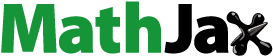
Abstract
Given sufficiently many components, it is often cited that finite mixture models can approximate any other probability density function (pdf) to an arbitrary degree of accuracy. Unfortunately, the nature of this approximation result is often left unclear. We prove that finite mixture models constructed from pdfs in can be used to conduct approximation of various classes of approximands in a number of different modes. That is, we prove approximands in
can be uniformly approximated, approximands in
can be uniformly approximated on compact sets, and approximands in
can be approximated with respect to the
, for
. Furthermore, we also prove that measurable functions can be approximated, almost everywhere.
PUBLIC INTEREST STATEMENT
Finite mixture models are an expansive and expressive class of probability models that have been successfully applied in many situations where data follow a complex generative process that may be highly heterogeneous. It has long been known that finite mixture models, under sufficient regularity conditions, can approximate any probability density functions to arbitrary degrees of accuracy, and such results have been established under varying assumptive restrictions. Our work seeks to provide the weakest set of assumptions in order to establish approximation theoretic results over the widest class of probability density problems, possible. The result provides further evidence towards the success of mixture models in applications, and provides mathematical guarantees to practitioners who apply mixture models in their analytic problems.
1 Introduction
Let be an element in the Euclidean space, defined by
and the norm
, for some
. Let
be a function, such that
, everywhere, and
, where
is the Lebesgue measure. We say that
is a probability density function (pdf) on the domain
(an expression that we will drop, from hereon in). Let
be another pdf, and for each
, define the functional class:
where ,
,
, and
is the matrix transposition operator. We say that any
is a
location-scale finite mixture of the pdf
.
The study of pdfs in the class is an evergreen area of applied and technical research, in statistics. We point the interested reader to the many comprehensive books on the topic, such as Everitt & Hand (Citation1981), Titterington et al. (Citation1985), McLachlan & Basford (Citation1988), Lindsay (Citation1995), McLachlan & Peel (Citation2000), Fruwirth-Schnatter (Citation2006), Schlattmann (Citation2009), Mengersen et al. (Citation2011), and Fruwirth-Schnatter et al. (Citation2019).
Much of the popularity of finite mixture models stem from the folk theorem, which states that for any density , there exists an
, for some sufficiently large number of components
, such that
approximates
arbitrarily closely, in some sense. Examples of this folk theorem come in statements such as “provided the number of component densities is not bounded above, certain forms of mixture can be used to provide arbitrarily close approximation to a given probability distribution”(Titterington et al., Citation1985, p. 50), “the [mixture] model forms can fit any distribution and significantly increase model fit” (Walker and Ben-Akiva, Citation2011, p. 173), and “a mixture model can approximate almost any distribution” (Yona, Citation2011, p. 500). Other statements conveying the same sentiment are reported in Nguyen & McLachlan (Citation2019). There is a sense of vagary in the reported statements, and little is ever made clear regarding the technical nature of the folk theorem.
In order to proceed, we require the following definitions. We say that is compactly supported on
, if
is compact and if
, where
is the indicator function that takes value 1 when
and
, elsewhere, and
is the set complement operator (i.e.,
). Here,
is a generic subset of
. Furthermore, we say that
for any
, if
and for , if
where we call the
on
. When
, we shall write
. In addition, we define the so-called Kullback-Leibler divergence, see Kullback & Leibler, (Citation1951), between any two pdfs
and
on
as
In Nguyen & McLachlan (Citation2019), the approximation of pdfs by the class
was explored in a restrictive setting. Let
be a sequence of functions that draw elements from the nested sequence of sets
(i.e.,
). The following result of Zeevi & Meir (Citation1997) was presented in Nguyen & McLachlan (Citation2019), along with a collection of its implications, such as the results of from Li & Barron (Citation1999) and Rakhlin et al. (Citation2005).
Theorem 1 (Zeevi and Meir, Citation1997). If
and are pdfs and
is compact, then there exists a sequence
such that
Although powerful, this result is restrictive in the sense that it only permits approximation in the norm on compact sets
, and that the result only allows for approximation of functions
that are strictly positive on
. In general, other modes of approximation are desirable, in particular, approximation in
for
or
are of interest, where the latter case is generally referred to as uniform approximation. Furthermore, the strict-positivity assumption, and the restriction on compact sets limits the scope of applicability of Theorem 1. An example of an interesting application of extensions beyond Theorem 1 is within the
approximation framework of Devroye & Lugosi (Citation2000).
Let again be a pdf. Then, for each
, we define
which we call the set of location-scale linear combinations of the pdf
. In the past, results regarding approximations of pdfs
via functions
have been more forthcoming. For example, in the case of
, where
is the standard normal pdf. Denoting the class of continuous functions with support on by
. We have the result that for every pdf
, compact set
, and
, there exists an
and
, such that
(Sandberg, 2001, Lem. 1). Furthermore, upon defining the set of continuous functions that vanish at infinity by
we also have the result: for every pdf and
, there exists an
and
, such that
[32, Thm. 2]. Both of the results from Sandberg (Citation2001) are simple implications of the famous Stone-Weierstrass theorem (cf. Stone, Citation1948 and De Branges, Citation1959).
To the best of our knowledge, the strongest available claim that is made regarding the folk theorem, within a probabilistic or statistical context, is that of DasGupta (Citation2008, Thm. 33.2). Let be a sequence of functions that draw elements from the nested sequence of sets
, in the same manner as
. We paraphrase the claim without loss of fidelity, as follows:
Claim 1. If are pdfs and
is compact, then there exists a sequence
, such that
Unfortunately, the proof of Claim 1 is not provided within DasGupta (Citation2008). The only reference of the result is to an undisclosed location in Cheney & Light (Citation2000), which, upon investigation, can be inferred to be Theorem 5 of Cheney & Light (Citation2000), Ch. 20. It is further notable that there is no proof provided for the theorem. Instead, it is stated that the proof is similar to that of Theorem 1 in Cheney & Light (Citation2000, Ch. 24), which is a reproduction of the proof for Xu et al. (Citation1993, Lem. 3.1).
There is a major problem in applying the proof technique of Xu et al. (Citation1993, Lem. 3.1) in order to prove Claim 1. The proof of [Xu et al. (Citation1993, Lem. 3.1)] critically depends upon the statement that “there is no loss of generality in assuming that for
“. Here, for
,
. The assumption is necessary in order to write any convolution with
and an arbitrary continuous function as an integral over a compact domain, and then to use a Riemann sum to approximate such an integral. Subsequently, such a proof technique does not work outside the class of continuous functions that are compactly supported on
. Thus, one cannot verify Claim 1 from the materials of Xu & Light, (Citation1993), Cheney & Light (Citation2000), and DasGupta (Citation2008), alone.
Some recent results in the spirit of Claim 1 have been obtained by Nestoridis & Stefanopoulos, (Citation2007) and Nestoridis et al. (Citation2011), using methods from the study of universal series (see, for example, in Nestoridis & Papadimitropoulos, Citation2005).
Let
denote the so-called Wiener’s algebra (see, e.g. Feichtinger, Citation1977)) and let
be a class of functions with tails decaying at a faster rate than . In Nestoridis et al. (Citation2011), it is noted that
. Further, let
denote the set of compactly supported continuous functions. The following theorem was proved in Nestoridis & Stefanopoulos (Citation2007).
Theorem 2 (Nestoridis and Stefanopoulos, Citation2007, Thm. 3.2). If , then the following statements hold.
(a) For any , there exists a sequence
(
), such that
(b) For any , there exists a sequence
(
), such that
(c) For any and
, there exists a sequence
(
), such that
(d) For any measurable , there exists a sequence
(
), such that
(e) If is a
Borel measure on
, then for any
, there exists a sequence
(
), such that
almost everywhere, with respect to .
The result was then improved upon, in Nestoridis et al. (Citation2011), whereupon the more general space was taken as a replacement for
, in Theorem 2. Denote the class of bounded continuous functions by
. The following theorem was proved in Nestoridis et al. (Citation2011).
Theorem 3 (Nestoridis et al., Citation2011, Thm. 3.2). If , then the following statements are true.
(a) The conclusion of Theorem 2(a) holds, with replaced by
.
(b) The conclusions of Theorem 2(b)–(e) hold.
(c) For any and compact
, there exists a sequence
, such that
Utilizing the techniques from Nestoridis & Stefanopoulos, (Citation2007), Bacharoglou, (Citation2010) proved a similar set of results to Theorem 2, under the restriction that is a non-negative function with support
, using
(i.e.
has form (1), where
) and taking
as the approximating sequence, instead of
. That is, the following result is obtained.
Theorem 4 (Bacharoglou, Citation2010, Cor. 2.5). If , then the following statements are true.
(a) For any pdf , there exists a sequence
(
), such that
(b) For any , such that
, there exists a sequence
(
), such that
(c) For any and
, such that
, there exists a sequence
(
), such that
(d) For any measurable , there exists a sequence
(
), such that
(e) For any pdf , there exists a sequence
(
), such that
To the best of our knowledge, Theorem 4 is the most complete characterization of the approximating capabilities of the mixture of normal distributions. However, it is restrictive in two ways. First, it does not permit the characterization of approximation via the class for any
except the normal pdf
. Although
is traditionally the most common choice for
in practice, the modern mixture model literature has seen the use of many more exotic component pdfs, such as the student-t pdf and its skew and modified variants (see, e.g. Peel & McLachlan, Citation2000, Forbes & Wraith, Citation2013, and Lee & McLachlan, Citation2016). Thus, its use is somewhat limited in the modern context. Furthermore, modern applications tend to call for
, further restricting the impact of the result as a theoretical bulwark for finite mixture modeling in practice. A remark in Bacharoglou, (Citation2010) states that the result can be generalized to the case where
instead of
. However, no suggestions were proposed, regarding the generalization of Theorem 4 to the case of
.
In this article, we prove a novel set of results that largely generalize Theorem 4. Using techniques inspired by Donahue et al. (Citation1997) and Cheney & Light, (Citation2000), we are able to obtain a set of results regarding the approximation capability of the class of mixture models
, when
or
, and for any
. By definition of
, the majority of our results extend beyond the proposed possible generalizations of Theorem 4.
The article proceeds as follows: Our main theorem is stated and its separate parts are proved in Section 2. Comments and discussion are provided in Section 3. Necessary technical lemmas and results are also included, for reference, in the Appendix.
2. Main result
The remainder of the article is devoted to proving the following theorem.
Theorem 5 (Main result). If we assume that and
are pdfs and that
, then the following statements are true.
(a) For any , there exists a sequence
(
), such that
(b) For any and compact
, there exists a sequence
(
), such that
(c) For any and
, there exists a sequence
(
), such that
(d) For any measurable , there exists a sequence
(
), such that
(e) If is a
Borel measure on
, then for any
, there exists a sequence
(
), such that
almost everywhere, with respect to .
If we assume instead that , then the following statement is also true.
(f) For any , there exists a sequence
(
), such that
2.1. Technical preliminaries
Before we begin to prove the main theorem, we establish some technical results regarding our class of component densities . Let
and denote the convolution of
and
by
. Further, we denote the sequence of dilates of
by
The following result is an alternative to Lemma 5 and Corollary 1. Here, we replace a boundedness assumption on the approximand, in the aforementioned theorem by a vanishing at infinity assumption, instead.
Lemma 1. Let be a pdf and
, such that
. Then,
Proof. It suffices to show that for any , there exists a
, such that
, for all
. By Lemma 6,
, and thus
. By making the substitution
, we obtain for each
By Corollary 1, we obtain and thus we can choose a
, such that
Since is a pdf, we have
By uniform continuity, for any , there exists a
such that
, for any
, such that
(Lemma 6). Thus, on the one hand, for any
, we can pick a
such that
and on the other hand
The proof is completed by summing (2) and (3).□
Lemma 2. If is such that
, and
, then there exists a
, such that
, and
Proof. Since , there exists a compact
such that
. By Lemma 7, there exists some
, such that
and
. Let
, which implies that
and
. Furthermore, notice that
and
, by construction. The proof is completed by observing that
For any , uniformly continuous function
, let
denote the modulus of continuity of . Furthermore, define the diameter of a set
by
and denote an open ball, centered at
with radius
by
.
Notice that the class can be parameterized as
where and
. The following result is the primary mechanism that permits us to construct finite mixture approximations for convolutions of form
. The argument motivated by the approaches taken in Theorem 1 in Cheney & Light (Citation2000, Ch. 24), Nestoridis & Stefanopoulos (Citation2007, Lem. 3.1), and Nestoridis et al. (Citation2011, Thm. 3.1).
Lemma 3. Let and
be pdfs. Furthermore, let
be compact and
, where
and
. Then for any
, there exists a sequence
, such that
Proof. It suffices to show that for any and
, there exists a sufficiently large enough
so that for all
such that
For any , we can write
Here, is continuous image of a compact set, and hence is compact (cf. Rudin, Citation1976, Thm. 4.14]). By Lemma 8, for any
, there exists
(
,
), such that
. Further, if
, then we have
. We can obtain a disjoint covering of
by taking
and
(
) and noting that
, by construction (cf. Cheney & Light, Citation2000, Ch. 24). Furthermore, each
is a Borel set and
.
For convenience, let denote the disjoint covering, or partition, of
. We seek to show that there exists an
and
, such that
where ,
and , for
.
Further, and
, with
chosen as follows: By Lemma 6,
for some positive
. Then,
. We may choose
so that
, so that
Since , the sum of
(
) satisfies the inequality
Thus, , and our construction implies that
where
We can bound the left-hand side of (4) as follows:
Since
we have , for each
. Since
(cf. Makarov & Podkorytov, Citation2013, Thm. 4.7.3), we may choose a
so that
. We may proceed from (5) as follows:
To conclude the proof, it suffices to choose an appropriate sequence of partitions , for some large but finite
, so that (5) and (6) hold, which is possible by Lemma 8.□
For any , let
be a closed ball of radius
, centered at the origin.
Lemma 4. If , such that
, then
Proof. By construction, each element of the sequence (
) is measurable,
, and
point-wise. We obtain our conclusion via the Lebesgue dominated convergence theorem.□
2.2. Proof of theorem 5(a)
We now proceed to prove each of the parts of Theorem 5. To prove Theorem 5(a) it suffices to show that for every , there exists a
, such that
Start by applying Lemma 2 to obtain , such that
and
. Then, we have
The goal is to find a , such that
. Since
, we may find a compact
such that
. Apply Lemma 1 to show the existence of a
, such that
for all . With a fixed
, apply Lemma 3 to show that there exists a
, such that
By the triangle inequality, we have
The proof is complete by substitution of (8) into (7).
2.3. Proof of Theorem 5(b)
For any and compact
, it suffices to show that there exists a sufficiently large enough
so that for all
such that
.
By Lemma 5, we can find a , such that
for every . Since
,
for some positive
, by Lemma 6. For any
, via Young’s convolution inequality:
For fixed , we may choose
, using Lemma 4, so that
and thus the final term of (10) is bounded from above by
for all
. Thus, for
and,
Using Lemma 3, with approximand , component density
, compact set
,
, and with
fixed, we have the existence of a density
such that
We obtain the desired result by combining (9), (11), and (12), via the triangle inequality.
2.4. Proof of Theorem 5(c)
The technique used to prove Theorem 5(c) is different from those used in the previous sections. Here, we use a result of Donahue et al. (Citation1997) that generalizes the classic Barron-Jones Hilbert space approximation result (cf. Jones, Citation1992 and Barron, Citation1993) to Banach spaces.
To prove Theorem 5(c), it suffices to show that for every , there exists a sufficiently large enough
so that for all
such that
. Begin by applying Corollary 1 to obtain a
, such that
for all .
For some pdf and fixed
, let us define the class
write the convex hull of
as
and call the convex hull of
. We further say that
is the closure of
.
Because is a pdf,
, and
, we observe that
. Thus,
, for any
, by Lemma 9. Since
is a pdf and
, we have the existence of
and the fact that
is finite.
Furthermore, for any , since
and by definition of
, we have
Thus, we have
by choosing .
Following van de Geer (Citation2003), we can write the closure of as
and thus we immediately have . Combined with (14), we can apply Lemma 11 to obtain the conclusion that there exists a function
, such that
where and
is a finite constant. Since
,
is strictly increasing, and hence we can choose an
, such that for all
,
The proof is then completed by combining (13) and (15) via the triangle inequality.
2.5. Proof of Theorem 5(d) and Theorem 5(e)
By Theorem 5(a), there exists a sequence that uniformly converges to
, as
. Thus, by Lemma 12,
almost uniformly converges to
and also converges almost everywhere, to
, with respect to any measure
. We prove Theorem 5(d) by setting
and we prove Theorem 5(e) by not specifying
.
2.6. Proof of Theorem 5(f)
It suffices to show that for any , there exists a sufficiently large enough
so that for all
, where
, such that
. Begin by applying Lemma 4 in order to find a
, for any
, such that for all
,
where , and
with compact support
.
Let and apply the triangle inequality to obtain
Hence, we need to show that there exists a function , such that
Since and
, by substitution, we have
where are independent of
. By Lemma 5 and Corollary 1, we can obtain a
, such that for all
,
Suppose that and let
where
By construction, and thus there exists a
such that
, for any
.
For any , we can show that
To do so, firstly, for any ,
To obtain a Riemann sum approximation of , we use an argument analogous to that of Lemma 3. That is, we partition
into
disjoint Borel sets
, and we approximate
by a
, where for each
,
,
, and
Define ,
, and
, where
by (16). Then, by a similar argument to Lemma 3, for all
and
. Thus, we may define an element
via the parameters above.
For sufficiently large , we use Lemma 3 to show that
which implies
and thus (19) is proved. Using (19), we write
where since
is a pdf. The aim is now to prove that
Using polar coordinates and (17), we have
where is the surface area of a unit sphere embedded in
. We then have
which implies that we can choose a , such that for all
,
Lastly, we write
which implies that we can choose the same as above to obtain the bound
for any .
Thus, we obtain the bound , for all
, by combining (18), (19), (20), (21), (22), and (23), via the triangle inequality. The result is proved by combing the bound above, with (16), for an appropriately large
.
3. Comments and discussion
3.1. Relationship to Theorem 1
In the proof of Theorem 1, the famous Hilbert space approximation result of Jones (Citation1992) and Barron (Citation1993) was used to bound the norm between any approximand
and a convex combination of bounded functions in
. This approximation theorem is exactly the
case of the more general theorem of Donahue et al. (Citation1997), as presented in Lemma 11. Thus, one can view Theorem 5(c) as the
generalization of Theorem 1.
3.2. The class 
is a proper subset of the class 

Here, we comment on the nature of class , which was investigated by Bacharoglou, (Citation2010) and Nestoridis et al. (Citation2011). We recall that Bacharoglou (Citation2010) conjectured that Theorem 4 generalizes from
to
. In Theorem 5(a)–(e), we assume that
. We can demonstrate that
is a strictly weaker condition than
or
.
For example, consider the function in such that
if
and
and note that
Since ,
. Furthermore,
is continuous since all stationary points of
are continuous. In
,
if
For , we observe that
and thus the left limit is satisfied. On the right, for any
, we have
, so that
, for all
, where
is the ceiling operator. Therefore,
.
Within each interval , we observe that
is locally maximized at
. The local maximum corresponding to each of these points is
. Thus
, since
where . Furthermore,
since
.
3.3. Convergence in measure
Along with the conclusions of Theorem 5(d) and (e), Lemma 12 also implies convergence in measure. That is, if is a
Borel measure on
, then for any
, there exists a sequence
, such that for any
,
A Technical results
Throughout the main text, we utilize a number of established technical results. For the convenience of the reader, we append these results within this Appendix. Sources from which we draw the unproved results are provided at the end of the section.
Lemma 5. Let be a sequence of pdfs in
and for every
Then, for all and
,
Furthermore, for all and any compact
,
The sequences from Lemma 5 are often called approximate identities or approximations of the identity. A simple construction of approximate identities is by taking dilations
, which yields the following corollary.
Corollary 1. Let be a pdf. Then the sequence of dilations
, satisfies the hypothesis of Lemma 5 and hence permits its conclusion.
Lemma 6. The class is a subset of
. Furthermore, if
, then
is uniformly continuous.
Lemma 7 (Urysohn’s Lemma). If is compact, then there exists some
, such that
and
.
Lemma 8. If is bounded, then for any
,
can be covered by
for some finite
, where
and
.
Lemma 9. If , then
.
Let be the usual gamma function, defined as
.
Lemma 10. If and
, for
, then
exists and we have
.
Lemma 11. Let , for some
, and let
. For any
, such that
, for all
, there exists a
, such that
where , and
Lemma 12. In any measure , uniform convergence implies almost uniform convergence, and almost uniform convergence implies almost everywhere convergence and convergence in measure, with respect to
.
B Sources of results
Lemma 5 is reported as Theorem 9.3.3 in Makarov & Podkorytov (Citation2013) (see also Theorem 2 of Rudin, Citation1976, Ch. 20). The proof of Corollary 1 can be taken from that of Theorem 4 of Cheney & Light, Citation2000, Ch. 20. Lemma 6 appears in Conway, Citation2012), as Proposition 1.4.5. Lemma 7 is taken from Corollary 1.2.9 of Conway (Citation2012). Lemma 8 appears as Theorem 1.2.2 in Conway (Citation2012). Lemma 9 can be found in Folland (Citation1999), Prop. 6.10. Lemma 10 can be found in [21, Thm. 9.3.1]. Lemma 11 appears as Corollary 2.6 in Donahue et al. (Citation1997). Lemma 12 can be obtained from the definition of almost uniform convergence, Lemma 7.10, and Theorem 7.11 of Bartle (Citation1995).
Acknowledgements
HDN is personally funded by Australian Research Council (ARC) grant DE170101134. HDN and GJM are supported by ARC grant DP180101192. FC is supported by Agence Nationale de la Recherche (ANR) grant SMILES ANR-18-CE40-0014 and by Région Normandie grant RIN.
Additional information
Notes on contributors
Geoffrey J. McLachlan
Mr. Nguyen, Dr. Nguyen, and Profs. Chamroukhi and McLachlan are each keenly interested in the study of finite mixture models and their modifications and extensions for various data analytic and machine learning applications. Their research spans the fields of computational statistics, mathematical statistics, machine learning and AI. Common threads of their research consist of the derivation of algorithms for the efficient estimation of mixture models and extensions, such as mixtures of experts, and mixtures of factor analysers; the derivation of theoretical results regarding the statistical and mathematical properties of such constructions and their estimators; and the application of mixture models to data analytic problems spanning the fields of biology, medical science, engineering, signal processing, among many others.
References
- Bacharoglou, A. G. 2010. Approximation of probability distributions by convex mixtures of Gaussian measures. Proceedings of the American Mathematical Society, 138:2619–18.
- Barron, A. R. (1993). Universal approximation bound for superpositions of a sigmoidal function. IEEE Transactions on Information Theory, 39(3), 930–945. doi: 10.1109/18.256500
- Bartle, R. G. (1995). The Elements of Integration and Lebesgue Measure. Wiley.
- Cheney, W. & Light, W. (2000). A Course in Approximation Theory. Brooks/Cole.
- Conway, J. B. (2012). A Course in Abstract Analysis. American Mathematical Society.
- DasGupta, A. (2008). Asymptotic Theory Of Statistics And Probability. Springer.
- De Branges, L. 1959. The Stone-Weierstrass theorem. Proceedings of the American Mathematical Society, 10:822–824.
- Devroye, L. & Lugosi, G. (2000). Combinatorial Methods in Density Estimation. Springer.
- Donahue, M. J., Gurvits, L., Darken, C. & Sontag, E. (1997). Rates of convex approximation in non-Hilbert spaces. Constructive Approximation, 13(2), 187–220. https://doi.org/10.1007/BF02678464
- Everitt, B. S. & Hand, D. J. (1981). Finite Mixture Distributions. Chapman and Hall.
- Feichtinger, H. G. (1977). A characterization of wiener’s algebra on locally compact groups. Archiv der Mathematik, 29(1), 136–140. https://doi.org/10.1007/BF01220386
- Folland, G. B. (1999). Real Analysis: Modern Techniques and Their Applications. Wiley.
- Forbes, F. & Wraith, D. (2013). A new family of multivariate heavy-tailed distributions with variable marginal amounts of tailweights. Statistics and computing, 24(6), 971–984. https://doi.org/10.1007/s11222-013-9414-4
- Fruwirth-Schnatter, S. (2006). Finite Mixture and Markov Switching Models. Springer.
- Fruwirth-Schnatter, S., Celeux, G., & Robert, C. P. (editors). (2019). Handbook of Mixture Analysis. CRC Press.
- Jones, L. K. (1992). A simple lemma on greedy approximation in Hilbert space and convergence rates for projection pursuit regression and neural network training. Annals of statistics, 20(1), 608–613. https://doi.org/10.1214/aos/1176348546
- Kullback, S. & Leibler, R. A. (1951). On information and sufficiency. Annals of Mathematical Statistics, 22(1), 79–86. https://doi.org/10.1214/aoms/1177729694
- Lee, S. X. & McLachlan, G. J. (2016). Finite mixtures of canonical fundamental skew t-distributions: the unification of the restricted and unrestricted skew t-mixture models. Statistics and computing, 26(3), 573–589. https://doi.org/10.1007/s11222-015-9545-x
- Li, J. Q. & Barron, A. R. (1999). Mixture density estimation. In S. A. Solla, T. K. Leen, & K. R. Mueller (Eds.), Advances in neural information processing systems (Vol. 12, pp. 279–285). MIT Press.
- Lindsay, B. G. 1995. Mixture models: theory, geometry and applications. In NSF-CBMS Regional Conference Series in Probability and Statistics. Hayward.
- Makarov, B. & Podkorytov, A. (2013). Real Analysis: Measures, Integrals and Applications. Springer.
- McLachlan, G. J. & Basford, K. E. (1988). Mixture Models: Inference and Applications to Clustering. Marcel Dekker.
- McLachlan, G. J. & Peel, D. (2000). Finite Mixture Models. Wiley.
- Mengersen, K. L., Robert, C. & Titterington, M. (2011). Mixtures: Estimation and Applications. Wiley.
- Nestoridis, V. & Papadimitropoulos, C. (2005). Abstract theory of universal series and an application to Dirichlet series. Comptes Rendus Academy of Science Paris Series I, 341(9), 530–543. doi: 10.1016/j.crma.2005.09.028
- Nestoridis, V., Schmutzhard, S. & Stefanopoulos, V. (2011). Universal series induced by approximate identities and some relevant applications. Journal of Approximation Theory, 163(12), 1783–1797. https://doi.org/10.1016/j.jat.2011.06.001
- Nestoridis, V. & Stefanopoulos, V. 2007. Universal series and approximate identities. Technical report.
- Nguyen, H. D. & McLachlan, G. J. (2019). On approximations via convolution-defined mixture models. Communications in Statistics - Theory and Methods, 48(16), 3945–3955. In press. https://doi.org/10.1080/03610926.2018.1487069
- Peel, D. & McLachlan, G. J. (2000). Robust mixture modelling using the t distribution. Statistics and computing, 10(4), 339–348. https://doi.org/10.1023/A:1008981510081
- Rakhlin, A., Panchenko, D. & Mukherjee, S. (2005). Risk bounds for mixture density estimation. ESAIM: Probability and Statistics, 9, 220–229. https://doi.org/10.1051/ps:2005011
- Rudin, W. (1976). Principles of Mathematical Analysis. McGraw-Hill.
- Sandberg, I. W. (2001). Gaussian radial basis functions and inner product space. Circuits, Systems and Signal Processing, 20(6), 635–642. https://doi.org/10.1007/BF01270933
- Schlattmann, P. (2009). Medical Applications of Finite Mixture Models. Springer.
- Stone, M. H. 1948. The generalized Weierstrass approximation theorem. Mathematical Magazine, 21:237–254.
- Titterington, D. M., Smith, A. F. M. & Makov, U. E. (1985). Statistical Analysis of Finite Mixture Distributions. Wiley.
- van de Geer, S. (2003). Asymptotic theory for maximum likelihood in nonparametric mixture models. Computational Statistics and Data Analysis, 41(3–4), 453–464. https://doi.org/10.1016/S0167-9473(02)00188-3
- Walker, J. L., & Ben-Akiva, M. (2011). Advances in discrete choice: mixture models. In A. De Palma, R. Lindsey, E. Quinet, & R. Vickerman (Eds.), A Handbook of transport economics (pp. 160–187). Edward Edgar.
- Xu, Y., & Light, W. A. (1993). and E W Cheney. Constructive methods of approximation by ridge functions and radial functions. Numerical Algorithms, 4(2), 205–223. https://doi.org/10.1007/BF02144104
- Yona, G. (2011). Introduction to Computational Proteomics. CRC Press.
- Zeevi, A. J., & Meir, R. (1997). Density estimation through convex combinations of densities: approximation and estimation bounds. Neural computation, 10(1), 99–109. doi: 10.1016/S0893-6080(96)00037-8