Abstract
Background
Circulating eosinophils are associated with tumor development. An eosinophil-related index, the neutrophil to eosinophil ratio (NER), can be used to predict the prognosis of patients with tumors. However, there is still a lack of efficient prognostic biomarkers for HCC. In this study, we aimed to investigate the predictive value of the NER and develop an optimal machine learning model for the recurrence of HCC patients. Patients and methods: A retrospective collection of 562 patients who underwent hepatectomy with a pathologic diagnosis of HCC was performed. The relationship between NER and progression-free survival (PFS) was investigated. We developed a new machine learning framework with 10 machine learning algorithms and their 101 combinations to select the best model for predicting recurrence after hepatectomy. The performance of the model was assessed by the area under the curve (AUC) of characteristics and calibration curves, and clinical utility was evaluated by decision curve analysis (DCA).
Results
Kaplan‒Meier curves showed that the PFS in the low NER group was significantly better than that in the high NER group. Multivariate Cox regression analysis showed that NER was an independent risk factor for recurrence after surgery. The random survival forests (RSF) model was selected as the best model that had good predictive efficacy and outperformed the TNM, BCLC, and CNLC staging systems.
Conclusion
The NER has good predictive value for postoperative recurrence in patients with hepatocellular carcinoma. Machine learning model based on NER can be used for accurate predictions.
Introduction
Primary liver cancer is the sixth most common cancer and the third most common cause of cancer-related deaths worldwide.Citation1,Citation2 Hepatocellular carcinoma (HCC) accounts for 75–85% of primary liver cancers.Citation1 Currently, hepatectomy is still considered one of the most effective therapeutic approaches for the treatment of primary liver cancer.Citation3 However, due to the high recurrence rate of HCC, the long-term prognosis of patients with HCC after hepatectomy remains poor, with a 5-year recurrence rate of 60–70%.Citation4 Although HCC-related biomarkers and prognostic models have been initially applied in clinical practice, the results are still unsatisfactory. Therefore, the question of how to screen more valuable indicators and construct models is the focus of clinical research.
Many studies have shown that chronic inflammation plays a major role in cancer cell proliferation, angiogenesis, and immunosuppression, and cancer-related inflammation (CRI) is associated with cancer prognosis.Citation5 Serological indicators such as neutrophil counts and lymphocyte counts have been used for biomarker development in clinical studies because of their advantages in convenience and low cost.Citation6 Several inflammatory markers have been shown to predict the prognosis of patients with HCC, such as the neutrophil-to-lymphocyte ratio (NLR), platelet-to-lymphocyte ratio (PLR),Citation7 and lymphocyte-to-monocyte ratio (LMR).Citation8 However, their predictive ability for the prognosis of HCC patients after hepatectomy remains controversial. The eosinophil count has been increasingly used in tumor prognostic studies as a newly developed inflammatory marker.Citation9 A retrospective study of HCC patients treated with sorafenib found that lower peripheral blood eosinophil counts were associated with a poorer prognosis.Citation10 A lower eosinophil-to-neutrophil ratio (NER) was found to be associated with a better prognosis in a study of patients with metastatic renal cell carcinoma treated with natalizumab plus ibritumomab.Citation11 Nevertheless, there is still a lack of studies correlating NER with HCC prognosis.
Favorable results have been reported from studies applying machine learning models to HCC. It has been demonstrated that machine learning models outperform traditional regression models in predicting HCC development.Citation12 In this study, we aimed to analyze the relationship between preoperative NER and recurrence after hepatectomy in patients with HCC, seeking to discover whether NER could be used as a new prognostic marker for HCC. Machine learning algorithms were used to construct and screen the optimal model for recurrence after surgery.
Patients and Methods
Patient Selection
A total of 562 patients who received liver resection and were pathologically diagnosed with HCC at the Department of Hepatobiliary and Pancreatic Surgery, The Affiliated Hospital of Qingdao University, between January 2013 and September 2021 were retrospectively included. We eliminated patients with other malignancies, preoperative antitumor therapy, macrovascular invasion, and postoperative liver transplantation for nontumor liver disease as well as patients lacking complete baseline and follow-up data. We also eliminated patients suffering from allergic diseases, blood disorders, and other conditions that could lead to alterations in the leucocyte count. The study followed the ethical guidelines of the Declaration of Helsinki. Subjects or their immediate family members signed an informed consent form before the procedure agreeing to the use of tumor tissue and clinical information for this study.
Clinicopathological Variables
Baseline characteristics of the patients, including date of surgery, sex, age, HBsAg (Hepatitis B surface antigen), alpha-fetoprotein (AFP), albumin (Alb), Total bilirubin (TBIL), direct bilirubin (DBIL), Indirect Bilirubin (IBIL), alanine aminotransferase (ALT), aspartate aminotransferase (AST), gamma glutamyl transferase (GGT), alkaline phosphatase (ALP), hemoglobin count (HB), platelet count (PLT), and peripheral blood cell counts, were obtained to calculate systemic inflammatory parameters, including NER, NLR, PLR, and LMR. Tumor characteristics included the largest tumor diameter, tumor number, cirrhosis, microvascular invasion, capsular invasion, tumor satellite nodules, and tumor differentiation.
Postoperative Follow-Up
All patients were discharged from the hospital and followed up regularly in the outpatient clinic. Follow-up was every 3 months for the first 2 years postoperatively and every 3–6 months thereafter. Dynamic contrast-enhanced computed tomography (CT) or gd- EOB- DTPA-enhanced magnetic resonance imaging (MRI) of the upper abdomen was performed if digestive ultrasound showed HCC recurrence and/or if there was substantially elevated alpha-fetoprotein (AFP). Furthermore, ancillary investigations included CT of the chest, CT of the lower abdomen, CT of the pelvis, or positron emission tomography (PET)-CT to be completed when clinically indicated. The diagnosis of tumor recurrence was confirmed by at least two imaging studies or liver histopathology. Once tumor recurrence was detected, surgical resection, local ablation, TACE, radiotherapy, systemic therapy, and supportive care could be selected based on the characteristics of tumor recurrence. Survival information was obtained through clinical follow-up or telephone follow-up. Follow-up surveys were conducted until September 2021. The start of follow-up was the date of surgery; the time interval from the start point to the first recurrence, first metastasis, death, or last follow-up was PFS.
Statistical Analysis
Serum alpha-fetoprotein was used as a cutoff value of 400 ng/mL, and the cutoff values of the rest of the continuous variables were determined using X-tile 3.6.1 software (Yale University, New Haven, CT, USA). The patients were assigned to two groups (high and low groups) based on the optimal cutoff value. The Mann–Whitney U-test was used to compare continuous variables. Categorical variables were compared using the χ2 test or Fisher test. Using the Cox proportional risk model, we determined the independent risk factors associated with postoperative progression or death and gave the risk ratio (HR) and 95% confidence interval (CI). We used 10 machine learning algorithms and 101 combinations of algorithms. The integrative algorithms included random survival forest (RSF), elastic network (Enet), Lasso, Ridge, stepwise Cox, CoxBoost, partial least squares regression for Cox (plsRcox), supervised principal components (SuperPC), generalized boosted regression modeling (GBM), and survival support vector machine (survival-SVM). For each model, Harrell’s consistency index (C-index) was calculated for both the training and validation sets, and the model with the highest average C-index was considered the optimal model. The study was statistically analyzed using SPSS software version 26.0 (SPSS, Chicago, IL, USA) and R language software version 4.2.2. The results were considered statistically significant when the P value was less than 0.05.
Results
The Relationship Between Clinicopathological Characteristics and NER
As shown in , there were 469 male and 93 female patients, and the average age was 54.8 years old (range, 14–82 years). The optimal cutoff value of 102.00 was obtained for NER using X-tile 3.6.1 software. High NER (>102.00) ratios were observed in 68 (12.10%) patients.
Table 1 Baseline Patient Clinicopathological Characteristics
Tumor diameters tended to be larger in patients with high NER than in those with low NER (P=0.009). There were also differences in serum albumin levels between the two groups of patients (P=0.034), but there was no significant difference between the two groups in terms of other baseline characteristics. Complete Baseline patient clinicopathological characteristics are in Supplementary Table 1.
Cox Regression Analyses Between Clinicopathological Variables Associated with PFS After Surgery
As shown in , univariate and multivariate analyses using the Cox proportional hazards model were performed. Age (P=0.011), NER (P=0.002), GGT (P=0.006), largest tumor size (P=0.003), tumor number (P=0.003), microvascular invasion (P=0.019), satellite nodules (P=0.035), BCLC (P<0.001) and TNM (P=0.020) were significantly correlated with patient PFS. The complete Cox regression results are in Supplementary Table 2.
Table 2 Univariate and Multivariate Cox Regression Analyses of Risk Factors Associated with Progression-Free Survival (PFS) After Surgery in the Total Cohort
PFS Analysis
Until the end of follow-up, no patients were lost to follow-up, the median follow-up of all study patients was 61.0 months, and the median PFS of the patients was 23.0 months. Based on the results of Cox regression, we plotted the KM curves of high and low NER patients in different subgroups of patients. Microvascular invasion, largest tumor size and tumor number were significant variables in multivariate Cox regression. AFP is currently the most commonly used serum tumor marker in the diagnosis and recurrence monitoring of liver cancer. The Kaplan‒Meier curves in show that patients in the low NER group had better PFS than those in the high NER group (P<0.0001). Among patients with AFP≤400 and negative microvascular invasion, largest tumor size ≤4.5 cm, and a single tumor, those in the low NER group had a higher PFS than those in the high NER group. The receiver operating characteristic (ROC) curve showed that the area under the curve for the NER was similar to the NLR and superior to the PLR and LMR (Supplementary Figure 1).
Figure 1 Kaplan–Meier curves of PFS for patients with different NER groups. (A) Kaplan–Meier curves of PFS for patients with different NER groups in the total cohort. (B–E) Kaplan-Meier curves of PFS in different NER groups in patients negative for microvascular invasion (B), alpha-fetoprotein (AFP) ≤400 (C), Tumor diameter ≤4.5cm (D), single tumor (E).
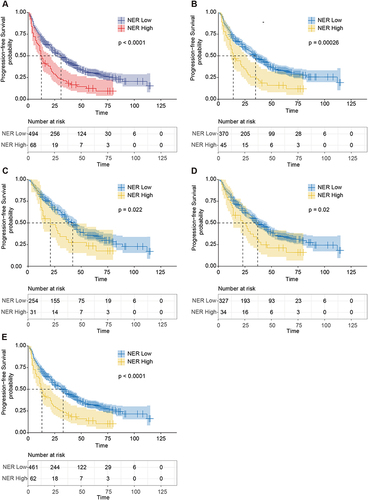
Development and Assessment of the Machine Learning Model
As shown in , all HCC patients were randomized into the training cohort (n=393) and validation cohort (n=169) at a ratio of 3:1. The complete clinicopathologic characteristics comparison between the two cohorts is in Supplementary Table 3. In the training cohort, we built a machine learning framework containing 10 machine learning algorithms and 101 combinations of them and computed the average C-index of each model in both cohorts to evaluate the predictive ability of the models (). The best model was the random survival forests model with the highest average C-index (0. 745). The error rate of the model stabilized when the ntree was 650 (). The C-index was 0.808 for the training set, and the C-index of the validation set was 0.682. According to the ranking of the importance of variables by the VIMP method in , NER was a relatively important metric.
Table 3 The Clinicopathologic Characteristics of Patients in the Training and Validation Cohorts
Figure 2 The computational framework for machine learning algorithms. (A) A combination of 101 machine learning algorithms was generated by synthesizing the computational framework. The C-index of each model was calculated through the training and validation cohorts and sorted by the average C-index. (B) Prediction error rates. (C) Ranking of variable importance (VIMP) of features. (D–E) ROC curve and AUC of the RSF model in the training cohort (D) and validation cohort (E).
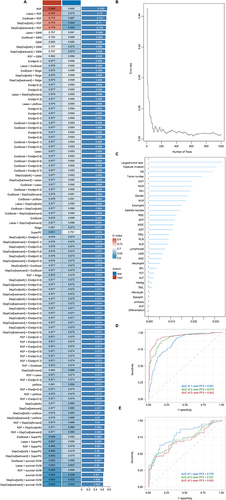
The AUCs of 1-, 2-, and 3-year PFS were 0.851, 0.919, and 0.942, respectively, in the training cohort and 0.779, 0.721, and 0.693, respectively, in the validation cohort (). The calibration curves had good prediction consistency, and the DCA curves of the model also indicated that the model had a good net benefit ().
Figure 3 The calibration curves and the DCA curves of the model. (A and B) The calibration curves in the training cohort (A) and validation cohort (B). (C–H) The DCA curves in the training cohort (C–E) and validation cohort (F–H).
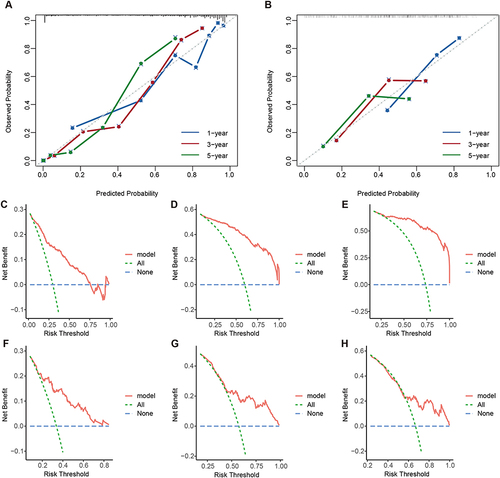
The model was compared with other widely used staging models (including the BCLC, TNM, and CNLC staging systems) using ROC curves in the total cohort. The 1-, 3-, and 5-year AUCs of the current model (0.830, 0.865, 0.862) were higher than those of TNM staging (0.652, 0.609, and 0.601), BCLC (0.645, 0.593, and 0.589), and CNLC (0.573, 0.540, and 0.531), respectively, and the predictive effectiveness of the above three models was not as good as that of the model. The comparison between different models indicates that this model had good predictive ability ().
Figure 4 Receiver operating characteristic (ROC) curves and Kaplan–Meier curves of the model. (A–D) ROC curve and AUC of the RSF model (A), TNM staging system (B), BCLC staging system (C), and CNLC staging system (D) in the total cohort. (E–G) Kaplan–Meier curve of PFS in the total cohort (E), training cohort (F), and validation cohort (G).
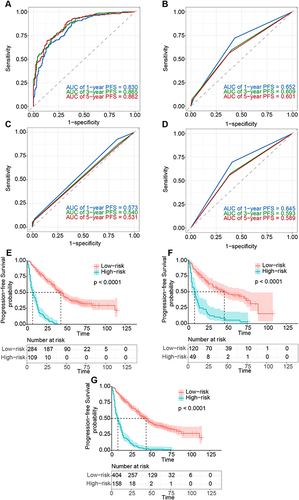
Risk Stratification
The scores for recurrence risk were calculated based on the model, and the optimal cutoff point (59.53) was automatically calculated by X-tile software. Patients were categorized into the low-risk group (≤59.53) and the high-risk group (>59.53), and survival curves were calculated to compare the PFS in the two different risk groups. The results showed that recurrence risk stratification had a good ability to identify patients for recurrence ().
Discussion
In this study, we used NER for the first time to predict postoperative recurrence in patients with hepatocellular carcinoma. Kaplan‒Meier curves showed that the PFS in the low NER group was significantly better than that in the high NER group. Multivariate Cox regression analysis showed that NER was an independent risk factor for recurrence after surgery. In the subgroup analyses of the total cohort of patients, such as for patients with AFP≤400, negative microvascular invasion, largest tumor size ≤4.5, and single tumor, lower NER was still associated with a better prognosis. Then, we built a model with the machine learning framework, which had good predictive efficacy and outperformed the TNM, BCLC, and CNLC staging systems.
An association between inflammation-based markers such as NLR and PLR and the prognosis of hepatocellular carcinoma has been demonstrated.Citation13,Citation14 However, the effects of current biomarkers and clinical models remain inconsistent. With the development of HCC precision treatment, there is an urgent need for more accurate predictive indices and models to guide the prognosis of HCC. Circulating neutrophils are thought to drive tumor progression through immunosuppression and direct enhancement of tumor cell survival.Citation15 Eosinophils are circulating leukocytes and are commonly associated with host immune and prognostic inflammatory responses to parasites.Citation16 However, recent studies have found that eosinophils also play a role in tumor development.Citation17,Citation18 Meanwhile, high baseline eosinophil counts were all significantly associated with better prognosis in metastatic renal cell carcinoma,Citation19 metastatic melanoma,Citation20 and non-small cell lung cancer.Citation21 The relationship between eosinophils and HCC is still undetermined, but some studies have suggested that eosinophils may play an antitumor role in hepatobiliary carcinoma.Citation22 Furthermore, a study found that IL-5-activated eosinophils inhibited the growth of HCC cells in vitro through eosinophil-mediated direct cytotoxicity.Citation23 Eosinophils can be recruited in the tumor region,Citation9 and a recent study found that eosinophils can be used to differentiate between alpha protein-negative HCC and cirrhotic patients.Citation24 As an eosinophil-related indicator, lower NER has been shown to correlate with improved prognosis in immunotherapy for melanoma and urothelial carcinoma (UC).Citation25,Citation26 In our study, NER was an independent risk factor for PFS and one of the most important factors in the recurrence model; this relationship has not been mentioned in other studies of HCC markers. Meanwhile, AFP, tumor diameter, MVI, and number of tumors were also correlated with the prognosis of the tumor, which is similar to the results obtained from existing studies.Citation27
Numerous studies have confirmed that patients with lower AFP have a better prognosis.Citation28 In our subgroup analysis, we found that among patients with AFP≤400 ng/mL, patients with NER >102.00 were more likely to experience recurrence. Similarly, in the subgroup of patients without vascular invasion, with the largest tumor diameter ≤4.5 cm, and with a solitary tumor, the prognosis of patients with NER >102.00 was also poor. In the clinical follow-up and treatment of this group of patients, close follow-up was needed. As a convenient, accessible, low-cost, and reliable biomarker, NER has the potential to become a novel marker for predicting the prognosis of hepatocellular carcinoma that deserves further exploration in subsequent studies.
Many studies have shown that machine learning plays an important role in research related to the medical field, and machine learning (ML) is increasingly used for clinical decision-making, disease diagnosis, and predicting patient prognosis.Citation29 Recurrence models for HCC patients using machine learning methods such as LASSO regression and random forests have better predictive efficacy.Citation30 In this study, the best model chosen by comparing 101 combinations of algorithms exhibits better predictive ability and avoids the limitations of inadequate algorithms. However, this study was not without limitations. We only performed internal validation; external validation in multicenter studies is still necessary.
Conclusion
This study confirms the good predictive value of NER for postoperative recurrence in patients with hepatocellular carcinoma. Lower NER was still associated with a better prognosis. Machine learning models based on NER can provide more accurate predictions.
Ethics Approval and Consent to Participate
The protocol of this study was approved by the ethical review board of Qingdao University (No. QYFY WZLL 28291).
Consent for Publication
Written informed consent for publication was obtained from all participants.
Author Contributions
All authors made a significant contribution to the work reported, whether that is in the conception, study design, execution, acquisition of data, analysis, and interpretation, or all these areas; took part in drafting, revising, or critically reviewing the article; gave final approval of the version to be published; have agreed on the journal to which the article has been submitted; and agree to be accountable for all aspects of the work.
Disclosure
The author(s) report no conflicts of interest in this work.
Acknowledgments
Thank all the staff authors for their contributions to this study.
Data Sharing Statement
The datasets used during the present study are available from the corresponding author, Dr. Peng Sun (email: [email protected]), upon reasonable request.
Additional information
Funding
References
- Sung H, Ferlay J, Siegel RL, et al. Global cancer statistics 2020: GLOBOCAN estimates of incidence and mortality worldwide for 36 cancers in 185 countries. CA Cancer J Clin. 2021;71(3):209–249. doi:10.3322/caac.21660
- Bray F, Ferlay J, Soerjomataram I, Siegel RL, Torre LA, Jemal A. Global cancer statistics 2018: GLOBOCAN estimates of incidence and mortality worldwide for 36 cancers in 185 countries. CA Cancer J Clin. 2018;68(6):394–424. doi:10.3322/caac.21492
- Zhang X, Li C, Wen T, et al. Appropriate treatment strategies for intrahepatic recurrence after curative resection of hepatocellular carcinoma initially within the Milan criteria: according to the recurrence pattern. Eur J Gastroenterol Hepatol. 2015;27(8):933–940. doi:10.1097/MEG.0000000000000383
- Imamura H, Matsuyama Y, Tanaka E, et al. Risk factors contributing to early and late phase intrahepatic recurrence of hepatocellular carcinoma after hepatectomy. J Hepatol. 2003;38(2):200–207. doi:10.1016/S0168-8278(02)00360-4
- Elinav E, Nowarski R, Thaiss CA, Hu B, Jin C, Flavell RA. Inflammation-induced cancer: crosstalk between tumours, immune cells and microorganisms. Nat Rev Cancer. 2013;13(11):759–771. doi:10.1038/nrc3611
- Chan SL, Wong LL, Chan KA, et al. Development of a novel inflammation-based index for Hepatocellular carcinoma. Liver Cancer. 2020;9(2):167–181. doi:10.1159/000504252
- Wang D, Bai N, Hu X, et al. Preoperative inflammatory markers of NLR and PLR as indicators of poor prognosis in resectable HCC. PeerJ. 2019;7:e7132. doi:10.7717/peerj.7132
- Yang T, Zhu J, Zhao L, et al. Lymphocyte to monocyte ratio and neutrophil to lymphocyte ratio are superior inflammation-based predictors of recurrence in patients with hepatocellular carcinoma after hepatic resection. J Surg Oncol. 2017;115(6):718–728. doi:10.1002/jso.24549
- Varricchi G, Galdiero MR, Lofredo S, et al. Eosinophils: the unsung heroes in cancer? Oncoimmunology. 2017;7(2):e1393134. doi:10.1080/2162402X.2017.1393134
- Orsi G, Tovoli F, Dadduzio V, et al. Prognostic role of blood Eosinophil count in patients with sorafenib-treated Hepatocellular carcinoma. Target Oncol. 2020;15(6):773–785. doi:10.1007/s11523-020-00757-3
- Tucker MD, Brown LC, Chen YW, et al. Association of baseline neutrophil-to-eosinophil ratio with response to nivolumab plus ipilimumab in patients with metastatic renal cell carcinoma. Biomark Res. 2021;9(1):80. doi:10.1186/s40364-021-00334-4
- Singal AG, Mukherjee A, Elmunzer BJ, et al. Machine learning algorithms outperform conventional regression models in predicting development of hepatocellular carcinoma. Am J Gastroenterol. 2013;108(11):1723–1730. doi:10.1038/ajg.2013.332
- Mao S, Yu X, Sun J, et al. Development of nomogram models of inflammatory markers based on clinical database to predict prognosis for hepatocellular carcinoma after surgical resection. BMC Cancer. 2022;22(1):249. doi:10.1186/s12885-022-09345-2
- Yang J, Bao Y, Chen W, Duan Y, Sun D. Nomogram based on systemic immune inflammation index and prognostic nutrition index predicts recurrence of Hepatocellular carcinoma after surgery. Front Oncol. 2020;10:551668. doi:10.3389/fonc.2020.551668
- Xiong S, Dong L, Cheng L. Neutrophils in cancer carcinogenesis and metastasis. J Hematol Oncol. 2021;14(1):173. doi:10.1186/s13045-021-01187-y
- Klion AD, Ackerman SJ, Bochner BS. Contributions of eosinophils to human health and disease. Annu Rev Pathol. 2020;15(1):179–209. doi:10.1146/annurev-pathmechdis-012419-032756
- Carretero R, Sektioglu IM, Garbi N, Salgado OC, Beckhove P, Hämmerling GJ. Eosinophils orchestrate cancer rejection by normalizing tumor vessels and enhancing infiltration of CD8(+) T cells. Nat Immunol. 2015;16(6):609–617. doi:10.1038/ni.3159
- Davis BP, Rothenberg ME. Eosinophils and cancer. Cancer Immunol Res. 2014;2(1):1–8. doi:10.1158/2326-6066.CIR-13-0196
- Zahoor H, Barata PC, Jia X, et al. Patterns, predictors and subsequent outcomes of disease progression in metastatic renal cell carcinoma patients treated with nivolumab. J Immunother Cancer. 2018;6(1):107. doi:10.1186/s40425-018-0425-8
- Rosner S, Kwong E, Shoushtari AN, et al. Peripheral blood clinical laboratory variables associated with outcomes following combination nivolumab and ipilimumab immunotherapy in melanoma. Cancer Med. 2018;7(3):690–697. doi:10.1002/cam4.1356
- Tanizaki J, Haratani K, Hayashi H, et al. Peripheral blood biomarkers associated with clinical outcome in non-small cell lung cancer patients treated with nivolumab. J Thorac Oncol. 2018;13(1):97–105. doi:10.1016/j.jtho.2017.10.030
- Steel JL, Kim KH, Dew MA, et al. Cancer-related symptom clusters, eosinophils, and survival in hepatobiliary cancer: an exploratory study. J Pain Symptom Manage. 2010;39(5):97–105. doi:10.1016/j.jpainsymman.2009.09.019
- Kataoka S, Konishi Y, Nishio Y, Fujikawa-Adachi K, Tominaga A. Antitumor activity of eosinophils activated by IL-5 and eotaxin against hepatocellular carcinoma. DNA Cell Biol. 2004;23(9):549–560. doi:10.1089/dna.2004.23.549
- Wu Y, Liu S, Fan Z, Tian Y, Zhang L, Liu S. Establishment and validation of a blood test-based nomogram to diagnose patients with AFP-negative HCC. Curr Cancer Drug Targets. 2024;24(5):556–564. doi:10.2174/0115680096264770231113103930
- Pozorski V, Park Y, Mohamoud Y, et al. Neutrophil-to-eosinophil ratio as a biomarker for clinical outcomes in advanced stage melanoma patients treated with anti-PD-1 therapy. Pigm Cell Melanoma Res. 2023;36(6):501–511. doi:10.1111/pcmr.13109
- Furubayashi N, Minato A, Negishi T, et al. The association of clinical outcomes with posttreatment changes in the relative Eosinophil counts and neutrophil-to-Eosinophil ratio in patients with advanced urothelial carcinoma treated with pembrolizumab. Cancer Manag Res. 2021;13:8049–8056. doi:10.2147/CMAR.S333823
- Liang L, Li C, Wang MD, et al. Development and validation of a novel online calculator for estimating survival benefit of adjuvant transcatheter arterial chemoembolization in patients undergoing surgery for hepatocellular carcinoma. J Hematol Oncol. 2021;14(1):165. doi:10.1186/s13045-021-01180-5
- Ma WJ, Wang HY, Teng LS. Correlation analysis of preoperative serum alpha-fetoprotein (AFP) level and prognosis of hepatocellular carcinoma (HCC) after hepatectomy. World J Surg Oncol. 2013;11:212. doi:10.1186/1477-7819-11-212
- Swanson K, Wu E, Zhang A, Alizadeh AA, Zou J. From patterns to patients: advances in clinical machine learning for cancer diagnosis, prognosis, and treatment. Cell. 2023;186(8):1772–1791. doi:10.1016/j.cell.2023.01.035
- Zeng J, Zeng J, Lin K, et al. Development of a machine learning model to predict early recurrence for hepatocellular carcinoma after curative resection. Hepatobiliary Surg Nutr. 2022;11(2):176–187. doi:10.21037/hbsn-20-466