts various CUDA device memory organizations. Each SM has a number of streaming co-processors (SMs) and a number of streaming processor cores (SPs). Each thread has the ability to access variables in local memory and registers. Each block has its own shared memory size of 16 KB or 48 KB, which is accessible to all block users. When all threads access the same location at the same time, constant memory supports low-latency, high-bandwidth, and read-only access by the device. The cache’s constant size was reduced to 64 KB. To avoid using global memory, texture memory can be used as a type of cache (Amjadian & Gharaei, Citation2021).
depicts the CUDA run stream. GPU threads are much lighter than CPU threads. The CUDA software begins with the host’s execution. The kernel function creates a huge number of threads in order to perform code parallelism. Before the kernel is started, all required data is passed from the host to the assigned device’s memory. The kernel operation is started by the CPU, and then execution is carried out on the system. The output data would be sent to the host for further processing.
Because our Navier-Stokes solver is already functional on multi-core architectures, the strategy of adding GPU computation to the original code is called strategy (\ minimal invasion). The minimal invasion strategy means replacing the codes to be optimized by some GPU codes while maintaining the same inputs and outputs. In this way, we will not be able to reach the optimal performance of the GPU, but it is easy to change between the CPU and (CPU/GPU) codes. (Awasthi & Omrani, Citation2019)
1.2.2. OpenFOAM tool and CFD solvers
The advancement of high-performance computing systems and the majority of simulations conducted on these platforms have resulted in advances on one level while exposing limitations in existing CFD programs on another. Consequently, hardware-aware design and optimization are essential for fully using contemporary computer resources. The advancement of high-performance computing systems and the majority of simulations conducted on these platforms have resulted in advances on one level while exposing limitations in existing CFD programs. Therefore, hardware-aware design and optimizations are essential for fully using contemporary computer resources (Zakaria et al., Citation2019).As a result, a heterogeneous decomposition approach was devised and implemented to divide the computational domain among heterogeneous devices, with the goal of balancing the burden of the devices during application execution. This technique combines the OpenFOAM-supported conventional domain decomposition methods with state-of-the-art heterogeneous data-partitioning algorithms. Furthermore, algorithmic advances of the conjugate gradient linear solver (Márquez Damián et al., Citation2012). OpenFOAM is a CFD framework that allows users to write solvers and tools (for pre- and post-processing) in a high-level programming language. This high-level language refers to writing in a notation that is closer to the mathematical explanation of the problem, freeing the user from the code’s internal workings. As a result, to accomplish abstraction from low-level coding, another path must be taken, and the object-oriented programming (OOP) paradigm must be chosen. When compared to strictly procedural approaches, this methodology provides a code that is easier to create, validate, and maintain. In comparison to OpenFOAM, it is entirely written in C++(Eckel, Citation2000). To accomplish abstraction from low-level coding, another path must be taken, and the object-oriented programming (OOP) paradigm must be chosen. When compared to strictly procedural approaches, this methodology provides a code that is easier to create, validate, and maintain. In comparison to OpenFOAM, it is entirely written in C++, as shown in .
The major advantages of OpenFOAM programming are its modularity and adaptability. The linear algebra kernel module is one of the modules, and it can be found in virtually all PDE solver programs. The major advantages of OpenFOAM programming are its modularity and adaptability. The linear algebra kernel module is one of the modules, and it can be found in virtually all PDE solver programs. OpenFOAM’s modularity is one of its most appealing features because it allows for a more efficient and adaptable design. It has a large number of solvers that are used in CFD, such as Laplace and Poisson equations, incompressible flow, and compressible flow models, as described in .
Table 1. Solvers with OpenFOAM
2. Methods and materiels
A 10-year-old child with congenital valve and valvular aortic stenosis on sensitive MRI and coarctation (operated and dilated) in the sense of shone syndrome underwent a retrospective cardiac MRI scan with 74 sequences and 3057 scans.
Technically, the reconstruction of the descending aorta was performed with
44 TRICKS angiographic slices in dynamic acquisition on the thoracic aorta
Infusion sequences after injection
Cine-fiesta MRI sequences T2 short-axis 4 cavities
Short-axis late infusion sequences
To develop the mesh, we used the OpenFOAMFootnote2 box in conjunction with the CFD module. The key benefit of this framework is that it provides an open, scalable Gui with great ease of use for mesh creation and solver adjustment. The accuracy of the analysis was shown as applied to the references and other commercial software. It should be noted that all methods, including mesh generation, numerical simulation, and post-processing, were used. (Kone et al., Citation2018). Numerical calculations using the OpenFOAM Open Source solver to solve the Reynolds-averaged Navier–Stokes (RANS) equation with the use of a substantial improvement in computational power availability and multi-core operation. Transient simulations with the turbulence model closure model (k -) were used in conjunction with a dynamic mesh interface (Nuernberg & Tao, Citation2018). The key goal of using a computational resolver for compressible Navier-Stokes equations was to demonstrate the predictive capacities of flows in our aortic geometry (Modesti & Pirozzoli, Citation2017).
We used the Python library (Allam & Krauthammer, Citation2017) (Lamy, Citation2017)(Müller et al., Citation2015) with Anaconda to program and configure the fluid solver with the physical properties of blood.
3. Results
In this section, we will proceed to the first phase of our design, which consists of defining the geometry of the descending aorta in 3D. This step states the reconstruction of the aortic model from the TRICKS sections, as shown in below:
Figure 5. (a) original TRIKS cardiac sequences acquired with MRI;(bsteps of reconstruction and segmentation of the 3D aorta structure); (c) 3D reconstruction of the descending aorta
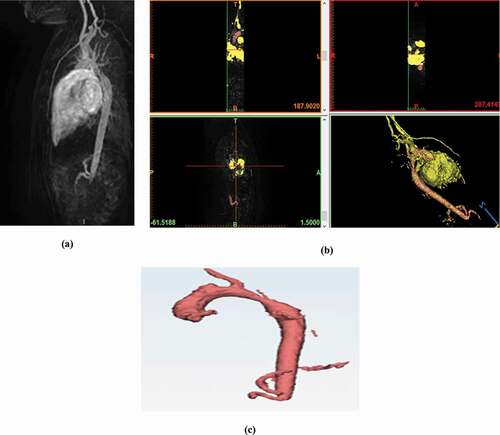
Evidently, the mesh phase necessitates parallel processing across several cores (CPU and GPU). The heterogeneous hardware design CPU-GPU and the execution paradigm result in a number of failures, including underutilization of computational power, connectivity overload between processing units, a lack of portability between architectures, and a lack of energy performance, as found by these issues. The gaps described above in heterogeneous CPU-GPU computing can be filled by collaborating with IT resources. GPGPU computing performance can be increased further if disabled CPU cores can be used by running the kernel on processors and graphics processors cooperatively. In other words, when the GPU runs the kernel, a portion of the GPU workload can be delegated to the CPU cores. As a result, CPU-GPU cooperative computing takes advantage of the parallelism between the GPU and CPU processor (Raju. & Chiplunkar, Citation2018). Furthermore, shows a time estimate for tracing, developing, and finalizing the mesh of the stenosing aorta.
Table 2. Parallel processing of the mesh of the aorta
From these simulation results, the time spent in tracing, decomposing, and finalizing the mesh crucially depends on the number of nuclei used in the parallel processing and the mesh quality chosen. For a fine and very fine mesh, a number of processors are acquired at the beginning of the treatment greater than or equal to 4 because these two characteristics require more refinement for the edges of the facets (Titarenko & Hildyard, Citation2017).
In this section, we explain our motivation to use GPU accelerators in the Navier-Stokes solver (Dollinger, Citation2015) (Michéa & Komatitsch, Citation2010) (Xu & Wright, Citation2016). We designed new algorithms and implementations for GPU fluid solvers, and obtained satisfactory acceleration. We then modified our Navier-Stokes solver to integrate the new GPU versions with certain routines. The resulting Navier-Stokes hybrid (CPU/GPU) solver is operational and can be used on different types of architectures. Experiments using the CPU/GPU solver on compute nodes showed good scalability of the execution time compared to the processor implementation. This algorithm consists of importing mesh geometry in standard tessellation language (STL) format in 3D (D.-X. Wang et al., Citation2006),(Y. Liu et al., Citation2017) as well as the libraries necessary to handle it (Pymesh, Pandas …). The first step is to make a self-scale to resize the mesh size according to the normal vector because the projection of the normal on two dimensions is performed on two axes. The implementation of the Navier–Stokes equation starts with the definition of the flux cavity (number of units of time, ρ, μ, ν, dx, dy) to treat the Newtonian linear case (constant viscosity is equal to μ and the velocity in the cavity of the aorta is equal to 1). The simulation of blood characteristics in our 2D mesh geometry depends on two velocity components with normal human blood characteristics: temperature = 37 °C, density between 1.056 and 1.066, viscosity = 0.004 Pa · S (Pascal) *second).Based on the PyMesh library,Footnote3 we imported the geometry of the mesh (mesh) in in Python. To simplify the calculation, the resolution of a fluid solver (NS) in 2D is implemented through the extraction of the normal vector 3D by projecting it on a 2D axis.
The second step is the creation of the simulation modules for the fluid solver as well as the creation of a module for the calculation (simulation on the mesh) on the CPU during a cardiac cycle, which takes into consideration the maximum ejection speed (0.4 ml/ms) and the maximum filling rate (0.3 ml/ms) according to the clinical assessment. The calculation time for the CPU is shown in .
Subsequently, we initialized the number of threads per block at 32 to estimate the GPU cost using the kernel. The number of threads per block in each grid depends on the size of the aortic geometry. The calculation time estimate for the GPU consumed by the fluid solver is implemented with the decorator class with Python in the CUDA environment, as shown in .
We first observe that, as expected, the GPU acceleration can significantly reduce the computation time for all mesh sizes considered. The improvement is quantified using the percentage of acceleration, defined as (Shah et al., Citation2020) (Michéa & Komatitsch, Citation2010):
Time (GPU) represents the execution time using CPU/GPU parallelism, as shown in . The acceleration percentage indicates that GPU parallelism becomes more efficient as the mesh size increases.
4. Discussion
One of the most difficult aspects of contemporary HPC systems is their heterogeneity. Multi-core processors are used in modern CPUs because concurrency is the only way to take advantage of Moore’s law. Furthermore, a strongly coupled multiprocessor system known as symmetric multi-processing is formed when many multi-core processors are joined to a shared main memory (SMP). An SMP may also have access to special-purpose processors, often known as co-processors, such as GPU devices. As previously stated, GPUs provide potential computing capabilities that have advanced against contemporary CPUs. This shift in HPC systems brings with it a slew of new difficulties and methods. Experiments that were mentioned in the previous section analyzed the efficiency of parallel architecture for 3D modeling of the aorta with computation time performed on the Gpu-Nvidia GEFORCE GT 635 M device and compute capability = 1.2. The speed of medical imaging may have benefited from the compressed detection of MRI, but the reconstruction computation time is relatively long owing to its iterative process. A parallel architecture based on multicore processors is proposed to accelerate the resolution of the fluid solver in a 3D structure of the aorta. The results of the simulation demonstrate that the acceleration factor cannot approximate the number of processor cores and that the overall reconstruction of the MRI-based could be performed in several seconds. The activity attributed to core CPU stains is considered to be among the reasons that require additional processing time. In addition, tasks may not be completed at the same time. Therefore, it is reasonable for the acceleration factor to be between 3 and 4 with four processor cores (Li et al., Citation2014).
In several studies, dynamic-parallel, global memory, and shared memory are the three GPU-solver implementations. Because of parallelization benefits, all GPU solvers are faster than CPU (serial) solvers. Compared to previous GPU solvers, the dynamic-parallel solver offers enhanced data security. Modeling with optimization and prediction(Bozorgmehr et al., Citation2021) Several research results with CPU-CUDA emphasized on improving the efficiency of the mixed Lagrangian–Eulerian (IMLE) method for modeling incompressible Navier–Stokes flows with CUDA programming on multi-GPUs (R. K.-S. Liu et al., Citation2019). Simulating the complete three-dimensional dissipative dynamics of the quark–gluon plasma with changing starting conditions is computationally costly and usually necessitates some parallelization. a GPU implementation of the Kurganov–Tadmor method for solving the 3 + 1d relativistic viscous hydrodynamic equations with both bulk and shear viscosities. The CUDA-based GPU code is approximately two orders of magnitude faster than the Kurganov–Tadmor algorithm’s comparable serial implementation(Bazow et al., Citation2018). CPU-GPU cross-platform coupled CFD-DEM approach for complex particle-fluid flows(He et al., Citation2020).A compared OpenFOAM conjugate gradient, which uses MPI for parallelization, with Cufflink conjugate gradient, uses CUDA to simulate the benchmark as a 3D lid-driven cavity flow scenario with 1,000,000 cells on a cubic mesh. The case is solved using icoFoam on Tesla, which runs for eight time steps and displays the results of parallel icoFoam solver execution for a variety of issue sizes. The number of processors utilized is limited by the number of GPUs accessible to the Tesla system, which is two. The precision used in the 3D cavity test case computation was double precision. The pressure field was determined using a conjugate gradient technique. The performance difference between the CPU and GPU solvers was minimal. This indicates that in a hybrid method, CPUs are suitable for incorporation in the computation alongside GPUs (AlOnazi, Citation2014).
5. Conclusion
The healthcare supply chain begins with the production of medical products and delivery to a distribution hub. Depending on the product, hospitals can acquire inventory directly from the manufacturer or distributor, or through a group purchasing organization, which creates a purchasing contract with the manufacturer on behalf of the hospital. Depending on the product, hospitals can acquire inventory directly from the manufacturer or distributor, or through a group purchasing organization, which creates a purchasing contract with the manufacturer on behalf of the hospital. This paper presents the design and implementation of hybrid solvers and heterogeneous decomposition to improve MRI tool performance. The motivation behind the hybrid solver is to include the CPUs in the computation with GPUs for the 3D MRI aorta model. A hybrid architecture (GPU/GPU) was proposed to improve the performance of image processing algorithms and medical applications acquired in real-time analysis and monitoring. Our interest in accelerating these methods is mainly due to the increase in the intense computation of MRI applications. With parallel processing based on the CPU-GPU architecture used at the mesh level to obtain the 3D model, we concluded that the response time depends crucially on the number of kernels used.
Ethical approval
All procedures performed in studies involving human participants were in accordance with the ethical standards of the institutional and/or national research committee and with the 1964 Helsinki declaration and its later amendments or comparable ethical standards.
Author contributions
Our research team within the Carthage International Medical Center in Tunisia“ https://www.carthagemedical.com.tn/en/accueil/ “ focus in the field of Data science (Artificial Intelligence,Big Data,blockchain, Internet of Medical things …) applied in Healthcare under the supervision of Dr.Mourad Said (Header of Radiology and Imaging unit and RSNA Member). We aim to focus on the quality-time ratio in supply Healthcare management. Healthcare businesses are being forced to reassess their business strategy and management approaches due to competitive challenges and dramatic cuts in public healthcare expenditure. Managers in this vital business must not only cut expenses but also maintain a high level of service quality. Supply chain management is now widely acknowledged as having a significant influence on healthcare cost reduction and performance improvement.
Informed consent
Informed consent was obtained from all participants included in the study.
Acknowledgements
We would like to thank the Radiology and Medical Imaging Unit, International Center Carthage Medical, which supported this work and to the medical staff for providing us with access to the patients’ archives and the administrative framework for the warm welcome in their team.
Disclosure statement
No potential conflict of interest was reported by the author(s).
Additional information
Funding
Notes
References
- Allam, A., & Krauthammer, M. (2017, November). PySeqLab: An open source Python package for sequence labeling and segmentation. Bioinformatics, 33(21), 3497–16. https://doi.org/https://doi.org/10.1093/bioinformatics/btx451
- AlOnazi, A. (2014). Design and optimization of OpenFOAM-based CFD Applications for Modern Hybrid and Heterogeneous HPC Platforms [Thesis], https://doi.org/https://doi.org/10.25781/KAUST-9M51I.
- Amjadian, A., & Gharaei, A. (2021, April). An integrated reliable five-level closed-loop supply chain with multi-stage products under quality control and green policies: Generalised outer approximation with exact penalty. International Journal of Systems Science: Operations and Logistics, 1–21. https://doi.org/https://doi.org/10.1080/23302674.2021.1919336
- Awasthi, A., & Omrani, H. (2019, January). A goal-oriented approach based on fuzzy axiomatic design for sustainable mobility project selection. International Journal of Systems Science: Operations and Logistics, 6(1), 86–98. https://doi.org/https://doi.org/10.1080/23302674.2018.1435834
- Bazow, D., Heinz, U., & Strickland, M. (2018, April). Massively parallel simulations of relativistic fluid dynamics on graphics processing units with CUDA. Computer Physics Communications, 225, 92–113. https://doi.org/https://doi.org/10.1016/j.cpc.2017.01.015
- Benchikh Le Hocine, A. E., Poncet, S., & Fellouah, H. (2021, July). CFD modeling and optimization by metamodels of a squirrel cage fan using OpenFoam and Dakota: Ventilation applications. Building and Environment, 205 2021 , 108145 . https://doi.org/https://doi.org/10.1016/j.buildenv.2021.108145
- Bergen, R. V., Lin, H.-Y., Alexander, M. E., & Bidinosti, C. P. (2015, January). 4D MR phase and magnitude segmentations with GPU parallel computing. Magnetic Resonance Imaging, 33(1), 134–145. https://doi.org/https://doi.org/10.1016/j.mri.2014.08.019
- Blanco-Aguilera, R., Lara, J. L., Barajas, G., Tejero, I., & Diez-Montero, R. (2020, April). CFD simulation of a novel anaerobic-anoxic reactor for biological nutrient removal: Model construction, validation and hydrodynamic analysis based on OpenFOAM®. Chemical Engineering Science, 215 2020 , 115390 . https://doi.org/https://doi.org/10.1016/j.ces.2019.115390
- Bozorgmehr, B., Willemsen, P., Gibbs, J. A., Stoll, R., Kim, -J.-J., & Pardyjak, E. R. (2021, March). Utilizing dynamic parallelism in CUDA to accelerate a 3D red-black successive over relaxation wind-field solver. Environmental Modelling & Software, 137, 104958. https://doi.org/https://doi.org/10.1016/j.envsoft.2021.104958
- Chen., W. Y. (2006). Simulation of arterial stenosis incorporating fluid-structural interaction and non-Newtonian blood flow. RMIT University.
- Deng, Y., Sun, D., Liang, Y., Yu, B., & Wang, P. (2017, November). Implementation of the IDEAL algorithm for complex steady-state incompressible fluid flow problems in OpenFOAM. International Communications in Heat and Mass Transfer, 88 2017, 63–73 https://doi.org/https://doi.org/10.1016/j.icheatmasstransfer.2017.08.004
- Deserno, T. M. Handels, H., Maier-Hein, K. H., Mersmann, S., Palm, C., Tolxdorff, T., Wagenknecht, G., Wittenberg, T. (2013, May). Viewpoints on medical image processing: From science to application. Current Medical Imaging Reviews, 9(2), 79–88 https://doi.org/https://doi.org/10.2174/1573405611309020002
- Dollinger, J.-F. (2015). A framework for efficient execution on GPU and CPU+GPU systems [phdthesis], Université de Strasbourg, Retrieved August. 05, 2018, from. https://hal.inria.fr/tel-01251719/document
- Duan, C., Deng, C., Gharaei, A., Wu, J., & Wang, B. (2018, December). Selective maintenance scheduling under stochastic maintenance quality with multiple maintenance actions. International Journal of Production Research, 56(23), 7160–7178. https://doi.org/https://doi.org/10.1080/00207543.2018.1436789
- Dubey, R., Gunasekaran, A., Sushil, & Singh, T. (2015, October). Building theory of sustainable manufacturing using total interpretive structural modelling. International Journal of Systems Science: Operations and Logistics, 2(4), 231–247. https://doi.org/https://doi.org/10.1080/23302674.2015.1025890
- Eckel, B. (2000). Thinking in C++, Vol. 1: Introduction to Standard C++, 2nd Edition (2nd ed.). Prentice Hall.
- Eklund, A., Dufort, P., Forsberg, D., & LaConte, S. M. (2013, December). Medical image processing on the GPU – Past, present and future. Medical Image Analysis, 17(8), 1073–1094. https://doi.org/https://doi.org/10.1016/j.media.2013.05.008
- Gharaei, A., Karimi, M., & Hoseini Shekarabi, S. A. (2019, May). An integrated multi-product, multi-buyer supply chain under penalty, green, and quality control polices and a vendor managed inventory with consignment stock agreement: The outer approximation with equality relaxation and augmented penalty algorithm. Applied Mathematical Modelling, 69, 223–254. https://doi.org/https://doi.org/10.1016/j.apm.2018.11.035
- Gharaei, A., Karimi, M., & Shekarabi, S. A. H. (2020, October). Joint Economic Lot-sizing in Multi-product Multi-level Integrated Supply Chains: Generalized Benders Decomposition. International Journal of Systems Science: Operations and Logistics, 7(4), 309–325. https://doi.org/https://doi.org/10.1080/23302674.2019.1585595
- Gharaei, A., Naderi, B., & Mohammadi, M. (2015). Optimization of rewards in single machine scheduling in the rewards-driven systems. Management Science Letters, 5(6), 629–638. https://doi.org/https://doi.org/10.5267/j.msl.2015.4.002
- Gharaei, A., Shekarabi, S. A. H., Karimi, M., Pourjavad, E., & Amjadian, A. (2021, April). An integrated stochastic EPQ model under quality and green policies: Generalised cross decomposition under the separability approach. International Journal of Systems Science: Operations and Logistics, 8(2), 119–131. https://doi.org/https://doi.org/10.1080/23302674.2019.1656296
- Gharaei, A., Shekarabi, S. A. H., & Karimi, M. (2020, July). Modelling And optimal lot-sizing of the replenishments in constrained, multi-product and bi-objective EPQ models with defective products: Generalised Cross Decomposition. International Journal of Systems Science: Operations and Logistics, 7(3), 262–274. https://doi.org/https://doi.org/10.1080/23302674.2019.1574364
- Giri, B. C., & Bardhan, S. (2014, October). Coordinating a supply chain with backup supplier through buyback contract under supply disruption and uncertain demand. International Journal of Systems Science: Operations and Logistics, 1(4), 193–204. https://doi.org/https://doi.org/10.1080/23302674.2014.951714
- Giri, B. C., & Masanta, M. (2020, April). Developing a closed-loop supply chain model with price and quality dependent demand and learning in production in a stochastic environment. International Journal of Systems Science: Operations and Logistics, 7(2), 147–163. https://doi.org/https://doi.org/10.1080/23302674.2018.1542042
- Hao, Y., Helo, P., & Shamsuzzoha, A. (2018, April). Virtual factory system design and implementation: Integrated sustainable manufacturing. International Journal of Systems Science: Operations and Logistics, 5(2), 116–132. https://doi.org/https://doi.org/10.1080/23302674.2016.1242819
- He, Y., Muller, F., Hassanpour, A., & Bayly, A. E. (2020, September). A CPU-GPU cross-platform coupled CFD-DEM approach for complex particle-fluid flows. Chemical Engineering Science, 223, 115712. https://doi.org/https://doi.org/10.1016/j.ces.2020.115712
- Kalaiselvi, T., Sriramakrishnan, P., & Somasundaram, K. (2017, January). Survey of using GPU CUDA programming model in medical image analysis. Informatics in Medicine Unlocked, 9, 133–144. https://doi.org/https://doi.org/10.1016/j.imu.2017.08.001
- Kazemi, N., Abdul-Rashid, S. H., Ghazilla, R. A. R., Shekarian, E., & Zanoni, S. (2018, April). Economic order quantity models for items with imperfect quality and emission considerations. International Journal of Systems Science: Operations and Logistics, 5(2), 99–115. https://doi.org/https://doi.org/10.1080/23302674.2016.1240254
- Kone, J.-P., Zhang, X., Yan, Y., Hu, G., & Ahmadi, G. (2018, July). CFD modeling and simulation of PEM fuel cell using OpenFOAM. Energy Procedia, 145, 64–69. https://doi.org/https://doi.org/10.1016/j.egypro.2018.04.011
- Lamy, J.-B. (2017). Owlready: Ontology-oriented programming in Python with automatic classification and high level constructs for biomedical ontologies. Artificial Intelligence in Medicine, 80, 11–28. https://doi.org/https://doi.org/10.1016/j.artmed.2017.07.002
- Lee, S. M., Lee, D., & Schniederjans, M. J. (2011, January). Supply chain innovation and organizational performance in the healthcare industry. International Journal of Operations & Production Management, 31(11), 1193–1214. https://doi.org/https://doi.org/10.1108/01443571111178493
- Li, Q., Qu, X., Liu, Y., Guo, D., Ye, J., Zhan, Z., & Chen, Z. (2014). Parallel computing of patch-based nonlocal operator and its application in compressed sensing MRI. Computational and Mathematical Methods in Medicine, 2014, 257435. https://doi.org/https://doi.org/10.1155/2014/257435
- Lippuner, J., & Elbakri, I. A. (2011). A GPU implementation of EGSnrc’s Monte Carlo photon transport for imaging applications. Physics in Medicine and Biology, 56(22), 7145. https://doi.org/https://doi.org/10.1088/0031-9155/56/22/010
- Liu, R. K.-S., Wu, C.-T., Kao, N. S.-C., & Sheu, T. W.-H. (2019, April). An improved mixed Lagrangian–Eulerian (IMLE) method for modelling incompressible Navier–Stokes flows with CUDA programming on multi-GPUs. Computers & Fluids, 184, 99–106. https://doi.org/https://doi.org/10.1016/j.compfluid.2019.03.024
- Liu, Y., Saputra, A. A., Wang, J., Tin-Loi, F., & Song, C. (2017, January). Automatic polyhedral mesh generation and scaled boundary finite element analysis of STL models. Computer Methods in Applied Mechanics and Engineering, 313, 106–132. https://doi.org/https://doi.org/10.1016/j.cma.2016.09.038
- Márquez Damián, S., Giménez, J. M., & Nigro, N. M. (2012, May). gdbOF: A debugging tool for OpenFOAM®. Advances in Engineering Software, 47(1), 17–23. https://doi.org/https://doi.org/10.1016/j.advengsoft.2011.12.006
- Materano, G., Araujo, C., & Ochoa, A. A. V. (2021, October). A new OpenFOAM proposal for the solution of diffusion problems. Thermal Science and Engineering Progress, 25, 100982. https://doi.org/https://doi.org/10.1016/j.tsep.2021.100982
- Michéa, D., & Komatitsch, D. (2010). Accelerating a three-dimensional finite-difference wave propagation code using GPU graphics cards. Geophysical Journal International, 182(1), 389–402. https://doi.org/https://doi.org/10.1111/j.1365-246X.2010.04616.x
- Modesti, D., & Pirozzoli, S. (2017, July). A low-dissipative solver for turbulent compressible flows on unstructured meshes, with OpenFOAM implementation. Computers & Fluids, 152, 14–23. https://doi.org/https://doi.org/10.1016/j.compfluid.2017.04.012
- Müller, P., Schürmann, M., & Guck, J. (2015, November). ODTbrain: A Python library for full-view, dense diffraction tomography. BMC Bioinformatics, 16(1), 367. https://doi.org/https://doi.org/10.1186/s12859-015-0764-0
- Nuernberg, M., & Tao, L. (2018, January). Three dimensional tidal turbine array simulations using OpenFOAM with dynamic mesh. Ocean Engineering, 147, 629–646. https://doi.org/https://doi.org/10.1016/j.oceaneng.2017.10.053
- Piccialli, F., Cuomo, S., & De Michele, P. (2013, January). A Regularized MRI Image Reconstruction based on Hessian Penalty Term on CPU/GPU Systems. Procedia Computer Science, 18, 2643–2646. https://doi.org/https://doi.org/10.1016/j.procs.2013.06.001
- Pinna, R., Carrus, P., & Marras, F. (2015). Emerging trends in healthcare supply chain management — An Italian experience. https://doi.org/https://doi.org/10.5772/59748
- Rabbani, M., Foroozesh, N., Mousavi, S. M., & Farrokhi-Asl, H. (2019, April). Sustainable supplier selection by a new decision model based on interval-valued fuzzy sets and possibilistic statistical reference point systems under uncertainty. International Journal of Systems Science: Operations and Logistics, 6(2), 162–178. https://doi.org/https://doi.org/10.1080/23302674.2017.1376232
- Rabbani, M., Hosseini-Mokhallesun, S. A. A., Ordibazar, A. H., & Farrokhi-Asl, H. (2020, January). A hybrid robust possibilistic approach for a sustainable supply chain location-allocation network design. International Journal of Systems Science: Operations and Logistics, 7(1), 60–75. https://doi.org/https://doi.org/10.1080/23302674.2018.1506061
- Raju., K., & Chiplunkar, N. N. (2018, August). A Survey on Techniques for Cooperative CPU-GPU Computing. Sustainable Computing: Informatics and Systems 19 . https://doi.org/https://doi.org/10.1016/j.suscom.2018.07.010
- Robertson, E., Choudhury, V., Bhushan, S., & Walters, D. K. (2015, December). Validation of OpenFOAM numerical methods and turbulence models for incompressible bluff body flows. Computers & Fluids, 123, 122–145. https://doi.org/https://doi.org/10.1016/j.compfluid.2015.09.010
- Samuel, C., Gonapa, K., Chaudhary, P. K., & Mishra, A. (2010, January). Supply chain dynamics in healthcare services. International Journal of Health Care Quality Assurance, 23(7), 631–642. https://doi.org/https://doi.org/10.1108/09526861011071562
- Sarkar, S., & Giri, B. C. (2020, April). Stochastic supply chain model with imperfect production and controllable defective rate. International Journal of Systems Science: Operations and Logistics, 7(2), 133–146. https://doi.org/https://doi.org/10.1080/23302674.2018.1536231
- Sayyadi, R., & Awasthi, A. (2018, April). A simulation-based optimisation approach for identifying key determinants for sustainable transportation planning. International Journal of Systems Science: Operations and Logistics, 5(2), 161–174. https://doi.org/https://doi.org/10.1080/23302674.2016.1244301
- Sayyadi, R., & Awasthi, A. (2020, April). An integrated approach based on system dynamics and ANP for evaluating sustainable transportation policies. International Journal of Systems Science: Operations and Logistics, 7(2), 182–191. https://doi.org/https://doi.org/10.1080/23302674.2018.1554168
- Shah, N. H., Chaudhari, U., & Cárdenas-Barrón, L. E. (2020, January). Integrating credit and replenishment policies for deteriorating items under quadratic demand in a three echelon supply chain. International Journal of Systems Science: Operations and Logistics, 7(1), 34–45. https://doi.org/https://doi.org/10.1080/23302674.2018.1487606
- Titarenko, S., & Hildyard, M. (2017, July). Hybrid multicore/vectorisation technique applied to the elastic wave equation on a staggered grid. Computer Physics Communications, 216, 53–62. https://doi.org/https://doi.org/10.1016/j.cpc.2017.02.022
- Tsao, Y.-C. (2015, July). Design of a carbon-efficient supply-chain network under trade credits. International Journal of Systems Science: Operations and Logistics, 2(3), 177–186. https://doi.org/https://doi.org/10.1080/23302674.2015.1024187
- Tsiolakis, V., Giacomini, M., Sevilla, R., Othmer, C., & Huerta, A. (2020, April). Nonintrusive proper generalised decomposition for parametrised incompressible flow problems in OpenFOAM. Computer Physics Communications, 249, 107013. https://doi.org/https://doi.org/10.1016/j.cpc.2019.107013
- Wang, C., Wang, G., & Huang, B. (2020, September). Characteristics and dynamics of compressible cavitating flows with special emphasis on compressibility effects. International Journal of Multiphase Flow, 130, 103357. https://doi.org/https://doi.org/10.1016/j.ijmultiphaseflow.2020.103357
- Wang, D.-X., Guo, D.-M., Jia, Z.-Y., & Leng, H.-W. (2006, January). Slicing of CAD models in color STL format. Computers in Industry, 57(1), 3–10. https://doi.org/https://doi.org/10.1016/j.compind.2005.03.007
- Xu, J., Liu, W., Wang, J., Liu, L., & Zhang, J. (2018, February). An efficient implementation of 3D high-resolution imaging for large-scale seismic data with GPU/CPU heterogeneous parallel computing. Computers & Geosciences, 111, 272–282. https://doi.org/https://doi.org/10.1016/j.cageo.2017.11.020
- Xu, R., & Wright, G. A. (2016, November). GPU accelerated dynamic respiratory motion model correction for MRI-guided cardiac interventions. Computer Methods and Programs in Biomedicine, 136, 31–43. https://doi.org/https://doi.org/10.1016/j.cmpb.2016.08.003
- Ye, B., Wang, Y., Huang, C., & Huang, J. (2019, September). Numerical study of the pressure wave-induced shedding mechanism in the cavitating flow around an axisymmetric projectile via a compressible multiphase solver. Ocean Engineering, 187, 106179. https://doi.org/https://doi.org/10.1016/j.oceaneng.2019.106179
- Yin, S., Nishi, T., & Zhang, G. (2016, April). A game theoretic model for coordination of single manufacturer and multiple suppliers with quality variations under uncertain demands. International Journal of Systems Science: Operations and Logistics, 3(2), 79–91. https://doi.org/https://doi.org/10.1080/23302674.2015.1050079
- Zakaria, M. S., Ismail, F., Tamagawa, M., Aziz, A. F. A., Wiriadidjaja, S., Basri, A. A., & Ahmad, K. A. (2019, May). A Cartesian non-boundary fitted grid method on complex geometries and its application to the blood flow in the aorta using OpenFOAM. Mathematics and Computers in Simulation, 159, 220–250. https://doi.org/https://doi.org/10.1016/j.matcom.2018.11.014