Abstract
QSAR analysis of a series of previously synthesised 1-(1H-1,2,4-triazole-1-yl)-2-(2,4-difluorophenyl)-3-substituted-2-propanols(TDFPP) as analogues of fluconazole were tested for growth inhibitory activity against Candida albicans using computer assisted multiple regression analysis. This was in order to explore the selectivity requirements for fungicidal activity against C. albicans among these congeners. A training set comprising 40 analogues and a test set comprising ten analogues of 1-(1H-1, 2, 4-triazole-1-yl)-2-(2,4-difluorophenyl)-3-substituted-2-propanols were selected for the present investigation by using the sphere exclusion method embedded in the Vlife MDS 3.5 software. With respect to the modelling of the growth inhibitory activity of the reported compounds, the regression analysis shows that even in the mono-parametric correlations the topological and physicochemical parameters give significant regression coefficients. The validation of the QSAR models was performed by cross-validation and external test set prediction. The model is not only able to predict the activity of new compounds but also explains the important region in the molecules in a quantitative manner.
Introduction
In recent years, it has been observed that life threatening systemic fungal infections have become increasingly common, especially in the immunocompromised hosts suffering from tuberculosis, cancer or AIDS and in organ transplant cases [Citation1,Citation2]. Candidiasis caused by a group of microscopic fungi or yeast, the most common being Candida albicans. Current research can explain the frequency with which unexplained oral candidiasis has led to unequivocal acquired immunodeficiency syndrome (AIDS) in patients at risk. Twenty-two previously healthy adults with unexplained oral candidiasis (19 of those tested had a reversed T4/T8 ratio and 20 had generalised lymphadenopathy) were compared with 20 similar patients with a reversed T4/T8 ratio and generalised lymphadenopathy that did not have oral candidiasis [Citation3]. Moreover Candida is the leading cause of oesophagitis (throat inflammation) in people with AIDS. Such a causative agent requires an equally effective focus of research.
Quantitative structure–activity relationship (QSAR) studies are undoubtedly of great importance in modern chemistry and biochemistry. Ideally, the activities and properties are connected by some known mathematical function, F where biological activity = F [structure (in the present study topological and physicochemical descriptors are used as the structural parameters)]. A 2D-QSAR study makes it simple to interpret the biological data in terms of different descriptors obtained from the two dimensional structures of the compounds without involving energy minimization procedures. In a congeneric series, where a relative study is being carried out, the 2D-descriptors may play an important role in deriving significant correlations with the biological activities of the compounds. Thus the novelty and importance of a 2D-QSAR study is mainly due to its simplicity of the calculations of different descriptors and their interpretation (in a physical sense) to explain the inhibition actions of compounds in a congeneric series.
1, 2, 4-Triazole and its derivatives represent one of the most biologically active classes of compounds, possessing a wide spectrum of activities. The 1, 2, 4-triazole derivatives have been reported to have diverse pharmacological activities such as antibacterial, antifungal, hypoglycaemic, antihypertensive and analgesic properties [Citation4–6]. Triazoles have been used widely and efficiently as antifungal agents. These antifungal drugs act by inhibiting CYP51, a necessary enzyme in the biosynthesis of ergosterol, through a mechanism in which the heterocyclic nitrogen atom (N-4 of triazole) binds to the haem iron atom. However, the increasing administration of antifungal agents has led to the development of fungal resistance. The design of new TDFPP derivatives requires a more detailed knowledge of the mechanism of CYP51 inhibition by this class of compounds. Hence in the present study, a 2D-quantitaive SAR (2D-QSAR) study on these analogues (, , , and ) along with their generalized structures ( and ) has been conducted to provide the rationale for drug design using Vlife Molecular Design suite 3.5 (Baghpat, U.P). This software offers several hundred descriptors from different perspectives relating to the physico-chemical, topological and geographical characteristics to the molecules under a multi-descriptor class environment.
Table 1. Observed and modelled fungicidal action of 1-(1H-1,2,4-triazole-1-yl)-2-(2,4-difluorophenyl)-3-substituted-2-propanols against Candida albican (Refer to ).
Table 2. Observed and modelled fungicidal action of 1-(1H-1,2,4-triazole-1-yl)-2-(2,4-difluorophenyl)-3-substituted-2-propanols against Candida albicans (Refer to ).
Table 3. Observed and modelled fungicidal action of 1-(1H-1,2,4-triazole-1-yl)-2-(2,4-difluorophenyl)-3-substituted-2-propanols against Candida albicans (Refer to ).
Table 4. Observed and modelled fungicidal action of 1-(1H-1,2,4-triazole-1-yl)-2-(2,4-difluorophenyl)-3-substituted-2-propanols against Candida albicans (Refer to ).
Table 5. Observed and modelled fungicidal action of 1-(1H-1,2,4-triazole-1-yl)-2-(2,4-difluorophenyl)-3-substituted-2-propanols against Candida albicans (Refer to ).
Material and methods
Data set
The compound derived from1-(1H-1,2,4-triazole-1-yl)-2-(2,4-difluorophenyl)-3-substituted-2-propanol moiety (, , , and together with and ) was selected from the literature[Citation7,Citation8] along with their in vitro antifungal activity against Candida albicans. The activity expressed as minimum inhibitory concentration (MIC80), was defined as the first well with an approximate 80% reduction in growth compared to the growth of the drug-free well and is expressed as logMIC80 on a micromolar basis to improve the normal distribution of the experimental data points. Two dimensional quantitative structure–activity relationship studies of these 1, 2, 4-triazole derivatives were carried out by using the Vlife Molecular Design Suite 3.5. (Vlife Sciences Technologies, India) [Citation9–11]. The structures of the compounds under study have been drawn in the 2D drawing application (2D Draw app) of MDS 3.5 using the standard procedure. These structures were converted into 3D objects by using the default conversion procedure implemented in the MDS 3.5 software. The generated 3D structures of the compounds were subjected to batch energy minimization in the force field module using Merck Molecular Force Field (MMFF) and MMFF charge followed by considering distance dependent dielectric constant of one and convergence criteria of 0.01 kcal/mol. This ensures a well defined conformer relationship across the compounds of the study. All the energy minimized structures of the respective compounds were ported to the 2D-QSAR of QSARPLUS module (MDS 3.5) for computing the parameters corresponding to the physico-chemical [Individual, Chi, Chiv, Path count, Chi Chain, Chiv Chain, Chain path count, Cluster, Path cluster, Kappa, Element count, Polar surface area] and alignment independent topological [Attributes- 2,T, C, N, O, F, S, Cl] descriptor classes. As the total number of the descriptors involved in this study is very large (around 278) after removal of invariable descriptors, only the name of the descriptor classes and the actual descriptor involved in the model have been listed. The multiple linear regression model with stepwise forward-backward variable selection (MLR-SFB) procedure used in developing QSAR models is briefly described below.
Model development
Vlife MDS 3.5 is very robust software. The model used here for the QSAR equation generation was MLR-SFB. MLR-SFB is a “filter” based variable selection procedure. It is a procedure to examine the impact of each variable step by step. An important task in building a QSAR equation is to evaluate the required descriptors for the molecules under consideration and the Vlife MDS worksheet was provided for this purpose. LogMIC80 was selected as a dependent variable as its value depends on various descriptors whereas the physico-chemical and topological descriptors were selected as independent variables. The sphere exclusion procedure was adopted as the training data set selection method (dissimilarity value filter set by 1.3, +ve ) and selected the following: TCA1c, TCA1m, TCA2f, TCA3c, TCA3e, TCA4d, TCA5e, TCA5k, TCA5n, TCA6b as the test set molecules. The dissimilarity value gives the sphere exclusion radius and ensures that points in both the sets are uniformly distributed with respect to chemical and biological space. For the generation of QSAR models using 2D descriptors, the procedure employed was a stepwise forward-backward strategy with multiple linear regression which resulted in the selection of a subset of regressions for the extraction of the diverse structure–activity models. The “filters” set in MLR-SFB are set at 1) cross-correlation limit as 0.9, 2) F test in [4.0] and out [3.99], 3) number of variables in the final equation [2 to 10], 4) r2 as term selection criteria, 5) 0.0 as variance cut off with autoscaling, 6) number of random iterations as 100, 7) number of groups for cross validation [40- default acceptable value]. All these filters make the variable selection process efficient and lead to a unique solution. After the completion of this process, the fitness plot for training and test set were viewed and the contribution of the individual descriptor for activity was examined. The MLR-SFB protocol has been applied with default filter thresholds to identify all possible models that could emerge from the descriptors of the compounds.
Results
In the current study, a QSAR model is presented for LogMIC80 of fifty 1,2,4-triazole antifungal agents involving theoretical descriptors, which have been calculated from the molecular structure. For the selection of the most important descriptors, the aforementioned stepwise forward-backward multiple linear regression technique was used. A graphical presentation of the relationship between the experimental and predicted LogMIC80 values of 1,2,4-triazoles is shown in . The predicted property was LogMIC80 expressed in μM.
Figure 3. Graphical plot of experimental versus logMIC80 according to the model in Equation 2.
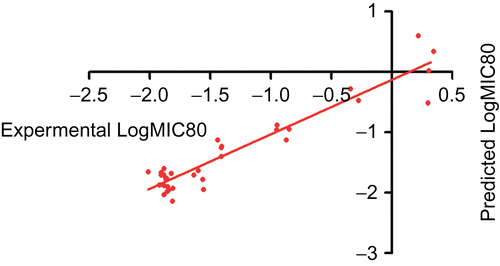
An explanation of the descriptors involved in the multiple linear regressions can be found at the end of the paper. A graphical presentation of the plot between the descriptors and correlation coefficient is shown in . As represented by the graph, R2 is directly proportional to the number of descriptors used. At the same time the N/5 rule should be adhered to. As our data set comprises of only 50 molecules, the number of descriptors should not exceed by 5.
The following regression equations represent the collective class structure activity model of the compounds, together with the statistical parameters of the regression.
N = 40, r2 = 0.8766, q2 = 0.7876, F test = 48.3096, pred_r2 = 0.6896
N = 40, r2 = 0.8533, q2 = 0.7989, F test = 50.8824, pred_r2 = 0.6831
N = 40, r2 = 0.7920, q2 = 0.7282, F test = 45.7013, pred_r2 = 0.6656
N = 40, r2 = 0.6651, q2 = 0.5995, F test = 36.7408, pred_r2 = 0.6482
Figure 5. Graphical plot of the observed MIC80 versus residual value according to the model in Equation 2.
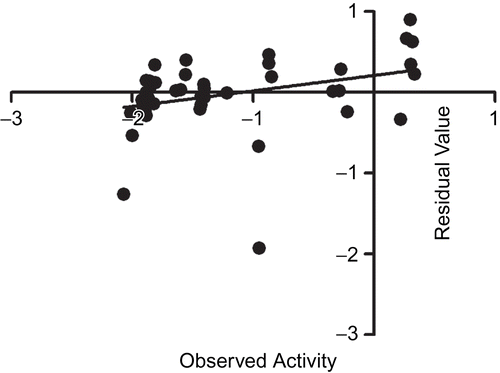
As can be observed from these equations, by increasing number of descriptors in the model, the r2 value increases significantly; however, further additions after four descriptors did not lead to any significant improvement in the r2 value.
Based on the training set of 40 molecules, the best correlation with the minimum descriptors was between the logMIC80 calculated and the logMIC80 experimental obtained using Equation 2. From the statistical point of view, this is a robust model. Statistical parameters for this equation were as follows: N = 40, r2 = 0.8533, q2 = 0.7989, F test = 50.8824, pred_r2 = 0.6831, alpha rand r2 = 0.00000, alpha rand q2 = 0.00000, alpha rand pred r2 = 0.01000. Cross-validation (q2) was performed on the training group (40) and showed that 79.89% of the 40 compounds i.e. 32 were correctly classified.
Discussion
The main objective of this study was to build a QSAR model which enables us to identify antifungal compounds from molecular databases using stepwise forward-backward MLR.
From the database of 50 antifungal compounds, we have obtained four stepwise forward-backward MLR equations capable of separating compounds according to their biological activity against Candida albicans and an MLR equation which enables a correlation between the MIC80 and chemical structure of each drug. Of the compounds 85.33% were correctly classified by r2 in the training set. The overlapping between the groups was small, which guarantees the quality of the selected discriminant function.
All these results confirm the effectiveness of the topological and physicochemical model proposed here for the search and selection of new potential antifungal drugs against Candida albicans. In this work we presented a valid model for the prediction of the antifungal activity of potential molecules against Candida albicans.
Conclusion
Our results lead to the conclusion that the fungicidal activity of TDFPP against Candida albicans can be successfully modelled with physicochemical and topological descriptors. The separation of the data into two independent sets (training and test) shows that our MLR model can predict external data with great accuracy. The proposed method is a useful aid to the costly and time-consuming experiments for determining fungicidal activity as the method has a high predictive ability. The proposed method can be used to screen existing databases or virtual libraries in order to identify novel potent compounds.
Descriptors
K3alpha: This descriptor signifies the third alpha modified shape index: (n-1) (n-3)2/p32 for odd no. and (n-3)(n-2)2/p32 for even no. where s = n+ a [Citation12].
T_N_O_3: This is the count of number of nitrogen atoms (single, double or triple bonded) separated from any oxygen atom (single, double, triple bonded) by 3 bonds in a molecule[Citation13].
T_N_Cl_6: This is the count of number of nitrogen atoms (single, double or triple bonded) separated from any chlorine atom (single, double, triple bonded) by 6 bonds in a molecule[Citation13].
T_C_F_5: This is the count of number of carbon atoms (single, double or triple bonded) separated from any fluorine atom (single, double, triple bonded) by 5 bonds in a molecule[Citation13].
T_O_O_4: This is the count of number of oxygen atoms (single, double or triple bonded) separated from any other oxygen atom (single, double, triple bonded) by 4 bonds in a molecule[Citation13].
Evaluation of the model
N, number of molecules
R2, coefficient of determination (>0.7)
Q2, cross-validated r2(>0.5)
Pred_r2, predicted r2 for external test(>0.5)
Alpha, statistical significance parameter by randomization test(<0.01)
F-test, F-test for statistical significance of the model (the higher the better, for the same set of descriptors of compounds)
Acknowledgements
The authors would like to thank Vlife Company for providing such user friendly software. The authors would also like to thank Xiaoyun Chai et al, Takuya Uchida and colleagues for providing the study literature.
Declaration of interest
The authors would like to thank Manipal University for funding of the software. The authors have no conflicting financial interests.
References
- Fridkin SK, Jarvis WR. Epidemiology of nosocomial fungal infections. Clin Microbiol Rev 1996;9:499–511.
- Wingard JR, Leather H. A new era of antifungal therapy. Biol Blood Marrow Transplant 2004;10:73–90.
- Klein RS, Harris CA, Small CB, Moll B, Lesser M, Friedland GH. Oral candidiasis in high risk patients as the initial manifestation of the acquired immunodeficiency syndrome. N Engl J Med 1984;311:354–358.
- Jones DH, Slack R, Squires S, Wooldridge KRH.Antiviral chemotherapy. I. The activity of pyridine and quinoline derivatives against neurovaccinia in mice. J Med Chem 1965;8:676–680.
- Goswami BN, Kataky JCS, Baruah JN. Synthesis and antibacterial activity of 1-(2,4-dichlorobenzoyl)-4-substituted thiosemicarbazides, 1,2,4-triazoles and their methyl derivatives. J Heterocycl Chem 1984;21:1225–1229.
- Holla BS, Kalluraya B, Sridhar KR. Synthesis of 4-(5-nitro-2-furfurylidene)amino-3-mercapto-5-(substituted)-l,2,4-triazoles as possible antibacterial agents. Curr Sci 1987; 56: 236–238.
- Xiaoyun C, Zhang J, Hu H, Yu S, Sun S, Dan Z, Jiang Y, Wu Q. Design, synthesis and biological evaluation of novel triazole derivatives as inhibitors of cytochrome P450 14alpha-demethylase. Eur J Med Chem 2009;44:1913–1920.
- Takuya U, Yoshiko K, Toshiyuki K. Amide analogs of antifungal dioxane-triazole derivatives: Synthesis and in vitro activities. Bioorganic and medicinal chemistry letters 2009;19:2013–2017.
- Bastikar VA, Fulsundar SR, Nair JS. In silico docking analysis of peptide deformylase(PDF)- A novel target for prophylaxis of leptospirosis. Nature Precedings 2008.
- Reena G, Subhash A, Sudhir AK. Modelling and interactions of Aspergillus fumigatus lanosterol 14-alpha demethylase “A” with azole antifungals. Bioorg Med Chem 2004;12:2937–2950.
- Subhash A, Sudhir AK. A dual response partial least squares regression QSAR model and its applications in design of dual activators of PPARα and PPAR γ. QSAR and combinatorial science 2008;27(11–12):1291–1304.
- Hall LH, Mohney BK, Kier LB. The electrotopological state: structure information at the atomic level for molecular graphs. J Chem Inf Comput Sci 1991;31:76–82.
- Baumann K. An alignment-independent versatile structure descriptor for QSAR and QSPR based on the distribution of molecular features. J Chem Inf Comput Sci 2002;42:26–35.