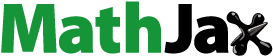
Abstract
The e-commerce adoption level within our society has been growing in the past decade, leading to dynamically evolving demand patterns across retailing channels. In this work, we study a dual-channel retailer’s optimal demand shaping strategy, through e-commerce marketing efforts and store service levels, in the presence of this dynamic evolution. Our stylized model integrates the growing adoption of e-commerce within society with individual consumers’ channel choice, and explicitly models the reference effects of the retailer’s prior decisions on consumer decision-making in a multi-period setting. This model allows us to characterize the settings in which e-commerce marketing is beneficial for the retailer, and to show that the retailer’s optimal demand shaping strategy depends on the product’s e-commerce adoption phase. Interestingly, we find that if the retailer provides the consumers with information on store availability levels, then the retailer’s optimal service levels stay constant over time, even if e-commerce adoption in the society grows.
1. Introduction
“Nearly all growth in retail comes from e-commerce,” states E-commerce Europe (Citation2016), as online sales in Europe grew by 13.3%, whereas the growth in total retail sales merely reached 1% in 2015. A similar trend prevails in the United States, where online sales have increased by 14.5% on average in the past 3 years—four times as fast as traditional retail (The U.S. Department of Commerce, Citation2017). For consumers, e-commerce can be considered a new service, and not all consumers are “adopters” of e-commerce (i.e., consider the option of buying a product online), as this requires confidence in, and access to, this new service, as well as willingness to wait for the product that is purchased online. However, as the sales volumes indicate, this is changing quickly, thanks to various factors, including not only retailers’ marketing efforts for e-commerce, but also the “word of mouth” effect, enhancements in cyber security, introduction of smartphones, etc.
Growing e-commerce adoption introduces new demand dynamics that have implications for dual-channel retailers’ marketing and operational decisions. Consumer purchasing decision now also critically depends on whether or not a certain consumer is an adopter of e-commerce. As a result, different service aspects gain importance over time. For instance, although the e-commerce delivery time may not be of major importance when e-commerce adoption is low, it may have a considerable impact on the demand captured if more consumers are willing to purchase the item online. Thus, depending on the e-commerce adoption level in society, the retailer can experience an expansion/shrinkage in her demand across channels at different periods in time. This brings a new dimension to the dual-channel retailer’s decision-making process: the retailer needs to decide how to split the potential demand between her online and store channels in each period, having varying degrees of e-commerce adoption. This is the problem we study in this article. The retailer can achieve this “demand shaping” through integration of marketing and operational decisions. This common industry practice is implemented by retailers such as Shopify, E-bay, and Intel through novel tools and technologies (Forbes, Citation2016; IBM, Citation2017). Consider Intel’s (Citation2013) new virtual reality tool: “Aside from reducing inventory-related costs, the solution provides a revolutionary in-store experience that can strengthen brand awareness.”
Motivated by these practices, we consider a multi-period setting in which a dual-channel retailer needs to determine the target inventory service levels in the store channel as well as the marketing efforts for the online channel, which has 100% availability. In other words, a rising number of e-commerce adopters are faced with a choice between guaranteed service from the online channel but in exchange for a delivery delay, and immediate service from the store channel but only if the product is in stock in each period. The product availability aspect is important, as consumers can learn about the stock levels through their own experiences or word-of-mouth over time, and make their future channel choices accordingly. In this dynamic environment, the retailer’s objective is to optimize over these two “levers,” in a way to “shape” the demand over the two channels, with the goal of maximizing the expected profit (i.e., revenue minus inventory and marketing-related costs) over the planning horizon.
We explicitly consider that e-commerce adoption levels in society evolve over time, as a result of endogenous factors, which we model in terms of the retailer’s e-commerce marketing efforts (e.g., using novel virtual reality technologies and mobile apps), and exogenous factors, which we model in terms of the word-of-mouth effect. This is in line with Roger’s (Citation1962) diffusion of innovations theory, in that consumers rely highly on word of mouth when it comes to adopting new services and shopping mechanisms, such as e-commerce. Industry research also supports this phenomenon: “With all the advertising and marketing tools […], nothing has quite the impact of referrals from friends, colleagues, and sometimes even complete strangers” Forbes (Citation2017a). This is because, “customer-to-customer interactions are important determinants that shape a firm’s demand or market share, especially […] for new, innovative products of which some features are unknown” (Debo and Veeraraghavan, Citation2009).
Our modeling of the consumer choice process as a function of product availability at the store is also important (Netessine and Tang, Citation2009): Due to the time and energy invested in their shopping trips, “consumers would not patronize a firm without some form of assurance that they can find what they are looking for” (Su and Zhang, Citation2009). Thus, our model brings a new dimension to the extensive literature on joint marketing and operational decisions for dual-channel retailers, through integrating e-commerce adoption, inventory-driven demand, and consumer learning into the modeling framework—all these aspects are essential for modeling the impact of evolving e-commerce adoption on a dual-channel retailer’s demand shaping decisions in a multi-period setting. In this study, we aim to address the following questions: How should a dual-channel retailer adjust its marketing efforts and inventory service levels over time to optimally shape demand under growing e-commerce adoption? Do products at different phases of e-commerce adoption and with different profit and sales margins necessitate different strategies? What is the impact of providing consumers with accurate information on the retailer’s service levels on the retailer’s demand shaping strategy?
In order to address these questions, we develop an analytical model that integrates a consumer choice model with a diffusion model in a multi-period setting. In particular, we utilize the consumer choice model to model consumers’ purchasing and channel choice decisions, which are influenced by: (i) store inventory availability, on which consumers build predictions through their own prior experiences with the retailer as well as through word of mouth; and (ii) consumer heterogeneity with respect to unwillingness to wait for online delivery, distance to the store, and adoption status of the e-commerce option, where the latter is derived from the diffusion model based on word-of-mouth and the retailer’s e-commerce marketing efforts. Consequently, as discussed above, the retailer’s demand across channels is influenced by the exogenous word of mouth effect, and shaped endogenously through its e-commerce marketing efforts and store service levels. Of course the retailer can utilize various levers that may cover a range of marketing and operations decisions to shape demand in this multi-period setting with growing adoption of e-commerce. As representative of those decisions, we consider the retailer’s store inventory and e-commerce marketing efforts in our stylized model, although our modeling framework applies to various other decisions.
To our knowledge, this article is the first to study the impact of growing e-commerce adoption on a dual-channel retailer’s tactical decisions in a multi-period setting. We contribute to the literature both from a modeling and from an insights perspective. First, by building onto the existing works in the literature, we provide a modeling framework that incorporates consumers’ e-commerce adoption status into consumer choice between different retail channels. The majority of the studies in the multi-channel retailing literature assume that all consumers are e-commerce adopters. This assumption leads to extremely high demand volumes for the online channel—in certain settings it is suggested that 100% of the total market demand can be captured through the online channel (Chen et al., Citation2008). Given that the e-commerce sales constitute less than 15% of the total retail sales, this may not be realistic, even for product categories whose e-commerce adoption levels are the highest (refer to Section 6.1 for the e-commerce sales percentages for different product categories). Next, we consider a multi-period setting in which the longer-term effects of the retailer’s decisions can be captured. The majority of the works in the multi-channel retailing literature considers a single-period setting where such effects cannot be modeled. Our modeling framework is able to capture those endogenous effects (i.e., e-commerce marketing efforts and store service levels) along with certain exogenous factors (i.e., word-of-mouth effect) that drive e-commerce adoption. In our analysis, we demonstrate the impact of incorporating these aspects into the model. For example, we find that ignoring the growth in e-commerce adoption would result in much lower inventory order quantities and sales volumes compared with the setting where evolving adoption dynamics are taken into account.
From an insights perspective, our work improves our understanding of how dual-channel retailers should operate, at a tactical level, in today’s dynamic demand environment. Our main findings are as follows. First, retailers whose strategy is to be in close proximity to their consumers can exploit the consumer’s tradeoff between convenience and product availability to expand their market coverage. This leads to lower service levels at a tactical level. Next, even though e-commerce marketing activities improve the retailer’s market coverage by allowing it to capture greater demand in settings where the store is not easily accesible to consumers, i.e., in large service radius, retailers should be cautious: when the majority of the consumers are located within close proximity to the store, e-commerce marketing leads to consumer migration, which is not necessarily profitable (see Forbes, Citation2017b). Otherwise, the retailer’s optimal demand-shaping policy depends on the product’s e-commerce adoption phase. For example, not surprisingly, our numerical results reveal that if a product is at a mature adoption phase, then the retailer does not need to promote the online channel as aggressively as in the earlier adoption phases, but needs to increase the store service levels. Interestingly, we show that the retailer’s optimal service levels vary over time, mainly due to the fact that consumers need to predict the retailer’s current availability level using prior information. That is, if the retailer provides the consumers with accurate information on their current store availability level, then the retailer’s optimal service levels do not need to vary over time, even as e-commerce adoption grows. This finding implies that by providing consumers with perfect information on current store availability level, the retailer enjoys an optimal service level policy that is much easier to implement than the optimal policy in the limited information setting.
In the following, we first present an overview of the related literature to clarify our contributions.
2. An overview of the related literature
To our knowledge, this article is the first to incorporate the growing e-commerce adoption in a dual-channel retailer’s demand-shaping strategy. The related literature includes research on dual-channel retailing, inventory-driven demand, adaptive learning, and technology diffusion.
There is a growing body of operations management (OM) literature on dual-channel retailing management (see Cattani et al. (Citation2004), Tsay and Agrawal (Citation2004a) and Agatz et al. (Citation2008) for excellent reviews). A strand of research in this area focuses on coordination issues in decentralized systems where a manufacturer and its intermediary are engaged in both vertical and horizontal competition through price and/or service interactions (Chiang et al., Citation2003; Tsay and Agrawal, Citation2004b; Boyaci, Citation2005; Chiang and Chhajed, Citation2005; Cattani et al., Citation2006; Chen et al., Citation2008). Recently, some OM scholars have examined the impact of new shopping trends on dual-channel retailers’ decisions in centralized settings (e.g., Balakrishnan et al. (Citation2014), and Gao and Su (Citation2016)). Most of the aforementioned works study a dual-channel retailer’s decisions at a strategic level, by considering single-period models, with the assumption that all consumers are adopters of e-commerce. Hence, neither the partial adoption of e-commerce and its dynamic growth within society, nor the longer-term effects of such decisions is adequately studied. We contribute to this literature by considering a tactical level, multi-period problem, which is able to capture those endogenous effects (i.e., e-commerce marketing efforts and store service levels) along with certain exogenous factors (i.e., word-of-mouth effect) that drive e-commerce adoption. Our findings show that ignoring the growth in e-commerce adoption would lead to much lower total order quantities and sales volumes compared with that of the setting in which these dynamics are modeled.
In order to model the endogeneity of retailer’s store service level decisions, we draw upon the literature on inventory-driven demand; that is, research in which product availability is used as a lever by the firm to stimulate demand. Dana and Petruzzi (Citation2001) extend the classic single-period single-channel newsvendor problem by assuming that utility-maximizing consumers choose between the product and an outside option based on the firm’s current availability, under both exogenous and endogenous price settings (see Dana and Petruzzi (Citation2001) for an extensive review of research on inventory-driven demand.) Chen et al. (Citation2008) utilize a similar demand model for a dual-channel retailer with a 100% availability in the online channel and an endogenous newsvendor type of setup in the store channel, similar to the setting in this article. The modeling of demand in our work is similar to the aforementioned works in that consumers are utility-maximizers, and the resulting demand is dependent on the retailer’s inventory availability. As opposed to Dana and Petruzzi (Citation2001), we replace the pricing decision with e-commerce marketing efforts. Most importantly, we extend these works to a multi-channel multi-period setting, which allows us to show that similar service level findings apply when: (i) a high service level in one channel (store) stimulates the channel’s own demand, while reducing the demand for the other channel (online), whose adoption level increases over time and is also impacted by the retailer’s decisions; (ii) consumers switch between channels in case of a stock-out; and (iii) consumers learn over time.
The adaptive learning paradigm is commonly used to model reference effects of retailer’s prior decisions on consumer decision-making in a multi-period setting. The adaptive learning phenomenon assumes that consumers do not always treat the most recently revealed information as a perfectly reliable reference; rather, they adjust to this new information gradually by merging it with past experience to update their beliefs (Liu and van Ryzin, Citation2011). It is common to model this type of learning through exponential smoothing in multi-period settings (Akerman, Citation1957; Monroe, Citation1973; Sterman, Citation1989; Popescu and Wu, Citation2007; Wu et al., Citation2015). In particular, similar to Liu and van Ryzin (Citation2011), we model consumers’ prediction of the retailer’s current product availability level as an exponential smoothed average of the product availability level in the previous period and consumers’ prior estimate. A fundamental difference between this work and our research is that although high availability stimulates demand in our model by providing consumers with a higher assurance of availability, in the setting studied by Liu and van Ryzin (Citation2011) the effect is the exact opposite: expectation of high availability during the sale season discourages consumers from buying during the regular season at full price. From that perspective, our work differs from Liu and van Ryzin (Citation2011), as there is no strategic “wait and decide” component. That is, all consumers make their purchasing decision under the uncertainty that the product may be out of stock at the store. The dual-channel retailing aspect of our work is also new within this stream of literature, and it allows us to study the impact of consumer learning on the demand split across channels.
We draw upon the literature on diffusion of innovations (Rogers, Citation1962) to model the penetration of e-commerce adoption within society. Diffusion models study the penetration of new technology, products, and services (e.g., e-commerce) within society over time (Sterman, Citation2000). In particular, the Bass diffusion model represents this phenomenon mathematically in a stylistic manner as a function of interactions among current adopters and non-adopters in the form of word of mouth, and external factors such as marketing efforts (Bass, Citation1969; Mahajan et al., Citation1995; Dodson, Citation2014). From that perspective, the papers that are most relevant to our research include Lewis and Long (Citation2009), which uses the Bass diffusion model to forecast e-commerce sales of a retailer, and Barabba et al. (Citation2002), which utilizes a diffusion model in conjunction with a logit choice model to study the “awareness generation” among consumers for a new service and the actual number of consumers who buy the new service given that they are aware of it. However, as opposed to our work, these studies solely concentrate on the forecasting aspect or use simulation studies to examine high-level strategic decisions of the firm, such as deciding between an incremental versus an aggressive marketing strategy.
3. The demand model
3.1. Overview and assumptions
We consider a dual-channel retailer that serves a “population” of consumers within a given store service radius k. In each time period, the product is demanded by a random subset of the population, i.e., “market size” or “total demand,” . Consumers are brand- and product-sure, interested in buying at most one unit of the product, and have a homogenous valuation, v, of the product. Heterogeneity in the consumer base is captured in three dimensions: travel distance to the store (variable
), unwillingness to wait for online delivery (variable
), and adoption status of the online channel at time t (Bernoulli variable Xt). Similar to Cattani et al. (Citation2006), Chen et al. (Citation2008), and Yoo and Lee (Citation2011), the distributions of random variables R and D are assumed to be independent and static across time. From a demand modeling perspective, we contribute to the literature by incorporating the e-commerce adoption status into consumer choice. We do this through the introduction of random variable Xt, which evolves over time and leads to two groups of consumers in each time period t: consumers who consider the online option for purchasing the product (i.e., adopters, with Xt = 1) and consumers who do not (i.e., non-adopters, with Xt = 0).
We assume that the retailer can influence the proportion of the adopters within the population () through the level of her e-commerce marketing efforts ω. That is, in addition to the exogenous factors that may play a role in e-commerce adoption, such as the word-of-mouth effect, the retailer can also endogenously influence the adoption level within the population at time t through e-commerce marketing efforts ω (
). This approach is in line with the literature on diffusion of innovations. In particular, we use the Bass diffusion model to represent, for a certain product, the penetration of e-commerce adoption within the population through imitation via word of mouth; and innovation through the retailer’s marketing efforts (Bass, Citation1969; Sterman, Citation2000). In our context, penetration, for a certain product, refers to the adoption of the “idea/option of buying online.” However, individual consumers still make rational choices on whether or not to purchase the item and through which channel (store or online) so as to maximize their utility. Hence, our demand model integrates the diffusion model with a consumer choice model to represent the demand generation process across channels over time. That is, two processes happen simultaneously in our demand model: (i) adoption of the idea of acquiring the item through the online channel penetrates within the population over time through retailer’s marketing efforts, which is determined endogenously, and word of mouth, which is exogenous; and (ii) individual (heterogeneous) consumers choose between channels based on their own characteristics and inventory availability (see ).
We assume that the online channel has 100% inventory availability, whereas the store setting is similar to a newsvendor setting: the retailer decides on the store inventory level before the random demand is realized. (This simplifying assumption preserves the essential aspects of the problem, and is in line with the literature and industry practices; see Appendix C.) In this setting, both consumer types make their purchasing and channel decisions so as to maximize their utility considering the predicted store inventory availability, as stock-outs are costly for consumers. That is, while non-adopters (Xt = 0) choose between the store and the no purchase option, adopters (Xt = 1) also include the online option in their choice set. Furthermore, consumers engage in availability-based channel substitution. That is, among the store-bound consumers who face a stock-out at the store, those who also derive a positive utility from the online channel switch to the online channel (see ).
Next, we present the consumers’ channel choice process. Throughout, we represent random variables in upper-case letters and their realization in lower-case letters. We also use indices o, s, and n to denote the online channel, store channel, and no purchase option, respectively.
3.2. Consumer choice
Let p denote the price of the item in both channels (v > p), and l the online delivery lead time. Then, a consumer with index pair (d, r) derives the following utilities from the online and store channels:where ξt is the consumers’ predicted store availability level, which is a function of the retailer’s store service levels as detailed in Section 3.3. We assume, without loss of generality, that consumers derive zero utility from the no purchase option. Then, given the uncertainty on product availability at the store, the consumer’s expected utility for the store channel in period t can be written as:
Our consumer choice model is similar to other works in the OM literature that focus on dual-channel retailing, e.g., Cattani et al. (Citation2006), Chen et al. (Citation2008), and Gao and Su (Citation2016). We extend these works by introducing variable Xt, which allows us to model the proportion of e-commerce adopters by time t. The proposed model is also in line with the economic theory of consumer choice, and incorporates the impact of channel-specific hassle costs in both purchasing and channel choice decisions (McFadden, Citation1973; Tirole, Citation1988). Observe that consumer choice, hence, demand, is driven endogenously by both the product availability level at the store (αt), and the retailer’s e-commerce marketing efforts (ω). Although the former directly impacts the consumer’s predicted availability level ξt, the latter determines the proportion of e-commerce adopters (i.e., ). Consequently, this model allows us to investigate how the interplay between the retailer’s store service levels and e-commerce marketing efforts impacts the retailer’s demand shaping capability.
3.3. Demand shaping
As explained in Section 3.1, the retailer observes a random market size, i.e., total demand, , in each period; that is, the probability distribution of the market size is exogenous and identical across the different time periods. In other words, although the market size in each period is exogenous, the split of the total demand across channels (and the no purchase option) in each period is determined endogenously. This assumption implies that the existence of an online channel does not grow the overall market but only serves to split the existing market. (These assumptions are common in practice, and are in line with the literature; see Appendix C.)
We first introduce the following events:
Events:
: Event that a random consumer obtains the highest utility from option
in period t, for
; e.g.,
.
: Event that a random consumer obtains the highest utility from the store channel and also derives a positive utility from the online channel in period t, for
;
i.e.,
.
Then, for a random market size of with probability distribution function
we can express the random demand volumes across channels (
), and the number of consumers who choose to not buy the item (
) in period t as follows:
(1)
(1) and qs,t denotes the retailer's order quantity for the store channel in period t.
In line with the Bass model (Citation1969), the proportion of the total demand which will have adopted the e-commerce option by time t () is given by the cumulative distribution function:
(2)
(2) where parameter ω (coefficient of innovation), which we endogenize in our model, signifies the level of the retailer’s e-commerce marketing efforts, and parameter a (coefficient of imitation) can be interpreted as the word-of-mouth effect on e-commerce adoption. An analogous model applies in discrete-time settings, such as the setting considered in this article, where the adoption rate can be discretized as
for
, with
representing the proportion of new adopters in time t, i.e., the probability mass function (Bass, Citation1969). In practice, parameter a depends on the product and the societal structure (Levin et al., Citation2005; Kacen et al., Citation2013), and can be estimated using maximum likelihood or regression analysis if preliminary data are available (Bass, Citation1969), or by evaluating data from similar products otherwise (e.g., data on mail orders or tele-marketing could be used for e-commerce) (Dodson, Citation2014). On the other hand, the retailer may have some control over the marketing parameter, ω, which we endogenize in our model. (Refer to Appendix C for a discussion on other factors that may impact the diffusion of e-commerce adoption.)
In this setting, for a given set of ω and ξt, the random store demand () consists of consumers (both adopters and non-adopters) who obtain the highest utility from the store channel. We do not consider inventory carry over and backlogging costs in order to be able to analyze the retailer’s optimal marketing efforts and service level decisions at a tactical level. Then, if the store demand does not exceed the order quantity (
), the retailer fully satisfies the store demand and ends up with inventory that is discarded with zero salvage value at the end of period t. Otherwise, among those consumers who face a stock-out upon their visit to the store,
, a proportion
switches to the online channel, as they also derive a positive utility from the online channel. Therefore, the random demand in the online channel consists of: (i) consumers who derive the highest utility from the online channel (
); and (ii) store-bound consumers who switch to the online channel due to availability-based channel substitution
see .
Both type 1 and type 2 service levels, detailed below, are relevant for the retailer’s demand-shaping decisions (see Dana (Citation2001), Chen et al. (Citation2008), and Su and Zhang (Citation2009) for a discussion of using type 2 service levels to model the endogenous inventory-driven demand in similar settings):
Type 1 service level, which we refer to as the “store service level,” defined as
Since
, the order quantity for the store channel follows:
(3)
(3) Similarly, considering 100% type 1 online service level and incorporating availability-based channel substitution, the retailer’s order quantity for the online channel,
, follows:
(4)
(4)
Type 2 service level, which we refer to as the “actual store availability level,” follows:
In the absence of perfect information on the current store availability level, we model the consumers’ prediction process using exponential smoothing. That is, at the beginning of each period t, consumers update their predicted store availability level using the realized availability level in period , which is obtained through word of mouth, social media, etc. (Bernstein and Federgruen, Citation2004):
Our modeling of the consumers’ prediction process is in line with experimental and empirical evidence, which suggests that consumers do not treat the most recent information as perfectly reliable; rather, they adjust to the new information gradually by merging it with past experience (Liu and van Ryzin, Citation2011). In that context, λ represents the rate with which consumers adjust to this new information. Our learning model implies that consumers are homogeneous in their learning speed, as well as in their predicted store availability. This is not an unreasonable assumption, as today’s consumers can easily share information with each other through word of mouth, social media, etc. to gather information about the past availability levels in order to form a common prediction of the current availability level (βt) of the company (Bernstein and Federgruen, Citation2004). These assumptions are commonly used in the literature that utilizes adaptive learning, see Section 2. (Please refer to Appendix C for a detailed explanation.)
3.4. Market segmentation
We characterize the retailer’s market segmentation in terms of exogenous parameters k, store service radius, and l, delivery time, which determine the business setting that the retailer operates in (see Appendix C for a discussion on the retailer’s business setting). Let,(5)
(5)
Then, consumers (both e-commerce adopters and non-adopters) whose travel distance to the store is less than (i.e.,
) derive a positive utility from the store. Similarly, e-commerce adopters whose unwillingness to wait for the online delivery of an item is less than
(i.e.,
) derive a positive utility from the online channel. On the other hand,
represents the set of e-commerce adopters who are indifferent between the store and online channels.
and illustrate the market segmentation for e-commerce non-adopters and adopters for the case where and
. In this case, a portion of the consumers choose the store channel, given by events
(for non-adopters) and
(for adopters). Furthermore, for adopters, the store-bound consumers who also receive a positive utility from the online channel (
) switch to the online channel if they face a stock-out at the store. Consumers who choose the online channel (
) consist of store-bound consumers switching to the online channel when they adopt e-commerce (the gridded region to the left of threshold
) and consumers captured by the online option who would, otherwise, opt not to purchase the item (the gridded region to the right of threshold
). Hence, the online channel expands the retailer’s market coverage by reducing the volume of “choice-based lost demand,” i.e., consumers who choose not to purchase the item through either channel (
). (We refer the reader to EquationEquation (1)
(1)
(1) for a general formulation of the demand volumes across channels as a function the aforementioned events, which form the basis for the demand split across channels. We provide the analytical expressions for the respective proportions in .) This result is in line with the findings of Deloitte (Citation2017), which states that for retailers “that have direct to consumer channels (i.e., an existing retail location) digital platforms can not only complement the existing channel strategy, but also broaden the markets.” Hence, by enabling retailers to expand their consumer reach, online channels may allow retailers to capture greater number of consumers and increase revenues. This partially explains why retailers, such as Walmart, Target, and Kohl’s, have strategically turned to multiple channels in the past.
Figure 2. Market segmentation for (a) e-commerce non-adopters, and (b) e-commerce adopters for the case where and
.
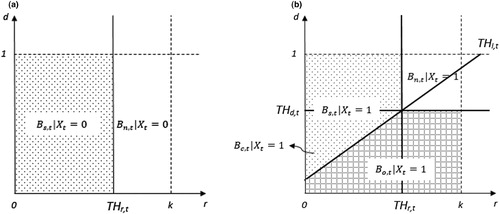
Table 1. Proportion of demand across channels as a function of ω and ξt
Lemma 1.
If , then the online channel expands the retailer’s market coverage by reducing the volume of choice-based lost demand in period t. Otherwise, there is no choice-based lost demand in period t, i.e.,
.
Lemma 1 suggests that for any product (defined by parameters v and p), there exists a threshold for ξt, i.e., the availability level that allows the retailer to capture the entire market demand within the store service radius, k. In particular, the retailer incurs no choice-based lost demand if . This finding is in line with empirical evidence that consumers are willing to travel further if certain stock availability (and/or price, which is exogenous in our model) is assured. As Grewal et al. (Citation2012) emphasize, this has an important implication: retailers located farther away from consumers need to ensure greater product availability in order to lure consumers to the store.
Lemma 2 fully characterizes the demand split across channels and the volume of choice-based lost demand for any given ω and ξt; see EquationEquation (1)(1)
(1) . Not surprisingly, the demand split depends on: (i) the retailer’s tactical decisions, including store service levels (αt, through dependence on ξt), and e-commerce marketing efforts (ω); (ii) the retailer’s business setting (k and l); (iii) product characteristics (v and p), and (iv) word-of-mouth effect. However, Lemma 2 also indicates that the retailer’s demand is piece-wise non-linear, which leads to a difficult optimization problem, as discussed in Section 4.
Lemma 2.
, and
, are (i) piece-wise continuous in
, and (ii) continuous and differentiable in
, and can be fully characterized as in .
4. The retailer’s optimization problem
In this section we study the retailer’s optimal demand shaping policy through e-commerce marketing efforts, , and store service levels over the planning horizon,
, in the presence of evolving adoption of e-commerce. We do this by explicitly considering the reference effects of such decisions within a multi-period setting.
Let , where p is the unit sales price, c is the retailer’s unit purchasing cost, and s is the unit lost sales cost, with s < c < p. That is, CF represents the “critical fractile” for the single-period newsvendor problem with exogenous demand. For analytical tractability, in the remainder of this article we restrict ourselves to the case where the initial service level equals critical fractile (
), and assume that CF > 0.547 (under this condition, the first-order optimality conditions become sufficient). This condition is satisfied for a variety of product categories such as fashion items, electronics, and home goods, as demonstrated in the numerical study of Section 6.
The retailer incurs a cost for its e-commerce marketing efforts. To improve presentation, we express the cumulative cost of marketing over the planning horizon by , which is convex increasing in the marketing effectiveness, ω. This is in line with the literature, e.g., “as with all inputs used in the production process, the marginal effectiveness of advertising diminishes as more and more is spent on advertising. Thus, advertising is subject to diminishing marginal returns.” (Mukherjee, Citation2002). Then, given the retailer’s marketing efforts, its expected profit function in period t,
, comprised of the expected sales revenue less the purchasing cost and the lost sales cost, is as follows:
(6)
(6)
Substituting EquationEquations (1) (1)
(1) to Equation(4)
(4)
(4) in EquationEquation (6)
(6)
(6) , the retailer’s optimization problem reduces to the following form (see the Appendix for derivations):
(7)
(7)
The retailer’s objective function is non-linear, non-separable in , and not necessarily well-behaved, as we show in Section 4.1. Consequently, in what follows, we study the structural properties of this problem. In particular, we first derive, in Section 4.1, the optimal service levels for a given marketing strategy,
. Then, in Section 4.2, we study the behavior of the objective function,
, in ω. This analysis leads to an efficient two-stage algorithm that provides the optimal solution to EquationEquation (7)
(7)
(7) . (See Appendix C for a discussion on the cost structure of the problem.)
4.1. The retailer’s optimal store service levels (stage 2 problem)
The following lemma exploits the structure of the retailer’s expected profit function for a given ω.
Lemma 3.
For any given is continuous, and (i) increasing in
for
, and (ii) jointly concave in
for
. Furthermore, for any
, and
.
Remark 1.
For the special case where λ = 0, for any
.
Lemma 3 indicates that demand endogeneity forces the optimal store service levels to be at least as high as the critical fractile. This intuitive finding is in line with, and extends upon, the results in the existing literature on inventory-driven demand in the single-channel single-period setting; see Dana and Petruzzi (Citation2001) and the references therein. Specifically, we show that such findings apply in a dynamic multi-channel multi-period setting in which: (i) a high service level in one channel (store) stimulates the channel’s own demand, while reducing the demand for the other channel (online), whose adoption level increases over time and is also impacted by the retailer’s decisions; (ii) consumers switch between channels in the case of a stock-out; and (iii) consumers learn over time. Indeed, as Remark 1 indicates, when there is no consumer learning (i.e., λ = 0), there is no dependence among the periods through store service levels and the problem reduces to the single-period newsvendor problem with exogenous demand, whose solution is given by CF.
In order to answer the research questions posed in Section 1, we next explore several structural properties of the retailer’s optimal service levels.
Theorem 1.
For a given and
, there exists a unique vector
such that the optimal solution
has the following structure:
where
and
, for
, and
, for
and
with
Theorem 1 fully characterizes the retailer’s optimal service levels for a given ω, and shows that they follow a threshold policy that is tailored to the retailer’s business setting (k) and product characteristics (v and p). Specifically, if W is sufficiently small, i.e., , then the retailer does not need to increase its store service levels beyond the critical fractile, as this policy enables the retailer to capture the entire market through the store channel, due to the sufficiently small service radius; see Lemma 1. Otherwise, the retailer increases its store service levels beyond the critical fractile. In particular, for relatively small W, i.e.
higher service levels, i.e.,
, allow the retailer to capture the entire market. However, higher service levels come at a cost. Therefore, for larger W, i.e., larger service radius, there is no benefit in increasing the service levels beyond a threshold
, and some choice-based lost demand becomes inevitable. (We provide an example to demonstrate the retailer’s optimal service levels as a function of k in Appendix D.) These findings may partially explain why many retailers such as Kohl’s and Target strive to “get closer to the customer” as a strategic move. For example, by introducing new stores, Kohl’s is able to expand its market reach beyond the traditional “suburban customer base” (Higgins, Citation2015). At a tactical level, this enables the retailer to exploit the consumer tradeoff between convenience and product availability (Grewal et al., Citation2012) and lower its service levels. Similarly, Target has announced that it will open 35 new stores in high-traffic urban areas and college campuses in 2018, with 12 suburban stores being closed in the same period (DeBaun, Citation2017). Such a strategy may naturally lead to changes in the market size, due to the adjustments in service radii, an aspect which is not explicitly captured in our model.
Observe that although the retailer’s optimal service levels for sufficiently small W are independent of the e-commerce marketing efforts (equivalently, e-commerce adoption level) and equal to CF, the growing e-commerce adoption does lead to changes in the optimal order quantity for each channel (), as highlighted in Theorem 2.
Theorem 2.
For each time period is decreasing,
is increasing, and
, is increasing in ω when
.
As e-commerce adoption grows, more people consider the online channel in their choice set. Although a portion of the additional online demand is due to those consumers, who would have constituted lost demand in the absence of an alternative channel, another portion is due to consumer migration from the store channel. Hence, the order quantity in the store (online) channel decreases (increases), while a net increase in the total order quantity prevails. This result is consistent with industry findings that a significant portion of the sales growth for multi-channel retailers comes from e-commerce (Business Insider, Citation2017). This has implications for retailers’ strategic decisions such as network design, warehousing, etc. In the next section, we study the retailer’s optimal marketing decision.
4.2. The retailer’s optimal marketing effort (stage 1 problem)
The following lemma characterizes the retailer’s expected profit function with respect to ω.
Lemma 4.
, is continuous in ω. Further:
1. For , for
, is strictly decreasing in ω when αt satisfies
and
2. For , for
, is strictly decreasing in ω when αt satisfies
Theorem 1 and Lemma 4 not only allow us to develop an effective two-stage algorithm to determine the retailer’s optimal solution, but also enable us to indicate the settings in which it is not profitable for the retailer to invest in e-commerce marketing to further increase online demand.
Theorem 3.
When , is strictly decreasing in ω.
Thus, if the retailer’s optimal service level strategy allows her to capture the entire market through the store channel (i.e., ), that is, no choice-based lost demand is incurred, then it is optimal to serve all consumers through the store channel only. Marketing for a competing online channel in this setting results in lower profits due to consumer migration. Hence, if the retailer opens an online channel as a strategic necessity, then e-commerce marketing efforts should be at a minimal level, i.e.,
goes to zero. In other cases, however, i.e., when the retailer cannot capture the entire market through the store channel only, the online channel becomes a complementary and profitable option. Therefore, in such settings, it is paramount that the retailer leverages its decision levers in order to attain the maximum profit. This result can be partially explained by industry findings that retailers that take pride in their close proximity to consumers, such as Walmart, Pier 1, and Michaels, are also the ones that are “criticized for their slowness to embrace digital shopping” (Forbes, Citation2017b). According to Forbes (Citation2017b), “more and more retailers are increasing their investment in online shopping and cross-channel integration only to experience a migration of sales from the store channel to e-commerce, frequently at lower profit.”
5. Impact of perfect information
In this section, we study the impact of information-sharing strategies retailers can adopt in order to improve consumers’ experience at the store. In particular, we study the setting in which the retailer shares with the consumers its store service level in the current period, i.e., . Then, consumers no longer need to predict the current store availability level. This can be achieved, to some extent, through mechanisms such as providing store service level information on the retailer’s website, mobile application, catalogs. This type of information sharing may incur some cost, which depends on the specific setting. We ignore this cost in our analysis, but it can easily be considered in the model. Our goal is to gain insight into the impact of this type of information sharing, which helps eliminate the consumers’ dependence on the partial information provided by other consumers in a multi-period setting. In theory, this approach is similar to the rational expectations paradigm, which suggests that consumers have correct beliefs on the retailer’s inventory decisions, and the retailer makes its decisions based on the correct beliefs of consumers’ purchasing response. As Wu et al. (Citation2015) state, rational expectations paradigm is normally adopted in single-period settings in which reference effects cannot be incorporated. By providing consumers with full/perfect information on availability, the rational expectations paradigm assumes that the retailer can optimize its inventory decisions, which would be accurately anticipated by the consumers.
We first present the structure of the retailer’s optimal solution for the perfect information setting, which we denote by the superscript P.
Lemma 5.
For a given is continuous, and (i) increasing in
for
, and (ii) jointly concave in
for
. Furthermore,
, for
.
Interestingly, the optimal store service levels in the perfect information setting become identical over time, i.e., independent of the consumers’ e-commerce adoption level. This surprising finding highlights that the retailer’s store service levels vary over time, mainly due to the fact that consumers need to predict them using past information, which may not accurately reflect the current availability level. From a technical perspective, this finding has an important implication: by providing consumers with availability information, the retailer reduces its optimization efforts, as it needs to solve the Stage 2 (service level) optimization problem only once regardless of the length of the planning horizon or e-commerce adoption level of the product. More importantly, from a practical perspective, this finding implies that by providing consumers with accurate information on the current store availability level, the retailer enjoys an optimal service level policy that is much easier to implement than that in the limited information (i.e., adaptive learning) setting.
The reasoning for this rather counter-intuitive finding is as follows. For a given ω in the perfect information model, there is no link between the different time periods (i.e., the profit in period t depends only on decision variable αt). That is, even though the adoption level Ft evolves throughout the planning horizon, the formulation of the retailer’s profit function is identical across time. Therefore, our multi-period problem reduces to a single-period problem in a perfect information setting. In each period, the retailer’s objective is to set service level αt in such a way that both e-commerce adopters and non-adopters are split across channels in an optimal way. In this setting, even though Ft determines the proportion of adopters and non-adopters within the consumer base, the channel choice decision for an adopter (or a non-adopter) is only dependent on the service level αt (or the expected probability of obtaining the item ) in a given time period t. That is, if service levels are kept identical over time, then the proportion of adopters (or non-adopters) who choose a certain channel would also be identical over time, as consumer decision-making remains the same. Therefore, if a certain inventory availability level provides the retailer with the highest profit in period t, it should also provide the highest profit in all other periods, as the split of adopters and non-adopters across channels remains the same. Consequently, the retailer does not need to adjust its service levels over time. Intuitively speaking, even though the number of adopters and non-adopters vary across time, each individual only considers the expected probability of obtaining the item (i.e., availability level) in their choice. Hence, as long as the retailer’s order quantities across channels are adjusted to meet the same availability level over time (under rising Ft), the retailer’s single service level decision guarantees optimal demand shaping. This finding is highlighted in Theorem 4.
Theorem 4.
For a given
, there exists a unique
such that the optimal solution,
, has the following structure:
For each time period
is decreasing,
is increasing, and
is increasing in ω;
When
, is strictly decreasing in ω.
Theorem 4 shows that the retailer’s optimal service level strategy continues to follow a threshold policy in the perfect information setting. More importantly, Theorem 4 states that the optimal order quantities across channels, , for
, should evolve over time in order to accommodate the change in the number of e-commerce adopters. Therefore, it is important to note that even though the optimal service levels remain constant over time, the dynamic nature of the problem is reflected in the time-varying (optimal) order quantities across channels throughout the planning horizon.
The results in Sections 4 and 5 enable us to bound the deviation between the optimal service levels in the limited versus perfect information settings. Specifically, Theorem 5 establishes an upper bound on the absolute difference between and
for any problem instance characterized by CF.
Theorem 5.
For any problem instance with a given CF, we have:
, for
if
;
for
if
,
where UB(CF) is an upper bound on , for a given CF, and corresponds to the solution of the following equation with λ = 1 and
:
Hence, the impact of information sharing on availability levels is especially important with sufficiently large service radii (i.e., ), and for products with small CF for which the absolute difference between
and
may go up to 8% (for CF = 0.57), see .
6. Numerical study
In this section, we present a numerical study based on real products to provide insight on how the retailer’s optimal demand shaping strategy is impacted by various parameters. We also quantify the impact of providing consumers with information on the retailer’s current store availability level.
6.1. Data and approach
We select three product categories that are currently at different phases of e-commerce adoption, i.e., early, established, and mature adoption phases. According to Egol et al. (Citation2012), a representative set of such product categories includes household items, apparel, and electronics, for which the percentage of e-commerce sales (over all retail sales) are given by 3%, 14%, and 34%, respectively, in 2012, when the overall e-commerce sales constituted 15.1% of the total retail sales. We use these percentages as a proxy for the current adoption levels (F0). According to Mahajan et al. (Citation1995), the parameter a for the word-of-mouth effect for monthly diffusion typically lies between 0.025 and 0.042. Hence, in our numerical study, we use for the household, apparel, and electronics items, respectively, for a planning horizon of four periods (T = 4), and with a random market size of
. (Similar findings are obtained for various other parameter values.)
For each product category, we choose a dual-channel retailer (Garmin Ltd., Citation2015; Fossil, Inc., Citation2015; The Yankee Candle Company, Inc., Citation2015) and an item that the corresponding retailer offers online (candle, wallet, and auto navigator). We determine the item’s online price, p, through the retailer’s website, and assume that the item is offered in the store at the same price and that its lost sale cost is zero (s = 0). We then use the published gross profit margin, , which equals the critical fractile for the product when s = 0, for each retailer (The Wall Street Journal, Citation2013: CSIMarket, Inc., Citation2015) to estimate the retailer’s purchasing cost, c (see ).
Table 2. Financial data for the items considered in the numerical study
6.2. Results
We first use the candle example as a representative product to demonstrate how the retailer’s optimal decisions and the resulting profit change with various problem parameters when in and . (The findings are similar for the other products considered.) The retailer’s optimal solution is trivial otherwise, see Theorems 1 and 3. Then, we compare the optimal decisions for all three products in . We consider that the e-commerce marketing cost function is in the form,
, with
, and assume
, and
for all products (unless otherwise noted). Retailers may work in different business environments, with different k and l parameters; consumers’ product valuation v can also vary across products. Therefore, we also conduct a sensitivity analysis with respect to k, l and v parameters, see Appendix D.
Table 3. Retailer’s optimal decisions and resulting expected profit for the (a) adaptive learning, and (b) perfect information settings, with a = 0.025 and
Table 4. Retailer’s optimal decisions and resulting expected profit under (a) various values of current adoption level, and (b) various values of word-of-mouth effect, with
Table 5. Retailer’s optimal decisions and resulting expected profit for the three products
and shows how the retailer’s optimal solution and the resulting profit change with λ. Interestingly, for smaller values of λ we observe a monotone pattern over time, i.e., , whereas for larger λ, the monotonicity disappears. This is because a smaller λ implies that the consumer places more (less) weight on her earlier (most recent) experience(s), which amplifies the effect of earlier availability levels on the retailer’s demand-shaping ability in the later stages. As a result, when λ is small (e.g.,
for the example in ), the optimal service levels decrease over time. As λ grows, however, the most recent observations start having a higher impact on the predicted availability level in a given period. With a higher λ, consumers adjust to the new information faster, and this incentivizes the retailer to increase its recent service levels, which are quickly transformed into higher predicted store availability by the consumers. This results in an overall growth in the service levels as λ increases, eventually making a non-monotonic service level pattern possible. Since store service levels and e-commerce marketing efforts pose a cost tradeoff, higher service levels lead to a lower investment in e-commerce marketing as λ gets higher. However, the retailer benefits from faster consumer learning, i.e., the expected profit increases in λ. In Appendix D, we provide a sensitivity analysis that shows the impact of the planning horizon on the retailer’s optimal decisions (Table 10 of Appendix D).
shows that when consumers are provided with accurate information on store inventory availability, both the retailer’s optimal total order quantity, i.e., , (hence, the number of consumers satisfied) and the optimal expected profit increase compared with the adaptive learning (limited information) setting. Furthermore, as λ increases, the optimal solution for the adaptive learning setting approaches the perfect information setting. Hence, sharing availability information with consumers is most beneficial in settings with slow-learning consumers. Finally, the percent loss in expected profit (
) due to not sharing the availability information with consumers may exceed 2% (when λ = 0), which can be significant in the retailing industry. In Table 11 of Appendix D, we present the values of some intermediary variables, e.g., the expected sales quantities across channels, at the optimal solutions provided in . Our findings show that both the store and the online sales exhibit dynamic change in volume across time, with store sales always exceeding the online sales volumes. Furthermore, our results show that the majority of the online sales can be attributed to additional sales due to market expansion.
Not surprisingly, the product’s current e-commerce adoption level has a large impact on the retailer’s optimal policy. For a given λ (), illustrates that as the initial adoption level increases, the retailer’s e-commerce marketing efforts decrease as expected, and the retailer can afford to increase its store service levels. Furthermore, as the dual-channel option becomes available to more consumers, the retailer satisfies a higher demand (due to a decrease in choice-based lost demand), and the retailer’s expected profit increases.
Interestingly, an increase in the word-of-mouth effect (a), which stimulates e-commerce adoption, leads to a non-monotone pattern in the retailer’s e-commerce marketing effort, as shown in . In particular, for sufficiently small (large) values of a, the retailer’s optimal marketing effort increases (decreases) in a. The word-of-mouth effect, a, not only affects the magnitude of the adoption level, but it also affects the shape of the overall adoption curve. Depending on the magnitude and speed of the overall growth in adoption during the planning horizon, the retailer may adopt a different marketing strategy, which, according to our results, need not follow a monotone pattern.
Finally, , which reports the optimal strategy for candle, wallet and auto navigator, underscores that the retailer’s optimal demand shaping strategy greatly depends on the product’s characteristics, including the e-commerce adoption phase as well as profit and sales margins, and a customized strategy highly benefits the retailer.
7. Conclusions and future research directions
In this article we study a dual-channel retailer’s demand shaping strategy in the presence of growing e-commerce adoption. Our findings indicate that while promoting e-commerce adoption through marketing efforts benefits the retailer by enabling it to expand its market coverage in large service radii, this strategy does not work for all retailers: when the majority of the consumers are located within close proximity to the store, e-commerce marketing leads to consumer migration, which is not necessarily profitable (see Forbes, Citation2017b). In such settings, retailers can exploit the consumer tradeoff between convenience and product availability to expand their market coverage. This enables retailers to maximize their profit via lower service levels. Otherwise, the retailer’s optimal demand shaping policy critically depends on the product’s e-commerce adoption phase. For example, not surprisingly, we find that if a product is at a mature adoption phase, then the retailer does not need to promote the online channel as aggressively as in the earlier adoption phases, but needs to increase the store service levels. By incorporating the reference effects of retailer’s prior service level decisions on consumer decision-making in this multi-period setting, we show that the retailer’s optimal service levels vary over time, mainly due to the fact that consumers need to predict the retailer’s current availability level using prior information. That is, if the retailer provides the consumers with accurate information on their current store availability level, then the retailer’s optimal service levels do not need to vary over time, even as e-commerce adoption grows. This implies that by providing consumers with perfect information on current store availability level, the retailer enjoys an optimal service level policy that is much easier to implement than the optimal policy in the limited information setting.
Our model, in its current form, assumes a uniform cost structure across channels, represented by unit product purchasing cost, as additional costs are highly dependent on the retailer’s business model. It is an important future research direction to expand our model to conduct case studies of retailers with varying cost structures. For example, operating a certain channel may offer several benefits, in terms of risk pooling and lower fulfillment costs. Since we study a dual-channel retailer’s tactical decisions, we ignore certain aspects of the problem, such as inventory carry over and backlogging; hence, it would be interesting to study an extension of this problem at an operational level, considering such components. An important research direction is to incorporate other decision levers in problem formulation, such as the ability to expand the service radius through investment. Lastly, studying this problem in a competitive setting would provide valuable insights with respect to dual-channel retailers’ marketing and inventory decisions. Despite these modeling assumptions, we believe that the insights gained can be of value to retailers on their demand shaping decisions under growing e-commerce adoption, and that the innovative modeling aspects of our work can provide a theoretical framework that can be used as a basis for a realistic tool, which can be used in practice.
Supplemental Material
Download PDF (396.2 KB)Acknowledgments
We thank Prof. Enver Yucesan, the Associate Editor, and three anonymous referees for their suggestions that greatly improved the content and the presentation of the paper.
Additional information
Notes on contributors
Nevin Mutlu
Nevin Mutlu is an assistant professor in the School of Industrial Engineering at Eindhoven University of Technology, the Netherlands. Her research interests include retail operations, revenue management, and logistics with a focus on customer behavior. She is the recipient of the prestigious EU Horizon 2020 Marie Sklodowska-Curie Individual Fellowship.
Ebru K. Bish
Ebru K. Bish is an associate professor in the Grado Department of Industrial and Systems Engineering and an associate professor of Health Sciences at Virginia Tech. Her research interests are on stochastic modeling and optimization, with applications in supply chain management, logistics, and healthcare operations. She is the recipient of the INFORMS JFIG best paper award, IISE Transactions Best Applications Paper award, and the INFORMS Pierskalla award.
References
- Agatz, N.A., Fleischmann, M. and van Nunen, J.A. (2008) E-fulfillment and multi-channel distribution–A review. European Journal of Operational Research, 187(2), 339–356.
- Akerman, G. (1957) The cobweb theorem: A reconsideration. The Quarterly Journal of Economics, 71(1), 151–160.
- Balakrishnan, A., Sundaresan, S. and Zhang, B. (2014) Browse-and-switch: Retail-online competition under value uncertainty. Production and Operations Management, 23(7), 1129–1145.
- Barabba, V., Huber, C., Cooke, F., Pudar, N., Smith, J. and Paich, M. (2002) A multimethod approach for creating new business models: The General Motors OnStar project. Interfaces, 32(1), 20–34.
- Bass, F. (1969) A new product growth for model consumer durables. Management Science, 15(5), 215–227.
- Bernstein, F. and Federgruen, A. (2004). A general equilibrium model for industries with price and service competition. Operations Research, 52(6), 868–886.
- Boyaci, T. (2005) Competitive stocking and coordination in a multiple-channel distribution system. IIE Transactions, 37(5), 407–427.
- Business Insider (2017) Walmart’s online sales are exploding. http://www.businessinsider.com/walmarts-online-sales-are-exploding-2017-5?international=true&r=US&IR=T. Accessed December 2017.
- Cattani, K., Gilland, W., Heese, H.S. and Swaminathan, J. (2006) Boiling frogs: Pricing strategies for a manufacturer adding a direct channel that competes with the traditional channel. Production and Operations Management, 15(1), 40–56.
- Cattani, K.D., Gilland, W.G. and Swaminathan, J.M. (2004) Coordinating traditional and internet supply chains, in Handbook of Quantitative Supply Chain Analysis. pp. 643–677, Springer, Boston, MA.
- Chen, K.Y., Kaya, M. and Özer, Ö. (2008) Dual sales channel management with service competition. Manufacturing & Service Operations Management, 10(4), 654–675.
- Chiang, W.K. and Chhajed, D. (2005) Multi-channel supply chain design in B2C electronic commerce, in Supply Chain Optimization. pp. 145–168, Springer, Boston, MA.
- Chiang, W.K., Chhajed, D. and Hess, J.D. (2003) Direct marketing, indirect profits: A strategic analysis of dual-channel supply-chain design. Management Science, 49(1), 1–20.
- CSIMarket, Inc. (2015) Consumer cyclical. http://csimarket.com/Industry/Industry_Profitability.php?s=400. Accessed July 2015.
- Dana, Jr., J.D. (2001) Competition in price and availability when availability is unobservable. Rand Journal of Economics, 32(3), 497–513.
- Dana, Jr., J.D. and Petruzzi, N.C. (2001) Note: The newsvendor model with endogenous demand. Management Science, 47(11), 1488–1497.
- DeBaun, D. (2017) Target closing a dozen stores next year, including two in Minnesota. https://www.bizjournals.com/twincities/news/2017/11/07/target-closing-a-dozen-stores-next-year-including.html. Accessed May 2018.
- Debo, L.G. and Veeraraghavan, S.K. (2009) Models of herding behavior in operations management, in Consumer-driven Demand and Operations Management Models: A Systematic Study of Information-technology-enabled Sales Mechanisms. pp. 81–112, Springer, New York, NY.
- Deloitte (2017) Going digital, going direct. https://www2.deloitte.com/content/dam/Deloitte/ca/Documents/consumer-business/ca-en-consumer-business-going-digital-going-direct.pdf. Accessed November 2017.
- Dodson, J. (2014) New product forecasting: The Bass model. http://www.learningace.com/doc/531160/fc1252ce3fc46224928578de3c098d6 0/mktgtool_bass. Accessed November 2017.
- E-commerce Europe (2016) European B2C E-commerce report 2016. https://www.ecommerce-europe.eu/app/uploads/2016/07/European-B2C-E-commerce-Report-2016-Light-Version-FINAL.pdf. Accessed November 2016.
- Egol, M., Rajagopalan, A. and Sayer, B. (2012) E-commerce and consumer goods. A strategy for omnichannel success. http://www.strategyand.pwc.com/media/file/Strategyand_E-Commerce-and-Consumer-Goods.pdf. Accessed July 2015.
- Forbes (2016) Utilizing virtual reality to revolutionize the eCommerce market. https://www.forbes.com/sites/haroldstark/2016/11/23/utilizing-virtual-reality-to-revolutionize-the-ecommerce-market/#42132ef26b6a. Accessed November 2017.
- Forbes (2017a) 6 things that can vastly improve your word of mouth marketing. https://www.forbes.com/sites/steveolenski/2017/08/11/6-things-that-can-vastly-improve-your-word-of-mouth-marketing/#5c5a4f1d16a5. Accessed November 2017.
- Forbes (2017b) The inconvenient truth about E-Commerce: It’s largely unprofitable. https://www.forbes.com/sites/stevendennis/2017/03/17/the-inconvenient-truth-about-e-commerce/#707c618f1bb2. Accessed November 2017.
- Fossil, Inc. (2015) Wallets. http://www.fossil.com/en_US/shop/women/wallets.html?parent_category_rn=357083&departmentCategoryId=331097. Accessed July 2015.
- Gao, F. and Su, X. (2016) Omnichannel retail operations with buy-online-and-pickup-in-store. Management Science. 63(8), 2478–2492.
- Garmin Ltd. (2015) Cars. https://buy.garmin.com/en-US/US/on-the-road/automotive/cOnTheRoad-cAutomotive-p1.html. Accessed July 2015.
- Grewal, D., Kopalle, P., Marmorstein, H. and Roggeveen, A.L. (2012) Does travel time to stores matter? The role of merchandise availability. Journal of Retailing, 88(3), 437–444.
- Higgins, J. (2015) Get small to grow sales. http://indigocenters.com/featured/get-small-grow-sales/. Accessed November 2017.
- IBM (2017) E-commerce statistics & technology trendsetters for 2017. https://www.ibm.com/developerworks/community/blogs/d27b1c65-986e-4a4f-a491-5e8eb23980be/entry/Ecommerce_Statistics_Technology_Trendsetters_for_20171?lang=en. Accessed November 2017.
- Intel (2013) Using retail marketing data to help curb inventory distortion. https://www.intel.com/content/dam/www/public/us/en/documents/solution-briefs/using-retail-marketing-data-curb-inventory-distortion-brief.pdf. Accessed November 2017.
- Kacen, J.J., Hess, J.D. and Chiang, W.K. (2013) Bricks or clicks? Consumer attitudes toward traditional stores and online stores. Global Economics and Management Review, 18(1), 12–21.
- Levin, A.M., Levin, I.P. and Weller, J.A. (2005) A multi-attribute analysis of preferences for online and offline shopping: Differences across products, consumers, and shopping stages. Journal of Electronic Commerce Research, 6(4), 281–290.
- Lewis, T. and Long, B. (2009) Case analysis studies of diffusion models on e-commerce transaction data. Master’s thesis, Massachusetts Institute of Technology, Sloan School of Management, Cambridge, MA.
- Liu, Q. and van Ryzin, G. (2011) Strategic capacity rationing when customers learn. Manufacturing & Service Operations Management, 13(1), 89–107.
- Mahajan, V., Muller, E. and Bass, F.M. (1995) Diffusion of new products: Empirical generalizations and managerial uses. Marketing Science, 14(3), G79–G88.
- McFadden, D. (1973) Conditional logit analysis of qualitative choice behaviour, in Frontiers in Econometrics. pp. 105–142, Academic Press, New York, NY.
- Monroe, K.B. (1973) Buyers’ subjective perceptions of price. Journal of Marketing Research, 10(1), 70–80.
- Mukherjee, S. (2002) Modern Economic Theory, New Age International, New Delhi.
- Netessine, S. and Tang, C.S. (2009) Introduction, in Consumer-driven Demand and Operations Management Models: A Systematic Study of Information-technology-enabled Sales Mechanisms. pp. ix–xiii, Springer, New York, NY.
- Popescu, I. and Wu, Y. (2007) Dynamic pricing strategies with reference effects. Operations Research, 55(3), 413–429.
- Rogers, E. (1962). Diffusion of Innovations, Free Press of Glencoe, New York, NY.
- Sterman, J.D. (1989) Modeling managerial behavior: Misperceptions of feedback in a dynamic decision making experiment. Management Science, 35(3), 321–339.
- Sterman, J.D. (2000) Business Dynamics: Systems Thinking and Modeling for a Complex World, Irwin/McGraw-Hill, Boston, MA.
- Su, X. and Zhang, F. (2009) On the value of commitment and availability guarantees when selling to strategic consumers. Management Science, 55(5), 713–726.
- The U.S. Department of Commerce (2017) Monthly and annual retail trade. http://www.census.gov/retail/. Accessed January 2017.
- The Wall Street Journal (2013) Yankee Candle agrees to $1.75 billion deal. http://www.wsj.com/articles/SB10001424127887324202304579051044165409288. Accessed July 2015.
- The Yankee Candle Company, Inc. (2015) Jar Candles. http://www.yankeecandle.com/browse/candles/jar-candles/_/N-8cd. Accessed July 2015.
- Tirole, J. (1988) The Theory of Industrial Organization, MIT Press, Cambridge, MA.
- Tsay, A.A. and Agrawal, N. (2004a) Modeling conflict and coordination in multi-channel distribution systems: A review, in Handbook of Quantitative Supply Chain Analysis. pp. 557–606, Springer, Boston, MA.
- Tsay, A.A. and Agrawal, N. (2004b) Channel conflict and coordination in the e-commerce age. Production and Operations Management, 13(1), 93–110.
- Wu, S., Liu, Q. and Zhang, R.Q. (2015) The reference effects on a retailers dynamic pricing and inventory strategies with strategic consumers. Operations Research, 63(6), 1320–1335.
- Yoo, W.S. and Lee, E. (2011) Internet channel entry: A strategic analysis of mixed channel structures. Marketing Science, 30(1), 29–41.