Abstract
The cyclooxygenase-2 (COX-2) inhibitory activity of 2-(4-methylsulphonylphenyl)pyrimidine derivatives has been quantitatively analyzed in terms of Dragon descriptors. The derived QSAR models have provided rationales to explain the activity of titled derivatives. The descriptors (Me, Mp, GATS1p and GATS5p) identified in CP-MLR analysis have highlighted the role of atomic properties, such as Sanderson electronegativity and polarizability, to explain the inhibitory activity. Additionally, prevalence of aromatic ether functionality (descriptor nRORPh) and certain structural fragments (number of Me groups, C-001; number of H attached to heteroatom, H-050 and number of H attached to α-C, H-051) in a molecular structure are helpful to rationalize the COX-2 inhibitory activity of pyrimidine derivatives. The partial least square (PLS) analysis has also confirmed the dominance of information content of CP-MLR-identified descriptors for modelling the activity.
Introduction
The selective inhibitors of cyclooxygenase-2 (COX-2) enzyme have come into their existence with a hope of offering clinical efficacy and reduced ulcerogenicity as compared with traditional non-steroidal anti-inflammatory drugs (NSAIDs)Citation1–8. The withdrawal of rofecoxib (Vioxx)Citation9, a selective COX-2 inhibitor, due to increased cardiovascular riskCitation10 has raised the concern for the safety of COX-2 inhibitorsCitation11–17. The other selective inhibitors of COX-2 enzyme are celecoxibCitation18, valdecoxibCitation19,Citation20 and etoricoxibCitation21–23. The follow-up studies of this class of compoundsCitation24–29 have pointed out that the mechanism of action as probable cause of adverse effectsCitation30,Citation31. Moreover, the traditional NSAIDs are not devoid of toxic effects on cardiovascular systemCitation32,Citation33. As a further development in COX-2 inhibitors, recently a series of pyrimidine derivatives has been reported as potent and highly selective onesCitation34. In view of the importance of anti-inflammatory agents in the clinical management of several disorders, a quantitative structure–activity relationship is attempted on the COX-2 inhibition activity of these pyrimidine derivatives. Ligand-based pharmacophores and atom-based 3D-QSAR model and Hansch analysis on similar type of inhibitors have also been reportedCitation35,Citation36. The present study is aimed at rationalizing the substituent variations of pyrimidine analogues to provide insight for the future endeavours.
Materials and methods
Dataset and molecular descriptors
The study has involved a chemical structure database of 2-(4-methylsulphonylphenyl)pyrimidine derivatives (), reported by Orjales et al.Citation34 The general structure of these derivatives has been given in . The substitutional variations at different positions of the generalized structure are shown in .
Table 1. Substitutional variations of 2-(4-methylsulphonylphenyl)-pyrimidine derivatives (see for general structure and reference [Citation34] for details).
The compounds were evaluated for their ability to inhibit COX-1 and COX-2, respectively, using the purified enzyme (PE) in ‘in vitro’ and human whole blood (HWB) assays in terms of IC50 (molar concentration for 50% inhibition) values. However, the activity data against COX-1 was reported only for limited number of compounds. Therefore, the activity for the inhibition of COX-2 using HWB has only been considered for present work. For modelling purpose, the IC50, representing the concentration of a compound to bring out 50% inhibition, has been expressed in the form of logarithm of inverse of IC50 (–logIC50 or simply pIC50) on molar basis.
The descriptors, accounting molecular features, for all the reported compounds have been calculated from Dragon softwareCitation37. The input for this software was the energy minimized 3D-structures of the compounds drawn in ChemDraw softwareCitation38. A total number of 501 descriptors corresponding to 0D-, 1D- and 2D-classes of Dragon software have been considered in the present modelling study. Before model development procedure, all those descriptors that are intercorrelated beyond 0.90 and showing a correlation of <0.1 with the biological endpoints (descriptor vs. activity, r < 0.1) were excluded. This procedure has reduced the total descriptors from 501 to 147 as relevant ones to explain the biological actions of titled compounds.
In the dataset compound 44 (R1 = Me, R2 = CF3, R3 = H, R4 = −CH2-2-Me-phenyl, X = NH) showed very low activity. Also, the initial assessment of activity with the all descriptors has suggested that two compounds 50 (R1 = Me, R2 = CF3, R3 = H, R4 = −CH2-4-OMe-phenyl, X = NH) and 55 (R1 = Me, R2 = CF3, R3 = H, R4 = −CH2-3-Me-thiophen-2-yl, X = NH) as potential outliers. In these compounds, in R4 moiety any substitution close to the linker position adversely affected the activity (). Compound 44 is an example of this situation. Compounds 50 and 55 also partly come under this situation. But, unlike compound 44, the behaviour of compounds 50 and 55 may be due to more factors. In view of this, the modelling study has been carried out without these three analogues. Inclusion of these three analogues to the dataset leads to statistically insignificant results.
Development and validation of model
The combinatorial protocol in multiple linear regression (CP-MLR)Citation39 and partial least squares (PLS)Citation40–42 procedures have been used in the present study for developing QSAR models. The CP-MLR is a ‘filter’-based variable selection procedure, which employs a combinatorial strategy with MLR to result in selected subset regressions for the extraction of diverse structure–activity models, each having unique combination of descriptors from the generated dataset of the compounds under study. The embedded filters make the variable selection process efficient and lead to unique solution. Further, to find out any chance correlations associated with the models recognized in CP-MLR, each cross-validated model has been subjected to randomization testCitation43,Citation44 by repeated randomization (100 simulation runs) of the biological responses. The datasets with randomized response vector have been reassessed by multiple regression analysis. The resulting regression equations, if any, with correlation coefficients better than or equal to the one corresponding to unscrambled response data were counted. This has been used as a measure to express the percent chance correlation of the model under scrutiny.
Validation of the derived model is necessary to test its prediction and generalization within the study domain. The dataset of the present study is randomly divided into training set for model development and test set for external prediction or validation. The compounds of test set were randomly picked using an in-house written randomization programme.
For each model, derived by involving n data points, a number of statistical parameters such as r (the multiple correlation coefficient), s (the standard deviation), F (the F-ratio between the variances of calculated and observed activities) and Q2LOO (the cross-validated index from leave-one-out procedure) have been obtained to access its overall statistical significance. In case of internal validation, Q2LOO is used as a criterion of both robustness and predictive ability of the model. A value greater than 0.5 of Q2-index suggests a statistically significant model. The predictive power of derived model is based on test set compounds. The model obtained from training set has a reliable predictive power if the value of the r2Test (the squared correlation coefficient between the observed and predicted values of compounds from test set) is greater than 0.5.
Applicability domain
The usefulness of a model is based on its accurate prediction ability for new congeners. A model is valid only within its training domain and new compounds must be assessed as belonging to the domain before the model is applied. The applicability domain is evaluated by the leverage values for each compoundCitation45. A Williams plot (the plot of standardized residuals vs. leverage values (h)) is constructed, which can be used for a simple graphical detection of both the response outliers (Y outliers) and structurally influential chemicals (X outliers) in the model. In this plot, the applicability domain is established inside a squared area within ±x standard deviations and a leverage threshold h* that is generally fixed at 3(k + 1)/n (n is the number of training set compounds and k is the number of model parameters), whereas x = 2 or 3. If the compounds have a high leverage value (h > h*), then the prediction is not trustworthy. On the other hand, when the leverage value of a compound is lower than the threshold value, the probability of accordance between predicted and observed values is as high as that for the training set compounds.
Results and discussion
QSAR results
In multi-descriptor class environment, exploring for best model equation(s) along the descriptor class provides an opportunity to unravel the phenomenon under investigation. In other words, the concepts embedded in the descriptor classes relate the biological actions revealed by the compounds. A total number of 147 significant descriptors from 0D-, 1D- and 2D-classes have been subjected to CP-MLR analysis with default ‘filters’ set in it. Statistical models in two, three and four descriptor(s) have been derived successively to achieve the best relationship correlating COX-2 inhibitory activity. These models along with their statistical parameters are given in .
Table 2. Models derived in two, three and four descriptors and the statistical parameters.
The signs of the regression coefficients suggest the direction of influence of explanatory variables in the models. These models (with 147 descriptors) were identified in CP-MLR by successively incrementing the filter-3 with increasing number of descriptors (per equation). For this, the optimum r-bar value of the preceding level model has been used as the new threshold of filter-3 for the next generation.
The descriptors Ss, Se, Mv and Mp emerged in models () are the constitutional descriptors. These descriptors reflect upon the molecular composition of a compound without any information about its molecular geometry. The descriptor Ss is the sum of Kier–Hall electrotopological states, Se is the sum of atomic Sanderson electronegativities (scaled on carbon atom), Mv is the mean atomic van der Waals volume (scaled on carbon atom) and Mp is the mean atomic polarizability (scaled on carbon atom). The descriptors Ss and Se contribute negatively to the activity, whereas Mv and Mp have shown positive contribution to the COX-2 inhibitory activity. Thus a lower value of descriptors Ss and Se and a higher value of descriptors Mv and Mp would be favourable to the activity.
The descriptors X0Av and Rww are topological descriptors. These descriptors are based on a graph representation of the molecule and are numerical quantifiers of molecular topology obtained by the application of algebraic operators to matrices representing molecular graphs and whose values are independent of vertex numbering or labelling. They can be sensitive to one or more structural features of the molecule such as size, shape, symmetry, branching and cyclicity and can also encode chemical information concerning atom type and bond multiplicity. The sign of regression coefficient of descriptor X0Av, the average valence connectivity index of zero order (chi-0) advocates that a higher positive value of it is supportive to the activity, whereas a higher positive value of descriptor Rww, the reciprocal hyper-detour index would be detrimental to the activity.
The descriptors nRSR, nRORPh and nCp are the functional class descriptors. These are simple molecular descriptors defined as the number of specific functional groups in a molecule. They are calculated by knowing the molecular composition and atom connectivities. All the descriptors participated in models from this class have shown positive correlation to the activity and recommend a higher number of sulphurs (descriptor nRSR), aromatic ethers (descriptor nRORPh) and total primary carbon atoms (descriptor nCp) functionality in a molecule for elevated inhibitory activity.
The descriptor H-051 belongs to atom-centred fragments. Atom-centred fragments are simple molecular descriptors defined as the number of specific atom types in a molecule and their calculation is based on the knowledge of the molecular composition and atom connectivities. The sign of regression coefficient of participated descriptor H-051, hydrogen attached to α-carbon atom, revealed negative influence of it on the activity. Thus a more number of such hydrogen atoms are venomous to the activity.
Descriptors MATS1e, MATS2e, MATS1v, GATS1p and GATS5p emerged in models of are 2D-autocorrelations. 2D-autocorrelations are molecular descriptors that describe how a considered property is distributed along a topological molecular structure. The 2D-AUTO descriptors have their origin in autocorrelation of topological structure of Broto-Moreau (ATS), of Moran (MATS) and of Geary (GATS). The computation of these descriptors involve the summations of different autocorrelation functions corresponding to the different fragment lengths and lead to different autocorrelation vectors corresponding to the lengths of the structural fragments. Also a weighting component in terms of a physicochemical property has been embedded in this descriptor. As a result, these descriptors address the topology of the structure or parts thereof in association with a selected physicochemical property. In these descriptors’ nomenclature, the penultimate character, a number, indicates the number of consecutively connected edges considered in its computation and is called as the autocorrelation vector of lag n (corresponding to the number of edges in the unit fragment). The very last character of the descriptor’s nomenclature indicates the physicochemical property considered in the weighting component—m for mass or v for volume or e for Sanderson electronegativity or p for polarizability—for its computation. It is evident from the signs of regression coefficients of the participating descriptors that except descriptor MATS1e from this class all have contributed negatively to the activity. Thus a higher positive value of descriptor MATS1e (Moran autocorrelation of lag 1 weighted by atomic Sanderson electronegativities) and a lower positive value of descriptors MATS2e (Moran autocorrelation of lag 1 weighted by atomic Sanderson electronegativities), MATS1v (Moran autocorrelation of lag 1 weighted by atomic van der Waals volumes), GATS1p and GATS5p (Geary autocorrelation of lag 1 and 5, respectively, weighted by atomic polarizabilities) will be in favour of activity. The participation of these descriptors thus shows the role of atomic properties in respective lags to explain the biological action of the titled compounds.
Descriptors BEHm3 and BEHp2 are the BCUT descriptors. The BCUT descriptors are the first eight highest and the lowest absolute eigenvalues, BEHwk and BELwk, respectively, for the modified Burden adjacency matrix. Here w refers to the atomic property and k to the eigenvalue rank. The ordered sequence of the highest and the lowest eigenvalues reflect upon the relevant aspects of molecular structure, useful for similarity searching. The positive regression coefficient of the descriptor BEHm3 (the third highest eigenvalue of the Burden matrix weighted by atomic masses) signifies that a higher value of it is supportive to the activity. On the other hand, the negative influence of descriptor BEHp2 (the second highest eigenvalues n.2 of Burden matrix weighted by atomic polarizabilities) on activity suggests that a lower value of this descriptor will augment the activity.
The significance of various emerged models, listed in , may be ascertained through the statistical parameters, the correlation coefficient r, the standard error of the estimate s and the Fisher’s ratio F. Additionally, the cross-validated index Q2, obtained from leave-one-out (LOO) procedure, may assist in identifying the robustness of these models.
In CP-MLR, a search for up to four-parameter models has led to the identification of 54 models. All these models have satisfactorily predicted the test set compounds with r2Test value more than or equal to 0.5 (r2Test ≥ 0.5). Among the identified four-parameter models, three are shown in . All these models shared 54 descriptors among them. These descriptors hold scope for even higher models. Following are the selected five-parameter models evolved from these descriptors:
In above and all follow-up regression equations, the values given in the parentheses are the standard errors of the regression coefficients. The r2randY(sd) is the mean random squared multiple correlation coefficient of the regressions in the activity (Y) randomization study with its standard deviation from 100 simulations. In the randomization study (100 simulations per model), none of the identified models has shown any chance correlation.
In Equation (12), BELm5 is a BCUT class descriptor. The positive sign of its regression coefficient advocates that a higher value of the fifth lowest eigenvalue of the Burden matrix weighted by atomic masses would be beneficiary to the activity. The other descriptors took part in Equations (9) to (12) have similar influence on the inhibitory activity as discussed above (). Considering the number of observation in the dataset, models with up to six descriptors were explored. Following are selected six-parameter models with test set r2 > 0.50.
None of the identified models, mentioned in Equations (13) to (16), has shown any chance correlation in the randomization study (100 simulations per model). The descriptor Me in Equations (13) and (15) is a constitutional class descriptor. It is mean atomic Sanderson electronegativity scaled on carbon atom. Its negative contribution to the activity suggests that a higher value of the mean of the atomic Sanderson electronegativity would be detrimental to activity. The descriptors C-001 (Equations 14 and 16) and H-050 (Equations 13–16) are atom-centred fragments. Descriptor C-001 is representative of CH3R or CH4 type fragment in a molecule, whereas descriptor H-050 correspond to hydrogen attached to hetero atom. The former descriptor has shown positive and the later one negative correlation to the activity. Thus, recommending more number of CH3R or CH4 type fragment and lesser number of hydrogen atoms attached to the hetero atom in a molecular structure for elevated COX-2 inhibitory activity. The remaining descriptors of Equations (13)–(16) convey the same meaning as discussed earlier. The pIC50 values of training set compounds calculated using Equations (13)–(16) and predicted from LOO procedure have been included in .
Table 3. Observed, modelled and predicted (LOO) COX-2 inhibitory activities of training set compounds.
The models (13) to (16) are validated with an external test set of twenty compounds listed in . The predictions of the test set compounds based on external validation are found to be satisfactory as reflected in the test set r2 (r2Test) values.
Table 4. Observed, modelled and residuala COX-2 inhibitory activities of test set compounds.
In the recent past, several groups have introduced a variety of regression diagnostics as a measure of the predictive power of the derived models. Among these, the notable ones are from Golbraikh and TropshaCitation46. They have recommended that the correlation coefficient r between the observed and predicted activities of compounds from an external test set should be close to 1. They have suggested that at least one of the correlation coefficients for regressions through the origin (observed vs. predicted activities, i.e. r02 or predicted vs. observed activities, i.e. r′02) should be close to r2. Furthermore, it is suggested that the slopes associated with these regression lines (k or k′) through the origin should be close to 1. Giving due consideration to all these criteria, it is suggested that the models may be considered acceptable if they show Q2 > 0.5, (ii) r2 > 0.6, (iii) r02 or r′02 is close to r2. In other words, the value of [(r2 − r02)/r2] or [(r2 − r′02)/r2] should be <0.1 and the slopes k or k′ (r02 or r′02 regression lines through origin) should be within the limits of 0.85 to 1.15 (0.85 ≤ k ≤ 1.15 or 0.85 ≤ k′ ≤ 1.15)Citation46. Unfortunately, there were misprints in the formulas mentioned in this highly cited workCitation47. However, the formulas are very common in statistics and can be found in statistics handbookCitation48. The formulas involved in the computation of k, k′, r02 and r′02 values reported for Equations (13)–(16) () are reproduced here under Equations (17–20). In these equations, y and ŷ are, respectively, the observed and predicted activities of test set compounds, and avgy and avgŷ are their mean values.
Table 5. The additional validation statistics for Equations (13–16).
Following Golbraikh and TropshaCitation46 observation, Roy and RoyCitation49 have come up with a regression validation statistics called as rm2, which is a derivative of test set r2 and r02 (the correlation coefficient of regression through the origin for the test set’s observed and predicted activities) (Equation 21). Also, they have suggested that for a model with good external predictability, rm2 value should be greater than 0.5.
In addition to rm2, Roy and coworkers have come up with another statistical measure called as Rp2 (Equation 22) to ensure that the models developed are not obtained by chanceCitation50. In Equation (22), r2 is squared correlation coefficient of non-randomized model, and r2randY is squared mean correlation coefficient of randomized models. They have also suggested that Rp2 should be greater than 0.5 for a model to be acceptable. Subsequent to the publication of RpCitation2,Citation50 Roy and coworkers on the communication of R. Todeschini, Milano Chemometrics, University of Milano-Bicocca, Milano, Italy have corrected its formula (Equation 22) as shown in Equation (23)Citation51.
Apart from the foregoing proposals, recommendations also exist in favour of LOO cross-validation approach alone than to the use of a hold-out test set (external test set) where the dataset modelled is not very largeCitation52. According to Hawkins and coworkers, the drawbacks of holding out a test set may include: (1) structural features of the held out chemicals are not included in the modelling process, resulting in a loss of information, (2) predictions are made on only a subset of the available compounds, whereas LOO predicts the activity value for all compounds, (3) there is no scientific tool that can guarantee similarity between the training and test sets and (4) personal bias can easily be introduced in selection of the external test setCitation52. Considering the academic interests of different researchers, the indices emerged from all above formulas (Equations 17–23) are shown for the regression equations (13)–(16) ().
For Equations (13)–(16), the original validation statistics and coefficients r02, r′02, k, k′ are found to be in the prescribed limits. Also, the R2p and Q2LOO (whole dataset) of these equations are found to be within the prescribed limits. However, rm2, the derivative of test set’s r2 and r02, falls short of the prescribed limit. For these equations, the root mean square error (RMSE) and PRESS, for both the training and the test sets, have also been computed (). By considering all statistical measures together, Equations (13)–(16) have shown reasonable predictive value. The plot showing goodness of fit between observed and calculated activities for the training and test set compounds is given in .
In modelling studies, different equations take into account different sub-structural regions in terms of descriptors of the compound in explaining the predictive and diagnostic aspects of the chosen phenomenon. A study of different sub-structural regions, in terms of descriptors, provides scope to understand the diagnostic aspects of these regions beyond the individual modelsCitation53. Since the Equations (13)–(16) have shown good predictive value in several respects, the identified eight descriptors of the models have been considered for PLS analysis. This analysis facilitates the development of a ‘single window’ structure–activity model and identifies descriptor’s potential in explaining the biological actions of the titled compounds. It also gives an opportunity to make a comparison of relative significance among the descriptors. The fraction contributions obtainable from the normalized regression coefficients of descriptors allow this comparison within modelled activity. For the purpose of PLS, the descriptors have been autoscaled (zero mean and unit s.d.) to give each one of them equal weight in the analysis. In the PLS cross-validation, two components are found to be the optimum for these eight descriptors and they explained 79.9% variance in the activity (r2 = 0.799, Q2LOO = 0.775, s = 0.437, F = 85.740, r2Test = 0.555). The MLR-like PLS coefficients of these eight descriptors are given in .
Table 6. PLS and MLR-like PLS models from the descriptors of six-parameter CP-MLR models for the COX-2 inhibition activity.
For the sake of comparison, the plot showing goodness of fit between observed and calculated activities (through PLS analysis) for the training and test set compounds is also given in . shows a plot of the fraction contribution of normalized regression coefficients of these descriptors to the activity (). The PLS analysis has also suggested nRORPh (a functional class descriptor) as the most determining descriptor for modelling the activity of the compounds (). The other seven descriptors in decreasing order of significance are Me, GATS5p, Mp, H-051, GATS1p, H-050 and C-001. All of these descriptors are part of model equations discussed above and convey same inference in the PLS model as well. It is also observed that PLS model from the dataset devoid of these eight descriptors is inferior in explaining the activity of the analogues.
Applicability domain
On analyzing the model applicability domain (AD) in the Williams plot () of the model based on the whole dataset (, Equations 13a–16a), it has appeared that none of the compounds were identified as an obvious outlier for the COX-2 inhibitory activity if the limit of normal values for the Y outliers (response outliers) was set as 2.5 (standard deviation) units. None of the compounds was found to have leverage (h) values greater than the threshold leverages (h*).
Table 7. The models for the complete data set (n = 66), from the descriptors of training set models, for the COX-2 inhibitory activity of pyrimidine derivatives.
Figure 4. Williams plot for the training set and external prediction set for COX-2 inhibitory activity of pyrimidine derivatives.
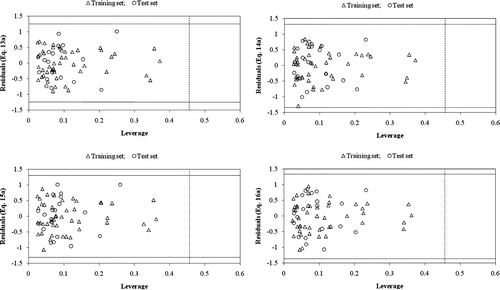
For both the training set and test set, the suggested model matches the high-quality parameters with good fitting power and the capability of assessing external data. Furthermore, almost all of the compounds was within the applicability domain of the proposed model and were evaluated correctly.
Conclusions
The chemical as well as biological properties of compounds are deeply rooted in the topology of chemical structure. The mathematical transformations of the topology of chemical structures offer variety of numerical invariants (descriptors) characteristic of the structures and parts thereof. They offer unique opportunity and scope to investigate the structure–activity relations in terms of simple vertices (0D), chemical bond types (1D) and array of structural fragments (2D). However, the information from pharmacophore-based QSARs get focused around the identified pharmacophores. In this perspective, the QSAR studies guided by the topological features scan the molecules in deriving the structure–activity rationales. Here the information generated may supplement the previously reported QSARs.
The derived QSAR models have provided rationales to explain the COX-2 inhibitory activity of 2-(4-methylsulphonylphenyl)pyrimidine derivatives. The descriptors (Me, Mp, GATS1p and GATS5p) identified in CP-MLR analysis have highlighted the role of atomic properties, such as Sanderson electronegativity and polarizability, to explain the inhibitory activity. Additionally, aromatic ether functionality (descriptor nRORPh) and the structural fragments corresponding to descriptors C-001 (number of Me groups), H-050 (number of H attached to heteroatom) and H-051(number of H attached to α-C) in a molecular structure are also prevalent to rationalize the COX-2 biological actions of pyrimidine derivatives. The PLS analysis has also confirmed the dominance of information content of CP-MLR-identified descriptors.
Acknowledgements
The authors are thankful to their Institutions for providing necessary facilities to complete this study. Drs. Alexander Tropsha and Alexander Golbraikh are thankfully acknowledged for clarifying the formulae involved in computing k, k′, r02 and r′02 coefficients. The anonymous referee is thanked for select references [Citation46,Citation49–51] in the article. CDRI communication number 8071.
Declaration of interest
The authors report no conflicts of interest. The authors alone are responsible for the content and writing of the paper.
References
- Fu JY, Masferrer JL, Seibert K, Raz A, Needleman P. The induction and suppression of prostaglandin H2 synthase (cyclooxygenase) in human monocytes. J Biol Chem 1990;265:16737–16740.
- Xie WL, Chipman JG, Robertson DL, Erikson RL, Simmons DL. Expression of a mitogen-responsive gene encoding prostaglandin synthase is regulated by mRNA splicing. Proc Natl Acad Sci USA 1991;88:2692–2696.
- Hla T, Neilson K. Human cyclooxygenase-2 cDNA. Proc Natl Acad Sci USA 1992;89:7384–7388.
- Kujubu DA, Fletcher BS, Varnum BC, Lim RW, Herschman HR. TIS10, a phorbol ester tumor promoter-inducible mRNA from Swiss 3T3 cells, encodes a novel prostaglandin synthase/cyclooxygenase homologue. J Biol Chem 1991;266:12866–12872.
- Vane J. Towards a better aspirin. Nature 1994;367:215–216.
- Masferrer JL, Zweifel BS, Manning PT, Hauser SD, Leahy KM, Smith WG et al. Selective inhibition of inducible cyclooxygenase 2 in vivo is antiinflammatory and nonulcerogenic. Proc Natl Acad Sci USA 1994;91:3228–3232.
- Vane JR, Bakhle YS, Botting RM. Cyclooxygenases 1 and 2. Annu Rev Pharmacol Toxicol 1998;38:97–120.
- Garavito RM, DeWitt DL. The cyclooxygenase isoforms: structural insights into the conversion of arachidonic acid to prostaglandins. Biochim Biophys Acta 1999;1441:278–287.
- Prasit P, Wang Z, Brideau C, Chan CC, Charleson S, Cromlish W et al. The discovery of rofecoxib [MK 966, Vioxx, 4-(4′-methylsulfonylphenyl)-3-phenyl-2(5H)-furanone], an orally active cyclooxygenase-2-inhibitor. Bioorg Med Chem Lett 1999;9:1773–1778.
- Merck & Co. Press Release; 30 September 2004. http://www.merck.com
- Mukherjee D, Nissen SE, Topol EJ. Risk of cardiovascular events associated with selective COX-2 inhibitors. JAMA 2001;286:954–959.
- Mamdani M, Juurlink DN, Lee DS, Rochon PA, Kopp A, Naglie G et al. Cyclo-oxygenase-2 inhibitors versus non-selective non-steroidal anti-inflammatory drugs and congestive heart failure outcomes in elderly patients: a population-based cohort study. Lancet 2004;363:1751–1756.
- Scheen AJ. [Withdrawal of rofecoxib (Vioxx): what about cardiovascular safety of COX-2 selective non-steroidal anti-inflammatory drugs?]. Rev Med Liege 2004;59:565–569.
- Bresalier RS, Sandler RS, Quan H, Bolognese JA, Oxenius B, Horgan K et al.; Adenomatous Polyp Prevention on Vioxx (APPROVe) Trial Investigators. Cardiovascular events associated with rofecoxib in a colorectal adenoma chemoprevention trial. N Engl J Med 2005;352:1092–1102.
- Solomon SD, McMurray JJ, Pfeffer MA, Wittes J, Fowler R, Finn P et al.; Adenoma Prevention with Celecoxib (APC) Study Investigators. Cardiovascular risk associated with celecoxib in a clinical trial for colorectal adenoma prevention. N Engl J Med 2005;352:1071–1080.
- Fitzgerald GA. Coxibs and cardiovascular disease. N Engl J Med 2004;351:1709–1711.
- Lagakos SW. Time-to-event analyses for long-term treatments—the APPROVe trial. N Engl J Med 2006;355:113–117.
- Penning TD, Talley JJ, Bertenshaw SR, Carter JS, Collins PW, Docter S et al. Synthesis and biological evaluation of the 1,5-diarylpyrazole class of cyclooxygenase-2 inhibitors: identification of 4-[5-(4-methylphenyl)-3-(trifluoromethyl)-1H-pyrazol-1-yl]benzenesulfonamide (SC-58635, celecoxib). J Med Chem 1997;40:1347–1365.
- Talley JJ, Brown DL, Carter JS, Graneto MJ, Koboldt CM, Masferrer JL et al. 4-[5-Methyl-3-phenylisoxazol-4-yl]-benzenesulfonamide, valdecoxib: a potent and selective inhibitor of COX-2. J Med Chem 2000;43:775–777.
- Ormrod D, Wellington K, Wagstaff AJ. Valdecoxib. Drugs 2002;62:2059–2071; discussion 2072.
- Friesen RW, Brideau C, Chan CC, Charleson S, Deschênes D, Dubé D et al. 2-Pyridinyl-3-(4-methylsulfonyl)phenylpyridines: selective and orally active cyclooxygenase-2 inhibitors. Bioorg Med Chem Lett 1998;8:2777–2782.
- Riendeau D, Percival MD, Brideau C, Charleson S, Dubé D, Ethier D et al. Etoricoxib (MK-0663): preclinical profile and comparison with other agents that selectively inhibit cyclooxygenase-2. J Pharmacol Exp Ther 2001;296:558–566.
- Davies IW, Marcoux JF, Corley EG, Journet M, Cai DW, Palucki M et al. A practical synthesis of a COX-2-specific inhibitor. J Org Chem 2000;65:8415–8420.
- Paul AD, Shah E, Richard MM, Peter J. Lessons from the withdrawal of rofecoxib: patients would be safer if drug companies disclosed adverse events before licensing. Br Med J 2004;329:867–868.
- FitzGerald GA. COX-2 and beyond: approaches to prostaglandin inhibition in human disease. Nat Rev Drug Discov 2003;2:879–890.
- Davies NM, Jamali F. COX-2 selective inhibitors cardiac toxicity: getting to the heart of the matter. J Pharm Pharm Sci 2004;7:332–336.
- Chung S, Lim ML, Shin SS. Profiles of COX-2 inhibitors: present and future. Expert Opin Ther Patents 2005;15:9–32.
- Dogné JM, Supuran CT, Pratico D. Adverse cardiovascular effects of the coxibs. J Med Chem 2005;48:2251–2257.
- Dogné JM, Hanson J, Supuran C, Pratico D. Coxibs and cardiovascular side-effects: from light to shadow. Curr Pharm Des 2006;12:971–975.
- Grosser T, Fries S, FitzGerald GA. Biological basis for the cardiovascular consequences of COX-2 inhibition: therapeutic challenges and opportunities. J Clin Invest 2006;116:4–15.
- Sohn HY, Krötz F. Cyclooxygenase inhibition and atherothrombosis. Curr Drug Targets 2006;7:1275–1284.
- Maillard M, Burnier M. Comparative cardiovascular safety of traditional nonsteroidal anti-inflammatory drugs. Expert Opin Drug Saf 2006;5:83–94.
- Hermann M, Ruschitzka F. Coxibs, non-steroidal anti-inflammatory drugs and cardiovascular risk. Intern Med J 2006;36:308–319.
- Orjales A, Mosquera R, López B, Olivera R, Labeaga L, Núñez MT. Novel 2-(4-methylsulfonylphenyl)pyrimidine derivatives as highly potent and specific COX-2 inhibitors. Bioorg Med Chem 2008;16:2183–2199.
- Shah UA, Deokar HS, Kadam SS, Kulkarni VM. Pharmacophore generation and atom-based 3D-QSAR of novel 2-(4-methylsulfonylphenyl)pyrimidines as COX-2 inhibitors. Mol Divers 2010;14:559–568.
- Khare A, Trivedi S, Rajak H, Pawar RS, Patil UK, Singour PK. Hansch analysis of novel pyrimidine derivatives as highly potent and specific COX-2 inhibitors. Med Chem Res. DOI: 10.1007/s00044-011–9566-8.
- Todeschini R, Consonni V. Dragon Software (version 1.11–2001). Milano, Italy.
- Chemdraw Ultra 6.0 and Chem3D Ultra, Cambridge Soft Corporation, Cambridge, USA.
- Prabhakar YS. A combinatorial approach to the variable selection in multiple linear regression: analysis of Selwood et al. Data Set—a case study. QSAR Comb Sci 2003;22:583–595.
- Wold S. Cross-validatory estimation of the number of components in factor and principal components models. Technometrics 1978;20:397–405.
- Kettaneh N, Berglund A, Wold S. PCA and PLS with very large data sets. Comput Stat Data Anal 2005;48:69–85.
- Stahle L, Wold S. Multivariate data analysis and experimental design, In: Ellis GP, West WB, eds. Biomedical Research, Progress in Medicinal Chemistry. Elsevier Science Publishers, BV,vol. 25, 1998, pp. 291–338.
- So SS, Karplus M. Three-dimensional quantitative structure-activity relationships from molecular similarity matrices and genetic neural networks. 1. Method and validations. J Med Chem 1997;40:4347–4359.
- Prabhakar YS, Solomon VR, Rawal RK, Gupta MK, Katti SB. CP-MLR/PLS directed structure–activity modeling of the HIV-1 RT inhibitory activity of 2,3-diaryl-1,3-thiazolidin-4-ones. QSAR Comb Sci 2004;23:234–244.
- Gramatica P. Principles of QSAR models validation: internal and external. QSAR Comb Sci 2007;26:694–701.
- Golbraikh A, Tropsha A. Beware of q2! J Mol Graph Model 2002;20:269–276.
- Tropsha A, Golbraikh A. UNC Eshelman School of Pharmacy, University of North Carolina at Chapel Hill, Chapel Hill, NC 27599, USA (personal communication), 2011.
- Sachs L. Applied Statistics. A Handbook of Techniques. Springer-Verlag, Berlin, 1982.
- Roy PP, Roy K. On some aspects of variable selection for partial least squares regression models. QSAR Comb Sci 2008;27:302–313.
- Pratim Roy P, Paul S, Mitra I, Roy K. On two novel parameters for validation of predictive QSAR models. Molecules 2009;14:1660–1701.
- Mitra I, Saha A, Roy K. Exploring quantitative structure–activity relationship studies of antioxidant phenolic compounds obtained from traditional Chinese medicinal plants. Mol Simulat 2010;36:1067–1079.
- Hawkins DM, Basak SC, Mills D. Assessing model fit by cross-validation. j Chem Inf Comput Sci 2003;43:579–586.
- Gupta MK, Prabhakar YS. Topological descriptors in modeling the antimalarial activity of 4-(3′,5′-disubstituted anilino)quinolines. J Chem Inf Model 2006;46:93–102.